Development and validation of a prognostic model for HER2-low breast cancer to evaluate neoadjuvant therapy
Highlight box
Key findings
• A new typing method (including CD1 and CD0 subtypes) was proposed with ideal performance in predicting pCR rate after neoadjuvant therapy in I-SP2 trial.
What is known and what is new?
• HER2-low breast cancer is a heterogeneous disease, which has been classified into basal and luminal subtypes according to hormone receptor status. The pCR rate varied widely between these two subtypes. It is critical to further subdivide the molecular subtypes in order to guide the neoadjuvant treatment.
• A new typing method (CD1 and CD0 subtypes) refined by deep learning was proposed, which achieved better performance in predicting the pCR rate than the traditional typing method.
What is the implication, and what should change now?
• With the new tying method, clinicians could accurately predict the pCR to neoadjuvant therapy in HER2-low breast cancer, thus effectively helping non-pCR patients to make timely changes to the treatment plan.
Introduction
Breast cancer (BC) is the most common malignant tumor among women in China, and worldwide (1,2). The success of BC treatment is dependent on precise subtyping. Based on the expression status of hormone receptor (HR) and human epidermal growth factor receptor 2 (HER2), BC can be divided into 5 subtypes: luminal A, luminal B1/B2, basal-like, and HER2-positive (3). Accordingly, HER2 is a very important therapeutic target. Trastuzumab, an effective targeted drug for BC with over-expressed HER2, does not work in subtypes with low HER2 expression (3). HER2-low BC, which accounts for 30–51% of all BC, is more common in HR positive cases and has more axillary nodal involvement than patients with an HER2 immunohistochemistry (IHC) score of 0 (4,5). According to the current typing system, HER2-low would be classified as either basal or luminal subtype. In the Destiny Breast 04 trial, a novel antibody-drug conjugate (ADC) drug named T-DXd (trastuzumab deruxtecan) showed inspiring results in improving the progression-free survival (PFS) by 4.7 months for HER2-low metastatic BC (6). However, due to the heterogeneity of tumor, some patients still show resistance to the advanced treatment. It is critical to further subdivide the molecular subtypes in order to guide treatment for HER2-low BC.
Patients who achieved pathological complete response (pCR) to neoadjuvant therapy could get a longer PFS (7). Therefore, pCR was commonly used as the surrogate endpoint for clinical trial. By machine learning, researchers developed a variety of effective pCR prediction models with gene expression, clinicopathology, and medical image, but still lacking in-depth analysis for HER2-low BC (8-11). Applying deep learning did bring better predictive performance, but few researchers could explain the weighting results from black boxes, thus limiting its clinical generalizability. In recent years, the axiomatic attribution method based on integral gradients had become an edge tool for deconstructing the black box within deep learning (12,13).
The I-SPY2 trial is an ongoing multi-center, phase II neoadjuvant platform trial for high-risk, early-stage BC to rapidly identify new treatments and treatment combinations with increased efficacy compared to standard-of-care (14). Its clinical, pathological, and messenger RNA (mRNA) data were released in May 2022. In this study, we attempt to refine the molecular subtypes to predict the efficacy of neoadjuvant therapy for HER2-low expression BC using I-SPY2 datasets through the deep learning method. We present the following article in accordance with the STARD reporting checklist (available at https://gs.amegroups.com/article/view/10.21037/gs-22-729/rc).
Methods
Data preparation
The data in this paper were all derived from the multi-arm adaptive randomization I-SPY2 trial, which included 987 cases of neoadjuvant therapy in BC. The clinical and pathological data were retrieved from GSE196096 (https://www.ncbi.nlm.nih.gov/geo/query/acc.cgi?acc=GSE196096). The mRNA data were obtained from GSE194040 which comprised 19,134 genes (https://www.ncbi.nlm.nih.gov/geo/query/acc.cgi?acc=GSE194040). All these data were published on 25 May 2022 (http://www.ispytrials.org/results/data). The study was conducted in accordance with the Declaration of Helsinki (as revised in 2013).
Inclusion and exclusion criteria
The inclusion criteria were as follows: (I) patients diagnosed with HER2-low BC, which was defined as having a 1+ or 2+ HER2 IHC score and a negative result of in situ hybridization; (II) patients with complete pathological information after neoadjuvant therapy. The exclusion criteria were as follows: patients who lacked clinical, pathological (biomarker scores), or mRNA data.
Data preprocessing
The data were pre-processed by (I) filtering missing data with the not available (NA) ratio >30%; (II) normalizing 3 omics data to the interval 0 to 1, separately. In order to reduce the fitting difficulty when training samples were far less than its variables, we refined mRNA data by differential gene analysis with the two-sided Wilcoxon rank sum test. The cut-off value was set at P<0.05.
All pCR samples of eligible subjects were randomly divided into training and validation sets by 8:2. The non-pCR cases were corporated into the above datasets with 1:1 ratio. The rest non-pCR cases were served as the test set (Figure 1).
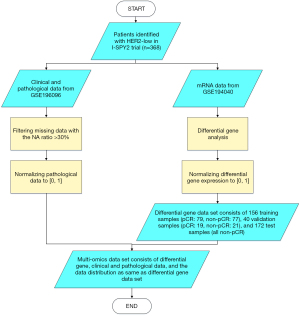
Modeling 1-dimensional (1D) omics data
The difficulty of model fusion would decrease when there were no dimensional differences in the model inputs. The method of feature extraction could be generalized across omics data. The flow chart of technology for 1D data modeling is shown in Figure 2. Fusion methods for omics data could be divided into 3 types: (I) merging input variables; (II) connecting the feature in extraction stage; (III) fusing the model in the decision stage (14). The feature connecting method could well balance over-fitting and weak correlation in most of the complex data fusion. However, it required more training samples to extract high dimension features. As a priori reference, the t-distributed stochastic neighbor embedding (t-SNE) method was used to visualize the multi-omics data distribution after dimensionality reduction (15,16) (Figure S1). Although t-SNE had a strong visualization ability, its visualization could only represent probability distribution, not the true classes-distance.
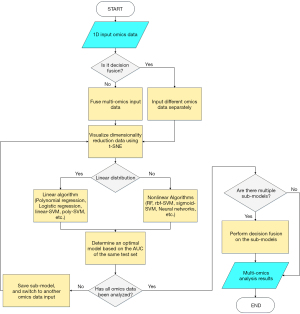
RF classifier
The RF is a representative method of bagging ensemble learning. By training multiple decision trees in parallel with random samples, RF could achieve strong classification ability without overfitting (17). An RF classifier was developed through the Sklearn-package (https://pypi.org/project/sklearn/) in this study. Specifically, the number of decision trees was set as 10, and the minimum number of training samples for each leaf node was set as 5.
SVM classifier
With the additional kernel functions, SVM had improved classification efficiency for high-dimensional data. Radial basis function (RBF) and sigmoid were commonly utilized for nonlinear mapping (18). In this study, 4 kernel functions were tested to optimize the SVM classifier by Sklearn-package. Specifically, for better generalization ability, the classification penalty parameter was set to 0.8, and the degree of polynomial was set to 2.
Neural network classifier
To derive special structures from multi-omics inputs, neural networks were employed. In terms of 1D data, fully connected neural network (FCNN), recurrent neural network (RNN), convolutional neural network (CNN) and transformer were all candidate methods for establishing network structures (19).
Since the input variables were time-independent, single-channel, and relatively small sample size, FCNN was the best option for this study. A classifier based on FCNN was created with through the Keras package (https://keras.io/) (Figure 3).
Features extraction
After training the classifier, the significance analysis was adopted to extract features highly correlated with pCR. Features analysis function dedicated to RF classifier was embedded in the Sklearn-package. Linear SVM was applied to distinguish the features though regression coefficients. FCNN classifier was analyzed by introducing integral gradients and unsupervised hierarchical clustering (12,20). The results of features analysis were validated by Gene Ontology (GO) enrichment analysis (21). Specifically, assuming each sample had n input attributes, the samplesi={x1, x2,…, xn}, i∈{1,2,…, number of samples}. Since the input of each sample had been normalized, all reference baselines could be set to 0 (rei={0, 0,…, 0). The value of significance attribution could be calculated with the Eq. [1]. The f(·) was defined as the cumulative probability of distribution function.
In Eq. [1], m should be determined according to data distribution (in this study, it was 50).
Then, all attributes of samples were merged and normalized to yield a matrix for visualization in a clustered heatmap.
Statistical analysis
The cut-off value of classification was based on iteratively selected prediction probability of each sample. All the true and false positive rates were recorded respectively into y-axis and x-axis of the receiver operating characteristic (ROC) curve. The area under the curve (AUC) of the ROC was calculated to reflect the discrimination of the predictive model. In this study, a model that could achieve an AUC value of 0.85 or above was considered excellent. Meanwhile, all the tests were performed by the two-sided Wilcoxon rank sum test. The P value of 0.05 was considered as the threshold for significance.
Results
Data processing
A total of 368 HER2-low BC cases were included in this study. They were divided into a training set (n=156, 79 pCR), a validation set (n=40, 19 pCR), and a test set (n=172, all non-pCR). The distribution of the pCR cases of BC in I-SPY2 is shown in Figure S2. The clinical characteristics of participants is shown in Table 1.
Table 1
Patient characteristics | Total patients | pCR |
---|---|---|
HR, n (%) | ||
HR-positive | 211 (57.3) | 35 (16.6) |
HR-negative | 157 (42.7) | 63 (40.1) |
MammaPrint, n (%) | ||
MP2-positive | 174 (47.3) | 70 (40.2) |
MP2-negative | 194 (52.7) | 28 (14.4) |
Immune responsive, n (%) | ||
Immune-positive | 178 (48.4) | 69 (38.8) |
Immune-negative | 190 (51.6) | 29 (15.3) |
DRD/platinum-responsive, n (%) | ||
DRD-positive | 146 (39.7) | 65 (44.5) |
DRD-negative | 221 (60.0) | 33 (14.9) |
Not available | 1 (0.3) | 0 (0.0) |
Arms, n (%) | ||
Paclitaxel + AMG 386 (trebananib) | 61 (16.6) | 17 (27.9) |
Paclitaxel + neratinib | 22 (6.0) | 4 (18.2) |
Paclitaxel + MK-2206 (AKT inhibitor) | 28 (7.6) | 8 (28.6) |
Paclitaxel + ganetespib | 56 (15.2) | 14 (25.0) |
Paclitaxel + ganitumab | 51 (13.9) | 13 (25.5) |
Paclitaxel + pembrolizumab | 34 (9.2) | 15 (44.1) |
Paclitaxel + ABT 888 (Veliparib) + carboplatin | 39 (10.6) | 15 (38.5) |
Paclitaxel | 77 (20.9) | 12 (15.6) |
pCR, pathological complete response; HR, hormone receptor; DRD, DNA repair deficient; AMG, company Amgen; MK, company Merck; AKT, protein kinase B; ABT, company AbbVie.
In I-SPY2, there were 6 molecular types. The prediction value of pCR/non-pCR across these typing methods is illustrated in Table 2. Although some subtypes had a high specificity of more than 90%, the low sensitivity limited their clinical availability [for example: the specificity of RPS-5: HER2−/Immune−/DNA repair deficiency (DRD).v3+ was 92.6%, whereas the sensitivity was 13.3%]. This was due to insufficient patients enrolled. Take 60% as the cut-off value of sensitivity and specificity for pCR, two subtypes in Table 2 were marked, the triple negative (TN) of the receptor subtype (64.3% sensitivity, 65.2% specificity) and the CD1 of CDn (classification of deep learning) subtype (87.8% sensitivity, 77.4% specificity).
Table 2
Type | Subtypes | Non-pCR (n) | pCR | ||
---|---|---|---|---|---|
n | Sensitivity | Specificity | |||
BP | Basal | 121 | 78 | 79.6% | 54.9% |
Luminal | 146 | 20 | 20.4% | 45.5% | |
HER2 | 1 | 0 | – | – | |
Receptor* | HR+HER2− | 176 | 35 | 35.7% | 34.8% |
TN* | 94 | 63 | 64.3%* | 65.2%* | |
I-SPY2 | HR+HER2−/MP1 | 142 | 20 | 20.4% | 47.4% |
HR+HER2−/MP2 | 34 | 15 | 15.3% | 87.4% | |
TN/MP1 | 24 | 8 | 8.2% | 91.1% | |
TN/MP2 | 70 | 55 | 56.1% | 74.1% | |
RPS-5 | HER2−/immune+ | 109 | 69 | 70.4% | 59.5% |
HER2−/immune−/DRD.v3+ | 20 | 13 | 13.3% | 92.6% | |
HER2−/immune−/DRD.v3− | 140 | 16 | 16.3% | 48.0% | |
RPS-7 | HER2=0.or.low/immune+ | 109 | 69 | 70.4% | 59.5% |
HER2=0.or.low/immune/DRD.v3+ | 20 | 13 | 13.3% | 92.6% | |
HR+/HER2low/immune/DRD.v3− | 107 | 6 | 6.1% | 60.2% | |
HR−/HER2low/immune/DRD.v3− | 33 | 10 | 10.2% | 87.7% | |
PAM50* | Basal* | 97 | 66 | 69.5%* | 63.9%* |
Her2 | 14 | 6 | 6.3% | 94.8% | |
LumA | 62 | 6 | 6.3% | 77.0% | |
LumB | 88 | 15 | 15.8% | 67.3% | |
Normal | 8 | 2 | 2.1% | 97.0% | |
CDn* | CD1* | 61* | 86* | 87.8%* | 77.4%* |
CD0 | 209 | 12 | – | – |
*, both of the sensitivity and specificity were greater than 60%. pCR, pathologic complete response; HER2, human epidermal growth factor receptor 2; BC, breast cancer; BP, BluePrint; HR, hormone receptor; TN, triple negative; MP, MammaPrint; DRD, DNA repair deficiency.
By comparing the mRNA expression between pCR and non-pCR cases, 23 genes with significant differences were identified for model construction (Figure S3). Of the 31 clinical and pathological indicators, 27 features were enrolled for multi-omics modeling by excluding 4 features (ERBB2.Y1248, EGFR.Y1173, mTOR.S2448, and TIE2.Y992) with incomplete information (Table S1).
Model construction
Classical nonlinear fitting algorithms including RF, SVM, and neural networks were applied to establish prediction model by one-dimensional variables (23 significant differential genes). The prediction value of classifiers based on RF and SVM was unsatisfactory, with an AUC of less than 0.85. After 100 epochs of training as shown in Figure S3A, the FCNN method demonstrated excellent fitting ability with AUC =0.89 (Figure 4), which was finally selected for latter multi-omics modeling.
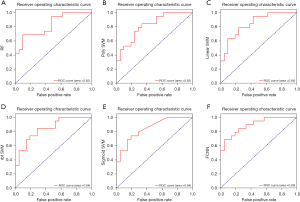
The feature analysis results of the FCNN-based classifier were well correlated with the GO enrichment analysis (Figure S3B and Figure S4). According to the upper clustering lines in Figure S3B, it can be seen that different pCR subgroups have distinguishable differences in attribution values, and the left clustering lines showed the attribution value changes of different inputs during model regression (red: maximum, blue: minimum). Hence, features with a bar color closer to red in Figure S3B were highly related to pCR classification, whereas those closer to blue were weakly related.
As shown in Figure S4A, 4 significant function pathways were found (adjusted P<0.025), which connected to 4 genes (ACOX2, DECR2, PRSS50, and PSMB3) in Figure S4B. Figure S4C-S4F shows that ACOX2, PSMB3, and PRSS50 were the most significant genes (P<0.001), among which 2 (ACOX2 and PSMB3) exhibited high attribution values in Figure S3B.
Fusion of omics data
A multi-omics model containing 23 differential genes, and 27 clinical and pathological features was established through FCNN method. After 100 epochs of training, the multi-omics model was established successfully (the red line implied the success of training, the orange line implied the success of validation) (Figure 5A). The multi-omics model demonstrated excellent predictive ability, with an AUC of 0.92 (Figure 5B). A heatmap of attribute matrix was created to visualize the correlation to pCR classification of all inputs. Bar color toward red represented a higher correlation with pCR (Figure 5C).

Subtypes analysis and refinement
The unsupervised hierarchical clustering method was introduced to identify pCR-related features across different pCR cohorts that had great gradient differences. Some 25 strong pCR-related features were screened out, including Mod7_ERBB2, PARPi7_score, MP2, ICS5_score, PSMB3, VCpred_TN, ACOX2, B_cells, Module5_TcellBcell_score, DRD+, STAT1_sig, LPAR2, KISS1R, ER_PGR_avg, MP_index_adj*(-1), GRP, LYMPHS_PCA_16704732, Luminal_Index, SPNS3, Immune+, STMN1_dat, ZNF442, LINC00467, B3GNT8, and Mod10_ECM (Figure 6A, green part), ranking in descending relevance. The other 25 weak pCR-related features were connected by an orange line (Figure 6A, orange part). To facilitate the clinical application, a nomogram was developed using the top 10 pCR-related features, with an AUC value of 0.803 (Figure S5).
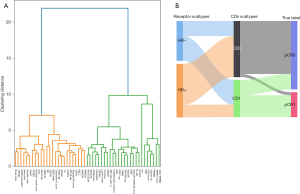
A new typing method for HER2-low BC was proposed by FCNN-based classifier, which was labeled as CD0 and CD1 subtypes. The CD0 and CD1 subtypes exhibited perfect ability in predicting the response of neoadjuvant therapy. Compared with traditional HR classification subtype (64.3% sensitivity and 65.2% specificity in the I-SPY2 trial), CD1 could increase the sensitivity for pCR by 23.5% to 87.8% [95% confidence interval (CI): 78% to 94%] and improved the specificity by 12.2% to 77.4% (95% CI: 69% to 87%) (Table 2 and Figure 6B).
The responses to 8 drugs associated with 25 pCR-related features among different subgroups were analyzed. These features mainly enriched in the following pathways, including ERBB2, DRD, immune, estrogen receptor (ER) and AKT/mammalian-target-of-rapamycin (AKT/mTOR), proliferation, theronine-type endopeptidase activity, and fatty acid beta-oxidation using acyl-CoA oxidase. Although CDn overestimated the pCR response of some drugs (Ctr: paclitaxel, ganitumab), the consistency of pCR responses in features was largely maintained, and both indicated that pembrolizumab (Pembro) and VC (veliparib + carboplatin) achieved the best pCR response among 25 features (top 2 drugs with pCR rates for HER2-low BC in I-SPY2 trial), demonstrating that deep-learning-based pCR prediction was reasonable and effective (Figure 7).
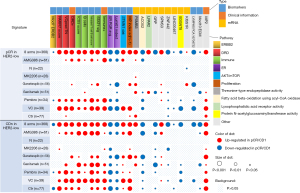
Discussion
The HER2-low subtype, which was proposed in recent years, accounts for a large part of BC. Little has been known about the intrinsic classification of this subtype. We refined the HER2-low subtype through deep learning. First, mRNA, clinical and pathological data were collected from the I-SPY2 trial. Then, 3 single-omic models based on RF, SVM, and FCNN methods were established to compare to prediction value. Third, the FCNN-based method was chosen to build the multi-omics pCR classifier. CD1 and CD0 were the new binary subtypes corresponding to pCR and non-pCR of HER2-low BC. This new typing method could effectively support the pCR prediction. Finally, with integral gradients and unsupervised hierarchical clustering, pCR-related features were identified.
DRD, immune, ERBB2 and fatty acid beta-oxidation using acyl-CoA oxidase were the main pathways enriched by 25 features associated with pCR in HER2-low BC.
DRD, corresponding to PARPi7_score, VCpred_TN and DRD+ in our input variables, was highly correlated with pCR. In I-SPY2, 85% patients with DRD− were non-pCR, but there were no significant pCR differences in DRD+ (55% pCR). However, this result can still support the point that a patient carrying DRD is more likely to achieve pCR (22,23). DRD can be caused by many factors such as the generation of reactive oxidative species during metabolism and exposure to harmful environmental stimuli (24). Homologous recombination defect (HRD), one of functional defects in DNA repair, refers to a situation wherein DNA damage cannot be repaired by the homologous recombination repair pathway (25). The primary role of HRD will shift from tumor-promoting to tumor-suppressing when it reaches a certain level (24). BRCA 1/2 is well-known for homologous recombination in BC. Hence, the HRD presence is often determined in clinic through the BRCA 1/2 mutations test to facilitate blocking the DNA repair pathway of tumor cells with drugs such as PARP inhibitors (26).
In recent years, immune checkpoint inhibitors have become a research hotspot in BC (27,28). In this study, 3 immune-related variables including ICS5_score, B_cells, and Module5_TcellBcell_score ranked in top 10 significant features. B-cells and T-cells were the main immune cells, which could influence the effect of tumor on immunotherapy (29). Pembro, an anti-programmed death 1 (PD-1) monoclonal antibody, was the immune checkpoint inhibitor commonly used in first-line treatment for triple negative breast cancer (TNBC) (30). It could prevent the immune escape of tumor cells and enhance the targeted attack of T-cells by blocking the binding of PD-1 and PD-L1 (31). Although B-cells could directly attack tumor cells, they play an important role in the process of antibody production, antigen presentation, and interaction with immune cells. They are also widely presented in tumor-infiltrating lymphocytes (32). In this study, that the Pembro and VC cohorts achieved satisfactory pCR rate implied that the HR negative HER-2 low subtype was sensitive to immunotherapy.
ERBB2 (also called HER2) is a proto-oncogene closely related to the recurrence and progression of BC (33). In this study, the FCNN classifier still considered ERBB2-low as a significant predictor for pCR. This suggested there might be an intrinsic HER-2 expression cut-off value which would be easily obtains pCR for HER2-low BC. An early study noted that it was hard to maintain consistency between pathologists for the laboratory test results of ERBB2 score using a 4-point scale (34). Therefore, the application of the current ERBB2 assays as the diagnostic test for anti-HER2 new drug (trastuzumab deruxtecan) deserved further study. The high-risk class (MP2) diagnosed by MammaPrint (4) could obtain pCR easier in the VC cohort. The 70-gene assay was developed to determine the risk of distant metastasis in early-stage BC. However, low-risk HER2-low BC classified by MammaPrint did not achieve favorable prognosis in our study. As a result, the value of this gene signature in HER2-low still need further validation.
Fatty acid beta-oxidation pathway corresponded to ACOX2 in the input variable. ACOX2 was down-regulated in the pCR cohort. It was abundantly expressed in the liver and kidneys, which acted as a tumor suppressor in hepatocellular carcinoma (35). However, its role in BC was still unclear.
Although the CDn typing method could effectively distinguish the patients who achieved pCR, there were still some limitations. Firstly, deep learning easily fell into model over-fitting when the sample size was small. In this study, the pCR and non-pCR samples in training set were well-balanced. However, the pCR cases for the testing and validation sets were relatively lacking which would reduce the stability of model. Secondly, the integral gradients method was applied to analyze the significance of predictor, but the interpolation function and integral path might not be the best option. Thirdly, the black box effect of FCNN still deserved attention.
Nevertheless, we raised a new typing method for HER2-low BC classification with excellent prediction value (AUC =0.92). The CDn subtype could help clinicians to predict the response to neoadjuvant therapy for HER2-low patients which would contribute to more precise treatment.
Conclusions
In this study, the FCNN-based pCR prediction model for HER2-low BC was established by integrating genes, clinical data, and biomarkers. A novel typing method (CD1 and CD0) with excellent predictive performance could provide a valuable reference for clinical practice.
Acknowledgments
The authors would like to thank the editors and anonymous reviewers for their insightful comments and suggestions.
Funding: This work was supported by the Wu Jieping Medical Foundation (No. 320.6750.2022-20-3 to Xiaoping Li); the Traditional Chinese Medicine of Guangdong Province (No. 20231383 to Xiaoping Li); the Elite Young Scholars Program of Jiangmen Central Hospital (No. J201905 to Xiaoping Li).
Footnote
Reporting Checklist: The authors have completed the STARD reporting checklist. Available at https://gs.amegroups.com/article/view/10.21037/gs-22-729/rc
Peer Review File: Available at https://gs.amegroups.com/article/view/10.21037/gs-22-729/prf
Conflicts of Interest: All authors have completed the ICMJE uniform disclosure form (available at https://gs.amegroups.com/article/view/10.21037/gs-22-729/coif). Xiaoping Li reports funding support from the Wu Jieping Medical Foundation (No. 320.6750.2022-20-3); the Traditional Chinese Medicine of Guangdong Province (No. 20231383); the Elite Young Scholars Program of Jiangmen Central Hospital (No. J201905). The other authors have no conflicts of interest to declare.
Ethical Statement: The authors are accountable for all aspects of the work in ensuring that questions related to the accuracy or integrity of any part of the work are appropriately investigated and resolved. The study was conducted in accordance with the Declaration of Helsinki (as revised in 2013).
Open Access Statement: This is an Open Access article distributed in accordance with the Creative Commons Attribution-NonCommercial-NoDerivs 4.0 International License (CC BY-NC-ND 4.0), which permits the non-commercial replication and distribution of the article with the strict proviso that no changes or edits are made and the original work is properly cited (including links to both the formal publication through the relevant DOI and the license). See: https://creativecommons.org/licenses/by-nc-nd/4.0/.
References
- Wilkinson L, Gathani T. Understanding breast cancer as a global health concern. Br J Radiol 2022;95:20211033. [Crossref] [PubMed]
- Cao W, Chen HD, Yu YW, et al. Changing profiles of cancer burden worldwide and in China: a secondary analysis of the global cancer statistics 2020. Chin Med J (Engl) 2021;134:783-91. [Crossref] [PubMed]
- Szymiczek A, Lone A, Akbari MR. Molecular intrinsic versus clinical subtyping in breast cancer: A comprehensive review. Clin Genet 2021;99:613-37. [Crossref] [PubMed]
- Zhang H, Katerji H, Turner BM, et al. HER2-low breast cancers: incidence, HER2 staining patterns, clinicopathologic features, MammaPrint and BluePrint genomic profiles. Mod Pathol 2022;35:1075-82. [Crossref] [PubMed]
- Agostinetto E, Rediti M, Fimereli D, et al. HER2-Low Breast Cancer: Molecular Characteristics and Prognosis. Cancers (Basel) 2021;13:2824. [Crossref] [PubMed]
- Modi S, Jacot W, Yamashita T, et al. Trastuzumab Deruxtecan in Previously Treated HER2-Low Advanced Breast Cancer. N Engl J Med 2022;387:9-20. [Crossref] [PubMed]
- Li Y, Zhou Y, Mao F, et al. The Diagnostic Performance of Minimally Invasive Biopsy in Predicting Breast Pathological Complete Response After Neoadjuvant Systemic Therapy in Breast Cancer: A Meta-Analysis. Front Oncol 2020;10:933. [Crossref] [PubMed]
- Hwang HW, Jung H, Hyeon J, et al. A nomogram to predict pathologic complete response (pCR) and the value of tumor-infiltrating lymphocytes (TILs) for prediction of response to neoadjuvant chemotherapy (NAC) in breast cancer patients. Breast Cancer Res Treat 2019;173:255-66. [Crossref] [PubMed]
- Weis JA, Miga MI, Arlinghaus LR, et al. Predicting the Response of Breast Cancer to Neoadjuvant Therapy Using a Mechanically Coupled Reaction-Diffusion Model. Cancer Res 2015;75:4697-707. [Crossref] [PubMed]
- Prat A, Lluch A, Albanell J, et al. Predicting response and survival in chemotherapy-treated triple-negative breast cancer. Br J Cancer 2014;111:1532-41. [Crossref] [PubMed]
- Antunovic L, De Sanctis R, Cozzi L, et al. PET/CT radiomics in breast cancer: promising tool for prediction of pathological response to neoadjuvant chemotherapy. Eur J Nucl Med Mol Imaging 2019;46:1468-77. [Crossref] [PubMed]
- Sundararajan M, Taly A, Yan Q. editors. Axiomatic attribution for deep networks. International conference on machine learning; 2017: PMLR.
- Erion G, Janizek JD, Sturmfels P, et al. Improving performance of deep learning models with axiomatic attribution priors and expected gradients. Nature Machine Intelligence 2021;3:620-31.
- Wolf DM, Yau C, Wulfkuhle J, et al. Redefining breast cancer subtypes to guide treatment prioritization and maximize response: Predictive biomarkers across 10 cancer therapies. Cancer Cell 2022;40:609-23.e6. [Crossref] [PubMed]
- Van der Maaten L, Hinton G. Visualizing data using t-SNE. Journal of Machine Learning Research 2008;9:11.
- Van Der Maaten L. Accelerating t-SNE using tree-based algorithms. Journal of Machine Learning Research 2014;15:3221-45.
- Biau G, Scornet E. A random forest guided tour. Test 2016;25:197-227.
- Huang S, Cai N, Pacheco PP, et al. Applications of Support Vector Machine (SVM) Learning in Cancer Genomics. Cancer Genomics Proteomics 2018;15:41-51. [Crossref] [PubMed]
- Sainath TN, Vinyals O, Senior A, et al. Convolutional, long short-term memory, fully connected deep neural networks. In 2015 IEEE international conference on acoustics, speech and signal processing (ICASSP); IEEE 2015:4580-4.
- Murtagh F, Legendre P. Ward's hierarchical clustering method: clustering criterion and agglomerative algorithm. Journal of Classification 2014;31:274-95.
- The Gene Ontology Resource. 20 years and still GOing strong. Nucleic Acids Res 2019;47:D330-8. [Crossref] [PubMed]
- Wolf DM, Yau C, Wulfkuhle J, et al. Integration of DNA repair deficiency and immune biomarkers to predict which early-stage triple-negative breast cancer patients are likely to respond to platinum-containing regimens vs. immunotherapy: the neoadjuvant I-SPY 2 trial. Cancer Res 2019;79:2679-9.
- Yuan Y, Lee JS, Yost SE, et al. Phase II Trial of Neoadjuvant Carboplatin and Nab-Paclitaxel in Patients with Triple-Negative Breast Cancer. Oncologist 2021;26:e382-93. [Crossref] [PubMed]
- Gilmore E, McCabe N, Kennedy RD, et al. DNA Repair Deficiency in Breast Cancer: Opportunities for Immunotherapy. J Oncol 2019;2019:4325105. [Crossref] [PubMed]
- Stover EH, Fuh K, Konstantinopoulos PA, et al. Clinical assays for assessment of homologous recombination DNA repair deficiency. Gynecol Oncol 2020;159:887-98. [Crossref] [PubMed]
- Ma J, Setton J, Lee NY, et al. The therapeutic significance of mutational signatures from DNA repair deficiency in cancer. Nat Commun 2018;9:3292. [Crossref] [PubMed]
- Tang HW, Hu Y, Chen CL, et al. The TORC1-Regulated CPA Complex Rewires an RNA Processing Network to Drive Autophagy and Metabolic Reprogramming. Cell Metab 2018;27:1040-54.e8. [Crossref] [PubMed]
- Tang HW, Weng JH, Lee WX, et al. mTORC1-chaperonin CCT signaling regulates m(6)A RNA methylation to suppress autophagy. Proc Natl Acad Sci U S A 2021;118:e2021945118. [Crossref] [PubMed]
- Huang RSP, Li X, Haberberger J, et al. Biomarkers in Breast Cancer: An Integrated Analysis of Comprehensive Genomic Profiling and PD-L1 Immunohistochemistry Biomarkers in 312 Patients with Breast Cancer. Oncologist 2020;25:943-53. [Crossref] [PubMed]
- Schmid P, Cortes J, Pusztai L, et al. Pembrolizumab for Early Triple-Negative Breast Cancer. N Engl J Med 2020;382:810-21. [Crossref] [PubMed]
- Gaynor N, Crown J, Collins DM. Immune checkpoint inhibitors: Key trials and an emerging role in breast cancer. Semin Cancer Biol 2022;79:44-57. [Crossref] [PubMed]
- Gatti-Mays ME, Balko JM, Gameiro SR, et al. If we build it they will come: targeting the immune response to breast cancer. NPJ Breast Cancer 2019;5:37. [Crossref] [PubMed]
- De Santis R. Anti-ErbB2 immunotherapeutics: struggling to make better antibodies for cancer therapy. MAbs 2020;12:1725346. [Crossref] [PubMed]
- Fernandez AI, Liu M, Bellizzi A, et al. Examination of Low ERBB2 Protein Expression in Breast Cancer Tissue. JAMA Oncol 2022;8:1-4. [Crossref] [PubMed]
- Zhang Q, Zhang Y, Sun S, et al. ACOX2 is a prognostic marker and impedes the progression of hepatocellular carcinoma via PPARα pathway. Cell Death Dis 2021;12:15. [Crossref] [PubMed]
(English Language Editor: J. Jones)