Construction and validation of a macrophage polarization-related prognostic index to predict the overall survival in patients with early-stage triple-negative breast cancer
Highlight box
Key findings
• We screened 4 key macrophage-related genes and constructed a prognostic model for patients with early-stage TNBC.
What is known and what is new?
• TNBC is a highly heterogeneous disease with poor prognosis, and the current TNM staging system performs poorly for prognosis. TME plays an important role in TNBC progression, and macrophages are a vital immune component in TME.
• In this study, 4 genes were identified and incorporated into a macrophage polarization-related prognostic index for the survival prediction of patients with early-stage TNBC. Our model possessed good accuracy and consistency in both the training and validation cohorts.
What is the implication, and what should change now?
• The current prognostic system for TNBC survival does not satisfy the need and should be improved or replaced. Our model can predict individual prognosis based on MRPI and thus facilitate the selection of treatment and follow-up options.
Introduction
Triple-negative breast cancer (TNBC), known as the most aggressive subtype of breast cancer, accounts for around 15% of all breast cancer cases (1). It is highly invasive and heterogeneous and is prone to metastasizing in the early stage. Even after application of aggressive treatment, nearly 40% of patients with TNBC still succumb to the disease within 5 years (2,3), and thus TNBC has the worst survival prognosis of among the breast cancer subtypes.
An increasing amount of evidence indicates that the tumor microenvironment (TME) has a key role in the progression of TNBC (4-6). As a vital component of immune cells, macrophages are pivotal to the immune response. Under the influence of TME, monocytes differentiate into M0 macrophages and then polarize into classically activated type 1 (M1-like) and alternatively activated type 2 (M2-like) macrophages. M1-like macrophages mainly play pro-inflammatory and antitumor roles, while M2-like macrophages are crucially involved in aggravating tumor development (7). During tumor development, M1-like and M2-like can be transformed into each other (8). In TNBC tumors, there exists significant infiltration of macrophages (9). Previous studies also have explored the function of macrophages in immune regulation and tumor therapy (10,11).
Due to a deficiency of estrogen receptor (ER), progesterone receptor (PR), and human epidermal growth factor receptor 2 (HER2), limited treatment options are left for patients with TNBC, and thus chemotherapy remains the primary systematic treatment (2). Although TNBC is highly aggressive, some early-stage patients can be completely cured with chemotherapy; nonetheless the treatment of patients with advanced TNBC is exceedingly complicated, and the treatment effect is poor. The median overall survival (OS) with current treatment options is only 13 to 18 months for those who develop metastatic disease (12,13). Therefore, early detection, early diagnosis, and early treatment of early TNBC is particularly important. In clinical practice, the prognosis of early-stage TNBC varies considerably, and traditional TNM staging performs poorly for survival prediction. The high degree of heterogeneity poses critical challenges to the diagnosis and treatment of TNBC, and effective biomarkers that can help guide individualized treatment in early-stage TNBC still remains a clinically unmet need.
Several studies have reported that the regulation of macrophage polarization can affect the progression of TNBC (14-16). Specifically, Xu et al. mapped the TME of TNBC and explored the M1-like macrophage-associated prognostic genes (17). However, these studies did not construct a prognostic model for clinical usage. There are some studies have been published for prognosis prediction for TNBC. Guo et al. retrieved clinical and survival data of 21,419 TNBC patients from the Surveillance, Epidemiology, and End Results Program (SEER) database and developed a nomogram to predict the OS and breast cancer specific survival (18). The C-indexes for their model were larger than 0.77 in both internal and external validations. However, their external validation data were also from the SEER database, which was not strictly “external validation” and might exaggerate the predictive accuracy in validation cohort (18). Shi et al. also developed nomograms for overall and disease-free survival prediction in TNBC patients (19). However, their model was developed with limited patient numbers (N=255) in only one center. This may bring some concern when extend their model in other medical institutions. Given the critical role of macrophages in TNBC immune response, we believe a model based on macrophages may yield great survival prediction in TNBC. Therefore, we constructed and validated a predictive model based on a macrophage polarization-related prognostic index (MRPI) to predict survival outcomes in patients with early-stage TNBC. Using prognostic stratification, our model can facilitate the identification of patients with poor-prognosis as candidates for intensive treatment so as to improve the survival prognosis of these patients. Figure 1A presents the study’s technical procedure and workflow while Figure 1B outlines the patient screening process. We present the following article in accordance with the TRIPOD and MDAR reporting checklists (available at https://gs.amegroups.com/article/view/10.21037/gs-23-6/rc).
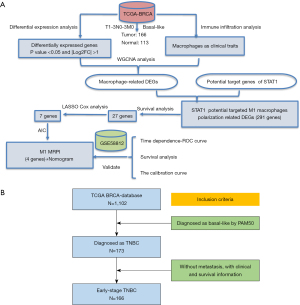
Methods
Selection of patients and acquisition of data
We collected RNA sequencing (RNA-seq) data and the clinicopathological details of patients with breast cancer (BRCA) from The Cancer Genome Atlas (TCGA) database (https://portal.gdc.cancer.gov/projects/TCGA-HNSC) and the GSE58812 data set in the Gene Expression Omnibus (GEO) database (https://www.ncbi.nlm.nih.gov/geo/). Due to the incomplete information of ER, PR and HER2 in some samples, PAM50 typing was used to select basal-like samples for subsequent analysis. The study only enrolled patients who met the following 2 criteria: (I) with complete survival and prognostic information including follow-up time and vital status and (II) without distant metastasis.
Finally, a total of 166 early TNBC cases and 113 normal tissue cases were included in TCGA cohort. We chose TCGA data set as a training set because it had a relatively sufficient number of cases and complete clinical information. The GSE58812 cohort, which included 76 samples obtained through the PAM50 method, was used as the validation set. We extracted the following data for each patient in TCGA data set: age, race, tumor size, lymph node status, and tumor stage. Each of these clinical characteristics was treated as a categorical variable. Age was divided into ≤50 years old and >50 years old groups; race included White, Asian, Black, and other race categories; lymph node status was divided into no and yes groups; and tumor stage was divided into I + IIA and IIB + III groups. OS was our primary outcome. In TCGA cohort, the follow-up days were acquired through investigation of the days to last follow-up or days to death; the interest event outcome was decided according to vital status, and death was regarded as the event of interest. In the GEO cohort, we extracted the messenger RNA (mRNA) expression data and survival outcomes. For model building, TCGA dataset included 163 cases, and the median follow-up time was 2.08 years. During the follow-up period, 18 deaths occurred. Meanwhile, the GEO data set included 76 cases, the median follow-up time was 6.92 years, and 3 deaths occurred during the follow-up time. After data screening, there were no missing data in our analysis. We included all eligible data from the GEO and TCGA databases to maximize the sample size in our study and gain the best predictive ability and generalizability of our developed model.
Moreover, online platform Cistrome Cancer (http://cistrome.org/CistromeCancer/) was used to acquire potential signal transducer and activator of transcription 1 (STAT1) target gene data in BRCA by combining TCGA molecular profiling data with public transcription factor chromatin immunoprecipitation sequencing (ChIP-Seq) profiles (20). TCGA data portal (https://portal.gdc.cancer.gov/) was used to obtain the somatic mutation profiles. The study was conducted in accordance with the Declaration of Helsinki (as revised in 2013).
Differential expression analysis
In TCGA cohort, differentially expressed genes (DEGs) were screened by using the “DEseq2” package in R (The R Foundation for Statistical Computing; adjusted P value <0.05, |log2fold change [FC]| >1), followed by variance stabilizing transformation (VST) transformation of the expression profile. Principal component analysis (PCA) was performed to visualize the disparity between the 2 groups with the R package “FactoMineR”, and the percentage of explained variances was analyzed with the R package “Factoextra”. To visualize the DEGs, R packages “ggpubr” and “ComplexHeatmap” were used to draw volcano maps and heat maps, respectively.
Tumor-infiltrating immune cell analysis
The Cell-type Identification by Estimating Relative Subsets of RNA Transcripts (CIBERSORT) algorithm is a widely used and effective tool for calculating the fractions of the 22 types of tumor-infiltrating immune cells with deconvolution analysis (21). First, we downloaded 22 immune cell reference marker gene expressions from the CIBERSORT website (https://CIBERSORT.stanford.edu/). We then used the R program “CIBERSORT” package to estimate the abundance of different immune cell subtypes in each TCGA sample. Subsequently, we applied the Wilcoxon test to compare the differences of infiltrating immune cells between tumor and normal tissue.
Weighted gene co-expression network analysis (WGCNA)
We selected immune cells of interest as clinical traits. With the help of the R package “WGCNA”, trait data and expression data were used to build a scale-free co-expression network to develop the gene module that was most strongly associated with macrophages (22). First, the expression profile underwent a hierarchical clustering analysis to identify any outliers. Via the calculation of the Pearson correlation coefficient between genes, the expression data were transformed into a similarity matrix. The similarity matrix was then converted into an adjacency matrix. Next, to describe the strength of the relationship between genes, the adjacency matrix was converted into a topology matrix using the topological overlap measure (TOM). Finally, a dynamic hybrid cutting method was applied for module identification.
Identification of candidate genes and construction of the M1-like MRPI
Among the modules, we selected the hub module with the highest relevance: the M1-like macrophage. Furthermore, we chose every gene in the hub module and created a protein-protein interaction (PPI) network using the Search Tool for the Retrieval of Interacting Genes (STRING; https://string-db.org/) database. The minimal interaction score was set as the highest confidence level (0.9) to look for central nodes (23). In order to visualize the top 20 genes according to the Matthews correlation coefficient (MCC) value, we made use of the cytoHubba plugin in Cytoscape (24). We identified candidate genes according to the intersection of genes in the hub module and those targeted by core genes in the PPI network through Venn analysis (http://bioinformatics.psb.ugent.be/webtools/Venn/). To gain insight into the function of candidate genes, we used the Metascape web tool (http://metascape.org) for enrichment analysis to identify the top 20 pathways and processes (25). The candidate genes were further screened by univariate regression and least absolute shrinkage and selection operator (LASSO) regression for the most robust genes (26). The minimum Akaike information criterion (AIC) value was selected to construct the final model. The top 20 pathways and processes were visualized using the Metascape web tool (http://metascape.org) for enrichment analysis (17). Univariate regression and LASSO regression were further used to screen the candidate genes for the most robust genes (18). To build the final Model, the minimum AIC value was chosen.
Validation of the MRPI model
The Harrell’s concordance index (CI), calibration curve and time-dependent receiver operating characteristic curve (ROC) were used to validate the accuracy of the MRPI model in training and validation sets. We calculated the area under the curve (AUC) of 2-year, 3-year and 5-year survival prediction in both training and validation sets.
The CI indicates the performance of the predictive model. A value of 0.7 or higher indicates that the model possesses relatively good discriminative ability. The calibration curve reflects the accuracy of prediction model. If the predictive model perfectly predicted the outcomes, the calibration curve will fall along the diagonal 45° line. ROC is also widely used for model evaluation. Generally speaking, when the AUC is larger than 0.7, the model shows relatively good accuracy.
Additional bioinformatic
R software (version 4.0.0; http://www.rproject.org) was used to analyze the data and create graphs. To obtain a clustering based on the gene expression matrix, nonnegative matrix factorization (NMF) consensus clustering (R package “NMF”) was used to reduce the dimensionality of the original matrix. To delineate the survival curves, the Kaplan-Meier method was used, while the log-rank test was used to assess the survival difference. With the help of the R package “survivalROC”, it was possible to compare the predictive capacity of survival among different variables using time-dependent receiver operating characteristic (tROC) analysis. Calibration curves and nomograms were drawn using R package “rms”, and risk assessment scatter charts were plotted using the R package “ggrisk”. Gene set enrichment analysis (GSEA) software (version 4.1.0; http://www.gsea-msigdb.org) and the Metascape network tool were used to perform enrichment analysis (25,27). The R package “maftools” was used to analyze somatic mutation data in Mutation Annotation Format (MAF).
Statistical analyses
Between the 2 groups, categorical variables were compared using the chi-squared test. The Kaplan-Meier survival curve and log-rank test were used to depict and compare the survival differences between the two groups. A two-sided P value ≤0.05 was considered statistically significant.
Results
Differentially expressed genes and immune cell infiltration
A total of 13,875 DEGs between tumor and normal tissues were found in TCGA TNBC cohort, comprising 8,543 upregulated genes and 5,332 downregulated genes (Figure 2A). PCA analysis suggested that these differential genes could distinguish TNBC from normal tissues (Figure 2B). The expression heatmap displayed the top 100 upregulated and downregulated genes in TNBC tissues and normal tissues (Figure 2C).
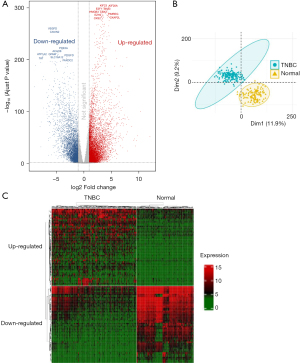
CIBERSORT analysis suggested that macrophages were the most infiltrated immune cells in early TNBC samples (Figure 3A), and there was a substantial distinction in macrophage infiltration compared with normal samples (Figure 3B and Figure S1).
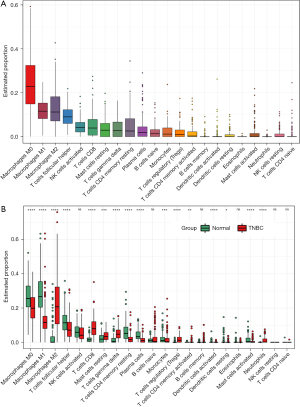
Identification of M1-like macrophage polarization-related genes
Considering the large amount of infiltration of macrophages in TNBC, we selected macrophages as the clinical trait and built a scale-free coexpression network together with differentially expressed genes. Three outliers were excluded from the hierarchical cluster analysis (Figure S2). After the removal of 3 outliers, the samples were clustered based on Euclidean distance (Figure S3). To meet the scale-free network role, we used the soft threshold β =3 (Figure 4A,4B). The 26 gene modules were established by setting the minimum module size to 50 and merging threshold to 0.25 (Figure 4C). Among the 26 modules, the brown module had the strongest connection with the M1-like macrophage-related genes (R2 =0.55; P=2e-14) (Figure 4D). Moreover, 1,106 genes in the brown module were substantially linked with membership degree and gene significance (correlation =0.82; P<1e-200; Figure 4E). As seen in Figure 4D, since genes in the brown module had the highest correlation with their traits, we extracted all genes in the brown module, constructed a PPI network and found STAT1 to be one of the core genes (Figure 4F). Considering that STAT1 has a vital function as a key transcription factor in initiating M1-like macrophage polarization through the JAK-STAT1 pathway (28,29), we intersected the 1,106 genes in M1-like macrophage–related modules with 3,391 genes potentially targeted by STAT1 in breast cancer. Ultimately, 291 candidate genes in the intersection were considered to be M1-like macrophage polarization-related genes (Figure 4G). According to Gene Ontology (GO) analysis, these 291 genes were mostly involved in immune receptor activity and cytokine receptor binding, adaptive immune response, and lymphocyte activation, among other processes (Figure 4H).
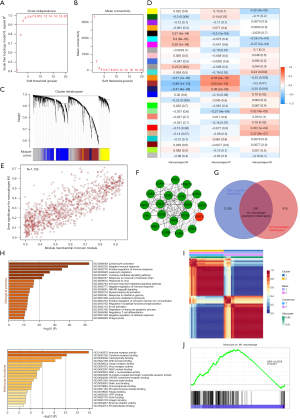
We further clustered 163 TCGA TNBC tumor samples (training set) with NMF analysis based on the expression of the 291 M1-like macrophage polarization-related genes. As shown in Figure 4I, 2 clusters were identified with an optimal k of 2. The M1-like macrophage versus monocyte upregulated gene set pathway was considerably enriched in cluster 1 compared to cluster 2 according to GSEA analysis, suggesting that these 291 candidate genes were involved in M1-like polarization (Figure 4J).
M1-MRPI development for OS prediction in patients with early-stage TNBC
First, the univariate Cox regression algorithm was used to select genes significantly related to OS from 291 candidate genes. In the subsequent analysis, 27 genes with P values less than 0.05 were included. Next, LASSO and Cox analysis was carried out to screen the most robust prognostic genes from the 27 survival-associated genes. To overcome the overfitting effect, the optimal λ value 0.019 was chosen by using 10-fold cross-validation, and 7 genes were screened out (Figure 5A). Following this, a multivariate Cox regression analysis was conducted to determine the role of the 7 genes as an independent predictive factor for patient survival. The forward and backward stepwise regression method was employed to further select the best model. The model was determined when the minimum AIC was 130.14. Finally, 4 genes including GCH1, KIR2DL4, KLHDC7B, and SDS were selected for the M1-MRPI (Table 1). The Cox model accorded with the proportional risk hypothesis (Figure S4). Multivariate Cox regression analysis was used to construct the model for MRPI calculation. To be specific, MRPIs for each sample were calculated as follows: MRPI = (–0.6780 × expression level of GCH1) + (–0.6412 × expression level of KIR2DL4) + (–0.1985 × expression level of KLHDC7B) + (1.1180 × expression level of SDS) (Figure S5).
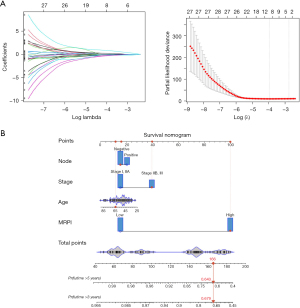
Table 1
Gene symbol | Entrez ID | Full name | Location | HR | P value | Expression |
---|---|---|---|---|---|---|
GCH1 | 2643 | GTP cyclohydrolase 1 | 14q22.2 | 0.54 | 0.018 | Up |
KIR2DL4 | 3805 | Killer cell immunoglobulin like receptor, 2 Ig domains and long cytoplasmic tail 4 | 19q13.42 | 0.29 | 0.004 | Up |
KLHDC7B | 113730 | Kelch domain containing 7B | 22q13.33 | 0.79 | 0.022 | Up |
SDS | 10993 | Serine dehydratase | 12q24.13 | 2.00 | 0.001 | Up |
HR, hazard ratio.
Next, together with the MRPI and other clinicopathological variables (age, lymph node status, race and stage), we explored a MRPI-based prognostic model for quantifying risk assessment in early-stage patients with TNBC. For model building, TCGA data set had 163 cases, and the median follow-up time was 2.08 months. During the follow-up period, 18 deaths occurred. After univariate and multivariate Cox model regression analysis, we found MRPI, age, lymph node status, and stage to be statistically significant independent prognostic variables. Race was not correlated with the prognosis and was excluded from the nomogram building. We developed the nomogram with multivariate Cox regression analysis and plotted the model (Figure 5B) and summarized the univariate and multivariate Cox regression analysis results (Figure 6). By simply summing the risk score in each variable, we obtained a total risk score for a given patient, and then we could acquire the survival probabilities at different times by checking the probabilities according to the risk score. In Figure 5B, the red arrow in the plot represents an example.
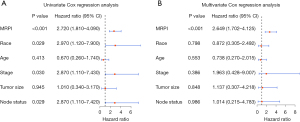
Comparison between MRPI and clinical characteristics
The detailed clinicopathological features of patients in different MRPI groups from the 163 patients with breast cancer in TCGA cohort are shown in Table 2, with the parameters generally balanced (except race) between the MRPI-high and MRPI-low groups. Univariate Cox regression analysis showed that higher MRPI [hazard ratio (HR) = 2.720; 95% CI: 1.810–4.090; P<0.001], Black race (HR: 2.970; 95% CI: 1.120–7.900; P=0.029), more advanced tumor stage (HR: 2.870; 95% CI: 1.110–7.430; P=0.030), and lymph node metastasis (HR: 2.870; 95% CI: 1.110–7.420; P=0.029) were risk factors for patients with TNBC (Figure 6A).
Table 2
Variable | MRPI low (n=82) | MRPI high (n=81) | P value |
---|---|---|---|
Age (years) | 0.566 | ||
≤50 | 35 (42.68%) | 31 (38.27%) | |
>50 | 47 (57.32%) | 50 (61.73%) | |
Race | 0.014 | ||
White and asian | 56 (68.29%) | 40 (49.38%) | |
Black and other | 26 (31.71%) | 41 (50.62%) | |
Tumor size | 0.515 | ||
≤2 cm | 13 (15.85%) | 16 (19.75%) | |
>2 cm | 69 (84.15%) | 65 (80.25%) | |
LN status | 0.380 | ||
No | 56 (68.29%) | 50 (61.73%) | |
Yes | 26 (31.71%) | 31 (38.27%) | |
Tumor stage | 0.478 | ||
I + IIA | 54 (65.85%) | 49 (60.49%) | |
IIB + III | 28 (34.15%) | 32 (39.51%) |
TCGA, The Cancer Genome Atlas; MRPI, macrophage polarization-related prognostic index; LN, lymph node.
Furthermore, despite control for other clinicopathological markers, multivariate Cox regression analysis revealed that MRPI remained an independent predictive predictor (HR: 2.649; 95% CI: 1.702–4.125; P<0.001; Figure 6B).
Evaluation and validation of the MRPI prognostic model
We further evaluated and validated the model from different perspectives. A GEO cohort was used as the validation cohort. The risk assessment scatter plot revealed that patients could be classified into high- and low-MRPI groups when the median cutoff values were –1.87 and 6.44 in TCGA and GEO cohorts, respectively. The survival time decreased and the mortality rate increased with increasing MRPI score (Figure 7A). Subsequently, using the median MRPI as the cutoff value, we categorized patients into 2 groups: high MRPI and low MRPI. Kaplan-Meier analysis revealed that patients with a higher MRPI had a considerably worse outcome (P<0.001; Figure 7B).
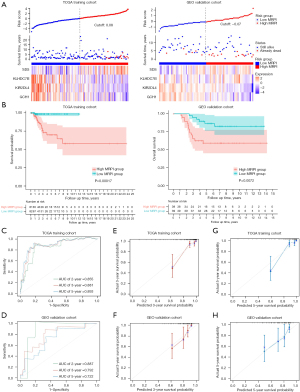
The time-dependent ROC curves showed that the area under the curves (AUCs) of 2-, 3-, and 5-year survival prediction were 0.855, 0.881, and 0.893, respectively, in TCGA group (Figure 7C). Meanwhile, the CI was 0.858 after 1,000 bootstrap repeats, and the corrected CI was 0.834. These results indicated that our prognostic model had a high accuracy. Consistent with the TCGA cohort’s reliable results, the predictive AUC of MRPI for the patients’ 2-, 3-, and 5-year survival rates in the GEO group were 0.887, 0.792, and 0.722, respectively (Figure 7D). Furthermore, the 3- and 5-year calibration curves of the 2 cohorts also support the consistency of MRPI (P=0.0072; Figure 7E-7H).
Enriched pathways and molecular characteristics in the different MRPI groups
GSEA was used to examine the gene sets that were enriched in various MRPI subgroups. Compared with those of MRPI-high group, the gene sets of the MRPI-low group were enriched in inflammatory response, interferon-γ (IFN-γ) response, and complement response [P<0.05; false discovery rate (FDR) <0.25; Figure 8A], suggesting possible active antitumor immune responses in the MRPI-low group. Next, we analyzed the genetic mutations in the 2 MRPI- groups, and the top 20 gene mutations and 7 mutation types are shown in Figure 8B. In the 2 groups, missense variations accounted for the highest proportion of mutation types, with TP53 being the most commonly mutated gene. However, the mutation type and mutation location of TP53 were different (Figure 8C). In addition, the mutation rates of TP53, TTN, and MUC16 were higher than 10% in both groups.
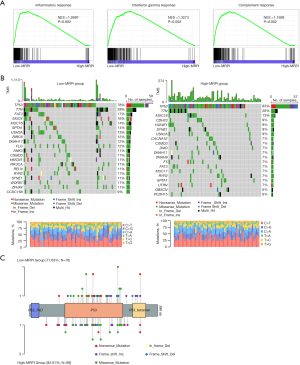
Discussion
As the most deleterious breast cancer subtype, TNBC has a terrible prognosis. Accumulated evidence indicates that immune regulation is critical in the progression of TNBC (30,31). Therefore, a deeper understanding from the perspective of the TME may provide new ideas for clinicians to improve the effectiveness of therapies in TNBC.
In our study, we screened the DEGs between TNBC and normal tissue, and further used WGCNA and CIBERSORT to identify macrophage modules. Univariate, LASSO, and multivariate Cox analyses were performed to construct the MRPI for predicting TNBC prognosis. The MRPI included 4 genes: GCH1, KIR2DL4, KLHDC7B, and SDS. Patients with low MRPI had a better prognosis than did those with high MRPI. ROC curves, CI, and calibration curves indicated that the model had good predictive ability and high consistency. Traditional clinical variables were included in the Cox multivariate analysis with MRPI, which demonstrated that MRPI was still an independent prognostic factor. However, our model has a few limitations that should be noted. First, it was calculated through the expression of 4 genes, which may limit the practicability of our model in clinic due to the cost and difficulties of gene expression detection; second, our results were verified retrospectively, and thus prospective validation may be required to confirm the reliability and clinical utility of our model.
We also developed a nomogram combining MRPI with clinicopathological characteristics for the clinical prediction and prognosis of patients with early-stage TNBC. With our model, we can identify poor-prognosis patients with MRPIs and intervene with intensive treatment so as to improve the survival prognosis of these patients. Moreover, our study may also be informative for exploring the progression of early-stage TNBC.
The 4 genes in the prognostic model also play important roles in a variety of cancer types and have been widely reported on. GCH1, also known as GTP cyclohydrolase 1, is a rate-limiting enzyme in the production of BH4 synthesis. Published studies have reported on both the pro- and antitumor effects of GCH1 in a variety of carcinomas. One study revealed that inhibiting GCH1 slowed tumor development and enhanced macrophage M2-like polarization (32). Another study also demonstrated that high GCH1 expression promotes TNBC proliferation and contributes to poor survival (33). These studies point to GCH1 as having protumor properties. However, other research has shown that GCH1 overexpression can lead to elevated levels of BH4 synthesis, which can stimulate CD4 and CD8 T cell responsiveness, thus the enhancing antitumor activity of GCH1 in vivo (34). The functions of a specific gene may vary considerably across different tumor type and may depend highly on the TME and the interacted proteins. Therefore, the specific function of these genes in patients with early-stage TNBC requires further exploration.
KIR2DL4 (killer cell immunoglobulin like receptor, 2 Ig domains and long cytoplasmic tail 4) is an atypical member of the KIR family, is expressed in natural killer (NK) cells (35), and participates in the stimulation of NK cell activity and secretion of IFN-γ (36). One recent study has indicated that the binding of KIR2DL4 with the HLA-G ligand can inhibit antibody-dependent cell-mediated cytotoxicity (ADCC) and contribute to trastuzumab resistance in breast cancer (37).
KLHDC7B (Kelch domain containing 7B) belongs to a protein-coding gene containing a Kelch domain that is composed of a 4-stranded β-sheet of the Kelch motif that serves as 1 blade of a β-propeller structure (38). According to research, KLHDC7B is overexpressed in breast cancer and may facilitate the malignant growth of disease (39,40). In another study, it was found that those with pancreatic cancer had higher levels of the long noncoding RNA lKLHDC7B reverse transcription (KLHDC7B-DT), which is associated with a worse prognosis. Furthermore, KLHDC7B-DT can promote macrophage M2-like polarization and accelerate pancreatic ductal adenocarcinoma (41). Finally, SDS (serine dehydratase) encodes 1 of the 3 enzymes that are involved in metabolizing serine and glycine. Many studies have shown that high rates of serine biosynthesis may promote the growth of tumor cells (42,43).
Conclusions
Our research showed that MRPI is a reliable tool for predicting the prognosis of early-stage TNBC. The prognosis of patients with lower MRPI scores was better than that of patients with higher MRPIs.
Acknowledgments
We acknowledge TCGA and the GEO databases for providing these publicly available data sets included in our study.
Funding: This study was supported by the Natural Science Foundation of Guangdong Province (No. 2019A1515011945) and the Sun Yat-sen University Clinical Research 5010 Program (No. 2017011).
Footnote
Reporting Checklist: The authors have completed the TRIPOD and MDAR reporting checklists. Available at https://gs.amegroups.com/article/view/10.21037/gs-23-6/rc
Conflicts of Interest: All authors have completed the ICMJE uniform disclosure form (available at https://gs.amegroups.com/article/view/10.21037/gs-23-6/coif). The authors have no conflicts of interest to declare.
Ethical Statement: The authors are accountable for all aspects of the work in ensuring that questions related to the accuracy or integrity of any part of the work are appropriately investigated and resolved. The study was conducted in accordance with the Declaration of Helsinki (as revised in 2013).
Open Access Statement: This is an Open Access article distributed in accordance with the Creative Commons Attribution-NonCommercial-NoDerivs 4.0 International License (CC BY-NC-ND 4.0), which permits the non-commercial replication and distribution of the article with the strict proviso that no changes or edits are made and the original work is properly cited (including links to both the formal publication through the relevant DOI and the license). See: https://creativecommons.org/licenses/by-nc-nd/4.0/.
References
- Gruosso T, Gigoux M, Manem VSK, et al. Spatially distinct tumor immune microenvironments stratify triple-negative breast cancers. J Clin Invest 2019;129:1785-800. [Crossref] [PubMed]
- Jia H, Truica CI, Wang B, et al. Immunotherapy for triple-negative breast cancer: Existing challenges and exciting prospects. Drug Resist Updat 2017;32:1-15. [Crossref] [PubMed]
- Pawar A, Prabhu P. Nanosoldiers: A promising strategy to combat triple negative breast cancer. Biomed Pharmacother 2019;110:319-41. [Crossref] [PubMed]
- Deepak KGK, Vempati R, Nagaraju GP, et al. Tumor microenvironment: Challenges and opportunities in targeting metastasis of triple negative breast cancer. Pharmacol Res 2020;153:104683. [Crossref] [PubMed]
- Malla RR, Vasudevaraju P, Vempati RK, et al. Regulatory T cells: Their role in triple-negative breast cancer progression and metastasis. Cancer 2022;128:1171-83. [Crossref] [PubMed]
- Xiao Y, Ma D, Zhao S, et al. Multi-Omics Profiling Reveals Distinct Microenvironment Characterization and Suggests Immune Escape Mechanisms of Triple-Negative Breast Cancer. Clin Cancer Res 2019;25:5002-14. [Crossref] [PubMed]
- Zhou D, Huang C, Lin Z, et al. Macrophage polarization and function with emphasis on the evolving roles of coordinated regulation of cellular signaling pathways. Cell Signal 2014;26:192-7. [Crossref] [PubMed]
- Murray PJ. Macrophage Polarization. Annu Rev Physiol 2017;79:541-66. [Crossref] [PubMed]
- Lu X, Yang R, Zhang L, et al. Macrophage Colony-stimulating Factor Mediates the Recruitment of Macrophages in Triple negative Breast Cancer. Int J Biol Sci 2019;15:2859-71. [Crossref] [PubMed]
- Noy R, Pollard JW. Tumor-associated macrophages: from mechanisms to therapy. Immunity 2014;41:49-61. [Crossref] [PubMed]
- Ruffell B, Coussens LM. Macrophages and therapeutic resistance in cancer. Cancer Cell 2015;27:462-72. [Crossref] [PubMed]
- Yin L, Duan JJ, Bian XW, et al. Triple-negative breast cancer molecular subtyping and treatment progress. Breast Cancer Res 2020;22:61. [Crossref] [PubMed]
- Vagia E, Mahalingam D, Cristofanilli M. The Landscape of Targeted Therapies in TNBC. Cancers (Basel) 2020;12:916. [Crossref] [PubMed]
- Yang R, Xie Y, Li Q, et al. Ruyiping extract reduces lung metastasis in triple negative breast cancer by regulating macrophage polarization. Biomed Pharmacother 2021;141:111883. [Crossref] [PubMed]
- Li J, Cai H, Sun H, et al. Extracts of Cordyceps sinensis inhibit breast cancer growth through promoting M1 macrophage polarization via NF-κB pathway activation. J Ethnopharmacol 2020;260:112969. [Crossref] [PubMed]
- Meng Z, Zhang R, Wang Y, et al. miR-200c/PAI-2 promotes the progression of triple negative breast cancer via M1/M2 polarization induction of macrophage. Int Immunopharmacol 2020;81:106028. [Crossref] [PubMed]
- Xu B, Sun H, Song X, et al. Mapping the Tumor Microenvironment in TNBC and Deep Exploration for M1 Macrophages-Associated Prognostic Genes. Front Immunol 2022;13:923481. [Crossref] [PubMed]
- Guo LW, Jiang LM, Gong Y, et al. Development and validation of nomograms for predicting overall and breast cancer-specific survival among patients with triple-negative breast cancer. Cancer Manag Res 2018;10:5881-94. [Crossref] [PubMed]
- Shi H, Wang XH, Gu JW, et al. Development and Validation of Nomograms for Predicting the Prognosis of Triple-Negative Breast Cancer Patients Based on 379 Chinese Patients. Cancer Manag Res 2019;11:10827-39. [Crossref] [PubMed]
- Mei S, Meyer CA, Zheng R, et al. Cistrome Cancer: A Web Resource for Integrative Gene Regulation Modeling in Cancer. Cancer Res 2017;77:e19-22. [Crossref] [PubMed]
- Newman AM, Liu CL, Green MR, et al. Robust enumeration of cell subsets from tissue expression profiles. Nat Methods 2015;12:453-7. [Crossref] [PubMed]
- Langfelder P, Horvath S. WGCNA: an R package for weighted correlation network analysis. BMC Bioinformatics 2008;9:559. [Crossref] [PubMed]
- Szklarczyk D, Gable AL, Lyon D, et al. STRING v11: protein-protein association networks with increased coverage, supporting functional discovery in genome-wide experimental datasets. Nucleic Acids Res 2019;47:D607-13. [Crossref] [PubMed]
- Shannon P, Markiel A, Ozier O, et al. Cytoscape: a software environment for integrated models of biomolecular interaction networks. Genome Res 2003;13:2498-504. [Crossref] [PubMed]
- Zhou Y, Zhou B, Pache L, et al. Metascape provides a biologist-oriented resource for the analysis of systems-level datasets. Nat Commun 2019;10:1523. [Crossref] [PubMed]
- Zhu X, Tian X, Sun T, et al. GeneExpressScore Signature: a robust prognostic and predictive classifier in gastric cancer. Mol Oncol 2018;12:1871-83. [Crossref] [PubMed]
- Subramanian A, Tamayo P, Mootha VK, et al. Gene set enrichment analysis: a knowledge-based approach for interpreting genome-wide expression profiles. Proc Natl Acad Sci U S A 2005;102:15545-50. [Crossref] [PubMed]
- Li L, Wei C, Cai S, et al. TRPM7 modulates macrophage polarization by STAT1/STAT6 pathways in RAW264.7 cells. Biochem Biophys Res Commun 2020;533:692-7. [Crossref] [PubMed]
- Ding N, Wang Y, Dou C, et al. Physalin D regulates macrophage M1/M2 polarization via the STAT1/6 pathway. J Cell Physiol 2019;234:8788-96. [Crossref] [PubMed]
- Karn T, Jiang T, Hatzis C, et al. Association Between Genomic Metrics and Immune Infiltration in Triple-Negative Breast Cancer. JAMA Oncol 2017;3:1707-11. [Crossref] [PubMed]
- Kim IS, Gao Y, Welte T, et al. Immuno-subtyping of breast cancer reveals distinct myeloid cell profiles and immunotherapy resistance mechanisms. Nat Cell Biol 2019;21:1113-26. [Crossref] [PubMed]
- Pickert G, Lim HY, Weigert A, et al. Inhibition of GTP cyclohydrolase attenuates tumor growth by reducing angiogenesis and M2-like polarization of tumor associated macrophages. Int J Cancer 2013;132:591-604. [Crossref] [PubMed]
- Wei JL, Wu SY, Yang YS, et al. GCH1 induces immunosuppression through metabolic reprogramming and IDO1 upregulation in triple-negative breast cancer. J Immunother Cancer 2021;9:e002383. [Crossref] [PubMed]
- Cronin SJF, Seehus C, Weidinger A, et al. The metabolite BH4 controls T cell proliferation in autoimmunity and cancer. Nature 2018;563:564-8. [Crossref] [PubMed]
- Faure M, Long EO. KIR2DL4 (CD158d), an NK cell-activating receptor with inhibitory potential. J Immunol 2002;168:6208-14. [Crossref] [PubMed]
- Rajagopalan S, Fu J, Long EO. Cutting edge: induction of IFN-gamma production but not cytotoxicity by the killer cell Ig-like receptor KIR2DL4 (CD158d) in resting NK cells. J Immunol 2001;167:1877-81. [Crossref] [PubMed]
- Zheng G, Guo Z, Li W, et al. Interaction between HLA-G and NK cell receptor KIR2DL4 orchestrates HER2-positive breast cancer resistance to trastuzumab. Signal Transduct Target Ther 2021;6:236. [Crossref] [PubMed]
- Adams J, Kelso R, Cooley L. The kelch repeat superfamily of proteins: propellers of cell function. Trends Cell Biol 2000;10:17-24. [Crossref] [PubMed]
- Martín-Pardillos A, Cajal SRY. Characterization of Kelch domain-containing protein 7B in breast tumours and breast cancer cell lines. Oncol Lett 2019;18:2853-60. [Crossref] [PubMed]
- Zhang G, Fan E, Yue G, et al. Five genes as a novel signature for predicting the prognosis of patients with laryngeal cancer. J Cell Biochem 2020;121:3804-13. [Crossref] [PubMed]
- Li MX, Wang HY, Yuan CH, et al. KLHDC7B-DT aggravates pancreatic ductal adenocarcinoma development via inducing cross-talk between cancer cells and macrophages. Clin Sci (Lond) 2021;135:629-49. [Crossref] [PubMed]
- Snell K. Enzymes of serine metabolism in normal, developing and neoplastic rat tissues. Adv Enzyme Regul 1984;22:325-400. [Crossref] [PubMed]
- Snell K, Weber G. Enzymic imbalance in serine metabolism in rat hepatomas. Biochem J 1986;233:617-20. [Crossref] [PubMed]
(English Language Editor: J. Gray)