A deep learning-based diagnostic pattern for ultrasound breast imaging: can it reduce unnecessary biopsy?
Introduction
Early diagnosis is key to controlling the second leading of death disease, breast cancer (BC), in women. Early detection of BC could reduce over 40% of mortality (1). The common examination methods include mammography, ultrasound (US), tomography, and biopsy, among others (2). Among them, US is applied world-wide for early BC screening due to advantages such as being radiation-free and cost-effective. The diagnostic performance of US has been continuously improved through substantial technological breakthroughs. However, by nature, it is inevitable that the clinician’s experience, operating skills, and US machine parameters will affect the US results. Thus, diagnostic opinions may vary between doctors, leading to misdiagnosis and missed diagnosis (3). A dilemma in breast US is the prevalence of false-positive findings, resulting in unnecessary biopsies in benign breast lesions (BLs). Berg et al. (4) determined that among 20% BLs that were classified as Breast Imaging Reporting and Data System (BI-RADS) category 3 (5), only around 10% were finally confirmed as malignant by biopsy. Cai et al. (6) also pointed out that 90% of patients undergo unnecessary invasive biopsies due to a biopsy recommendation having been made to avoid misdiagnosis of BC in 10% of patients. Thus, a non-invasive and reliable diagnostic method with a good performance is highly desirable to reduce unnecessary biopsies considering the low prevalence of biologically significant malignancy.
The success of deep learning (DL) signals a new epoch in terms of object detection and classification, imaging analysis, and pattern recognition; an unprecedented enthusiasm for computer-aided diagnosis (CAD) in medical imaging has been witnessed (7,8). Therefore, DL-based CAD could be expected to evolve to a level where certain processes can be automated, such as differentiating malignant and benign cases in US to assist radiologists in improving efficiency and accuracy. In terms of object classification, DL was shown to perform better than traditional machine learning patterns (9). In particular, DL methods using convolutional neural networks (CNNs) have displayed noticeable strengths in medical imaging analysis (10). The research community has applied CNN architectures in liver disease classification (11), brain tumor detection and classification (12), and thyroid cancer classification (13). Early studies conducted the investigation of DL values in evaluating breast cancers (14,15). Nevertheless, the diagnosis of BLs using DL algorithms needs substantial improvement and thus further studies are necessary. Therefore, the aim of this study was to evaluate whether the proposed CNN model can increase the accuracy for classifying BC and minimize unnecessary biopsies. We present the following article in accordance with the STARD reporting checklist (available at https://gs.amegroups.com/article/view/10.21037/gs-22-473/rc).
Methods
Participants and data acquisition
The study was conducted in accordance with the Declaration of Helsinki (as revised in 2013). The study was approved by the ethics committee of Pudong New Area People’s Hospital (No. 2022-131), and individual consent for this retrospective analysis was waived. The images were consecutively collected between 1 August 2020 and 1 December 2021. A total of 557 benign and 506 malignant BLs from Pudong New Area People’s Hospital were screened, and 1,063 patients were enrolled in this work. For patients with multiple BLs, only the most suspicious or the largest diameter BL was selected. The exclusion criteria and enrollment process are shown in Figure 1. The patients were aged from 29 to 78 years. The size of each BL was measured as the largest diameter of each BL. All US images were obtained from Siemens S3000 (Siemens Healthineers, Erlangen, Germany), Philips Epiq 7 (Philips Healthcare, Amsterdam, The Netherlands), Philips IU Elite (Philips Healthcare), GE Voluson E8 (GE Medical Systems, Chicago, IL, USA), and Toshiba Aplio 400 (Toshiba, Tokyo, Japan).
The US image data were reviewed by 2 radiologists with at least 5 years of experience in interpreting breast US images by consensus. The radiologists were blinded to the pathology results. In cases of disagreement, a senior radiologist with more than 20 years of experience in breast US imaging was consulted.
Model construction and evaluation
Pre-processing
By nature, DL requires training data on large scale, but medical data is known to be difficult in acquisition. Thus, we performed data augmentation of different kinds to enrich the training dataset, in an attempt to train the network in a much more cost-effective way with better robustness. We adopted the mirroring method together with the singular value decompositions (SVD) method in 5, 10, and 15 degrees for data augmentation. In the end, the augmentation method resulted in 3,168 (396×2×4) malignant and 3,576 (447×2×8) benign BLs for the training set, together with 110 malignant and 110 benign unaugmented BLs for the external testing set.
Model construction
In this research, the conventional very deep convolutional network (VGGNet) was used to classify US images into benign or malignant. The VGGNet is a CNN classifier that was initially proposed in 2014 (16) for object recognition purposes and has achieved very good results in the ImageNet Large Scale Visual Recognition Challenge (ILSVRC; https://www.image-net.org/challenges/LSVRC/). Our research adopted the 2 most common VGGNet architectures for comparative study, which are VGG-16 and VGG-19, named after their unique 16-layers and 19-layers designs, respectively. In more detail, VGG-16 has 13 convolutional layers, whereas VGG-19 has 16. Both of them have 3 fully connected layers according to the SoftMax function.
As our study involved data on a much smaller scale compared to the data used in the original study (16), transfer learning was used with adaptations in utilizing our experiment under the VGGNet framework. First, in meeting the requirement in the input layer of the VGGNet, we resized our input image into a pixel size of 224×224 with bilinear transformation and duplicated it 3 times in mimicking the 3 channels of the RGB input. Second, instead of having 1,000 nodes in the last layer of the output layer, we updated the number of nodes to 2 in reflecting the benign and malignant classes used in our study. At the last, all the pre-trained parameters in the convolutional layer were preserved and only the parameters in the output layer were finetuned with backpropagation in solving the task defined in our study (identifying benign from malignant). The 2 adopted VGG models were trained using a batch size of 10 in 40 epochs with Adam optimizer, cross-entropy loss function, and a learning rate of 0.01.
Reading sessions
The malignant (suggestion for biopsy) and benign (suggestion for follow-up) BLs were determined by 2 radiologists with a 2-step reading session. The first step was based on conventional US images alone to make a biopsy or follow-up decision. The second step was to take DL results into account for the decision adjustment. We used the receiver operating characteristic (ROC) curve area under the curve (AUC) to determine the optimal cut-off. The Youden index was used to determine the best cut-off value. Therefore, if a BL was firstly assessed as benign and received a DL model result higher than the cut-off value, then the benign BL would be re-classified as malignant (for biopsy). In other words, if a BL was defined as malignant in the first step reading session and received a DL model result lower than the cut-off value, then it would be re-classified as benign (for follow-up) (Figure 2).
Statistical analyses
The statistical analysis was performed using SPSS 25.0 (IBM Corp., Armonk, NY, USA). We adopted the AUC to compare the diagnostic performance among radiologists and DL models, using Delong’s test. The agreement of 2 different DL models was evaluated by using intraclass correlation coefficients (ICCs). We used the pathology report as the gold standard. A 2-sided P value <0.05 was considered indicative of significant differences.
Results
Characteristics of the enrolled data sample
The mean age of malignant patients and benign patients were 57.48±12.31 and 57.83±13.11, respectively (P=0.688). Most benign BLs (70.7%) showed a size between 1.1 and 2.0 cm, whereas the most malignant BLs showed a size between 1.1 and 3.0 cm (1.1–2.0 cm, 43.7%, and 2.1–3.0 cm, 27.8%) (P<0.001). Most malignant cases showed irregular shape (61.4%), absent with halo signs (70.5%), not circumscribed margin (93.4%), and hypoechoic (92.7%) (Table 1).
Table 1
Features | Malignant (n=396) | Benign (n=447) | P value |
---|---|---|---|
Age, years | 57.48±12.31 | 57.83±13.11 | 0.688 |
Size | <0.001 | ||
>3 cm | 62 (15.7) | 16 (3.6) | |
2.1–3 cm | 110 (27.8) | 54 (12.1) | |
1.1–2.0 cm | 173 (43.7) | 316 (70.7) | |
≤1 cm | 51 (12.9) | 61 (13.6) | |
Shape | <0.001 | ||
Oval | 23 (5.8) | 209 (46.8) | |
Round | 21 (5.3) | 31 (6.9) | |
Lobulated | 109 (27.5) | 127 (28.4) | |
Irregular | 243 (61.4) | 80 (17.9) | |
Margin | <0.001 | ||
Circumscribed | 26 (6.6) | 205 (45.9) | |
Not circumscribed | 370 (93.4) | 242 (54.1) | |
Halo sign | <0.001 | ||
Present | 117 (29.5) | 3 (0.7) | |
Absent | 279 (70.5) | 444 (99.3) | |
Echo patterns | <0.001 | ||
Anechoic | 0 (0.0) | 0 (0.0) | |
Hypoechoic | 367 (92.7) | 376 (84.1) | |
Isoechoic | 24 (6.1) | 67 (15.0) | |
Hyperechoic | 5 (1.3) | 4 (0.9) |
Data are shown as mean ± standard deviation or number (%).
Comparison of the diagnostic performance and the unnecessary biopsy between the CNN model and radiologists
The VGG-19 achieved a better diagnostic performance in the training dataset in terms of AUC [0.939, 95% confidence interval (CI): 0.924–0.954], compared to VGG-16 (0.919, 95% CI: 0.901–0.938) (ICC =0.559) (Figure 3).
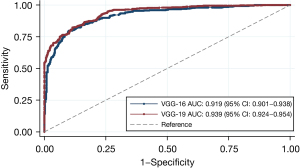
Among the external dataset, VGG-19 also yielded the best diagnostic performance in terms of AUC (0.959, 95% CI: 0.937–0.982), followed by VGG-16 (0.903, 95% CI: 0.864–0.942), and radiologist (0.805, 95% CI: 0.744–0.865) (Figure 4). Based on the results of DL models, we further adjusted radiologists’ interpretation of malignant and benign BLs. Both adjustments showed higher specificity (Radiologist + VGG-16: 92.73%, and Radiologist + VGG-19: 99.09%) and equivalent (Radiologist + VGG-16: 72.73%) or higher sensitivity (Radiologist + VGG-19: 83.64%) (Table 2). Figure 5 summarizes 6 cases of this study.
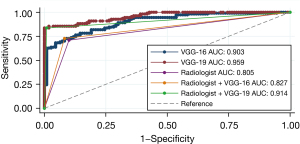
Table 2
Diagnostic model | AUC (95% CI) | Sensitivity, % | Specificity, % | Accuracy, % |
---|---|---|---|---|
VGG-16 | 0.903 (0.864–0.942) | 72.73 | 91.82 | 82.27 |
VGG-19 | 0.959 (0.937–0.982) | 85.45 | 98.18 | 91.82 |
Radiologist | 0.805 (0.744–0.865) | 70.91 | 90.00 | 80.45 |
Radiologist + VGG-16 | 0.827 (0.771–0.875) | 72.73 | 92.73 | 82.73 |
Radiologist + VGG-19 | 0.914 (0.871–0.957) | 83.64 | 99.09 | 81.36 |
AUC, area under the curve.
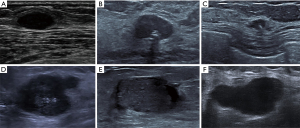
The Youden index of VGG-16 model was 0.6455, which meant when a benign BL received a VGG-16 result higher than 0.6238, then it would be re-evaluated as malignant and recommended for biopsy. The Youden index of VGG-19 model was 0.8364, which meant when a benign BL received a VGG-19 result higher than 0.6669, then it would be re-evaluated as malignant and recommended for biopsy. Thus, with the aid of VGG-19 result, the unnecessary biopsy [false positive/(true negative + false positive)] was drastically decreased from 10.0% to 0.9%, whereas with the assist of VGG-16 results, the unnecessary biopsy rate was decreased to 8.2% (Table 3).
Table 3
Amendment scheme | Benign (n=110) | Malignant (n=110) | |||
---|---|---|---|---|---|
True negative | False positive | True positive | False negative | ||
Before correction | 99 | 11 | 78 | 32 | |
Corrected based on VGG-16 results | 101 | 9 | 80 | 30 | |
Corrected based on VGG-19 results | 109 | 1 | 92 | 18 |
Discussion
Our study revealed that the DL models yielded a satisfactory performance in classifying BC, with an AUC of 0.919 (VGG-16 model) and 0.939 (VGG-19 model) for the validation dataset and an AUC of 0.903 (VGG-16 model) 0.959 (VGG-19 model), a sensitivity of 72.73% (VGG-16 model) and 85.45% (VGG-19 model), and a specificity of 91.82% (VGG-16 model) and 98.18% (VGG-19 model) for the independent test dataset. In addition, our results show that DL models, especially, VGG-19 model, can significantly outperform than the radiologists in terms of diagnosing BC.
Compared to previous research that adopted CAD patterns to differentiate malignant and benign BLs (17), our DL models were designed to assist radiologist to determine which BL warrants biopsy. Wang et al. (17) used a commercially available software (S-Detect; Samsung Medison, Seoul, South Korea) to evaluate the added value of an artificial intelligence system adjunct to breast US regarding reducing unnecessary biopsies. The DL models presented superior performances in terms of specificity, offering a substantial decrease trend of unnecessary biopsy. The reduction of unnecessary biopsy would help to free the financial burden of the healthcare system to some extent as well as relief the financial cost and anxiety borne by patients. Their research results gave a positive answer. Wang et al. (17) introduced a 2-way stratification for downgrading BI-RADS category 4a (biopsy) BLs to category 3 (follow-up). The biopsy rate for BI-RADS 4a BLs decreased from 100% to 67.4% (stratification A) and 37.2% (stratification B), with 4.7% of malignancies missed. However, their limitation was obvious, including a small study sample (78 malignant BLs and 95 benign BLs). In addition, with the assistance of S-Detect, there was no improvement in terms of sensitivity. Zhao et al. (18) also investigated the unnecessary biopsy among 82 malignant and 113 benign BLs using S-Detect. The biopsy rate was decreased from 100% to 25%, 16.2%, 26.9%, 17.9%, and 23.6%, respectively, with a low misdiagnosis rate (0%, 9.7%, 7.9%, 6.3%, and 4.8%, respectively). Although they compared the sensitivity and specificity among the CAD system and radiologists, their study sample was too small to provide a solid conclusion. Both studies adopted BI-RADS categories when assessing unnecessary biopsies as BI-RADS 4a BLs are recommended by BI-RADS guidelines to undertake biopsy (19). It was stated that BI-RADS 4a BLs present a malignant rate between 3% and 10% with a positive predictive value of 6% (20).
In terms of the comparison of diagnostic performance between radiologist (AUC: 0.805) and DL models, our results showed better diagnostic performance, with AUCs of 0.903 (VGG-16) and 0.959 (VGG-16). Our results were similar to those of the previous publications. Fujioka et al. (21) retrospectively analyzed 96 benign BLs and 144 malignant BLs for training and 48 benign BLs and 72 malignant BLs for testing to use DL with CNN to classify malignant and benign BLs based on ultrasound images. The CNN model achieved the best AUC (0.913), followed by 3 radiologists (0.728, 0.841, and 0.845, respectively). However, they did not further evaluate the added value of CNN model to radiologists’ interpretation. Romeo et al. (22) assessed the machine learning’s value in classifying non-cystic benign and malignant BLs. They enrolled 135 BLs for training and 66 for external testing. Their results showed an improved accuracy (82%) and sensitivity (93%) of the machine learning model, compared to that of the radiologists (accuracy: 79.4%, sensitivity: 77.8%), but a lower specificity (57%, radiologist: 81%). There was an elevation of radiologist performance when adopting the machine learning technique (80.2%), but with no statistical significance (P=0.508). The machine learning model was based on feature extraction, including, among others, two-dimensional (2D) shape, gray level co-occurrence matrix (GLCM), and gray level size zone matrix. Machine learning models, such as ANN, are data-driven. In other words, the quality of input data can affect the generated results to some extent. However, CNNs are powerful tools that can identify and extract their own radiomic features from input images. Thus, CNNs can link these features to the outcome for better results. That maybe one of the reasons why Romeo et al.’s (22) results were different from ours. When our radiologist adjusted their interpretation based on CNN results, both CNN models helped improving sensitivity, specificity, and accuracy rate. The initial development of a VGG network was to offer deeper networks with smaller filters for a better understanding of more complicated image features. Consequently, a growing number of medical data analysis adopted VGG networks as they can provide “blackbox” features.
Our study had some limitations. Benign BLs that have not received US-guided fine needle aspiration biopsy (FNAB) were excluded. In our practice, such BLs are commonly recommended for US follow-up. Thus, sampling bias was inevitable. Furthermore, the malignant rate (46.9%) was high in our training session. The malignant rate and benign rate were balanced in our external testing. These results conflicted with the real-world data, and would also bring result bias. Thirdly, we adopted a manual cropping of regions of interest (ROIs) that may also have affected the results since it is operator dependent. We will try automatic segmentation in our future research to overcome this issue.
The application of DL patterns in breast US may improve the diagnostic performance of radiologists by offering a second opinion. Adding a DL-based model, especially VGG-19, could reduce unnecessary breast lesion biopsies and minimize the radiologists’ workload.
Acknowledgments
Funding: This study was funded by Outstanding Young Medical Talents Program of Pudong Health Bureau of Shanghai (No. PWRq2020-40) and Shanghai Municipal Health Commission (No. 202040113).
Footnote
Reporting Checklist: The authors have completed the STARD reporting checklist. Available at https://gs.amegroups.com/article/view/10.21037/gs-22-473/rc
Data Sharing Statement: Available at https://gs.amegroups.com/article/view/10.21037/gs-22-473/dss
Conflicts of Interest: All authors have completed the ICMJE uniform disclosure form (available at https://gs.amegroups.com/article/view/10.21037/gs-22-473/coif). The authors have no conflicts of interest to declare.
Ethical Statement: The authors are accountable for all aspects of the work in ensuring that questions related to the accuracy or integrity of any part of the work are appropriately investigated and resolved. The study was conducted in accordance with the Declaration of Helsinki (as revised in 2013). The study was approved by the ethics committee of Pudong New Area People’s Hospital (No. 2022-131), and individual consent for this retrospective analysis was waived.
Open Access Statement: This is an Open Access article distributed in accordance with the Creative Commons Attribution-NonCommercial-NoDerivs 4.0 International License (CC BY-NC-ND 4.0), which permits the non-commercial replication and distribution of the article with the strict proviso that no changes or edits are made and the original work is properly cited (including links to both the formal publication through the relevant DOI and the license). See: https://creativecommons.org/licenses/by-nc-nd/4.0/.
References
- Cheng HD, Shan J, Ju W, et al. Automated breast cancer detection and classification using ultrasound images: A survey. Pattern Recognition 2010;43:299-317. [Crossref]
- Wang L. Early Diagnosis of Breast Cancer. Sensors (Basel) 2017;17:1572. [Crossref] [PubMed]
- Calas MJ, Almeida RM, Gutfilen B, et al. Intraobserver interpretation of breast ultrasonography following the BI-RADS classification. Eur J Radiol 2010;74:525-8. [Crossref] [PubMed]
- Berg WA, Athina V. Screening Breast Ultrasound Using Handheld or Automated Technique in Women with Dense Breasts. Journal of Breast Imaging 2019;1:283-96. [Crossref]
- Tessler FN, Middleton WD, Grant EG, et al. ACR Thyroid Imaging, Reporting and Data System (TI-RADS): White Paper of the ACR TI-RADS Committee. J Am Coll Radiol 2017;14:587-95. [Crossref] [PubMed]
- Cai Y, Zhu C, Chen Q, et al. Application of a second opinion ultrasound in Breast Imaging Reporting and Data System 4A cases: can immediate biopsy be avoided? J Int Med Res 2021;49:3000605211024452. [Crossref] [PubMed]
- Oberije C, Nalbantov G, Dekker A, et al. A prospective study comparing the predictions of doctors versus models for treatment outcome of lung cancer patients: a step toward individualized care and shared decision making. Radiother Oncol 2014;112:37-43. [Crossref] [PubMed]
- van Griethuysen JJM, Fedorov A, Parmar C, et al. Computational Radiomics System to Decode the Radiographic Phenotype. Cancer Res 2017;77:e104-7. [Crossref] [PubMed]
- Szegedy C, Liu W, Jia Y, et al. Going deeper with convolutions. 2015 IEEE Conference on Computer Vision and Pattern Recognition (CVPR), 2015:1-9.
- Fourcade A, Khonsari RH. Deep learning in medical image analysis: A third eye for doctors. J Stomatol Oral Maxillofac Surg 2019;120:279-88. [Crossref] [PubMed]
- Che H, Brown LG, Foran DJ, et al. Liver disease classification from ultrasound using multi-scale CNN. Int J Comput Assist Radiol Surg 2021;16:1537-48. [Crossref] [PubMed]
- El Kader IA, Xu G, Shuai Z, et al. Brain Tumor Detection and Classification by Hybrid CNN-DWA Model Using MR Images. Curr Med Imaging 2021;17:1248-55. [Crossref] [PubMed]
- Qin P, Wu K, Hu Y, et al. Diagnosis of Benign and Malignant Thyroid Nodules Using Combined Conventional Ultrasound and Ultrasound Elasticity Imaging. IEEE J Biomed Health Inform 2020;24:1028-36. [Crossref] [PubMed]
- Kim J, Kim HJ, Kim C, et al. Weakly-supervised deep learning for ultrasound diagnosis of breast cancer. Sci Rep 2021;11:24382. [Crossref] [PubMed]
- Kim S, Choi Y, Kim E, et al. Deep learning-based computer-aided diagnosis in screening breast ultrasound to reduce false-positive diagnoses. Sci Rep 2021;11:395. [Crossref] [PubMed]
- Karen Simonyan and Andrew Zisserman. Very deep convolutional networks for large-scale image recognition. arXiv1409 2014:1556.
- Wang XY, Cui LG, Feng J, et al. Artificial intelligence for breast ultrasound: An adjunct tool to reduce excessive lesion biopsy. Eur J Radiol 2021;138:109624. [Crossref] [PubMed]
- Zhao C, Xiao M, Liu H, et al. Reducing the number of unnecessary biopsies of US-BI-RADS 4a lesions through a deep learning method for residents-in-training: a cross-sectional study. BMJ Open 2020;10:e035757. [Crossref] [PubMed]
- Spak DA, Plaxco JS, Santiago L, et al. BI-RADS((R)) fifth edition: A summary of changes. Diagn Interv Imaging 2017;98:179-90.
- Lazarus E, Mainiero MB, Schepps B, et al. BI-RADS lexicon for US and mammography: interobserver variability and positive predictive value. Radiology 2006;239:385-91. [Crossref] [PubMed]
- Fujioka T, Kubota K, Mori M, et al. Distinction between benign and malignant breast masses at breast ultrasound using deep learning method with convolutional neural network. Jpn J Radiol 2019;37:466-72. [Crossref] [PubMed]
- Romeo V, Cuocolo R, Apolito R, et al. Clinical value of radiomics and machine learning in breast ultrasound: a multicenter study for differential diagnosis of benign and malignant lesions. Eur Radiol 2021;31:9511-9. [Crossref] [PubMed]
(English Language Editor: J. Jones)