Identification of biomarkers related to tumorigenesis and prognosis in breast cancer
Introduction
According to new data released by the World Health Organization’s International Agency for Research on Cancer (IARC), breast cancer has replaced lung cancer as the world’s most common cancer (1). Despite the progress made in cancer-related treatment technology during past years, breast cancer has high rates of morbidity and mortality worldwide (2). The latest data released by China’s National Cancer Center show that the incidence of breast cancer in China has exceeded 300,000, with an increase of 3–4% annually. Breast cancer has become one of the major diseases threatening the health of women in China, with more than 10% of women dying of breast cancer. Therefore, the discovery of specific detection markers and therapeutic targets is key to improving the survival rate of breast cancer patients.
In the present study, we analyzed differentially expressed genes (DEGs) in breast cancer and paracancerous to determine the potential mechanism that might induce the development of breast cancer. A search of The Cancer Genome Atlas (TCGA) and Gene Expression Omnibus (GEO) found that cyclin A2 (CCNA2), cyclin dependent kinase 1 (CDK1), centromere protein F (CENPF), kinesin family member 2C (KIF2C), kinesin family member 4A (KIF4A), maternal embryonic leucine zipper kinase (MELK), PDZ binding kinase (PBK), protein regulator of cytokinesis 1 (PRC1), DNA topoisomerase II alpha (TOP2A), and TPX2 microtubule nucleation factor (TPX2) are potential biomarkers of breast cancer related to the prognosis of breast cancer patients. These genes were found to be involved in many biological processes, including the peroxisome proliferator-activated receptors (PPAR) signaling pathway, tyrosine metabolism pathway, and other signaling pathways (3,4). Additionally, their expression levels were found to positively correlate with prognosis, infiltration of immune and molecular subtypes, and tumor infiltrating lymphocytes (TILs), which play a major role in the tumor microenvironment and can directly or indirectly regulate tumor immunity to achieve antitumor effect (5,6). Therefore, our findings indicate that there are immune-related biomarkers that could be used in breast cancer treatment.
Methods
Dataset selection and DEG identification
We downloaded the following two gene expression datasets of breast cancer from the GEO database (www.ncbi.nlm.nih.gov/gds/?term=): GSE109169, and GSE115144. The detailed datasets are shown in Table 1. The standard for DEGs is that the P value is <0.05, and the criteria of the groups were |log2FC (fold change)| ≥1. The gene expression quantification data of breast cancer were downloaded from TCGA (https://www.cancer.gov/about-nci/organization/ccg/research/structural-genomics/tcga). All data were normalized and processed with Sangerbox (http://sangerbox.com/Tool), which is a widely used online platform for TCGA data analysis (7). The parameters set for differential expression analysis were P<0.05 with |log2FC| >1. Subsequently, we combined the DEGs acquired from GEO and TCGA databases to obtain the convergence gene signatures. Volcano maps of DEGs were constructed using the ggplot2 package of R software. Following, the cross DEGs of the 3 datasets were extracted with Venny 2.1 (http://bioinfogp.cnb.csic.es/tools/venny/index.html). The study was conducted in accordance with the Declaration of Helsinki (as revised in 2013).
Table 1
Data source | Platform | Year | Sample size (tumor/normal) | Type |
---|---|---|---|---|
GSE109169 | GPL5175 | 2018 | 25/25 | mRNA |
GSE115144 | GPL17586 | 2018 | 21/21 | mRNA |
Function enrichment analysis of DEGs
To expound the biological significance of the screened DEGs in breast cancer, the Gene Ontology (GO) enrichment and Kyoto Encyclopedia of Genes and Genomes (KEGG) pathways were analyzed using the Database for Annotation, Visualization, and Integrated Discovery 6.8 (https://david.ncifcrf.gov) (8,9). P<0.05 was considered statistically significant. GO enrichment and KEGG pathway results were visualized as a bubble chart using R software.
Protein-protein interaction (PPI) analysis of DEGs
The STRING database version 11.5 (http://string-db.org) was used to construct and analyze the PPI of DEGs (10). An interaction with a combined score >0.4 was considered statistically significant. The results of the analysis were visualized using the Cytoscape version 3.7.2 (11), and Cytohubba from Cytoscape. The top 10 scores of the maximal clique centrality (MCC) algorithm were used as the standard to screen out hub genes with high connectivity in the gene expression network. Simultaneously, Molecular Complex Detection (MCODE) reduces the most significant model in the PPI network. The conditions were as follows: Degree of cutoff =2, node score cutoff =0.2, k-core =2, and maximum depth =100.
The University of ALabama at Birmingham CANcer data analysis portal (UALCAN)
UALCAN (http://ualcan.path.uab.edu) is a widely use online web resource for analyzing publicly available gene expression in tumor and normal tissues (12,13). In the present study, the database was used to perform a thorough analysis of hub gene expression from breast cancer. P<0.05 was considered statistically significant.
Gene Expression Profile Interactive Analysis (GEPIA)
GEPIA (http://gepia2.cancer-pku.cn/#index) is an analysis tool that includes 9736 tumors and 8587 normal tissue samples RNA sequence expression data from TCGA and the GTEx projects (14). In the present study, the gene expression analysis based on data from TCGA and GTEx databases was analyzed using GEPIA software. Analysis of variance (ANOVA) was used to analyze the expression between tumor and normal tissue samples. P<0.05 was considered statistically significant.
The Human Protein Atlas (HPA)
The HPA (www.proteinatlas.org/) is an online software that allows for genome-wide exploration of the impact of individual proteins on clinical outcomes in major human cancers (15). In the present study, we used the HPA to compare the protein expression of hub genes between normal and breast cancer tissues.
Mutation analysis using the cBioPortal database
The cBioPortal database (www.cbioportal.org/) is a comprehensive web resource that analyzes and visualizes multidimensional cancer genomics data (16,17). The database was used to explore hub gene genomic alterations in breast cancer.
Immune infiltration using the Tumor-Immune System Interaction Database (TISIDB)
The TISIDB (http://cis.hku.hk/TISIDB/index.php) is an integrated repository portal for tumor-immune system interactions (18). Interactions between hub gene expression and immune, molecular subtypes, or TILs of breast cancer were investigated using the TISIDB. Correlations between hub genes and TILs were analyzed by Spearman’s test. P<0.05 was considered statistically significant.
Hub genes survival analysis
To further reveal the relationship between hub gene expression and breast cancer prognosis, Kaplan-Meier Plotter (http://kmplot.com/analysis/index.php?p=service) was used for the survival analysis (19). P<0.05 was considered statistically significant.
Statistical analysis
The expression volcano and GO enrichment and KEGG pathways were analyzed and visualized by volcano and bubble chart packages in R software. T-test or ANOVA was used to estimate the significance of differences in expression levels between normal and tumor tissues. P<0.05 was considered statistically significant in both tests.
Results
Identification of DEGs
As shown in Figure 1A-1C showed, based on the screening conditions, 366 overexpressed genes and 469 downexpressed genes were obtained from the GSE109169 database, 152 overexpressed genes and 185 downexpressed genes were obtained from the GSE115144 database, 1,413 overexpressed genes and 2,814 downexpressed genes were obtained from TCGA database. Venny 2.1 was used to select the common DEGs from 3 databases (GSE109169, GSE115144 and TCGA), and visualized by Venn diagrams (Figure 1D). Finally, 89 upregulated and 115 downregulated breast cancer-related DEGs with high reliability were obtained.
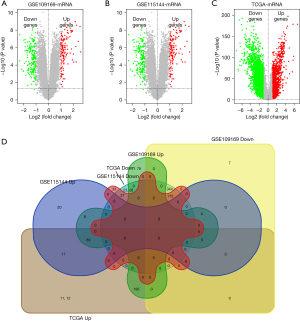
GO enrichment and KEGG signaling pathway analysis of DEGs
GO enrichment analysis showed that the GO annotations of DEGs included cell composition (CC), biological process (BP), and molecular function (MF). P values (P<0.05) were used to arrange the terms. After screening, we identified DEGs enriched in BP, CC, and MF; the top 10 are shown in Figure 2A-2C (for example: mitotic spindle organization, cell division, positive regulation of cell proliferation, extracellular space, extracellular matrix, extracellular region, heparin binding, extracellular matrix structural constituent, and microtubule binding). KEGG analysis showed that the DEGs were mainly concentrated in the PPAR signaling pathway, tyrosine metabolism, cell cycle, and other signaling pathways (Figure 2D).
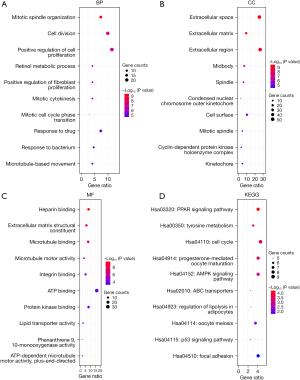
PPI network of DEGs in breast cancer
The STRING database was used to analyze the obtained DEGs and remove the isolated non-interacting genes. The relevant PPI was visualized and included 203 nodes and 367 edges (Figure 3A). Cytoscape was used to analyze the interacting genes for network visualization (Figure 3B-3E). Cytoscape and the plug-in apps Cytohubba and MCODE were used to analyze the network. According to the MCC algorithm, 10 genes (CDK1, TOP2A, KIF4A, CENPF, PRC1, CCNA2, TPX2, PBK, KIF2C, and MELK) with the most stable and highest scores in the network selected as hub genes (Figure 3B). Moreover, the top 3 modules were chosen by the MCODE app (Figure 3C-3E).
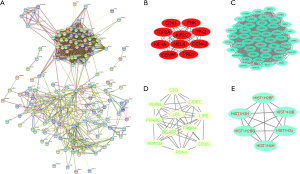
Validation of mRNA and protein expression of the 10 breast cancer hub genes
Based on the DEG analysis, we found that the 10 hub genes were upregulated in breast cancer. To further validate the results, the GEPIA and UALCAN databases were used to verify the findings. As shown in Figures 4,5, the mRNA expression of the 10 central genes (CCNA2, CDK1, CENPF, KIF2C, KIF4A, MELK, PBK, PRC1, TOP2A, and TPX2) were significantly higher in breast cancer tissue than in normal tissue (P<0.001; Figures 4,5). These findings were consistent with the obtained microarray data.
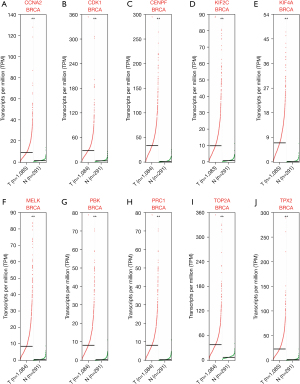
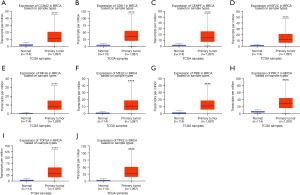
To further examine the protein expression of the 10 hub gene in human tumor tissues, the HPA database was used to perform the experiment. The results revealed that the protein expression of the 10 hub genes (CCNA2, CDK1, CENPF, KIF2C, KIF4A, MELK, PBK, PRC1, TOP2A, and TPX2) was higher in breast cancer tissue compared with normal breast tissue (Figure 6).
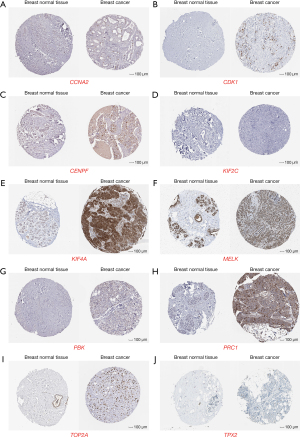
Genetic alteration of 10 hub genes in patients with breast cancer
The cBioPortal website was used to analyze the 10 hub gene genomic alterations in breast cancer. The 10 hub gene alterations varied in type, leading to changes in gene expression (Figure 7A). The findings indicated that 2.2% (CDK1), 5% (PBK), 2% (TPX2), 0.9% (CCNA2), 2.5% (PRC1), 10% (CENPF), 1.8% (KIF4A), 5% (TOP2A), 1.1% (KIF2C), and 1.2% (MELK) of breast cancer samples had genetic alteration (Figure 7B). These findings indicated that the genomic alteration of the 10 hub genes occurs in tumor tissue, and could play a major role in tumor genesis and development.
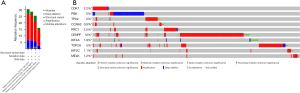
Immune infiltration analysis of the expression of the 10 hub genes
The role of the expression of the 10 hub genes on molecular and immune subtypes in breast cancer was analyzed using TISIDB. C1 (wound healing), C2 (interferon-γ dominant), C3 (inflammatory), C4 (lymphocyte depleted), C5 (immunologically quiet), and C6 (transforming growth factor-β dominant) subtypes constitute the immune subtypes. As shown in Figure 8, the expression of the 10 hub genes was correlated with different immune subtypes of breast cancer, with high expression in the C1 and C2 types, low expression in the C3 types, and no expression in the C5 type.
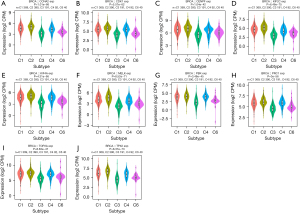
The expression of the 10 hub genes was significantly associated with different molecular subtypes of cancer in breast cancer (Figure 9), and showed low expression in luminal A type. Based on these findings, we found that the expression of the 10 hub genes differed in the immune and molecular subtypes of breast cancer.
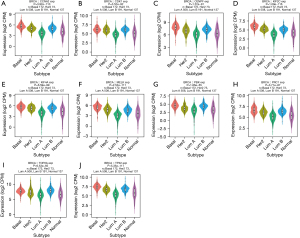
Further, we also found that these 10 hub genes were significantly associated with 28 types of TILs in heterogeneous human cancers (Figure 10). CCNA2 was significantly positively associated with 28 TIL species, such as activated CD4 T cells (Act_CD4 T cells, rho =0.626, P<2.2e−16) and activated CD8 T cells (Act_CD8 T cells, rho =0.209, P<2.88e−12). Similar results were found for CDK1, CENPF, KIF2C, KIF4A, MELK, PBK, PRC1, and TPX2 (Figure 10). The correlation between TOP2A and activated CD8 T cells was not significant (Figure 10I).
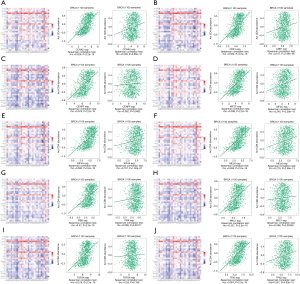
Prognostic analysis of hub genes
Kaplan-Meier Plotter was used to determine the relationship between hub gene expression and the prognosis of breast cancer. The findings indicated that that high expression of hub genes was associated with lower overall survival (P<0.001; Figure 11).
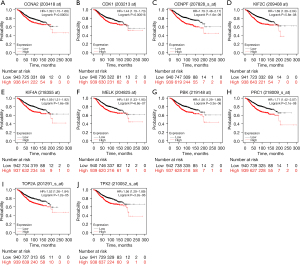
Discussion
Breast cancer is one of the leading causes of mortality among women. Although traditional surgery, radiotherapy, chemotherapy, and targeted immunotherapy prolong the lives of many patients, more than 680,000 women still die of breast cancer every year (1,20). Therefore, more therapeutic targets and prognostic biomarkers are needed.
In our study, 89 upregulated and 115 downregulated breast cancer-related DEGs were found in breast cancer and normal breast tissues. Further, 10 vital regulated genes (CCNA2, CENPF, KIF2C, KIF4A, MELK, PBK, PRC1, TOP2A, TPX2, and CDK1) were screened from the PPI network complex by the Cytohubba plug-in app in Cytoscape. Based on GEPIA, UALCAN, and HPA analyses, we found that the expression level of hub genes was higher in breast cancer samples than normal samples, showing the same trend in expression as predicted by bioinformatics, and verifying the accuracy of our method. The prognosis of the hub genes was found to be associated with significantly worse survival according to the Kaplan-Meier Plotter analysis. In addition, based on the genomic alteration analysis, 10 hub genes were found to occur in tumor tissue. These findings indicated that the hub genes could be potential prognostic biomarkers and/or therapeutic targets for breast cancer.
Functional annotation indicated that these genes were closely related to breast cancer tumorigenesis. The KEGG pathway in hub genes consisted of the PPAR signaling pathway, tyrosine metabolism, cell cycle, and other signaling pathways. CCNA2 and CDK1 play an important role in the cell cycle. CCNA2 regulates the G1-S and G2-M transitions of the cell cycle, is a known prognostic biomarker for survival in breast cancer patients, and is associated with tamoxifen resistance (21,22). Knockdown of CCNA2 can significantly inhibit cell growth by impairing cell cycle progression and inducing apoptosis (23). CDK1 is known as a key point in driving all cell cycle phases in mammals, performing key steps in the process of cell division (24-26). Xia et al. reported that CDK1 silencing significantly impaired tumor growth and promoted tumor cell apoptosis in triple-negative breast cancer (27). Additionally, compared with low CDK1 expression in breast cancer patients, high CDK1 expression was found to be associated with poor overall survival, which is consistent with our findings (28). Studies of other tumors, such as colorectal cancer, lung cancer, and renal cell carcinoma, reported similar results (29-31). CENPF is a component of the nuclear matrix during the G2 phase of interphase, which affects cell division and proliferation (32). Sun et al. reported on the metastatic promoter function of CENPF in BC progression and bone metastasis (33). CENPF has also been reported to be associated with tumor development in cancers, such as papillary thyroid cancer, prostate cancer, and cervical cancer (34-36). KIF2C and KIF4A belong to the kinesin superfamily, which has varied functions in tumor pathobiology (28,37). Previous studies have reported that KIF2C is involved the tumorigenesis of lung cancer, glioma cancer, and breast cancer (38-40). Studies have shown that KIF4A serves as a potential contributor of several malignant tumors, such as breast cancer, lung cancer, hepatocellular carcinoma, cervical cancer, and oral cancer, while in gastric cancer, KIF4A was observed to inhibit tumor cell growth (41-45). MELK expression has been reported to be higher in various cancer cells and tissues than in their normal, non-neoplastic counterparts (46). MELK expression was associated with cell proliferation, immune response, and NAC breast cancer response (47). PRC1 is recognized as an oncoprotein in various cancer types, and PRC1 deficiency leads to cell cycle G2/M arrest and apoptosis, breast cancer was one of the cancer types (39-42). Bu et al. reported that the abnormal expression of PRC1 can induce aberrant cytokine expression, contributing to tumorigenesis and tumor progression (48). Li et al. found that the PRC1 phospho-mimic PRC1T481D mutant could partially rescue the cell proliferation defect induced by CDK16 deletion in TNBC cells (49). Previous bioinformatics analyses have revealed that PRC1 is associated with the immune invasion of hepatocellular carcinoma (50). PBK, a serine/threonine kinase, is tightly controlled in normal tissues, but elevated in many tumors, and plays a role in tumorigenesis and metastasis. PBK knockdown significantly impairs MDA-MB-231 cell proliferation (51). A bioinformatics analysis showed that PBK is correlated with overall survival in breast cancer patients (52). TOP2A is frequently altered in HER2-amplified tumors (53), such as in breast cancer and gastric cancer. TOP2A expression was found to be associated with the prognostic of breast cancer (54). TPX2 is a microtubule-associated protein, is a strong predictor of aggressive behavior, has a reduced response to therapy, and has poor survival in breast cancer (55). These studies demonstrate theses 10 hub genes correlation with breast cancer and are consistent with our results, which predicted that they have the potential to become breast cancer biomarkers.
Because tumor-infiltrating immune cells have a clear relationship with tumor diagnosis and prognosis (56), we explored the correlation between the 3 most useful prognostic indicators and immune infiltration by TISIDB. CDK1, CENPF, KIF2C, KIF4A, MELK, PBK, PRC1, and TPX2 were found to be positively correlated with CD4 T cells. The correlation between TOP2A and activated CD8 T cells was not significant. In summary, CDK1, CENPF, KIF2C, KIF4A, MELK, PBK, PRC1, and TPX2 are considered to have a relationship with the immunoregulation of the tumor environment.
The present study has some limitations. First, the study was based on bioinformatics analysis and lacked experiments (in vivo and in vitro validation). Second, one of the hub genes, TPX2, is upregulated in almost every cancer type, and its value as a prognostic or diagnostic biomarker for breast cancer decreased significantly. Third, the mechanism of the 10 hub genes was not clear. More biological evidence is needed. Therefore, further molecular experiments are needed to determine the function of these central genes and their role in the progression of breast cancer.
Conclusions
The findings on the present study indicated that the 10 potential biomarkers of breast cancer could be involved in breast cancer prognosis. The 10 hub genes were identified as possible indicators for future breast cancer diagnosis and treatment. The identification of the correlation between the prognostic indicators and tumor-infiltrating immune cell levels in breast cancer showed that 9 prognostic indicators play a role in cancer immunoregulation, which could be useful in cancer immunotherapy. Further research is needed to confirm these findings. The findings of our study provide a strong basis for future breast cancer gene targeted therapies, and these 10 hub genes could potentially be new breast cancer target genes.
Acknowledgments
Funding: This project was supported by the Special Project for the Construction of Innovative Environment (Talents, Bases) in the Autonomous Region (Natural Science Foundation Program No. 2022D01C283).
Footnote
Conflicts of Interest: All authors have completed the ICMJE uniform disclosure form (available at https://gs.amegroups.com/article/view/10.21037/gs-22-449/coif). The authors have no conflicts of interest to declare.
Ethical Statement: The authors are accountable for all aspects of the work in ensuring that questions related to the accuracy or integrity of any part of the work are appropriately investigated and resolved. The study was conducted in accordance with the Declaration of Helsinki (as revised in 2013).
Open Access Statement: This is an Open Access article distributed in accordance with the Creative Commons Attribution-NonCommercial-NoDerivs 4.0 International License (CC BY-NC-ND 4.0), which permits the non-commercial replication and distribution of the article with the strict proviso that no changes or edits are made and the original work is properly cited (including links to both the formal publication through the relevant DOI and the license). See: https://creativecommons.org/licenses/by-nc-nd/4.0/.
References
- Sung H, Ferlay J, Siegel RL, et al. Global Cancer Statistics 2020: GLOBOCAN Estimates of Incidence and Mortality Worldwide for 36 Cancers in 185 Countries. CA Cancer J Clin 2021;71:209-49. [Crossref] [PubMed]
- Trayes KP, Cokenakes SEH. Breast Cancer Treatment. Am Fam Physician 2021;104:171-8. [PubMed]
- Zeng W, Yin X, Jiang Y, et al. PPARalpha at the crossroad of metabolic-immune regulation in cancer. FEBS J 2021; Epub ahead of print. [Crossref] [PubMed]
- Sivaganesh V, Sivaganesh V, Scanlon C, et al. Protein Tyrosine Phosphatases: Mechanisms in Cancer. Int J Mol Sci 2021;22:12865. [Crossref] [PubMed]
- Ozga AJ, Chow MT, Luster AD. Chemokines and the immune response to cancer. Immunity 2021;54:859-74. [Crossref] [PubMed]
- Liu H, Yang Z, Lu W, et al. Chemokines and chemokine receptors: A new strategy for breast cancer therapy. Cancer Med 2020;9:3786-99. [Crossref] [PubMed]
- Wang D, Wang Y, Zou X, et al. FOXO1 inhibition prevents renal ischemia-reperfusion injury via cAMP-response element binding protein/PPAR-γ coactivator-1α-mediated mitochondrial biogenesis. Br J Pharmacol 2020;177:432-48. [Crossref] [PubMed]
- Huang da W. Sherman BT, Lempicki RA. Bioinformatics enrichment tools: paths toward the comprehensive functional analysis of large gene lists. Nucleic Acids Res 2009;37:1-13. [Crossref] [PubMed]
- Huang da W. Sherman BT, Lempicki RA. Systematic and integrative analysis of large gene lists using DAVID bioinformatics resources. Nat Protoc 2009;4:44-57. [Crossref] [PubMed]
- Szklarczyk D, Gable AL, Nastou KC, et al. The STRING database in 2021: customizable protein-protein networks, and functional characterization of user-uploaded gene/measurement sets. Nucleic Acids Res 2021;49:D605-12. [Crossref] [PubMed]
- Doncheva NT, Morris JH, Gorodkin J, et al. Cytoscape StringApp: Network Analysis and Visualization of Proteomics Data. J Proteome Res 2019;18:623-32. [Crossref] [PubMed]
- Chandrashekar DS, Bashel B, Balasubramanya SAH, et al. UALCAN: A Portal for Facilitating Tumor Subgroup Gene Expression and Survival Analyses. Neoplasia 2017;19:649-58. [Crossref] [PubMed]
- Chandrashekar DS, Karthikeyan SK, Korla PK, et al. UALCAN: An update to the integrated cancer data analysis platform. Neoplasia 2022;25:18-27. [Crossref] [PubMed]
- Tang Z, Kang B, Li C, et al. GEPIA2: an enhanced web server for large-scale expression profiling and interactive analysis. Nucleic Acids Res 2019;47:W556-60. [Crossref] [PubMed]
- Uhlen M, Zhang C, Lee S, et al. A pathology atlas of the human cancer transcriptome. Science 2017;357:eaan2507. [Crossref] [PubMed]
- Gao J, Aksoy BA, Dogrusoz U, et al. Integrative analysis of complex cancer genomics and clinical profiles using the cBioPortal. Sci Signal 2013;6:pl1. [Crossref] [PubMed]
- Cerami E, Gao J, Dogrusoz U, et al. The cBio cancer genomics portal: an open platform for exploring multidimensional cancer genomics data. Cancer Discov 2012;2:401-4. [Crossref] [PubMed]
- Ru B, Wong CN, Tong Y, et al. TISIDB: an integrated repository portal for tumor-immune system interactions. Bioinformatics 2019;35:4200-2. [Crossref] [PubMed]
- Győrffy B. Survival analysis across the entire transcriptome identifies biomarkers with the highest prognostic power in breast cancer. Comput Struct Biotechnol J 2021;19:4101-9. [Crossref] [PubMed]
- Katsura C, Ogunmwonyi I, Kankam HK, et al. Breast cancer: presentation, investigation and management. Br J Hosp Med (Lond) 2022;83:1-7. [Crossref] [PubMed]
- Gao T, Han Y, Yu L, et al. CCNA2 is a prognostic biomarker for ER+ breast cancer and tamoxifen resistance. PLoS One 2014;9:e91771. [Crossref] [PubMed]
- Markaverich BM, Shoulars K, Rodriguez MA. Luteolin Regulation of Estrogen Signaling and Cell Cycle Pathway Genes in MCF-7 Human Breast Cancer Cells. Int J Biomed Sci 2011;7:101-11. [PubMed]
- Gan Y, Li Y, Li T, et al. CCNA2 acts as a novel biomarker in regulating the growth and apoptosis of colorectal cancer. Cancer Manag Res 2018;10:5113-24. [Crossref] [PubMed]
- Santamaría D, Barrière C, Cerqueira A, et al. Cdk1 is sufficient to drive the mammalian cell cycle. Nature 2007;448:811-5. [Crossref] [PubMed]
- Malumbres M, Barbacid M. Cell cycle, CDKs and cancer: a changing paradigm. Nat Rev Cancer 2009;9:153-66. [Crossref] [PubMed]
- Otto T, Sicinski P. Cell cycle proteins as promising targets in cancer therapy. Nat Rev Cancer 2017;17:93-115. [Crossref] [PubMed]
- Xia Q, Cai Y, Peng R, et al. The CDK1 inhibitor RO3306 improves the response of BRCA-proficient breast cancer cells to PARP inhibition. Int J Oncol 2014;44:735-44. [Crossref] [PubMed]
- Kim SJ, Nakayama S, Miyoshi Y, et al. Determination of the specific activity of CDK1 and CDK2 as a novel prognostic indicator for early breast cancer. Ann Oncol 2008;19:68-72. [Crossref] [PubMed]
- Tong Y, Huang Y, Zhang Y, et al. DPP3/CDK1 contributes to the progression of colorectal cancer through regulating cell proliferation, cell apoptosis, and cell migration. Cell Death Dis 2021;12:529. [Crossref] [PubMed]
- Huang Z, Shen G, Gao J. CDK1 promotes the stemness of lung cancer cells through interacting with Sox2. Clin Transl Oncol 2021;23:1743-51. [Crossref] [PubMed]
- Zhang E, Chen S, Tang H, et al. CDK1/FBXW7 facilitates degradation and ubiquitination of MLST8 to inhibit progression of renal cell carcinoma. Cancer Sci 2022;113:91-108. [Crossref] [PubMed]
- Testa JR, Zhou JY, Bell DW, et al. Chromosomal localization of the genes encoding the kinetochore proteins CENPE and CENPF to human chromosomes 4q24-->q25 and 1q32-->q41, respectively, by fluorescence in situ hybridization. Genomics 1994;23:691-3. [Crossref] [PubMed]
- Sun J, Huang J, Lan J, et al. Overexpression of CENPF correlates with poor prognosis and tumor bone metastasis in breast cancer. Cancer Cell Int 2019;19:264. [Crossref] [PubMed]
- Han Y, Xu S, Cheng K, et al. CENPF promotes papillary thyroid cancer progression by mediating cell proliferation and apoptosis. Exp Ther Med 2021;21:401. [Crossref] [PubMed]
- Shahid M, Kim M, Lee MY, et al. Downregulation of CENPF Remodels Prostate Cancer Cells and Alters Cellular Metabolism. Proteomics 2019;19:e1900038. [Crossref] [PubMed]
- Yu B, Chen L, Zhang W, et al. TOP2A and CENPF are synergistic master regulators activated in cervical cancer. BMC Med Genomics 2020;13:145. [Crossref] [PubMed]
- Miki H, Setou M, Kaneshiro K, et al. All kinesin superfamily protein, KIF, genes in mouse and human. Proc Natl Acad Sci U S A 2001;98:7004-11. [Crossref] [PubMed]
- Bai Y, Xiong L, Zhu M, et al. Co-expression network analysis identified KIF2C in association with progression and prognosis in lung adenocarcinoma. Cancer Biomark 2019;24:371-82. [Crossref] [PubMed]
- Bie L, Zhao G, Wang YP, et al. Kinesin family member 2C (KIF2C/MCAK) is a novel marker for prognosis in human gliomas. Clin Neurol Neurosurg 2012;114:356-60. [Crossref] [PubMed]
- Jiang CF, Xie YX, Qian YC, et al. TBX15/miR-152/KIF2C pathway regulates breast cancer doxorubicin resistance via promoting PKM2 ubiquitination. Cancer Cell Int 2021;21:542. [Crossref] [PubMed]
- Wang H, Lu C, Li Q, et al. The role of Kif4A in doxorubicin-induced apoptosis in breast cancer cells. Mol Cells 2014;37:812-8. [Crossref] [PubMed]
- Zhang L, Huang Q, Lou J, et al. A novel PHD-finger protein 14/KIF4A complex overexpressed in lung cancer is involved in cell mitosis regulation and tumorigenesis. Oncotarget 2017;8:19684-98. [Crossref] [PubMed]
- Hou G, Dong C, Dong Z, et al. Upregulate KIF4A Enhances Proliferation, Invasion of Hepatocellular Carcinoma and Indicates poor prognosis Across Human Cancer Types. Sci Rep 2017;7:4148. [Crossref] [PubMed]
- Narayan G, Bourdon V, Chaganti S, et al. Gene dosage alterations revealed by cDNA microarray analysis in cervical cancer: identification of candidate amplified and overexpressed genes. Genes Chromosomes Cancer 2007;46:373-84. [Crossref] [PubMed]
- Zhang Y, Liu S, Qu D, et al. Kif4A mediate the accumulation and reeducation of THP-1 derived macrophages via regulation of CCL2-CCR2 expression in crosstalking with OSCC. Sci Rep 2017;7:2226. [Crossref] [PubMed]
- McDonald IM, Graves LM. Enigmatic MELK: The controversy surrounding its complex role in cancer. J Biol Chem 2020;295:8195-203. [Crossref] [PubMed]
- Oshi M, Gandhi S, Huyser MR, et al. MELK expression in breast cancer is associated with infiltration of immune cell and pathological compete response (pCR) after neoadjuvant chemotherapy. Am J Cancer Res 2021;11:4421-37. [PubMed]
- Bu H, Li Y, Jin C, et al. Overexpression of PRC1 indicates a poor prognosis in ovarian cancer. Int J Oncol 2020;56:685-96. [Crossref] [PubMed]
- Li X, Li J, Xu L, et al. CDK16 promotes the progression and metastasis of triple-negative breast cancer by phosphorylating PRC1. J Exp Clin Cancer Res 2022;41:149. [PubMed]
- Chen H, Wu J, Lu L, et al. Identification of Hub Genes Associated With Immune Infiltration and Predict Prognosis in Hepatocellular Carcinoma via Bioinformatics Approaches. Front Genet 2020;11:575762. [Crossref] [PubMed]
- Dou X, Wei J, Sun A, et al. PBK/TOPK mediates geranylgeranylation signaling for breast cancer cell proliferation. Cancer Cell Int 2015;15:27. [Crossref] [PubMed]
- He Y, Cao Y, Wang X, et al. Identification of Hub Genes to Regulate Breast Cancer Spinal Metastases by Bioinformatics Analyses. Comput Math Methods Med 2021;2021:5548918. [Crossref] [PubMed]
- Wei L, Wang Y, Zhou D, et al. Bioinformatics analysis on enrichment analysis of potential hub genes in breast cancer. Transl Cancer Res 2021;10:2399-408. [Crossref] [PubMed]
- Xu YC, Zhang FC, Li JJ, et al. RRM1, TUBB3, TOP2A, CYP19A1, CYP2D6: Difference between mRNA and protein expression in predicting prognosis of breast cancer patients. Oncol Rep 2015;34:1883-94. [Crossref] [PubMed]
- Matson DR, Denu RA, Zasadil LM, et al. High nuclear TPX2 expression correlates with TP53 mutation and poor clinical behavior in a large breast cancer cohort, but is not an independent predictor of chromosomal instability. BMC Cancer 2021;21:186. [Crossref] [PubMed]
- Jia D, Li S, Li D, et al. Mining TCGA database for genes of prognostic value in glioblastoma microenvironment. Aging (Albany NY) 2018;10:592-605. [Crossref] [PubMed]
(English Language Editor: R. Scott)