Development and validation of a prediction model for the diagnosis of breast cancer based on clinical and ultrasonic features
Highlight box
Key findings
• A prediction model was developed with the clinical and ultrasonic features for the precise and intuitive probability of breast cancer, this could provide a reliable reference for further examination.
What is known and what is new?
• The Breast Imaging Reporting and Data System (BI-RADS) is an important guideline for the evaluation of benign and malignant breast lesions and for further examination and treatment.
• In this study, the malignancy probability of breast lesions can be calculated in real time by selecting relevant parameters, and the predicted probability is a specific value.
What is the implication, and what should change now?
• The inspection process should be optimized after the detection of suspected breast lesions to reduce unnecessary suffering and expense for patients.
Introduction
Breast cancer is the most common cancer and the leading cause of cancer deaths among women in the world. The incidence rate increased by 0.5% annually during the most recent data years, and the age of incidence is trending younger (1). Cases in China accounted for 12.2% of all newly diagnosed breast cancers and 9.6% of all deaths from breast cancer worldwide (2,3). Early detection and diagnosis are particularly crucial for proper treatment and long-term prognosis of breast cancer patients.
The diagnosis methods for benign and malignant breast tumors include mammography, computed tomography (CT), magnetic resonance imaging (MRI) and ultrasound. Mammography has a high sensitivity of up to 85% for the detection of the microcalcification of the breast lesions, so it is usually used for early detection and effective diagnosis of breast cancer with microcalcifications as the only manifestation. However, the sensitivity of mammography is susceptible to breast density and the radiation prevents mammography from being a common screening modality for women under 40 years of age (4,5). CT has certain advantages of observing early metastasis in the lung, chest wall and axillary lymph nodes, but it is not the first choice for breast examination for the low spatial resolution and limited ability to detect small breast lesions (6). With the high resolution of tissues and clarity of dissection structure, MRI is a good modality in the early detection of occult breast lesions (7). However, it is difficult to be widely applied due to its high cost and long examination time.
Ultrasound is one of the common methods for detecting breast cancer. Compared with mammography and MRI, ultrasound has some advantages, such as simplicity, lack of radiation, real-time dynamics, low cost, and the ability to be performed at the bedside, making it play an important role in the screening of breast lesions and the diagnosis of breast cancer. The Breast Imaging Reporting and Data System (BI-RADS) developed by the American College of Radiology (ACR) is an important guideline for the evaluation of benign and malignant breast lesions and for further examination and treatment. This guideline standardized ultrasound diagnostic features of breast lesions (8). However, the current ACR BI-RADS categories predict the malignancy probability for breast tumors with a wide range rather than an exact value. The category 4 predicts 2% to 95% malignant probability of the breast lesion. The category 5 predicts more than 95% malignant probability, which means that lesion is highly suspected as breast cancer. Even though that category 4 is divided into 4A, 4B and 4C, the malignant probability of each is still spanning a relatively wide range. When the breast lesion is diagnosed as category 4 or 5, the patient will be recommended to undergo biopsy and pathological examination according to the guideline (9). However, the positive predictive value of biopsy currently only reaches 42.7%, indicating that the diagnosis and BI-RADS category for the breast lesion are not precise enough. The pathology after biopsy or surgery is still the gold standard for deciding if the lesion is breast cancer or not at present. In addition, although some lesions have been classified as category 4 or 5, they are not suitable for biopsy as recommended by ACR BI-RADS and the patients’ consultation process should be refined. How to diagnose breast cancer in a simple and effective way is the burning issue we need to focus on.
In addition, the occurrence of breast cancer is concerned with the inherited susceptibility, and the ultrasonographic manifestations of breast diseases are varied compare to that of thyroid (10). Besides, intersections exist in the imaging morphology between benign and malignant breast cases in clinical practice. Therefore, it is difficult to differentiate breast cancer only based on ultrasound images, especially for primary physicians who lack of clinical experience. And these are the reasons leading to inconsistent diagnosis between sonographers for BI-RADS category. Based on the reasons above, clinical history and clinical manifestation of patients are crucial for distinguishing benign lesion from breast cancer. For example, when suspicious and tumor-like mass is found in the mammary duct via ultrasound screening, the nature and color of the patient’s nipple discharge will be conducive to the differential diagnosis between pure dilation of the breast ducts and intraductal papilloma. However, sonographers tend to overlook medical history of which most of the time being inquired in person before the ultrasound examination, and the final diagnosis is inaccurate and more subjective (11,12). Consequently, a precise, convenient, and effective tool providing a quantified risk prediction of breast cancer is strongly needed for making a preliminary diagnosis based on clinical and sonographic features. Therefore, this study established and validated a prediction model by least absolute shrinkage and selection operator (LASSO) and logistic regression, with a view to assisting in the clinical diagnosis of breast cancer and providing a more optimized inspection scheme. This article is written following the TRIPOD reporting checklist (available at https://gs.amegroups.com/article/view/10.21037/gs-22-663/rc).
Methods
Study population
The study was conducted in accordance with the Declaration of Helsinki (as revised in 2013) and was approved by the ethics committee of PLA General Hospital (No. S2021-291-01). All patients provided informed consent.
Sample size
No gold-standard approach is currently available for the calculation of the sample size requirements of risk prediction models. However, it is widely accepted that at least 10 events per candidate variable for the logistic regression analysis are needed for the derivation of a risk prediction model (13). As 22 candidate variables were included in the multivariable regression analysis, at least 220 lesions were required for this study.
Development group
Patients diagnosed with breast lesions by ultrasound in the ultrasound department of PLA General Hospital from March 1st, 2020 to April 1st, 2021 were selected. Apart from that, the inclusion criteria were as follows: (I) female aged ≥18 years; (II) patients with complete clinical information, including age, clinical history, and clinical manifestation of breast diseases; (III) patients with clear ultrasonic images of breast, including two-dimensional ultrasonic images, color Doppler images, spectral Doppler images and ultrasonic images of bilateral axillary lymph nodes; (IV) patients with puncture biopsy or surgery after breast ultrasound examination. The following exclusion criteria were used: (I) male patients; (II) patients with incomplete clinical information; (III) patients with unclear or incomplete breast ultrasound images; (IV) patients without an exact pathology. Finally, a total of 402 lesions from 304 patients (mean age, 46±11 years) were included.
Validation group
Patients diagnosed with breast lesions by ultrasound in the physical examination center of PLA General Hospital from April 1st, 2021 to March 1st, 2022 were selected, with the same inclusion and exclusion criteria as the development group. Finally, a total of 121 lesions from 98 patients (mean age, 51±13 years) were included. Flow chart of the study population enrolment are shown in Figure S1.
Medical history inquiring
Prior to the examination of breast ultrasound, the sonographer inquired the patients about their clinical history and recorded them, including the family history of breast cancer, history of benign breast tumors, as well as clinical manifestations of pain, fever and bloody nipple discharge.
Breast ultrasound examination
Two sonographers with 10 years of experience performed the breast ultrasound examination for the development and validation group. Patients were placed in the supine position with the breast fully exposed for image acquisition firstly, sonographers then performed comprehensive scanning of the patient’s bilateral breasts and armpits, and saved the two- dimensional images, color Doppler images and spectral doppler images for suspicious lesions and axillary lymph nodes in the meantime. In addition, 4–10 MHz linear-array transducers (Philips EPIQ 7, Philips Healthcare, Amsterdam, The Netherlands) were used to conduct ultrasound examinations.
Images analysis
All ultrasonic images of the development and validation group were reviewed by the examining sonographer and a sonographer with more than 15 years of experience who were blinded to the pathology. The two sonographers discussed together to reach an agreement when the sonographers’ opinions differed. To be specific, the descriptions of ultrasound features were based on the ACR descriptions, including size, aspect ratio, shape, margin, uniformity of internal echogenicity, calcification, posterior echogenicity of the lesion, relationship with the breast ducts, structural changes in the tissue surrounding the lesion, internal blood flow of the lesion and axillary lymph nodes were interpretated. Among them, vertical growth direction, irregular shape, irregular border, hypoechoic echo, heterogeneous echo, microcalcification, suspicious microcalcification, attenuation effects, decreased echo in surrounding tissues, lesion in duct, abnormal lymph node morphology, significantly increased blood flow signals, nourishing vessel and the nourishing vessel’ resistance index (RI) >0.70 were sorted as malignant characters. Moreover, the BI-RADS categories of the lesions were given according to the guideline. Subsequently, BI-RADS categories 1–3 of the lesions in both of the development and validation group were sorted as benign cases, and BI-RADS categories 4–6 were sorted as malignant cases.
Follow-up of pathological findings
Pathology was the gold standard for final diagnosis. Inclusive lesions were followed up with surgery or biopsy pathology results. When the lesions’ puncture results were inconsistent with the surgical findings, the surgical findings prevailed. Then, the pathology of each lesion was classified as benign or malignant case. Besides, lesions without pathological findings were excluded.
Statistical analysis
In this study, R soft version 4.0.3 (https://www.r-project.org/, The R Foundation) and “glmnet” package (R Foundation for Statistical Computing, Vienna, Austria) were used for statistical analysis. Additionally, continuous data were expressed as means ± standard deviations or medians with interquartile ranges, while categorical data were presented as percentages and counts. Then, the development and validation groups were compared using independent t-test, Mann-Whitney U-test, and chi-square test, respectively. A P value of <0.05 was set as the significance level. LASSO was adopted as the variable selection method for the prediction model. Additionally, the best lambda value was selected using the 10-fold cross-validation method, which the selection criterion was the maximum lambda value corresponding to the mean error value obtained from ten cross-validations within a minimum of 1 standard deviation (lambda.1se) (Figure 1). Besides, the generalized linear modeling (GLM) and logistic regression were used in the development group to construct the prediction model. The receiver operating characteristic (ROC) was performed to assess the ability of the model. The area under the curve (AUC), sensitivity, specificity, odds ratio (OR) and 95% confidence interval (CI) were calculated. The cut off values were the optimum threshold values of sensitivity and specificity. A P value of <0.05 was set as the significance level. R’s shiny package was applied to build a web version of the interactive dynamic column line graph.
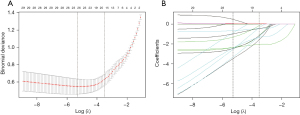
The model performance of the development and validation groups included calibration, discrimination and usefulness evaluation, respectively. Model calibration was conducted by R2, and model discriminant was performed by AUC, which is generally considered to be moderately discriminant between 0.70–0.80 and highly discriminant when AUC >0.80. Apart from that, a decision curve analysis (DCA) was used for usefulness evaluation.
Results
Patients’ characteristics
Patients’ clinical and ultrasound image features are shown in Table 1. A total of 402 breast lesions from 304 patients were included as the development group, with a mean age of 46±11 years. Furthermore, 121 breast lesions from 98 patients were enrolled as the validation group, and the mean age was 51±13 years. Among the lesions, 179 were malignant and 223 were benign in the development group, whereas 62 were malignant and 59 were benign in the validation group. The mean largest diameter of lesions was 12.0 mm [interquartile range (IQR), 8.0–20.0 mm] in the development group, and 15.0 mm [IQR, 10.0–20.0 mm] in the validation group. In the development and validation group, the lesions with family history of breast cancer were 9 (2.2%) vs. 3 (2.5%), lesions with history of the breast benign tumor were 110 (27.4%) vs. 26 (21.5%), lesions with clinical signs of pain were 36 (9.0%) vs. 8 (6.6%), lesions with clinical signs of fever were 1 (0.2%) vs. 0 (0.0%), and lesions with clinical signs of bloody nipple discharge were 18 (4.5%) vs. 7 (5.8%). Among the sonographic features, vertical growth direction of lesions in the development and validation group were 44 (10.9%) vs. 20 (16.5%), lesions with irregular shape were 280 (69.7%) vs. 99 (81.8%), lesions with irregular border were 166 (41.3%) vs. 71 (58.7%), hypoechoic lesions were 235 (58.5%) vs. 114 (94.2%), lesions with heterogeneous echo were 343 (85.3%) vs. 121 (100.0%), lesions with microcalcification were 66 (16.4%) vs. 33 (27.3%), lesions with suspicious microcalcification were 26 (6.5%) vs. 10 (8.3%), lesions with attenuation effects were 120 (29.9%) vs. 31 (25.6%), lesions with decreased echo in surrounding tissues were 17 (4.2%) vs. 13 (10.7%), lesions in duct were 61 (15.2%) vs. 11 (9.1%), lesions with abnormal lymph node morphology were 107 (26.6%) vs. 26 (21.5%), lesions with significantly increased blood flow signals were 87 (21.6%) vs. 52 (43.0%), lesions with nourishing vessel were 133 (33.1%) vs. 32 (26.4%), lesions with nourishing vessel’s RI >0.70 were 67 (16.7%) vs. 16 (13.2%), and malignant lesions were 179 (44.5%) vs. 62 (51.2%).
Table 1
Variable | Development (n=402) | Validation (n=121) | P value |
---|---|---|---|
Age (year), mean ± SD | 46±11 | 51±13 | <0.001*** |
The largest diameter (mm), mean (IQR) | 12.0 (8.0–20.0) | 15.0 (10.0–20.0) | 0.015* |
Family history of breast cancer, n (%) | 0.877 | ||
No | 393 (97.8) | 118 (97.5) | |
Yes | 9 (2.2) | 3 (2.5) | |
History of benign breast tumor, n (%) | 0.196 | ||
No | 292 (72.6) | 95 (78.5) | |
Yes | 110 (27.4) | 26 (21.5) | |
Pain, n (%) | 0.416 | ||
No | 366 (91.0) | 113 (93.4) | |
Yes | 36 (9.0) | 8 (6.6) | |
Fever, n (%) | 0.583 | ||
No | 401 (99.8) | 121 (100.0) | |
Yes | 1 (0.2) | 0 (0.0) | |
Bloody nipple discharge, n (%) | 0.554 | ||
No | 384 (95.5) | 114 (94.2) | |
Yes | 18 (4.5) | 7 (5.8) | |
Vertical growth direction, n (%) | 0.100 | ||
No | 358 (89.1) | 101 (83.5) | |
Yes | 44 (10.9) | 20 (16.5) | |
Irregular shape, n (%) | 0.009** | ||
No | 122 (30.3) | 22 (18.2) | |
Yes | 280 (69.7) | 99 (81.8) | |
Irregular border, n (%) | <0.001*** | ||
No | 236 (58.7) | 50 (41.3) | |
Yes | 166 (41.3) | 71 (58.7) | |
Hypoechoic echo, n (%) | <0.001*** | ||
No | 167 (41.5) | 7 (5.8) | |
Yes | 235 (58.5) | 114 (94.2) | |
Heterogeneous echo, n (%) | <0.001*** | ||
No | 59 (14.7) | 0 (0.0) | |
Yes | 343 (85.3) | 121 (100.0) | |
Microcalcification, n (%) | 0.008** | ||
No | 336 (83.6) | 88 (72.7) | |
Yes | 66 (16.4) | 33 (27.3) | |
Suspicious microcalcification, n (%) | 0.494 | ||
No | 376 (93.5) | 111 (91.7) | |
Yes | 26 (6.5) | 10 (8.3) | |
Attenuation effects, n (%) | 0.368 | ||
No | 282 (70.1) | 90 (74.4) | |
Yes | 120 (29.9) | 31 (25.6) | |
Decreased echo in surrounding tissues, n (%) | 0.007** | ||
No | 385 (95.8) | 108 (89.3) | |
Yes | 17 (4.2) | 13 (10.7) | |
In duct, n (%) | 0.089 | ||
No | 341 (84.8) | 110 (90.9) | |
Yes | 61 (15.2) | 11 (9.1) | |
Abnormal lymph node morphology, n (%) | 0.256 | ||
No | 295 (73.4) | 95 (78.5) | |
Yes | 107 (26.6) | 26 (21.5) | |
Significantly increased blood flow signals, n (%) | <0.001*** | ||
No | 315 (78.4) | 69 (57.0) | |
Yes | 87 (21.6) | 52 (43.0) | |
Nourishing vessel, n (%) | 0.168 | ||
No | 269 (66.9) | 89 (73.6) | |
Yes | 133 (33.1) | 32 (26.4) | |
RI >0.70, n (%) | 0.363 | ||
No | 335 (83.3) | 105 (86.8) | |
Yes | 67 (16.7) | 16 (13.2) | |
Malignant, n (%) | 0.194 | ||
No | 223 (55.5) | 59 (48.8) | |
Yes | 179 (44.5) | 62 (51.2) |
*, P<0.05; **, P<0.01; ***, P<0.001. SD, standard deviation; IQR, interquartile range; RI, resistance index.
Development of the prediction model
Since the study had many variables and relatively few cases, LASSO was applied to pick out the variables most associated with breast cancer, ten-fold cross-validation was utilized to screen the penalty term, 12 variables were selected for further logistic regression analysis finally. Figure 1 displays the screening process of LASSO. The selected independent risk factors were: age, bloody nipple discharge, irregular shape, irregular border, heterogeneous echo, microcalcification, attenuation effects, decreased echo in surrounding tissues, lesions in ducts, abnormal lymph node morphology, and nourishing vessel and nourishing vessel’s RI >0.70 (Table 2). Based on logistic regression analysis, the regression was set as:
Table 2
Variable | Estimate | Std. error | P value | OR | 2.50% | 97.50% |
---|---|---|---|---|---|---|
(Intercept) | −9.39 | 1.55 | <0.001*** | <0.001 | 0 | 0.001 |
Age | 0.06 | 0.02 | 0.001** | 1.060 | 1.02 | 1.09 |
Bloody nipple discharge | 2.06 | 0.85 | 0.016* | 7.870 | 1.51 | 45.28 |
Irregular shape | 2.37 | 0.58 | <0.001*** | 10.660 | 3.70 | 37.05 |
Irregular border | 3.22 | 0.43 | <0.001*** | 25.118 | 11.28 | 60.97 |
Heterogeneous echo | 2.06 | 1.20 | 0.085 | 7.869 | 1.13 | 174.21 |
Microcalcification | 1.96 | 0.65 | 0.003** | 7.124 | 2.11 | 27.77 |
Attenuation effects | 1.06 | 0.44 | 0.015* | 2.879 | 1.23 | 6.89 |
Decreased echo in surrounding tissues | 2.82 | 1.19 | 0.018* | 16.733 | 2.05 | 221.44 |
In duct | 1.42 | 0.50 | 0.004** | 4.170 | 1.58 | 11.45 |
Abnormal lymph node morphology | 0.80 | 0.41 | 0.049* | 2.230 | 1.01 | 5.04 |
Nourishing vessel | 0.22 | 0.48 | 0.647 | 1.248 | 0.48 | 3.24 |
RI >0.70 | 1.25 | 0.63 | 0.047* | 3.482 | 1.03 | 12.25 |
*, P<0.05; **, P<0.01; ***, P<0.001. LASSO, least absolute shrinkage and selection operator; OR, odds ratio; RI, resistance index.
Logit(Y) = −9.3925 + 0.0552 × age + 2.0628 × bloody nipple discharge (yes) + 2.3665 × irregular shape (yes) + 3.2236 × irregular border (yes) + 2.0629 × heterogeneous (yes) + 1.9634 × microcalcification (yes) + 1.0576 × attenuation effects (yes) + 2.8174 × decreased echo in surrounding tissues (yes) + 1.4279 × in duct (yes) + 0.8022 × abnormal lymph node morphology (yes) + 0.2216 × nourishing vessel (yes) + 1.2476 × RI gte 0.70 (yes).
The web-based version of the interactive column line chart based on the shiny package could be used to automatically calculate the diagnostic probability of a patient by visiting the webpage: https://echohx925.shinyapps.io/characteristics_of_breast_lesions/ (Figure 2).
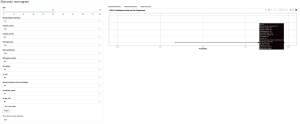
For the development group of the prediction model, the AUC, cut off value, sensitivity, and specificity were 0.959, 0.618, 0.969 and 0.883, respectively. For the validation group of prediction model, the AUC, cut off value, sensitivity, and specificity were 0.952, 0.724, 0.881 and 0.935, respectively (Figure 3). Besides, the AUC of the prediction model’s development group was higher than that of the BI-RADS category’s development group (0.959 vs. 0.953). At the same time, the AUC of the prediction model’s validation group was higher than that of the BI-RADS category’s validation group (0.952 vs. 0.932) (Figures 3,4). However, no significant difference was observed in AUC between the model’s development group and the BI-RADS category’s development group (P=0.531, Z=0.626). Likewise, no significant difference was found between the model’s validation group and the BI-RADS category’s validation group (P=0.356, Z=0.923) (Table 3).
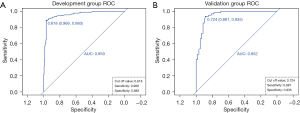
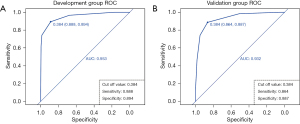
Table 3
Group | AUC | Cut off | Sensitivity | Specificity | P | Z |
---|---|---|---|---|---|---|
Development group | 0.531 | 0.626 | ||||
Prediction model | 0.959 | 0.618 | 0.969 | 0.883 | ||
BI-RADS category | 0.953 | 0.384 | 0.888 | 0.894 | ||
Validation group | 0.356 | 0.923 | ||||
Prediction model | 0.952 | 0.724 | 0.881 | 0.935 | ||
BI-RADS category | 0.932 | 0.384 | 0.864 | 0.887 |
BI-RADS, Breast Imaging Reporting and Data System. AUC, area under the curve.
Evaluation of model performance
The evaluation of model performance includes calibration, discrimination and usefulness. Calibration evaluates the model performance in the overall study population. For the prediction model, the R2 of the development group and the validation group was 0.78 and 0.72, respectively. It can be seen that the actual and fitted values of the development and validation groups were similar. Thus, the calibration ability of the model is acceptable. Discrimination is the ability of a model to separate individuals who will experience the event of interest from those who will not, it is often measured based on the AUC. The AUC of development and validation groups (0.96 vs. 0.95) were greater than 0.80, and the differentiation of model was good (Figure 3). Besides, for the usefulness, DCA showed development group had a diagnostic probability in the range of 0–100%, whereas the validation group had a diagnostic probability in the range of 0–90%. Obviously, there were the highest accuracy and net benefit (NB) of model application in the above ranges, beyond which the model accuracy was limited and the NB decreased significantly (Figure 5).
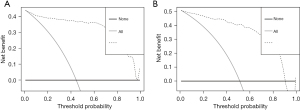
Discussion
Breast ultrasound has many advantages including real-time dynamics, easy operation, and no radiation. Besides, it is more suitable for Asian women whose breasts are predominantly glandular in composition. In 1992, the ACR first proposed BI-RADS. By the fourth revision in 2003, ultrasound and MRI were added to the terminology of features and were used to describe and categorize the breast lesions. Currently, it is in its fifth edition which is widely used (14).
However, the terminology describing features are numerous and the weights are not clear. In addition, pathological types and characteristics of breast disease are various, making diagnosis more difficult for doctors. Clinicians tend to judge the category of breast lesion on relatively subjective judgments, but fail to strictly follow the guideline, which lead to intra- and interobserver variability largely and impede the next examination and treatment for patient. Among them, lesions categorized as BI-RADS 4 are more susceptible to subjective diagnosis of the physicians. Besides, the malignancy rate in the ACR BI-RADS ultrasound atlas is a wide range rather than an exact value. For example, the malignancy likelihood of BI-RADS category 4 is 2–95%, which is obviously too large for being a predictive span to distinguish the benign lesions from malignant diseases (15,16). Therefore, it is of great significance to analyze the risk factors of breast lesions and establish prediction model for breast cancer prevention and control.
Prediction model can predict and detect high-risk individuals more accurately through extensive data analysis and complex algorithms, so that more targeted management strategies and recommendations can be adopted to improve patients’ prognosis. Prediction model is now widely used to predict the risk of breast cancer, germline genetic mutation, metastatic relapse, even prognosis and drug-response of patients (17-21). Besides, some prediction models have been constructed with the gene signature and clinicopathological features to improve the risk stratification and quantify the risk assessment of individual patients (22). However, previous risk prediction models were based solely on clinical features or selected two-dimensional images without color Doppler and spectral Doppler images (23). Sufficient detailed clinical features such as age are crucial for the diagnose of sonographers (24), but clinical history of patients is usually overlooked before examination. For breast diseases with similar imaging features, different clinical symptoms are helpful for diagnosis of the lesions. For example, if a tumor is small and its border is relatively clear, the tumor may be diagnosed as benign based on imaging features only, whereas it may be malignant with bloody nipple discharge. Besides, images of color Doppler and spectral Doppler are able to provide essential information of blood flow within the lesion and surrounding tissues. Therefore, clinical and ultrasonic image features were included in this study to improve the accuracy and practicability of the model.
In the previous studies, clinical decision trees, logistic regression analysis, machine learning classifiers, convolutional neural network and support vector regression (SVR) have been used to select independent risk factors of breast cancer (25-28). In this current study, a prediction model based on patients’ clinical information and ultrasonic features was established. Beyond that, LASSO was used to process all candidate variables of the development group by constructing a penalty function instead of stepwise processing, which could lead to improved stability of the model. In addition, web application is a user-friendly web interface and it can make calculations much easier for health-care professionals and members of the public (29). Unlike the previous studies, this study developed the web application based on R shiny (https://echohx925.shinyapps.io/characteristics_of_breast_lesions/). Besides, the malignancy probability and CI of breast lesions could be calculated in real time by selecting relevant parameters, and the specific probability was favorable to the visualization of results and the understanding of patients. Meanwhile, this study showed that the diagnostic value of prediction model was not inferior to that of the ACR BI-RADS category, which is the standard widely recognized and applied worldwide.
Besides, the edition of ACR BI-RADS updated in 2013 merely describes ultrasound features without the weight of each feature. Through construction of logistic regression formula for this prediction model, we selected and assigned coefficients to the clinical and ultrasound features of the breast lesions. Combined with the selected features’ coefficients and weights, physicians could make more accurate clinical decisions and guidance for patients. For example, if lesions are diagnosed as category 4A, 4B or 4C, the malignancy probability of ACR BI-RADS are 2–10%, 10–50% and 50–95%, respectively (10), and the predictions are not so definitive. For the following examination, biopsy will be recommended according to the guideline. Nevertheless, false positive rate exits in results of biopsy to some extent (30). Instead of performing a puncture biopsy immediately after the detection of suspected lesions, additional accessory examinations are essential for the early diagnosis and prognosis assessment, which can reduce unnecessary suffering and expense for the patients. According to the selected features and corresponding coefficients in this model, if the calculated malignancy probability is 45% and the lesion has malignant features such as calcification in ultrasonic images, mammography will be performed first. If the blood flow of the lesion is unclear in two-dimensional ultrasound, an examination of contrast-enhanced ultrasound will be needed. In the case of irregular margins of the lesion, MRI will be recommended first for accurate diagnosis (21-33). Based on this model, a precise inspection process is conductive to reducing the number of unnecessary biopsies and lowering negative rates.
Though this study is novel, it still has some shortcomings worthy of further research. Firstly, the sample size of this dataset is not large enough. Secondly, this study lacks clinical and ultrasonic information of specific types of breast tumors such as medullary carcinoma and breast lymphoma. Lastly, the risk factors for breast cancer are unidentical in different countries, regions and races, it is essential to validate the clinical usefulness and accuracy of the prediction model further. The multicenter studies with a large sample data from domestic and international databases are needed to strengthen the model’s credibility and generalizability.
Conclusions
In conclusion, the independent risk factors of clinic and ultrasonic features for breast cancer have been screened out, and an interactive web version of the prediction model has been built. The breast cancer probability of patients from the model is intuitive and precise, which can provide reliable references for further examination and treatment.
Acknowledgments
Funding: None.
Footnote
Reporting Checklist: The authors have completed the TRIPOD reporting checklist. Available at https://gs.amegroups.com/article/view/10.21037/gs-22-663/rc
Data Sharing Statement: Available at https://gs.amegroups.com/article/view/10.21037/gs-22-663/dss
Conflicts of Interest: All authors have completed the ICMJE uniform disclosure form (available at https://gs.amegroups.com/article/view/10.21037/gs-22-663/coif). The authors have no conflicts of interest to declare.
Ethical Statement: The authors are accountable for all aspects of the work in ensuring that questions related to the accuracy or integrity of any part of the work are appropriately investigated and resolved. The study was conducted in accordance with the Declaration of Helsinki (as revised in 2013) and was approved by the ethics committee of PLA General Hospital (No. S2021-291-01). All patients provided informed consent.
Open Access Statement: This is an Open Access article distributed in accordance with the Creative Commons Attribution-NonCommercial-NoDerivs 4.0 International License (CC BY-NC-ND 4.0), which permits the non-commercial replication and distribution of the article with the strict proviso that no changes or edits are made and the original work is properly cited (including links to both the formal publication through the relevant DOI and the license). See: https://creativecommons.org/licenses/by-nc-nd/4.0/.
References
- Giaquinto AN, Sung H, Miller KD, et al. Breast Cancer Statistics, 2022. CA Cancer J Clin 2022;72:524-41. [Crossref] [PubMed]
- Liu C, Chen M, Shi Y. Downregulation of hsa_circ_0006220 and its correlation with clinicopathological factors in human breast cancer. Gland Surg 2021;10:816-25. [Crossref] [PubMed]
- Fan L, Strasser-Weippl K, Li JJ, et al. Breast cancer in China. Lancet Oncol 2014;15:e279-89. [Crossref] [PubMed]
- Choi JS, Han BK, Ko EY, et al. Comparison of synthetic and digital mammography with digital breast tomosynthesis or alone for the detection and classification of microcalcifications. Eur Radiol 2019;29:319-29. [Crossref] [PubMed]
- Suzuki A, Ishida T, Ohuchi N. Controversies in breast cancer screening for women aged 40-49 years. Jpn J Clin Oncol 2014;44:613-8. [Crossref] [PubMed]
- He Z, Chen Z, Tan M, et al. A review on methods for diagnosis of breast cancer cells and tissues. Cell Prolif 2020;53:e12822. [Crossref] [PubMed]
- Hooley RJ, Andrejeva L, Scoutt LM. Breast cancer screening and problem solving using mammography, ultrasound, and magnetic resonance imaging. Ultrasound Q 2011;27:23-47. [Crossref] [PubMed]
- D’Orsi CJ, Sickles EA, Mendelson EB, et al. ACR BI-RADS® Atlas, Breast Imaging Reporting and Data System. Reston, VA, American College of Radiology; 2013.
- Strigel RM, Burnside ES, Elezaby M, et al. Utility of BI-RADS Assessment Category 4 Subdivisions for Screening Breast MRI. AJR Am J Roentgenol 2017;208:1392-9. [Crossref] [PubMed]
- Ha SM, Chae EY, Cha JH, et al. Association of BRCA Mutation Types, Imaging Features, and Pathologic Findings in Patients With Breast Cancer With BRCA1 and BRCA2 Mutations. AJR Am J Roentgenol 2017;209:920-8. [Crossref] [PubMed]
- Steyerberg EW, Vickers AJ, Cook NR, et al. Assessing the performance of prediction models: a framework for traditional and novel measures. Epidemiology 2010;21:128-38. [Crossref] [PubMed]
- Anothaisintawee T, Teerawattananon Y, Wiratkapun C, et al. Risk prediction models of breast cancer: a systematic review of model performances. Breast Cancer Res Treat 2012;133:1-10. [Crossref] [PubMed]
- Lin X, Zhuang S, Yang S, et al. Development and internal validation of a conventional ultrasound-based nomogram for predicting malignant nonmasslike breast lesions. Quant Imaging Med Surg 2022;12:5452-61. [Crossref] [PubMed]
- Luo WQ, Huang QX, Huang XW, et al. Predicting Breast Cancer in Breast Imaging Reporting and Data System (BI-RADS) Ultrasound Category 4 or 5 Lesions: A Nomogram Combining Radiomics and BI-RADS. Sci Rep 2019;9:11921. [Crossref] [PubMed]
- Gu Y, Tian JW, Ran HT, et al. The Utility of the Fifth Edition of the BI-RADS Ultrasound Lexicon in Category 4 Breast Lesions: A Prospective Multicenter Study in China. Acad Radiol 2022;29 Suppl 1:S26-34.
- Niu Z, Tian JW, Ran HT, et al. Risk-predicted dual nomograms consisting of clinical and ultrasound factors for downgrading BI-RADS category 4a breast lesions - A multiple centre study. J Cancer 2021;12:292-304. [Crossref] [PubMed]
- Cintolo-Gonzalez JA, Braun D, Blackford AL, et al. Breast cancer risk models: a comprehensive overview of existing models, validation, and clinical applications. Breast Cancer Res Treat 2017;164:263-84. [Crossref] [PubMed]
- Han Y, Wang J, Sun Y, et al. Prognostic Model and Nomogram for Estimating Survival of Small Breast Cancer: A SEER-based Analysis. Clin Breast Cancer 2021;21:e497-505. [Crossref] [PubMed]
- Malik V, Kalakoti Y, Sundar D. Deep learning assisted multi-omics integration for survival and drug-response prediction in breast cancer. BMC Genomics 2021;22:214. [Crossref] [PubMed]
- Nicolò C, Périer C, Prague M, et al. Machine Learning and Mechanistic Modeling for Prediction of Metastatic Relapse in Early-Stage Breast Cancer. JCO Clin Cancer Inform 2020;4:259-74. [Crossref] [PubMed]
- Phung MT, Tin Tin S, Elwood JM. Prognostic models for breast cancer: a systematic review. BMC Cancer 2019;19:230. [Crossref] [PubMed]
- Peng Y, Yu H, Jin Y, et al. Construction and Validation of an Immune Infiltration-Related Gene Signature for the Prediction of Prognosis and Therapeutic Response in Breast Cancer. Front Immunol 2021;12:666137. [Crossref] [PubMed]
- Wang H, Li Y, Khan SA, et al. Prediction of breast cancer distant recurrence using natural language processing and knowledge-guided convolutional neural network. Artif Intell Med 2020;110:101977. [Crossref] [PubMed]
- Yang K, Ye X, Tian H, et al. Development and validation of a nomogram for discriminating between benign and malignant breast masses by conventional ultrasound and dual-mode elastography: a multicenter study. Quant Imaging Med Surg 2023;13:865-77. [Crossref] [PubMed]
- Cheng LH, Hsu TC, Lin C. Integrating ensemble systems biology feature selection and bimodal deep neural network for breast cancer prognosis prediction. Sci Rep 2021;11:14914. [Crossref] [PubMed]
- Goli S, Mahjub H, Faradmal J, et al. Survival Prediction and Feature Selection in Patients with Breast Cancer Using Support Vector Regression. Comput Math Methods Med 2016;2016:2157984. [Crossref] [PubMed]
- Lee AJ, Cunningham AP, Kuchenbaecker KB, et al. BOADICEA breast cancer risk prediction model: updates to cancer incidences, tumour pathology and web interface. Br J Cancer 2014;110:535-45. [Crossref] [PubMed]
- Joo S, Ko ES, Kwon S, et al. Multimodal deep learning models for the prediction of pathologic response to neoadjuvant chemotherapy in breast cancer. Sci Rep 2021;11:18800. [Crossref] [PubMed]
- Euhus DM, Leitch AM, Huth JF, et al. Limitations of the Gail model in the specialized breast cancer risk assessment clinic. Breast J 2002;8:23-7. [Crossref] [PubMed]
- Zhu YC, Sheng JG, Deng SH, et al. A deep learning-based diagnostic pattern for ultrasound breast imaging: can it reduce unnecessary biopsy? Gland Surg 2022;11:1529-37. [Crossref] [PubMed]
- Shi Q, Wang J, Ai X, et al. Development and validation of a prognostic nomogram for early HER2-positive and lymph node-negative breast cancer. Gland Surg 2021;10:2255-65. [Crossref] [PubMed]
- Yu Y, Tan Y, Xie C, et al. Development and Validation of a Preoperative Magnetic Resonance Imaging Radiomics-Based Signature to Predict Axillary Lymph Node Metastasis and Disease-Free Survival in Patients With Early-Stage Breast Cancer. JAMA Netw Open 2020;3:e2028086. [Crossref] [PubMed]
- Evans DG, Howell A. Breast cancer risk-assessment models. Breast Cancer Res 2007;9:213. [Crossref] [PubMed]