A nomogram for intraoperatively predicting non-sentinel lymph node metastases in early breast cancer patients with positive sentinel lymph nodes
Highlight box
Key findings
• In this study, we developed and validated a nomogram based on the clinical characteristics and inflammatory indicators related to non-sentinel lymph node (non-SLN) metastasis to predict the risk of non-SLN metastases in early-stage breast cancer patients with positive SLNs. This nomogram showed high predictive performance for non-SLN metastases.
What is known and what is new?
• Patients with 1–2 SLN metastases who have undergone breast-conserving surgery and are scheduled to receive postoperative systemic therapy and radiation therapy do not require axillary lymph node dissection (ALND).
• The nomogram used preoperative and intraoperative indicators instead of postoperative information, such as vascular cancer embolism, and maintained a high performance for the prediction of non-SLN metastases.
What is the implication, and what should change now?
• The nomogram developed and validated in this study could assist clinicians in deciding whether to perform ALND in early-stage patients with positive SLNs regardless of whether they meet the Z-0011 trial criteria.
Introduction
Axillary lymph node status is a key prognostic factor affecting overall survival (OS) in breast cancer patients (1). In the past, 60% of breast patients staged N0 after routine axillary lymph node dissection (ALND), and several complications frequently occurred following ALND, including lymphedema, neuralgia, as well as limited movement in the shoulder and arm (2,3). Therefore, identifying patients who require ALND is necessary. Sentinel lymph node biopsy (SLNB), which was recommended by the National Comprehensive Cancer Network (NCCN), has been widely confirmed as a standard procedure for patients without positive SLNs instead of ALND (4,5).
Following the development of adjuvant therapy, the management of early breast cancer (cT1-2N0M0) entered a new era. The American College of Surgeons Oncology Group Z-0011 trial further changed the status of ALND. Early-stage patients with SLNB and standard adjuvant therapy, even if harboring a few involved sentinel lymph nodes (SLNs), could still obtain a favorable prognosis (6). Thus, patients with 1 to 2 SLN metastases who have undergone breast-conserving surgery and are scheduled to receive postoperative systemic therapy and radiation therapy would suffer less ALND and fewer complications. However, the necessity of complete ALND remains debatable in patients who receive total mastectomy and are willing to undergo radiotherapy or breast-conserving surgery with three or more SLN metastases. A tool to determine which patients cannot omit ALND may be needed, especially for patients who do not meet the Z-0011 trial criteria.
At present, only 50% of patients with positive SLNs have additional positive lymph nodes after complete ALND (2). These patients hardly benefit from complete ALND. Therefore, models have been developed to predict the risk of additional non-SLN metastases (7-9), among which the Memorial Sloan-Kettering cancer center (MSKCC) nomogram is the most representative (7). However, these models still have limitations. For instance, several postoperative pathologic characteristics were included in these nomograms, indicating that their use in intraoperative surgical volume decisions is difficult.
Inflammatory cells and mediators play a significant role in cancer progression, such as breast cancer (10). Recently, some combined inflammatory indicators have been reported to be associated with worse prognostic outcomes in various cancers (11). These inflammatory indicators have been applied to predict the recurrence, metastasis, prognosis, adjuvant therapy response, and molecular subtypes in breast cancer (12-16). A meta-analysis showed there were significant differences in the incidence of high levels of platelet-to-lymphocyte ratio (PLR) between patients with and without lymph nodes metastases [odds ratio (OR) =4.24, 95% confidence interval (CI): 2.73–6.59, Tau2<0.001, and I2=0.0%] (17). Yang et al. validated that the high level of PLR and vascular tumor thrombus are risk factors for SLN metastases (12). These inflammatory indicators may play an important role in the prediction of non-SLNs metastases.
The present study aims to develop and validate a nomogram based on the clinical characteristics and inflammatory indicators related to non-SLN metastasis to predict the risk of non-SLN metastases in patients with positive SLNs. This novel nomogram can help clinicians determine the extent of intraoperative resection in early breast cancer patients with positive SLNs. We present this article in accordance with the TRIPOD reporting checklist (available at https://gs.amegroups.com/article/view/10.21037/gs-22-585/rc).
Methods
Study population
The original study cohort included 489 patients with primary invasive breast cancer who underwent SLNB between December 2014 to August 2021 at Xuanwu Hospital, Capital Medical University. Our study population met the following criteria: (I) early-stage primary invasive breast carcinoma, stage cT1-2N0M0; (II) successful SLNB according to the fluorescence tracer and dye methods; (III) positive SLN intraoperatively; and (IV) completion of ALND with at least 10 nodes examined. Patients who received neoadjuvant chemo/radiotherapy treatment were excluded.
The validation cohort included patients from another treatment group in the same hospital during the same period, with the same inclusion criteria as the original series. A total of 46 patients were included in the external validation of the performance of the predictive tool.
The study was conducted in accordance with the Declaration of Helsinki (as revised in 2013). The study was approved by the Ethics Committee of Xuanwu Hospital, Capital Medical University [No. (2020)009]. Individual consent for this retrospective analysis was waived, and the patient data were kept confidential.
SLNB procedure
SLNB was performed intraoperatively with a fluorescence tracer and nano-carbon dye. Before surgery, the nano-carbon dye was injected around the areola of the breast. After sterilization of the operating area, indocyanine green was injected into the subareolar tissue. After 2 to 5 minutes of massage, subcutaneous lymphatic drainage was observed on fluorescent images. Lymph nodes that were fluorescent or stained with the dye were accepted as SLNs.
The frozen SLN tissue was examined by hematoxylin and eosin (H&E) staining intraoperatively. Subsequently, complete ALND was performed on patients with positive SLNs. Postoperatively, routine H&E analysis was conducted for the remaining SLNs tissues and all additional nodes identified by ALND.
Measure of the tumor size
The resected tumor size was defined according to the widest diameter of the largest tumor, and the maximum section width was measured using calipers during the operation. The tumor size on ultrasound (US) and the pathologic tumor size were reported by authoritative ultrasonographers and pathologists.
Detection of inflammatory indicators in peripheral blood
Peripheral blood samples were collected before surgery, and the number of lymphocytes, neutrophil-to-lymphocyte ratio (NLR), PLR, systemic inflammation response index (SIRI), systemic immune-inflammation index (SII), and lymphocyte-to-monocyte ratio (LMR) were calculated according to the preoperative blood routine examination results.
Data processing
This was a retrospective study, and data were collected from the electronic medical record. Univariate analysis and the forward stepwise likelihood ratio method were applied to reduce the included independent variables to obtain stable regression coefficients in the logistic regression.
For the continuous variables, we excluded independent variables with extreme and missing values, preventing interference with the results. Furthermore, due to the study grouping, blinding for study subjects and medical staff was not available. Thus, we also excluded sensitive information, ensuring that the statistical analysts were blinded.
Statistical methods
A univariate analysis was performed to determine the risk factors associated with non-SLN metastases in patients with positive SLNs. The chi-square test or Fisher’s exact test was used for categorical variables, and the continuous variables were analyzed by Student’s t-test or the Mann-Whitney U test, as appropriate.
To identify the predictive factors of non-SLN metastases, all variables with a P value <0.1 in the univariate analysis were analyzed by multivariable logistic regression, using a forward stepwise likelihood ratio method. Only preoperative and intraoperative variables were considered in our regression. A nomogram model for analyzing the risk of non-SLN metastases was created based on the R package “rms” (version 6.2-0; Frank E. Harrell Jr, Email: fh@fharrell.com). The length of the line in the nomogram reflected the contribution of each factor to non-SLN metastases. The risk score was calculated via the R package “nomogramFormula” (version 1.2.0.0; Jing Zhang, Email: zj391120@163.com). The calibration curve was used to examine the predictive capability of the nomogram. The goodness-of-fit of the nomogram model was evaluated with the Hosmer-Lemeshow test using the R Package “ResourceSelection” (version 0.3-5; Peter Solymos, Email: solymos@ualberta.ca).
The nomogram model was then constructed. The accuracy of the models was measured using the area under the receiver operating characteristic (ROC) curve (AUC) with the R Package “pROC” (version 1.17.0.1; Xavier Robin, Email: pROC-cran@xavier.robin.name). The likelihood of non-SLN metastasis in the MSKCC model was obtained based on an online calculation tool (http://www.nomograms.org). The clinical decision value between the nomogram and MSKCC models was compared by decision curve analysis (DCA) using the R Package “rmda” (version 1.6; Marshall Brown, Email: mdbrown@fredhutch.org). The population prevalence of this case-control study was set to 50% based on previous research (2). A 10-fold cross-validation with 400 times repetitions was performed for training and testing our model caret via the R Package “caret” (version 6.0-88; Max Kuhn, Email: mxkuhn@gmail.com). Validation data were calculated by the nomogram, and its accuracy was expressed by the AUC.
Data analyses were performed using R software (version 4.4.1, R Foundation for Statistical Computing). In this study, a two-sided P value <0.05 was considered statistically significant.
Results
Patient characteristics
The original cohort enrolled 489 female breast cancer patients who underwent SLNB between December 2014 and August 2021. Among them, 96 patients (19.6%) were diagnosed as having positive SLNs during operation, including one patient with micrometastasis. The false negative rate in the frozen section was 0.41%, and two patients were reoperated after intraoperative false negative. Based on the exclusion criteria, a total of 83 patients were included in this study, and non-SLN metastases were observed in 42 of these patients (50.6%). The mean age of the patients was 56.4 (range, 33–87) years. The median size of the tumor was 2.00 (range, 1.50–2.65) cm. The average number of removed SLNs per single patient was 3±1.33. The clinical characteristics of the original and validation cohorts are presented in Tables 1,2. All of the patients were Chinese women.
Table 1
Variables | Non-SLN | All patients (n=83) | P value | |
---|---|---|---|---|
Negative (n=41) | Positive (n=42) | |||
Age (years) | 0.098 | |||
≤65 | 29 (70.7) | 36 (85.7) | 65 (78.3) | |
>65 | 12 (29.3) | 6 (14.3) | 18 (21.7) | |
BMI (kg/m2) | 25.00±4.24 | 24.21±3.05 | 24.59±3.67 | 0.221 |
Type of surgery | 0.006 | |||
Breast conserving surgery | 17 (41.5) | 6 (14.3) | 23 (27.7) | |
Mastectomy | 24 (58.5) | 36 (85.7) | 60 (72.3) | |
Tumor size in US (cm) | 1.90 (1.50, 2.20) | 1.80 (1.40, 2.50) | 1.85 (1.50, 2.35) | 0.863 |
Clinical T stage | 0.291 | |||
1 | 28 (53.8) | 13 (41.9) | 41 (49.4) | |
2 | 24 (46.2) | 18 (58.1) | 42 (50.6) | |
No. of positive SLN | 1.0 (1.0, 1.0) | 1.0 (1.0, 2.0) | 1.0 (1.0, 2.0) | 0.002 |
No. of negative SLN | 2.0 (1.0, 3.0) | 1.0 (0.0, 2.0) | 1.0 (0.0, 2.0) | 0.000 |
No. of positive non-SLN | – | 4.5 (1.0, 9.0) | – | – |
Resected positive SLN size (cm) | 1.48±0.52 | 1.58±0.60 | 1.53±0.56 | 0.399 |
Resected tumor size (cm) | 2.00 (1.80, 2.50) | 2.50 (2.10, 3.30) | 2.20 (1.80, 3.00) | 0.004 |
Pathologic tumor size (cm) | 1.80 (1.50, 2.10) | 2.00 (1.60, 3.00) | 2.00 (1.50, 2.65) | 0.071 |
Histopathological grade | 0.645 | |||
Ductal, II | 32 (78.0) | 29 (69.0) | 61 (73.5) | |
Ductal, III | 6 (14.6) | 9 (21.4) | 15 (18.1) | |
Other | 3 (7.3) | 4 (9.5) | 7 (8.4) | |
Multifocal | 0.971 | |||
No | 37 (90.2) | 38 (90.5) | 75 (90.4) | |
Yes | 4 (9.8) | 4 (9.5) | 8 (9.6) | |
LVI | 0.086 | |||
No | 29 (70.7) | 22 (52.4) | 51 (61.4) | |
Yes | 12 (29.3) | 20 (47.6) | 32 (38.6) | |
ER status | 0.591 | |||
No | 3 (7.3) | 1 (2.4) | 4 (4.8) | |
Yes | 38 (92.7) | 41 (97.6) | 79 (95.2) | |
PR status | 0.591 | |||
No | 6 (14.6) | 8 (19.0) | 14 (16.9) | |
Yes | 35 (85.4) | 34 (81.0) | 69 (83.1) | |
Her-2 | 0.722 | |||
No | 28 (80.0) | 26 (76.5) | 54 (78.3) | |
Yes | 7 (20.0) | 8 (23.5) | 15 (21.7) | |
Ki-67 | 25.0 (15.0, 30.0) | 30.0 (20.0, 30.0) | 25.0 (15.0, 30.0) | 0.488 |
History of menopause | 0.415 | |||
No | 14 (34.1) | 18 (42.9) | 32 (38.6) | |
Yes | 27 (65.9) | 24 (57.1) | 51 (61.4) | |
Family history of breast cancer | 1.000 | |||
No | 38 (92.7) | 38 (90.5) | 76 (91.6) | |
Yes | 3 (7.3) | 4 (9.5) | 7 (8.4) | |
NLR | 1.76 (1.29, 2.30) | 1.79 (1.47, 2.24) | 1.78 (1.35, 2.30) | 0.348 |
PLR | 125.00 (103.48, 149.15) | 131.50 (109.54, 162.14) | 131.31 (107.29, 159.64) | 0.729 |
SIRI, ×109/L | 0.48 (0.39, 0.69) | 0.64 (0.42, 0.81) | 0.54 (0.39, 0.77) | 0.069 |
SII, ×109/L | 436.65 (286.78, 551.88) | 421.47 (347.44, 604.30) | 429.06 (317.89, 580.67) | 0.461 |
LMR | 6.59 (5.26, 7.51) | 5.47 (4.13, 7.04) | 5.91 (4.50, 7.29) | 0.047 |
Platelet count | 246.0 (201.5, 283.0) | 238.0 (212.0, 279.0) | 245.0 (206.0, 286.0) | 0.757 |
Neutrophil count | 3.09 (2.63, 3.98) | 3.34 (2.91, 4.13) | 3.25 (2.65, 4.06) | 0.337 |
Lymphocyte count | 1.86 (1.45, 2.42) | 1.83 (1.42, 2.00) | 1.83 (1.43, 2.31) | 0.582 |
Monocyte count | 0.29 (0.26, 0.34) | 0.32 (0.27, 0.44) | 0.31 (0.26, 0.38) | 0.048 |
Values are presented as n (%) or mean ± SD or median (Q1, Q3). SLN, sentinel lymph node; BMI, body mass index; US, ultrasound; LVI, lymphovascular invasion; ER, estrogen-receptor; PR, progesterone receptor; Her-2, human epidermal growth factor receptor 2; NLR, neutrophil-to-lymphocyte ratio; PLR, platelet-to-lymphocyte ratio; SIRI, systemic inflammation response index; SII, systemic immune-inflammation index; LMR, lymphocyte-to-monocyte ratio; SD, standard deviation.
Table 2
Variables | Non-SLN | All patients (n=46) | |
---|---|---|---|
Negative (n=27) | Positive (n=19) | ||
Age (years) | |||
≤65 | 22 (81.5) | 15 (78.9) | 37 (80.4) |
>65 | 5 (18.5) | 4 (21.1) | 9 (19.6) |
BMI (kg/m2) | 26.03±4.58 | 25.23±3.56 | 25.70±4.16 |
Type of surgery | |||
Breast conserving surgery | 8 (29.6) | 6 (31.6) | 14 (30.4) |
Mastectomy | 19 (70.4) | 13 (68.4) | 32 (69.6) |
Tumor size in US (cm) | 2.00 (1.80, 2.30) | 2.00 (1.50, 2.60) | 2.00 (1.60, 2.30) |
Clinical T stage | |||
1 | 14 (51.9) | 10 (52.6) | 24 (52.2) |
2 | 13 (48.1) | 9 (47.4) | 22 (47.8) |
No. of positive SLN | 1.0 (1.0, 1.0) | 2.0 (1.0, 3.0) | 1.0 (1.0, 2.0) |
No. of negative SLN | 3.0 (2.0, 4.0) | 2.0 (1.0, 3.0) | 2.5 (2.0, 4.0) |
No. of positive non-SLN | – | 2.0 (1.0, 4.5) | – |
Resected tumor size (cm) | 2.3 (1.8, 3.0) | 2.5 (2.0, 3.0) | 2.40 (2.00, 3.00) |
Pathologic tumor size (cm) | 2.0 (1.6, 2.5) | 2.3 (1.6, 3.2) | 2.05 (1.60, 2.50) |
Histopathological grade | |||
Ductal, II | 23 (85.2) | 17 (89.5) | 40 (87.0) |
Ductal, III | 1 (3.7) | 2 (10.5) | 3 (6.5) |
Other | 3 (11.1) | 0 (0.0) | 3 (6.5) |
Multifocal | |||
No | 24 (88.9) | 19 (100.0) | 43 (93.5) |
Yes | 3 (11.1) | 0 (0.0) | 3 (6.5) |
LVI | |||
No | 19 (70.4) | 6 (31.6) | 25 (54.3) |
Yes | 8 (29.6) | 13 (68.4) | 21 (45.7) |
ER status | |||
No | 2 (7.4) | 1 (5.3) | 3 (6.5) |
Yes | 25 (92.6) | 18 (94.7) | 43 (93.5) |
PR status | |||
No | 2 (7.4) | 0 (0.0) | 2 (4.3) |
Yes | 25 (92.6) | 19 (100.0) | 44 (95.7) |
Her-2 | |||
No | 21 (87.5) | 15 (83.3) | 36 (85.7) |
Yes | 3 (12.5) | 3 (16.7) | 6 (14.3) |
Ki-67 | 30.0 (15.0, 40.0) | 25.0 (10.0, 40.0) | 30.0 (15.0, 40.0) |
History of menopause | |||
No | 9 (33.3) | 7 (36.8) | 16 (34.8) |
Yes | 18 (66.7) | 12 (63.2) | 30 (65.2) |
Family history of breast cancer | |||
No | 26 (96.3) | 18 (94.7) | 44 (95.7) |
Yes | 1 (3.7) | 1 (5.3) | 2 (4.3) |
NLR | 2.23 (1.68, 2.42) | 1.64 (1.29, 2.39) | 1.99 (1.38, 2.41) |
PLR | 121.37 (91.23, 176.65) | 124.34 (94.88, 187.08) | 122.86 (94.88, 176.65) |
SIRI, ×109/L | 0.70 (0.49, 0.91) | 0.48 (0.31, 0.86) | 0.64 (0.43, 0.86) |
SII, ×109/L | 459.39 (302.12, 691.08) | 383.32 (293.30, 638.15) | 419.15 (299.08, 674.79) |
LMR | 5.45 (4.51, 6.30) | 5.97 (4.82, 11.27) | 5.46 (4.60, 7.17) |
Platelet count | 223.0 (177.0, 293.0) | 247.0 (202.0, 286.0) | 242.50 (191.0, 289.0) |
Neutrophil count | 3.81 (2.53, 4.57) | 3.37 (2.42, 4.52) | 3.74 (2.53, 4.52) |
Lymphocyte count | 1.7 (1.45, 1.97) | 1.79 (1.54, 2.71) | 1.75 (1.54, 2.16) |
Monocyte count | 0.33 (0.27, 0.40) | 0.30 (0.22, 0.44) | 0.31 (0.25, 0.40) |
Values are presented as n (%) or mean ± SD or median (Q1, Q3). SLN, sentinel lymph node; BMI, body mass index; US, ultrasound; LVI, lymphovascular invasion; ER, estrogen-receptor; PR, progesterone receptor; Her-2, human epidermal growth factor receptor 2; NLR, neutrophil-to-lymphocyte ratio; PLR, platelet-to-lymphocyte ratio; SIRI, systemic inflammation response index; SII, systemic immune-inflammation index; LMR, lymphocyte-to-monocyte ratio; SD, standard deviation.
Univariate and multivariate analyses and identification of risk factors
Univariate analysis demonstrated that ten variables were associated with non-SLN metastases, including age, type of surgery, number of positive SLNs, number of negative SLNs, resected tumor size, pathologic tumor size, lymphovascular invasion (LVI), SIRI, LMR, and monocyte count (all P<0.1, Table 1). The postoperative variables were excluded from the filtered variables. In the multivariable logistic regression analysis, age [odds ratio (OR) =0.171; 95% confidence interval (CI): 0.034 to 0.859; P=0.032], number of positive SLNs (OR =5.067; 95% CI: 1.290 to 19.91; P=0.020), number of negative SLNs (OR =0.550; 95% CI: 0.347 to 0.871; P=0.011), resected tumor size (OR =2.477; 95% CI: 1.052 to 5.830; P=0.038), and monocyte count (OR =1.009; 95% CI: 1.002 to 1.016; P=0.012) were associated with non-SLN metastases (Table 3).
Table 3
Variables | Coefficient | Standard error | Wald | P value | Odds ratio (95% CI) |
---|---|---|---|---|---|
Age (>65 years) | −1.765 | 0.823 | 4.599 | 0.032 | 0.171 (0.034 to 0.859) |
No. of positive SLN | 1.623 | 0.698 | 5.403 | 0.020 | 5.067 (1.290 to 19.91) |
No. of negative SLN | −0.598 | 0.235 | 6.492 | 0.011 | 0.550 (0.347 to 0.871) |
Resected tumor size | 0.907 | 0.437 | 4.31 | 0.038 | 2.477 (1.052 to 5.830) |
Monocyte count | 0.009 | 0.004 | 6.327 | 0.012 | 1.009 (1.002 to 1.016) |
Constant | −4.075 | 1.909 | 4.557 | 0.033 | 0.017 |
CI, confidence interval; SLN, sentinel lymph node.
Development and evaluation of nomogram
As shown in Figure 1, a nomogram model named five-factor was developed. The calibration curve was generated by 1,000 times resample via the bootstrap method for predicting the value of the nomogram for non-SLN metastases. The calibration curve exhibited good consistency between the actual observation and predicted probability (Figure 2). The Hosmer-Lemeshow test also showed non-significant goodness of fit in the nomogram (Chi-square =8.224, P=0.412).
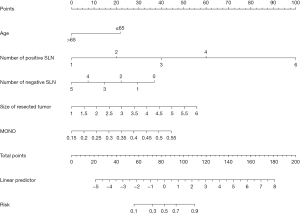
Validation of the nomogram model
The ROC curves were drawn in Figure 3. The five-factor model achieved an AUC of 0.867 (95% CI: 0.788–0.945, Table 4), whereas that of the MSKCC model was 0.754 (95% CI: 0.646–0.862, Table 4).
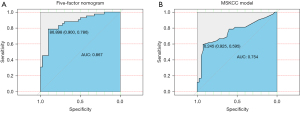
Table 4
Model | AUC | 95% CI | Sensitivity | Specificity |
---|---|---|---|---|
Five-factors | 0.867 | 0.788 to 0.945 | 0.900 | 0.786 |
MSKCC | 0.754 | 0.646 to 0.862 | 0.925 | 0.595 |
External validation | 0.727 | 0.576 to 0.878 | 0.556 | 0.895 |
All without Z-0011 | 0.828 | 0.741 to 0.915 | 0.881 | 0.682 |
All without Z-0011, the patients in original and validation cohort who do not meet the criteria of the Z-0011 trial. ROC, receiver operating characteristic; AUC, area under the receiver operating characteristics curve; CI, confidence interval; MSKCC, Memorial Sloan Kettering Cancer Center.
The DCA of the models was drawn in Figure 4. The net benefit was defined as the benefit of true positives minus the harm of false positives (18). The risk threshold was a reference for treatment decisions, above which ALND would be performed. The model with a gray line represented that all patients would undergo ALND, while that with a black line represented that all patients would not undergo ALND. DCA showed high net benefits in both models among almost all threshold probabilities. The five-factor model exhibited a better clinical effect than the MSKCC model.
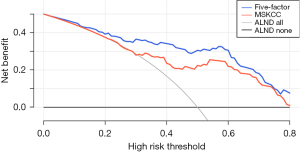
To validate the nomogram model, we used 10-fold cross-validation with 400 times repetitions internally. The AUC of the five-factor nomogram model was still 0.830, indicating a stable predictive effect.
Each patient’s information from the external validation cohort was calculated using the five-factor nomogram to validate the predictive model. The ROC and calibration curves are displayed in Figure 5. The external validation AUC was 0.727 (95% CI: 0.5763–0.8779, Table 4), and the consistency between the actual observation and predicted probability was good. The accuracy of the prediction in patients who did not meet the criteria of the Z-0011 trial was also evaluated; the ROC curve and calibration curves are shown in Figure 6, and the AUC was 0.828 (95% CI: 0.7405–0.9154, Table 4).
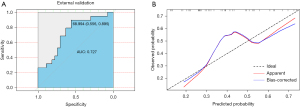
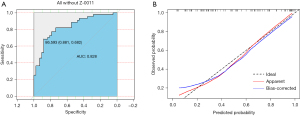
Discussion
After a series of clinical trials, SLNB has already replaced ALND as the standard procedure for patients with negative SLNs (4,19). With the increasing application of SLNB, scholars are paying more attention to patients with one or more positive SLNs. Among these patients, 53% have additional axillary non-SLN metastases, based on the findings of a large meta-analysis (2). This means the remaining patients undergo unnecessary ALND, without therapeutic benefit. A recent large study also validated that the 10-year OS for patients treated with SLNB alone was non-inferior to those treated with ALND, among women with early-stage invasive breast cancer with 1 or 2 SLN metastases (20). These trials confirmed that we may have a chance at further reducing the application of ALND for patients without additional non-SLN metastases.
Although several predictive models have aimed to evaluate the risk of non-SLN metastases, postoperative factors, including pathological tumor size, LVI, and histologic grade, were always included in these models (7-9,21,22). Among these, the nomogram developed by the MSKCC was the representative model, which has been verified in different countries (22-26). In our present study, a five-factor nomogram model was constructed to guide intraoperative decisions, based on a comparison with the MSKCC model and model validation.
According to the ROC curves, both the five-factor model and the MSKCC model exhibited a high predictive capability. The AUC of the MSKCC model was 0.754, which was close to the original MSKCC result of 0.75, which reflected the general adaptability of the model (7). Our five-factor model had a high AUC of 0.867, higher than that of the MSKCC model and other previous models. Thus, we explored the clinical value of the two models. DCA showed more value by examining model performance when there is no consensus regarding the risk threshold of non-SLN metastases (27). Compared with the MSKCC model, our nomogram had a better performance across a range of plausible risk thresholds and can be applied for intraoperative decision-making. After further internal cross-validation and external validation, the five-factor nomogram was confirmed as a reliable tool for intraoperatively predicting the likelihood of non-SLN metastasis in patients with positive SLNs.
The number of positive and negative SLNs were powerful predictive risk factors, which were included in nearly all previously published models (7-9,21,22). In our nomogram, the number of positive SLNs contributed significantly based on the length of the line in Figure 1. Age was also included in previous models as a protective predictor, which is consistent with our findings (21,22). In this study, age was divided into two groups based on the following reasons. On the one hand, neither age >50 years nor menopause status was confirmed as an independent predictor of non-SLN metastases in the previous models (21,22,28). On another hand, older patients would hardly tolerate ALND and its adverse effects, and omitting ALND may not affect their OS benefits. In our model, the size of the resected tumor was chosen as a predictive factor, instead of the pathological tumor size. It is easy for the resected size to be obtained intraoperatively, compared with the pathological size. We observed no significant differences in the resected positive SLN size. The accidental omission of micrometastasis in the frozen section was acceptable.
Local immune response and systemic inflammatory response contribute to tumor growth, invasion, and metastasis (29). The indicators in peripheral blood have been shown to reflect the inflammatory conditions of tumor cells (30). In this study, the LMR and monocyte count were statistically significant. LMR was calculated using the lymphocyte and monocyte counts [lymphocyte count/monocyte count (LMR)]. Lymphocytes play a critical role in tumor immune surveillance and anti-tumor effects (31-33). As for monocytes, different subpopulations may play opposite roles. Monocytes and macrophages are typically the first line of defense against tumor cells (34). However, once tumor cells escape the immune machinery, these cells turn to promote tumorigenesis and tumor progression (10). Goto et al. also found that the ratio of lymphocytes to monocytes is a protective prognostic factor for breast cancer patients (35). However, the mechanism through which the ratio of these indicators may impact breast cancer prognosis remains unclear. We hypothesized further amplifying the inflammatory response’s impact by calculating this ratio. From this point of view, the ratio of the indicators was predicted to be associated with metastasis. Various combinations of inflammatory indicators in peripheral blood may be novel tumor markers. The inflammatory indicators in peripheral blood could provide a cheap and simple method for breast cancer prognostication.
Several limitations to our study should be noted. Firstly, some potential factors may not have been discovered owing to the sample size, and further research with a larger sample size is needed. Secondly, the nomogram is merely an initial exploration with validation. We plan to begin multi-center external verifications to support the accuracy of our model. Thirdly, although the number of positive SLNs was the most powerful predictor, the nomogram could not be used if no SLNs are removed.
Conclusions
At present, there seems to be a tendency to omit ALND in early-stage breast cancer patients. We developed a novel nomogram for predicting non-SLN metastases in early-stage patients using preoperative and intraoperative factors. This nomogram model could assist clinicians to decide whether to perform ALND in early-stage breast cancer patients with positive SLNs.
Acknowledgments
We thank surgeons, patients, and family members who provided clinical information.
Funding: This work was supported by the Beijing Breast Disease Prevention and Treatment Society, Cancer Prevention and Treatment Research Projects, China (No. 2015-8-8).
Footnote
Reporting Checklist: The authors have completed the TRIPOD reporting checklist. Available at https://gs.amegroups.com/article/view/10.21037/gs-22-585/rc
Data Sharing Statement: Available at https://gs.amegroups.com/article/view/10.21037/gs-22-585/dss
Peer Review File: Available at https://gs.amegroups.com/article/view/10.21037/gs-22-585/prf
Conflicts of Interest: All authors have completed the ICMJE uniform disclosure form (available at https://gs.amegroups.com/article/view/10.21037/gs-22-585/coif). The authors have no conflicts of interest to declare.
Ethical Statement:
Open Access Statement: This is an Open Access article distributed in accordance with the Creative Commons Attribution-NonCommercial-NoDerivs 4.0 International License (CC BY-NC-ND 4.0), which permits the non-commercial replication and distribution of the article with the strict proviso that no changes or edits are made and the original work is properly cited (including links to both the formal publication through the relevant DOI and the license). See: https://creativecommons.org/licenses/by-nc-nd/4.0/.
References
- Rao R, Euhus D, Mayo HG, et al. Axillary node interventions in breast cancer: a systematic review. JAMA 2013;310:1385-94. [Crossref] [PubMed]
- Kim T, Giuliano AE, Lyman GH. Lymphatic mapping and sentinel lymph node biopsy in early-stage breast carcinoma: a metaanalysis. Cancer 2006;106:4-16. [Crossref] [PubMed]
- Shaw JH, Rumball EM. Complications and local recurrence following lymphadenectomy. Br J Surg 1990;77:760-4. [Crossref] [PubMed]
- Krag DN, Anderson SJ, Julian TB, et al. Sentinel-lymph-node resection compared with conventional axillary-lymph-node dissection in clinically node-negative patients with breast cancer: overall survival findings from the NSABP B-32 randomised phase 3 trial. Lancet Oncol 2010;11:927-33. [Crossref] [PubMed]
- Gradishar WJ, Moran MS, Abraham J, et al. NCCN Guidelines® Insights: Breast Cancer, Version 4.2021. J Natl Compr Canc Netw 2021;19:484-93. [Crossref] [PubMed]
- Donker M, van Tienhoven G, Straver ME, et al. Radiotherapy or surgery of the axilla after a positive sentinel node in breast cancer (EORTC 10981-22023 AMAROS): a randomised, multicentre, open-label, phase 3 non-inferiority trial. Lancet Oncol 2014;15:1303-10. [Crossref] [PubMed]
- Van Zee KJ, Manasseh DM, Bevilacqua JL, et al. A nomogram for predicting the likelihood of additional nodal metastases in breast cancer patients with a positive sentinel node biopsy. Ann Surg Oncol 2003;10:1140-51. [Crossref] [PubMed]
- Meretoja TJ, Leidenius MH, Heikkilä PS, et al. International multicenter tool to predict the risk of nonsentinel node metastases in breast cancer. J Natl Cancer Inst 2012;104:1888-96. [Crossref] [PubMed]
- Reynders A, Brouckaert O, Smeets A, et al. Prediction of non-sentinel lymph node involvement in breast cancer patients with a positive sentinel lymph node. Breast 2014;23:453-9. [Crossref] [PubMed]
- Mantovani A, Allavena P, Sica A, et al. Cancer-related inflammation. Nature 2008;454:436-44. [Crossref] [PubMed]
- Ethier JL, Desautels D, Templeton A, et al. Prognostic role of neutrophil-to-lymphocyte ratio in breast cancer: a systematic review and meta-analysis. Breast Cancer Res 2017;19:2. [Crossref] [PubMed]
- Yang L, Wang H, Ma J, et al. Association between the platelet to lymphocyte ratio, neutrophil to lymphocyte ratio and axillary lymph node metastasis in cT1N0 breast cancer patients. Am J Transl Res 2021;13:1854-61. [PubMed]
- Zenan H, Zixiong L, Zhicheng Y, et al. Clinical prognostic evaluation of immunocytes in different molecular subtypes of breast cancer. J Cell Physiol 2019;234:20584-602. [Crossref] [PubMed]
- Kim JY, Jung EJ, Kim JM, et al. Dynamic changes of neutrophil-to-lymphocyte ratio and platelet-to-lymphocyte ratio predicts breast cancer prognosis. BMC Cancer 2020;20:1206. [Crossref] [PubMed]
- Graziano V, Grassadonia A, Iezzi L, et al. Combination of peripheral neutrophil-to-lymphocyte ratio and platelet-to-lymphocyte ratio is predictive of pathological complete response after neoadjuvant chemotherapy in breast cancer patients. Breast 2019;44:33-8. [Crossref] [PubMed]
- Vernieri C, Mennitto A, Prisciandaro M, et al. The neutrophil-to-lymphocyte and platelet-to-lymphocyte ratios predict efficacy of platinum-based chemotherapy in patients with metastatic triple negative breast cancer. Sci Rep 2018;8:8703. [Crossref] [PubMed]
- Zhang M, Huang XZ, Song YX, et al. High Platelet-to-Lymphocyte Ratio Predicts Poor Prognosis and Clinicopathological Characteristics in Patients with Breast Cancer: A Meta-Analysis. Biomed Res Int 2017;2017:9503025. [Crossref] [PubMed]
- Van Calster B, Wynants L, Verbeek JFM, et al. Reporting and Interpreting Decision Curve Analysis: A Guide for Investigators. Eur Urol 2018;74:796-804. [Crossref] [PubMed]
- Giuliano AE, Kirgan DM, Guenther JM, et al. Lymphatic mapping and sentinel lymphadenectomy for breast cancer. Ann Surg 1994;220:391-8; discussion 398-401. [Crossref] [PubMed]
- Giuliano AE, Ballman KV, McCall L, et al. Effect of Axillary Dissection vs No Axillary Dissection on 10-Year Overall Survival Among Women With Invasive Breast Cancer and Sentinel Node Metastasis: The ACOSOG Z0011 (Alliance) Randomized Clinical Trial. JAMA 2017;318:918-26. [Crossref] [PubMed]
- Bevilacqua JL, Kattan MW, Fey JV, et al. Doctor, what are my chances of having a positive sentinel node? A validated nomogram for risk estimation. J Clin Oncol 2007;25:3670-9. [Crossref] [PubMed]
- Chen JY, Chen JJ, Yang BL, et al. Predicting sentinel lymph node metastasis in a Chinese breast cancer population: assessment of an existing nomogram and a new predictive nomogram. Breast Cancer Res Treat 2012;135:839-48. [Crossref] [PubMed]
- Specht MC, Kattan MW, Gonen M, et al. Predicting nonsentinel node status after positive sentinel lymph biopsy for breast cancer: clinicians versus nomogram. Ann Surg Oncol 2005;12:654-9. [Crossref] [PubMed]
- Klar M, Foeldi M, Markert S, et al. Good prediction of the likelihood for sentinel lymph node metastasis by using the MSKCC nomogram in a German breast cancer population. Ann Surg Oncol 2009;16:1136-42. [Crossref] [PubMed]
- Cho J, Han W, Lee JW, et al. A scoring system to predict nonsentinel lymph node status in breast cancer patients with metastatic sentinel lymph nodes: a comparison with other scoring systems. Ann Surg Oncol 2008;15:2278-86. [Crossref] [PubMed]
- Gur AS, Unal B, Ozbek U, et al. Validation of breast cancer nomograms for predicting the non-sentinel lymph node metastases after a positive sentinel lymph node biopsy in a multi-center study. Eur J Surg Oncol 2010;36:30-5. [Crossref] [PubMed]
- Kerr KF, Brown MD, Zhu K, et al. Assessing the Clinical Impact of Risk Prediction Models With Decision Curves: Guidance for Correct Interpretation and Appropriate Use. J Clin Oncol 2016;34:2534-40. [Crossref] [PubMed]
- Ishizuka Y, Horimoto Y, Nakamura M, et al. Predictive Factors for Non-sentinel Nodal Metastasis in Patients With Sentinel Lymph Node-positive Breast Cancer. Anticancer Res 2020;40:4405-12. [Crossref] [PubMed]
- Diakos CI, Charles KA, McMillan DC, et al. Cancer-related inflammation and treatment effectiveness. Lancet Oncol 2014;15:e493-503. [Crossref] [PubMed]
- Chen L, Kong X, Yan C, et al. The Research Progress on the Prognostic Value of the Common Hematological Parameters in Peripheral Venous Blood in Breast Cancer. Onco Targets Ther 2020;13:1397-412. [Crossref] [PubMed]
- Denkert C, Loibl S, Noske A, et al. Tumor-associated lymphocytes as an independent predictor of response to neoadjuvant chemotherapy in breast cancer. J Clin Oncol 2010;28:105-13. [Crossref] [PubMed]
- Gregory AD, Houghton AM. Tumor-associated neutrophils: new targets for cancer therapy. Cancer Res 2011;71:2411-6. [Crossref] [PubMed]
- Swann JB, Smyth MJ. Immune surveillance of tumors. J Clin Invest 2007;117:1137-46. [Crossref] [PubMed]
- Gouveia-Fernandes S. Monocytes and Macrophages in Cancer: Unsuspected Roles. Adv Exp Med Biol 2020;1219:161-85. [Crossref] [PubMed]
- Goto W, Kashiwagi S, Asano Y, et al. Predictive value of lymphocyte-to-monocyte ratio in the preoperative setting for progression of patients with breast cancer. BMC Cancer 2018;18:1137. [Crossref] [PubMed]
(English Language Editor: A. Kassem)