Development of a basement membrane gene signature and identification of the potential candidate therapeutic targets for pancreatic cancer
Highlight box
Key findings
• Our gene signature predictive model based on BMGs performed exceptionally well in predicting the prognosis of pancreatic cancer patients.
What is known and what is new?
• BMGs are known to be associated with tumorigenesis.
• A gene signature based on BMGs for predicting pancreatic cancer prognosis was established.
What is the implication, and what should change now?
• A therapeutic target based on BMGs was discovered that could be used to develop potential drugs for pancreatic cancer treatment.
Introduction
With 495,773 new cases recorded in 2020, the incidence of pancreatic cancer continues to increase each year (1). It has been predicted that pancreatic cancer will be the second most common cause of cancer-related mortality in women and men in the United States by 2030 (2). Pancreatic ductal adenocarcinoma (PDAC) is the most common type of pancreatic cancer, has the worst prognosis of any solid tumor, and has a 5-year survival rate of <10% (3). Due to the absence of distinctive clinical signs and a lack of effective biomarkers for early identification and diagnosis, most PDAC patients have locally advanced or metastatic disease at the time of diagnosis, which is not amenable to potentially curative surgical removal due to its aggressive biology (4). Patients’ prognosis and survival rates have not improved considerably over time, as PDAC is resistant to standard therapeutic techniques, such as radiation and chemotherapy and has a limited response to current targeted immunotherapies and treatments (5). A landscape of biomarkers in pancreatic cancer in predicting prognostic value was introduce accordingly, providing us with the promising role in PDAC treatment (6,7).
Basement membranes (BMs), which are the most basic extracellular matrices (ECMs) in animals, are sheet-like structures that support epithelia and encircle the bulk of tissues (8). Proteases, growth factors, and matricellular proteins are also prevalent in BMs (8). BMs have a variety of compositions that are optimized for mechanical stress resistance, shape tissue, and generate diffusion hurdles (9-11). BMs also deliver signals that control differentiation, cell polarity, survival, and migration (10,12). Research has shown that variants in over 20 basement membrane genes (BMGs) are responsible for many human illnesses, which highlights their varied and crucial activities. And it was found that the aberrant stromal genes were significantly associated with a vital prognostic value in PDAC (6,7). In immune diseases and BM abnormalities, autoantibodies target BM proteins. Protein expression and turnover are important pathogenic components of cancer, diabetes, and fibrosis (13-17).
A broader understanding of BMGs is essential to the development of pancreatic cancer, therapy, and future research. It is elucidated that the basement membrane destruction and stromal invasion can be induced by MMP2 and thus promoting local invasion of PDAC. One study investigated the role of basement membrane proteins in PDAC. By experiment validation, they found that BMGs especially collagen IV, laminin, and fibronectin promoted cancer cells invasion by influencing ERK1/2 phosphorylation (18). However, to date, only a few research studies have thoroughly investigated the association between BMGs and PDAC. This study sought to visualize the expression levels and prognostic values of BMGs in PDAC using bioinformatics. We successfully created and validated a prognostic signature build on 7 BMGs that may reliably predict PDAC patient survival. Further, we observed an association between the immune infiltration and the prognostic signature in PDAC. Our findings provide a theoretical foundation for immune checkpoint treatment methods. We also identified 5 small-molecule medicines that have the potential to treat PDAC. We present the following article in accordance with the TRIPOD reporting checklist (available at https://gs.amegroups.com/article/view/10.21037/gs-23-24/rc).
Methods
Data gathering and identification of differentially expressed genes
BMGs (https://cdn.amegroups.cn/static/public/gs-23-24-1.xlsx) were obtained from the previous study (19). We obtained the messenger ribonucleic acid (mRNA) expression and relevant clinical data from a publicly available data set from The Cancer Genome Atlas (TCGA) (https://portal.gdc.cancer.gov). The data set comprised data on 4 normal pancreatic tissues and 178 pancreatic cancer tissues. For the validation group, the GSE76427 data set was obtained from the Gene Expression Omnibus (GEO) database. The mRNA expression data were normalized using of the corresponding R program. Differentially expressed genes that fulfilled the criteria of a | log2 fold change | >0 and a P value <0.05 were obtained using the R-based limma program.
Functional enrichment and protein-protein interaction analysis
ClusterProfler was used to examine the Gene Ontology (GO) “biological process (BP)”, “cellular component (CC)”, and “molecular function” (MF) terms. The Kyoto Encyclopedia of Genes and Genomes (KEGG) data were also analyzed using this approach. The false discovery rate (FDR) and P value cutoff for significant enrichment were set at <0.05. The differentially expressed gene data were uploaded to the STRING database (http://www.string-db.org/) to examine the protein-protein relationships.
Small-molecule drug screening
To test the possible small-molecule medications associated with BMGs for the treatment of PDAC patients, we uploaded the variously expressed BMGs to the Enrichr database (https://maayanlab.cloud/Enrichr/). The top 5 drug molecules were chosen after the possible drugs were rated based on their P values.
Establishment and validation of a prognostic model builds on BMGs
To further examine the predictive efficacy of the BMGs, we conducted a univariate Cox regression analysis. The R package glmnet was then used to construct the prognostic risk model from the least absolute shrinkage and selection operator (LASSO)-penalized Cox regression analysis. The provided instrument was used to calculate risk rankings. To determine the risk score, the following formula was used: risk = (Coef1 * expression mRNA1) + (Coef2 * expression mRNA2) + (Coef n * expression mRNA n), where Coef is the coefficient from the LASSO Cox regression model for the corresponding mRNA. Based on the median risk score, we divided the PDAC patients into subgroups. The survival analysis used Kaplan-Meier curves to compare the outcomes of the 2 groups (low- and high-risk groups). A time-related receiver operating characteristic (ROC) analysis was conducted to evaluate the prognostic capacity of the risk model using the survival ROC package.
Gene Set Enrichment Analysis (GSEA)
A GSEA was conducted to examine the shared molecular pathways between the high- and low-risk groups. Significant results were defined as having a P value or FDR of <0.05.
Nomogram construction based on clinical data and the risk score
We examined the connections between the clinical factors and the BMG-based signature. Additionally, we conducted both multivariate and univariate Cox regression analyses in combination with other clinical factors to examine whether the risk scores had an independent predictive value for PDAC patients. Using clinical variables and the BMG-based signature risk score, a nomogram was developed to predict a patient’s 1-, 3-, and 5-year overall survival (OS) after a diagnosis of PDAC. The predictive power of the nomogram was calculated using a calibration curve and concordance index (C-index).
Clinical samples
Both normal and pancreatic cancer tissue samples were obtained from patients undergoing surgery at the First Affiliated Hospital of Nanjing Medical University between January 2021 and May 2022. The study was conducted in accordance with the Declaration of Helsinki (as revised in 2013). The study was approved by the Ethics Committee of Nanjing Medical University (No. 2021-SR-483) and informed consent was taken from all the patients.
RNA extraction and quantitative real-time polymerase chain reaction (qRT-PCR)
Following the protocol of the manufacturer of the Trizol reagent (Invitrogen, USA), total RNA was extracted from the cells and tissues. The mRNA levels were determined using a LightCycler 480 (Roche, USA) or an Applied Biosystems StepOne Plus Real-Time Polymerase Chain Reaction machine to conduct the qRT-PCR analysis. Complementary deoxyribonucleic acid was synthesized using HiScript II (Vazyme, China). For the mRNA detection, glyceraldehyde 3-phosphate dehydrogenase served as the internal standard control. All the experiments were triplicated, and the results were compared using the Ct (cycle threshold) values. All the PCR primers were supplied by GeneCopoeia (Guangzhou, China). The sequences of tubulointerstitial nephritis antigen (TINAG) primers were F-CAGGTTCCAAGGAGAAGCCC and R-CAGGTTCCAAGGAGAAGCCC.
Immune cell infiltration analysis
There is growing evidence that the immune infiltration of tumor cells contributes to the development of cancer and is associated with patient’ prognosis. Thus, using the EPIC algorithms, CIBERSORT, QUANTISEQ, CIBERSORT-ABS, XCELL, MCP-counter, and TIMER, we assessed the degree of immune cell penetration among the subgroups based on the gene signature. We also examined the expression of a number of immune checkpoints to estimate the outcome of immune checkpoint–blockade treatment (https://cdn.amegroups.cn/static/public/gs-23-24-2.xlsx). Additionally, we used the TIMER data set (https://cistrome.shinyapps.io/timer/) to examine the association between immune cells and the 7 BMGs to better understand the function of BMGs in PDAC.
Statistical analyses
The statistical analyses were conducted in R (version 4.0.5). The Wilcoxon test was used to examine dissimilarities between the 2 groups. A probability value of <0.05 was considered statistically significant.
Results
Establishment and validation of a BMG-based signature
Compared to the normal pancreatic tissues, 23 BMGs from the TCGA-PDAC data set were shown to have differing levels of expression, of which 11 were downregulated and 12 were upregulated (Figure 1). A univariate Cox regression analysis was conducted to evaluate the prognostic significance of the BMGs based on these aberrant genes. The results showed that only 8 of the BMGs had prognostic relevance (Figure 2). Next, a LASSO Cox regression analysis was conducted and totally, 7 genes [i.e., glypican 2 (GPC2), integrin alpha-3 (ITGA3), integrin alpha-6 (ITGA6), laminin subunit alpha 3 (LAMA3), myelin protein zero like 2 (MPZL2), roundabout guidance receptor 4 (ROBO4), and TINAG] were included in the risk model, and the heatmap of the 7 genes was shown in Figure S1A. The relevant coefficients of the 7 genes were used to calculate the risk score using the following formula: Risk score = (–0.9084 for GPC2 expression, 0.0013 for ITGA3, 0.0018 for ITGA6, 0.0075 for LAMA3, 0.0027 for MPZL2, –0.0589 for ROBO4, and 0.0042 for TINAG expression). Based on the median risk score, the patients were divided into low- and high-risk groups (Figure S1B). Patients in the high-risk group had more deaths and a shorter survival time than those in the low-risk group (Figure S1B). As the low-risk group had fewer fatalities than the high-risk group (P<0.001), we concluded that the risk score was inversely related to prognosis (Figure 3A). The time-dependent ROC analysis results showed that the BMG-based signature in TCGA database had a prognosis correctness of 0.844 at 5 years, 0.808 at 3 years, and 0.728 at 1 year (Figure 3B). In the GEO data set, we adopted the same method to confirm the prognostic value of the BMG-based signature. And confirmed the efficiency of prognostic model established by the gene signature (Figure 3C,3D, Figure S1C). The ROC curves’ time dependence revealed that the areas under the curve (AUCs) were 0.695 at 1 year and 0.741 at 3 years (Figure 3D).
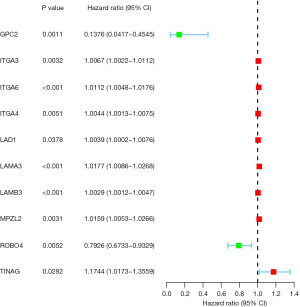
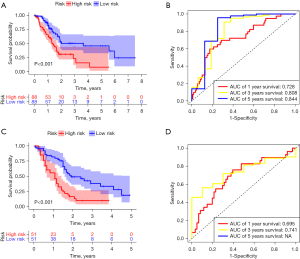
The BMG-based signature was used as an independent predictor of the prognosis of PDAC patients
Multivariable and univariable Cox analyses were performed to determine if the signature could be employed as an independent prognostic predictor. The results of the univariate analysis showed that age and risk score were significantly associated with PDAC patient survival (P<0.05) (Figure 4A). Similarly, the results of the multivariate analysis showed that age and risk score were significantly linked to prognosis (P<0.05) (Figure 4B). These results provided evidence that the BMG-based signature could be used as a prognostic predictor for patients with PDAC.
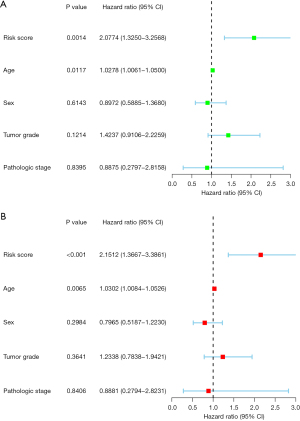
Associations between the clinical features and the signature
The Chi-square test was used to determine if the prognostic signature contributed to the development of PDAC. Surprisingly, no significant differences were found between the groups in terms of tumor stage, age, sex, or tumor grade (P>0.05; Figure 5 and Figure S2). Further, a stratification analysis was used to assess the signature’s prognostic usefulness in several patient subsets. The BMG-based signature was found to be very effective in predicting the prognosis of patients aged >60 years (P=0.005), those with Stage I–II disease (P=0.001), males (P=0.006), and those with low-grade (G1 + G2) tumors (Figure 6).
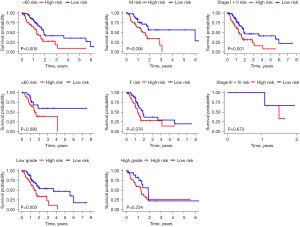
Nomogram establishment
Many prognostic indicators were added to the nomogram to graphically evaluate an individual’s survival probability. To precisely predict the survival of PDAC patients, we devised a nomogram that included tumor stage, tumor grade, gender, age, and risk score. Nomography was used to predict PDAC patients’ 1-, 3-, and 5-year survival rates (Figure 7A). As the calibration curve (Figure 7B) shows, patient survival was in line with expectations. The C-index for the nomogram was 0.677, indicating that it had excellent predictive power.
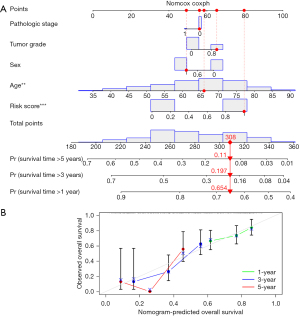
Functional enrichment analyses and protein-protein interactions
KEGG and GO analyses were performed to evaluate the possible function of the differentially expressed BMGs (Figure 8A,8B). The BP (biological process) analysis results revealed that 23 BMGs were significantly engaged in the establishment of the primary germ layer and in cell-substrate adhesion, cell-matrix adhesion, the integrin-mediated signaling pathway, and cell adhesion mediated by integrin. The CC (cellular component) analysis results showed that the 23 BMGs were primarily enriched in collagen-containing ECM, the integrin complex, the protein complex involved in cell adhesion, the plasma membrane signaling receptor complex, and BMs. The MF (molecular function) analysis results showed that the 23 BMGs were mostly involved in integrin binding, the ECM structural component, cell adhesion mediator activity, insulin-like growth factor I binding, and opsonin binding (Figure 8A). The KEGG pathway analysis results showed that these genes were mainly involved in the ECM-receptor interaction, the regulation of actin cytoskeleton, focal adhesion, human papillomavirus infection, and the PI3K-Akt (phosphatidylinositol 3 kinase-protein kinase B) signaling pathway (Figure 8B). The STRING database revealed the protein-protein interaction network of the BMGs (Figure 9).
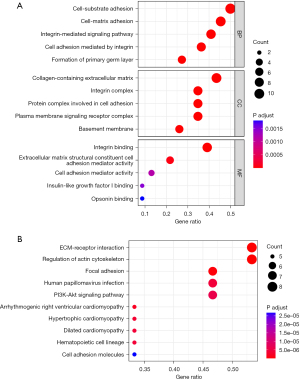
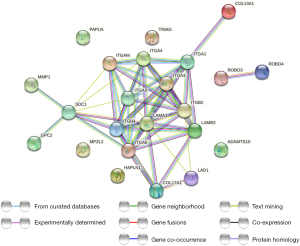
GSEA
A GSEA was performed to clarify the molecular processes of the BMG-based signature. The findings of the GSEA demonstrated that in the high-risk group, the BMGs were mostly enriched in the biosynthesis of nucleotide sugars, circadian rhythm, hypertrophic cardiomyopathy, other types of O-glycan biosynthesis, and RNA polymerase (Figure 10).
Immune infiltration–level analysis of the BMG-based signature
MCP-counter, CIBERSORT, XCELL, CIBERSORT-ABS, EPIC, QUANTISEQ, and TIMER analyses were used to generate the heatmap, which showed the link between immune infiltration and the signature (Figure 11). Given the importance of immune therapy using checkpoint inhibitors, we further examined the link between important immune checkpoints and risk scores (20). We discovered a significant difference in the expressions of ADORA2A (adenosine A2a receptor), BTLA (B and T lymphocyte associated), CD27 (CD27 molecule), CD28 (CD28 molecule), CD40LG (CD40 ligand), CD48 (CD48 molecule), CD160 (CD160 molecule), CD200 (CD200 molecule), CD200R1 (CD200 receptor 1), CTLA4 (cytotoxic T-lymphocyte associated protein 4), IDO2 (indoleamine 2,3-dioxygenase 2), LAG3 (lymphocyte activating 3), PDCD1 (programmed cell death 1), TMIGD2 (transmembrane and immunoglobulin domain containing 2), TNFRSF4 (tumor necrosis factor receptor superfamily, member 4), TNFRSF8 (TNF receptor superfamily member 8), and TNFSF14 (TNF superfamily member 14) between the high- and low-risk patients (Figure S3).
Small-molecule drug identification
On the basis of the BMGs, we identified the following top 5 small-molecule medications using the Enrichr database: cyclohexanecarboxamide, folic acid, colforsin, N-nitrosodiethylamine, and ML-7 (Table 1).
Table 1
Term | P value | Adjusted P value | Odds ratio | Combined score |
---|---|---|---|---|
ML-7 CTD 00002750 | 1.71E-07 | 2.12E-05 | 467.8359375 | 7,289.664133 |
Cyclohexanecarboxamide, 4-(1-aminoethyl)-N-4-pyridinyl-, trans- CTD 00003513 | 6.46E-07 | 4.01E-05 | 293.2647059 | 4,179.6671 |
Colforsin MCF7 UP | 5.74E-04 | 0.023737189 | 76.49615385 | 570.8434889 |
N-NITROSODIETHYLAMINE CTD 00005817 | 0.001268879 | 0.036133473 | 50.86410256 | 339.2443277 |
Folic acid CTD 00005997 | 0.001923155 | 0.036133473 | 41.03626943 | 256.6321285 |
TIMER analysis
The TIMER database was used to examine the connection between the 7 prognostic BMGs and the immunological cells (Figure 12). The results showed ITGA3 was negatively linked with macrophages and cluster differentiation (CD)4+ B, and T cells, and was positively linked with CD8+ T cells, neutrophils, and dendritic cells. ITGA6, LAMA3, and MPZL2 were only negatively linked with CD4+ T cells. ROBO4 was positively linked with all the immune cells. TINAG was negatively associated with macrophages. GPC2 was negatively associated with B cells and CD8+ T cells.
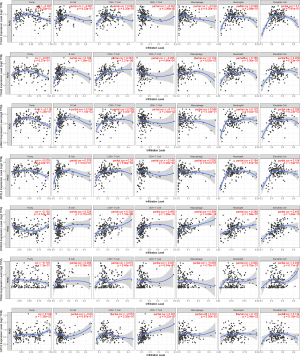
Validation of TINAG expression and function in pancreatic cancer
According to our analysis of the differently expressed BMGs, TINAG was the most upregulated gene among all the BMGs (https://cdn.amegroups.cn/static/public/gs-23-24-3.xlsx). And this difference in the expression level indicated the potential oncogenic role of TINAG in PDAC. Thus, we chose TINAG as the candidate for the following validation. We searched GTEx and TCGA databases through GEPIA (http://gepia.cancer-pku.cn/) and found that TINAG was more upregulated in pancreatic cancer tissues than normal pancreatic tissues (Figure 13A). The high expression of TINAG was linked to a worse prognosis in PDAC (Figure 13B). We also performed PCR using the samples of patients at our hospital and found that TINAG was relatively lowly expressed in normal pancreatic tissues compared to pancreatic cancer tissues (Figure 13C). Colony formation assays revealed that knocking down TINAG reduced colony formation efficiency (Figure 13D,13E). The Cell Counting Kit 8 (CCK-8) assays showed that TINAG knockdown reduced cell proliferation (Figure 13F). A flow cytometry analysis revealed an increase in the proportion of G2 cells in the TINAG knocked-down cells (Figure 13G and Figure S4).
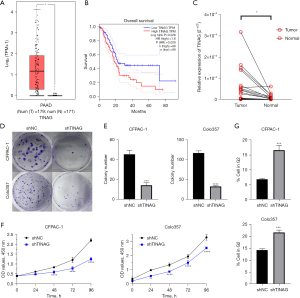
Discussion
Most tissues are enclosed by BMs, which are the first ECMs in mammals (8). Gene signatures can be used to predict the prognosis of cancer patients (21). In this study, we first searched for the BMGs in TCGA database that were differentially expressed in PDAC tissues compared to normal pancreatic tissues. We created protein-protein interaction networks for BMGs and thoroughly investigated the biological pathways. Next, using univariable and LASSO-penalized Cox regression analysis, we discovered 23 BMGs associated with PDAC prognosis. We created and confirmed a risk model based on these 23 BMGs. ROC and survival analyses demonstrated the strong predictive power of the model. Multivariate and univariate Cox analyses confirmed that a risk score based on 7 BMGs (GPC2, ITGA3, ITGA6, LAMA3, MPZL2, ROBO4, and TINAG) was an independent predictive factor for patients with PDAC. The 7 BMGs were significantly differently expressed in PDAC and correlated with the prognosis of PDAC. Gene signature constructed with the 7 genes divided patients into high- and low-risk groups. And significant correlation between clinical characteristics and gene signature were found in our research, such as tumor stage, age, sex, or tumor grade (P<0.05). We also found that the signature was linked to immune cell infiltration, and identified 5 small compounds that may be used to treat and cure PDAC patients. Validation of the gene signature with GEO data showed an excellent performance in predicting patients’ prognosis.
The GO analysis showed that the BMGs were mostly associated with cell adhesion mediated by integrin, integrin-mediated signaling, cell adhesion mediator activity, and insulin-like growth factor I binding. The pathway enrichment analysis revealed that the BMGs participated heavily in the ECM-receptor interaction, focal adhesion, and PI3K-AKT signaling pathways. There was a strong association between these pathways and the malignant phenotypes of a number of cancer types, which provides evidence of the role of BMGs in cancer development and progression. In addition, a TIMER data-set analysis revealed an association between the immune cell infiltrates and prognostic BMGs of the model, which suggests that BMGs may control cancer growth by inhibiting immune infiltrates.
GPC2 is a protein-coding gene that produces a cell surface proteoglycan that includes heparan sulfate (22). The glycosylphosphatidylinositol anchor allows GPC, which is encoded by the glypican (GPC) family of genes, to bind to the cell membrane (23). A research showed GPC2 is a potential diagnostic, immunological, and prognostic biomarker (24). The importance of GPC2 in early detection, regulatory pathways, related genes, and chemicals has also been explored by researchers, who emphasized the extent to which it is expressed differently in cancer and healthy tissues (24). Pancreatic cancer prognostic prediction using GPC2 combined with GPC3 and GPC5 has shown great efficacy (25).
ITGA3, a protein belonging to the integrin family, is encoded by the ITGA3 gene. ITGA3 serves as a cell adhesion molecule on the cell membrane. Previous researches have shown that the ITGA3 expression is associated with cancer development and metastasis, and that it binds to ECM proteins (26-29). One study found that the high pancreatic expression of ITGA3 is linked to histological grade, T classification, the histological type of the pancreatic cancer, vital status, cancer stage, and recurrence (30). Further, we discovered that worse OS and relapse-free survival were independently linked to higher ITGA3 expression levels. Thus, ITGA3 expression appears to be a valuable biomarker for the diagnosis and staging of pancreatic cancer (30). Earlier research indicated that the integrin family is significant in the regulation of stemness, metastasis, and medication resistance in different tumor types. Integrins can create a link between cells and the ECM because they are receptors on cell surface membranes. A member of the integrin family is ITGA6. It often forms an integrin complex with the beta 1 or 4 subunits that interact with the ECM to take part in the BPs related to cancer (31). Numerous tumor types have been found to express ITGA6 abnormally. Research has shown that the N6-methyladenosine modification of ITGA6 mRNA leads to abnormal expression and promotes the development of bladder cancer (32). ITGA6 is closely linked to the strong capacity of pancreatic cancer cells for invasion and metastasis (33).
Every human organ contains laminin, which regulates cell attachment and migration during embryonic growth through interactions with different ECM components and by binding to cells via high-affinity receptors, thereby indirectly promoting and inhibiting the growth of tumor cells. LAMA3 encodes laminin and is widely present in every human organ (34). According to previous studies, the expression level of LAMA3 is associated with gastric cancer development, and its high expression can prevent the disease (35). The high expression of the LAMA3/AC245041.2 gene pair in pancreatic cancer is linked to the KRAS (KRAS proto-oncogene) mutation and a poor prognosis (36).
MPZL2 encodes the Epithelial Junctional Protein Myelin Protein Zero-like 2. It has been reported that MPZL2 is associated with hearing loss (37-39), but the role of MPZL2 in cancer is not yet known. A recent study revealed that potential role of MPZL2 in pancreatic cancer development (40), but the underlying mechanism has not yet been uncovered.
A gene termed Magic Roundabout, previously known as ROBO4, was initially identified as being exclusive to endothelium cells (41). To date, no research on ROBO4 and pancreatic cancer appears to have been conducted. Numerous studies have shown that ROBO4 stabilizes the vascular network under normal circumstances, which prevents the vasculature from leaking (41-43). ROBO4 had an effect in various cancers by promoting angiogenesis (44,45).
Only a few studies have investigated the role of TINAG in cancer. Recently Zhang et al. demonstrated that TINAG is involved in the proliferation, invasion, and migration of hepatocellular carcinoma (46). Thus, TINAG may be a promising target for cancer treatment. TINAG has also been shown to have prognostic value in pancreatic cancer (47). We showed that TINAG plays a significant role in promoting cell proliferation in pancreatic cancer.
Attempts to target immune checkpoint interactions in the context of cancer to improve anti-tumor immunity continue to increase. In our research, we discovered numerous immunological checkpoints with variable expression levels in the low and high groups. It is feasible to create inhibitors for the many immunological checkpoints that manifest differently.
Our study has multiple advantages. First, this study is the first to develop a prognostic model on the basis of BMGs in PDAC. Basement membrane is the basic structure of cells which is indispensable for cell integrity and function. Compared to other prognosis gene signature, BMGs are more universal and basic for various cancers. According to our analysis, we also predict 5 small molecules for PDAC treatment. Such analysis may bring up new insight for the deep understanding of PDAC. Besides, it is the first time to do immune infiltration analysis with BMGs in PDAC. Immune therapy plays more and more important role in cancer treatment, our analysis provided new prospect of immune therapy in PDAC. Moreover, it is our first time to validate the function of TINAG in PDAC cells. And we are the first study to uncover that TINAG promoted proliferation in PDAC cells.
This study had some limitations. Further experiments should be performed to confirm the methods by which BMGs control the biological behaviors of PDAC cells. The viability of the prognostic model should also be checked using a multicenter clinical cohort.
Conclusions
In conclusion, we identified differentially expressed BMGs that may have a function in the growth and progression of PDAC. We showed that TINAG, the most upregulated BMG in pancreatic cancer, promoted the proliferation of pancreatic cancer cells and is associated with a poor prognosis. BMGs have substantial predictive significance for PDAC patients, and targeting BMGs may be a potential therapy for pancreatic cancer. Further investigations should be conducted to verify our findings.
Acknowledgments
Funding: This study was supported in part by grants from the National Natural Science Foundation of China (No. 81904121) and the Science and Technology Planning Project of Jiangsu Province (No. BK20191087).
Footnote
Reporting Checklist: The authors have completed the TRIPOD reporting checklist. Available at https://gs.amegroups.com/article/view/10.21037/gs-23-24/rc
Data Sharing Statement: Available at https://gs.amegroups.com/article/view/10.21037/gs-23-24/dss
Peer Review File: Available at https://gs.amegroups.com/article/view/10.21037/gs-23-24/prf
Conflicts of Interest: All authors have completed the ICMJE uniform disclosure form (available at https://gs.amegroups.com/article/view/10.21037/gs-23-24/coif). The authors have no conflicts of interest to declare.
Ethical Statement: The authors are accountable for all aspects of the work in ensuring that questions related to the accuracy or integrity of any part of the work are appropriately investigated and resolved. The study was conducted in accordance with the Declaration of Helsinki (as revised in 2013). The study involving human participants were reviewed and approved by the Ethics Committee of Nanjing Medical University (No. 2021-SR-483). The patients provided their written informed consent to participate in this study.
Open Access Statement: This is an Open Access article distributed in accordance with the Creative Commons Attribution-NonCommercial-NoDerivs 4.0 International License (CC BY-NC-ND 4.0), which permits the non-commercial replication and distribution of the article with the strict proviso that no changes or edits are made and the original work is properly cited (including links to both the formal publication through the relevant DOI and the license). See: https://creativecommons.org/licenses/by-nc-nd/4.0/.
References
- Sung H, Ferlay J, Siegel RL, et al. Global Cancer Statistics 2020: GLOBOCAN Estimates of Incidence and Mortality Worldwide for 36 Cancers in 185 Countries. CA Cancer J Clin 2021;71:209-49. [Crossref] [PubMed]
- Rahib L, Wehner MR, Matrisian LM, et al. Estimated Projection of US Cancer Incidence and Death to 2040. JAMA Netw Open 2021;4:e214708. [Crossref] [PubMed]
- Mizrahi JD, Surana R, Valle JW, et al. Pancreatic cancer. Lancet 2020;395:2008-20. [Crossref] [PubMed]
- Park W, Chawla A, O'Reilly EM. Pancreatic Cancer: A Review. JAMA 2021;326:851-62. [Crossref] [PubMed]
- McGuigan A, Kelly P, Turkington RC, et al. Pancreatic cancer: A review of clinical diagnosis, epidemiology, treatment and outcomes. World J Gastroenterol 2018;24:4846-61. [Crossref] [PubMed]
- Pu N, Chen Q, Gao S, et al. Genetic landscape of prognostic value in pancreatic ductal adenocarcinoma microenvironment. Ann Transl Med 2019;7:645. [Crossref] [PubMed]
- Mishra NK, Southekal S, Guda C. Prognostic value of biomarkers in the tumor microenvironment of pancreatic ductal adenocarcinoma. Ann Transl Med 2020;8:615. [Crossref] [PubMed]
- Pozzi A, Yurchenco PD, Iozzo RV. The nature and biology of basement membranes. Matrix Biol 2017;57-58:1-11. [Crossref] [PubMed]
- Jayadev R, Chi Q, Keeley DP, et al. α-Integrins dictate distinct modes of type IV collagen recruitment to basement membranes. J Cell Biol 2019;218:3098-116. [Crossref] [PubMed]
- Li S, Qi Y, McKee K, et al. Integrin and dystroglycan compensate each other to mediate laminin-dependent basement membrane assembly and epiblast polarization. Matrix Biol 2017;57-58:272-84. [Crossref] [PubMed]
- Jayadev R, Sherwood DR. Basement membranes. Curr Biol 2017;27:R207-11. [Crossref] [PubMed]
- Sherwood DR. Basement membrane remodeling guides cell migration and cell morphogenesis during development. Curr Opin Cell Biol 2021;72:19-27. [Crossref] [PubMed]
- Foster MH. Basement membranes and autoimmune diseases. Matrix Biol 2017;57-58:149-68. [Crossref] [PubMed]
- Naba A, Clauser KR, Whittaker CA, et al. Extracellular matrix signatures of human primary metastatic colon cancers and their metastases to liver. BMC Cancer 2014;14:518. [Crossref] [PubMed]
- Randles MJ, Lausecker F, Kong Q, et al. Identification of an Altered Matrix Signature in Kidney Aging and Disease. J Am Soc Nephrol 2021;32:1713-32. [Crossref] [PubMed]
- Huang J, Zhang L, Wan D, et al. Extracellular matrix and its therapeutic potential for cancer treatment. Signal Transduct Target Ther 2021;6:153. [Crossref] [PubMed]
- Walker C, Mojares E, Del Río Hernández A. Role of Extracellular Matrix in Development and Cancer Progression. Int J Mol Sci 2018;19:3028. [Crossref] [PubMed]
- Sawai H, Okada Y, Funahashi H, et al. Basement membrane proteins play an important role in the invasive processes of human pancreatic cancer cells. J Surg Res 2008;144:117-23. [Crossref] [PubMed]
- Jayadev R, Morais MRPT, Ellingford JM, et al. A basement membrane discovery pipeline uncovers network complexity, regulators, and human disease associations. Sci Adv 2022;8:eabn2265. [Crossref] [PubMed]
- Danilova L, Ho WJ, Zhu Q, et al. Programmed Cell Death Ligand-1 (PD-L1) and CD8 Expression Profiling Identify an Immunologic Subtype of Pancreatic Ductal Adenocarcinomas with Favorable Survival. Cancer Immunol Res 2019;7:886-95. [Crossref] [PubMed]
- Zhu K, Liu X, Deng W, et al. Identification of a chromatin regulator signature and potential candidate drugs for bladder cancer. Hereditas 2022;159:13. [Crossref] [PubMed]
- Li N, Gao W, Zhang YF, et al. Glypicans as Cancer Therapeutic Targets. Trends Cancer 2018;4:741-54. [Crossref] [PubMed]
- Filmus J, Selleck SB. Glypicans: proteoglycans with a surprise. J Clin Invest 2001;108:497-501. [Crossref] [PubMed]
- Chen G, Luo D, Zhong N, et al. GPC2 Is a Potential Diagnostic, Immunological, and Prognostic Biomarker in Pan-Cancer. Front Immunol 2022;13:857308. [Crossref] [PubMed]
- Liu JQ, Liao XW, Wang XK, et al. Prognostic value of Glypican family genes in early-stage pancreatic ductal adenocarcinoma after pancreaticoduodenectomy and possible mechanisms. BMC Gastroenterol 2020;20:415. [Crossref] [PubMed]
- Islam MK, Syed P, Dhondt B, et al. Detection of bladder cancer with aberrantly fucosylated ITGA3. Anal Biochem 2021;628:114283. [Crossref] [PubMed]
- Li Y, Li F, Bai X, et al. ITGA3 Is Associated With Immune Cell Infiltration and Serves as a Favorable Prognostic Biomarker for Breast Cancer. Front Oncol 2021;11:658547. [Crossref] [PubMed]
- Sa KD, Zhang X, Li XF, et al. A miR-124/ITGA3 axis contributes to colorectal cancer metastasis by regulating anoikis susceptibility. Biochem Biophys Res Commun 2018;501:758-64. [Crossref] [PubMed]
- Zhang H, Cui X, Cao A, et al. ITGA3 interacts with VASP to regulate stemness and epithelial-mesenchymal transition of breast cancer cells. Gene 2020;734:144396. [Crossref] [PubMed]
- Jiao Y, Li Y, Liu S, et al. ITGA3 serves as a diagnostic and prognostic biomarker for pancreatic cancer. Onco Targets Ther 2019;12:4141-52. [Crossref] [PubMed]
- Seguin L, Desgrosellier JS, Weis SM, et al. Integrins and cancer: regulators of cancer stemness, metastasis, and drug resistance. Trends Cell Biol 2015;25:234-40. [Crossref] [PubMed]
- Jin H, Ying X, Que B, et al. N(6)-methyladenosine modification of ITGA6 mRNA promotes the development and progression of bladder cancer. EBioMedicine 2019;47:195-207. [Crossref] [PubMed]
- Wu Y, Tan X, Liu P, et al. ITGA6 and RPSA synergistically promote pancreatic cancer invasion and metastasis via PI3K and MAPK signaling pathways. Exp Cell Res 2019;379:30-47. [Crossref] [PubMed]
- Stemmler S, Parwez Q, Petrasch-Parwez E, et al. Association of variation in the LAMA3 gene, encoding the alpha-chain of laminin 5, with atopic dermatitis in a German case-control cohort. BMC Dermatol 2014;14:17. [Crossref] [PubMed]
- Lincoln V, Cogan J, Hou Y, et al. Gentamicin induces LAMB3 nonsense mutation readthrough and restores functional laminin 332 in junctional epidermolysis bullosa. Proc Natl Acad Sci U S A 2018;115:E6536-45. [Crossref] [PubMed]
- Tian C, Li X, Ge C. High expression of LAMA3/AC245041.2 gene pair associated with KRAS mutation and poor survival in pancreatic adenocarcinoma: a comprehensive TCGA analysis. Mol Med 2021;27:62. [Crossref] [PubMed]
- Bademci G, Abad C, Incesulu A, et al. MPZL2 is a novel gene associated with autosomal recessive nonsyndromic moderate hearing loss. Hum Genet 2018;137:479-86. [Crossref] [PubMed]
- Wang Z, Jiang M, Wu H, et al. A novel MPZL2 c.68delC variant is associated with progressive hearing loss in Chinese population and literature review. Laryngoscope Investig Otolaryngol 2022;7:870-6. [Crossref] [PubMed]
- Wesdorp M, Murillo-Cuesta S, Peters T, et al. MPZL2, Encoding the Epithelial Junctional Protein Myelin Protein Zero-like 2, Is Essential for Hearing in Man and Mouse. Am J Hum Genet 2018;103:74-88. [Crossref] [PubMed]
- Atay S. Integrated transcriptome meta-analysis of pancreatic ductal adenocarcinoma and matched adjacent pancreatic tissues. PeerJ 2020;8:e10141. [Crossref] [PubMed]
- Dai C, Gong Q, Cheng Y, et al. Regulatory mechanisms of Robo4 and their effects on angiogenesis. Biosci Rep 2019;39:BSR20190513. [Crossref] [PubMed]
- Romano E, Manetti M, Rosa I, et al. Slit2/Robo4 axis may contribute to endothelial cell dysfunction and angiogenesis disturbance in systemic sclerosis. Ann Rheum Dis 2018;77:1665-74. [Crossref] [PubMed]
- Xiao W, Pinilla-Baquero A, Faulkner J, et al. Robo4 is constitutively shed by ADAMs from endothelial cells and the shed Robo4 functions to inhibit Slit3-induced angiogenesis. Sci Rep 2022;12:4352. [Crossref] [PubMed]
- Pircher A, Schäfer G, Eigentler A, et al. Robo 4 - the double-edged sword in prostate cancer: impact on cancer cell aggressiveness and tumor vasculature. Int J Med Sci 2019;16:115-24. [Crossref] [PubMed]
- Yamanaka M, Hayashi M, Sonohara F, et al. ASO Visual Abstract: Downregulation of ROBO4 in Pancreatic Cancer Serves as a Biomarker of Poor Prognosis and Indicates Increased Cell Motility and Proliferation Through Activation of MMP-9. Ann Surg Oncol 2022;29:7192-3. [Crossref] [PubMed]
- Zhang MH, Niu H, Li Z, et al. Activation of PI3K/AKT is involved in TINAG-mediated promotion of proliferation, invasion and migration of hepatocellular carcinoma. Cancer Biomark 2018;23:33-43. [Crossref] [PubMed]
- Liu Y, Zhu D, Xing H, et al. A 6-gene risk score system constructed for predicting the clinical prognosis of pancreatic adenocarcinoma patients. Oncol Rep 2019;41:1521-30. [Crossref] [PubMed]
(English Language Editor: L. Huleatt)