Comprehensive analysis reveals potential hub genes and therapeutic drugs in an acquired lymphedema model
Introduction
In 2020, female breast neoplasms exceeded lung cancer as the primary cancer type worldwide in terms of incidence (1). Axillary lymph node dissection (ALND) is performed when lymph node metastasis of breast cancer is confirmed. Due to devastation of the axillary lymphatic structure, upper limb lymphedema affects approximately 30–50% of ALND patients (2). Acquired lymphedema is a common and often severe complication of breast cancer surgery and radiology that seriously affects patients’ quality of life. In addition, many patients experience a series of physical and psychological symptoms (3-5).
There are many risk factors for acquired lymphedema, including overweight or obesity, older age, axillary radiotherapy, infection or trauma of the ipsilateral upper extremity, a broad scope of axillary surgery, and adjuvant chemotherapy. However, the conventional view of lymphatic obstruction does not adequately explain the generation of lymphedema. Presently, there are 3 main hypotheses regarding lymphedema pathogenesis (6): the lymphatic failure hypothesis, the hemodynamic hypothesis, and the interstitial hypothesis. Most researchers believe that acquired lymphedema is caused by the obstruction or interruption of lymphatic reflux of the upper limb due to axillary surgery and radiotherapy (7). A large amount of protein-rich lymph liquid is retained in the tissue space to form high protein edema. Nevertheless, the pathogenesis for lymphedema is complex and remains unclear.
Currently, acquired lymphedema diagnoses commonly rely on history, physical findings, and even imaging studies (8). Lymphedema therapy mainly involves complete decongestive physiotherapy (multilayer low-stretch bandage application, manual lymph drainage, skin care, exercises), surgical treatment, and other approaches. However, early diagnosis of lymphedema can be difficult, and lymphedema treatment efficacy is not ideal. The main reason for these challenges is a lack of clarity regarding the potential molecular mechanism of acquired lymphedema. In addition, research focusing on the genetics of acquired lymphedema pathogenesis has been relatively scarce.
To further study the genetic pathogenesis of acquired lymphoedema, we performed comprehensive bioinformatics analyses to determine the hub genes and signaling pathways in samples from a model imitating human acquired lymphedema. The treatment of lymphedema is mainly non-surgical method. Physical therapy is the primary and effective treatment. It is difficult and unethical to obtain human tissue samples from acquired lymphedema patients. So mouse lymphedema models were applied for analysis. Initially, the GSE4333 gene expression datasets (9) were downloaded from the National Center for Biotechnology Information (NCBI) databases (10). These datasets included data for female hairless immunocompetent (the capacity for a normal immune response) SKH-1 mice, which represent a model of human acquired lymphedema. Subsequently, we identified dysregulated genes between different groups. In addition, we used several methods to perform Gene Ontology (GO), signaling pathway enrichment annotation, and protein-protein interaction (PPI) analyses (11,12). Ultimately, the potential hub genes, related pathways, and existing candidate drugs were identified. The findings might provide insights into the molecular mechanisms underlying acquired lymphedema and provide theoretical support for clinical treatment. We present the following article in accordance with the STREGA reporting checklist (available at https://gs.amegroups.com/article/view/10.21037/gs-22-453/rc).
Methods
Microarray data information
We downloaded the GSE4333 expression profile (.txt format files) and correlated information built in the GPL3506 platform (.soft format file) from the NCBI-Gene Expression Omnibus (GEO) website (10,13). The GSE4333 datasets contain 3 arrays of tail skin from normal mice (no intervention) and 3 arrays of tail skin from lymphedema mice (with surgical lymphatic vessel blockage). We analyzed 6 samples from the GSE4333 datasets to identify differentially expressed genes (DEGs) between the normal and lymphedema groups.
Data preprocessing
First, the messenger RNA (mRNA) probes were retained, and the other non-mRNA probes were discarded. According to the information of the GPL3506 platform, we replaced the probe identification numbers with the official gene symbols. Finally, we used Python to explore the gene expression matrix and obtain DEGs between the normal group and the lymphedema group (14). The fold change (FC) cut-off was ≥1.4, and the adjusted P value cut-off was <0.05.
GO and pathway enrichment analysis
The GO analysis method is a common and helpful method for interpreting gene products and functional characteristics (11). The GO analysis terms were classified into biological process (BP), cellular component (CC), and molecular function (MF) categories. The Kyoto Encyclopedia of Genes and Genomes (KEGG) database is used to interpret biological functions and features of organic systems (12). We used the Database for Annotation, Visualization and Integrated Discovery (DAVID) to determine the GO and KEGG enrichment of the DEGs (15). The DEGs were used as search input for GO and KEGG pathway analyses using the official gene symbol and species information (Mus musculus).
PPI analysis and module determination
The online database Search Tool for the Retrieval of Interacting Genes (STRING; version 11.5) is a well-known tool for prediction of correlations between distinct proteins encoded by related genes (16). First, the DEGs were input into the STRING website. Then, a.tsv format file containing the PPI information was obtained. We used Cytoscape software to build PPI networks (17). Next, we used the Molecular Complex Detection (MCODE) tool to categorize the important gene modules. These modules had markedly interconnected groups in the PPI networks (18,19). We used the default parameters for all analyses. Finally, we used the above gene modules to carry out drug-gene interaction analysis.
Determination of the hub genes
We used the cytoHubba plugin of Cytoscape software to identify hub genes. This tool uses an algorithm to predict the critical nodes and subnetworks in an internal network. We used the maximal clique centrality (MCC) method to identify key genes.
Drug-gene interaction and functional analyses of potential hub genes
The Drug-Gene Interaction Database (DGIdb; https://www.dgidb.org) is an online resource for filtering drug-gene interactions. We used the module genes as search terms and determined the extant drugs targeting these genes.
Statistical analysis
We used the moderate t-test to identify DEGs. The GO and KEGG annotation enrichment results were analyzed by Fisher’s exact test. The analysis process diagram (Figure 1) shows the workflow of the study.
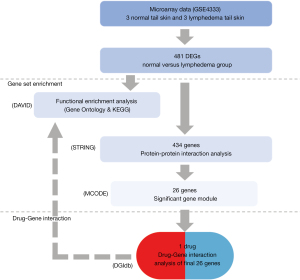
Results
Identification of DEGs
According to the above criteria, a total of 481 DEGs between the normal and lymphedema groups were identified, including 307 upregulated genes and 174 downregulated genes (Table 1). A volcano map and heatmap depicting these DEGs are shown in Figure 2A,2B.
Table 1
Regulation direction | Gene name |
---|---|
Upregulated | Fcer1g, Dynlt1c/Dynlt1f, Tecpr1, B2m, Tlr2, Ifitm1, Cald1, Prkcz, Rptn, Nt5e, Rarres2, S100a8, Wdr18, Socs3, Csrp2, Syngr1, Ctsz, S100a9, Mfap4, Alox5ap, Cyba, Irf7, Tmem176b, Lcn2, Svep1, Srgn, Lyz2, Cdc25a, Trim30d, Erdr1, Calm1, Rac2, Ctsc, Trdn, C1qc, Txndc5, 2610528A11Rik, Ppp1r14b, Ctla2a, Ptx3, Marcks, Tmsb10, Stfa1, Gm10252/Ftl1, Sprr2a3/Sprr2a1, Ms4a6d, Stfa3, C1qa, Traf4, Capg, Sssca1, C3, Col5a2, Serpinb3a, Syngr3, Renbp, Cxcl12, Ccl6, Atxn7l1, Akap2, Cyth4, Ccl7, St13, Ifit1, Zpr1, Lox, Gnl2, 1700029K24Rik/Eno3, Slc28a2, Sec24d, Usp33, Chil4, Sepp1, Coro1a, Hdc, Lst1, Fam49b, Tpi1, Aif1, Lyn, Bgn, Sebox, Tnc, Pf4, C1qtnf3, Ctsb, Gngt2, Cbr2, Pgk2, Rnase1, Lgals1, Mfap2, H2-D1, Esd, Ndn, Tpm2, Cybb, Unc93b1, Dnaja1, Nelfe/Cfb, Fhl2, St6galnac4, Sdhd, Hexa, Cd53, Col5a1, Fth1, Col8a1, Ifitm2, Arhgdib, Ccr4, Lcp1, Plac8, Cd52, Col4a2, Plek, Dnajc13, Lgmn, Aqp1, 4930406D14Rik, Ehhadh, Ms4a6b, Selp, Col4a1, Cd44, Tmem176a, Tfpi, Ifitm3, LOC547349/5430410E06Rik/H2-L/H2-K1/H2-D1, Gpihbp1, Entpd1, Bicc1/4930533K18Rik, Ncf4, Ccl3, Tpm1, Cthrc1, Mt1, Rgs2, Ifngr1, Flot1, Arpc3, Tnfaip6, Fabp5, F2r, Gpm6b, Itm2a, Eln, Txlna, Slc35b2, Reg3g, Myeov2, Csta1, Anxa6, Col12a1, Slc38a1, Parp9, Mpp1, Oxct1, Impa1, Wdr83os, Ctla2b, Inpp5d, Ubl3, Psmb10, Zfp57, Cyc1, Vim, Gm10840/Limd2, Tmsb4x, Efemp2, Smpdl3a, Ftl1, Mob3a, Dnajb5, Ube2s, Cotl1, Szrd1, Cfh, Hspbap1, Ptma, Pnp, Fbln1, Prcp, Serping1, Anxa3, Snx32, Sat1, Mmp9, Sepw1, Fetub, Dynlt1f/Dynlt1b, Hsd17b4, Cystm1, Stim1, Igtp, Ifnar2, Ly86, Hsp90b1, Galnt10, Col27a1, Spink4, Gusb/Aebp1, Cmtm3, Clec4n, Ccnb1, Loxl1, Wnt7b, Cfp, Ly6c1, Bcl2a1a, Laptm5, Flrt2, H2-Q5, Samhd1, ar2, Psmb8, Thbs1, Krtcap2, Myh6, Slpi, 5730458M16Rik/Cd302, Gimap4, Lsp1, Itga11, Keg1, Snx5, Tmem55a, 1700071M16Rik/Pla2g7, Runx1t1, Tspan32, H2-DMa, Il10rb, Alx3, Ncf2, Msn, Ufsp2, H2-Aa, Ndufa3, Rpl7l1, Fn1, Cxcl16, Pigq, Irf1, LOC102641613/H2-K1, H2-Eb1, Nrp1, Prelp, Stab1, Ttr, Stc2, Ccr2, Gata1, Tmed9, Slc25a45, Usp36, Rps29, Lama4, Irf8, Ccl9, Sod3, AV051173, Marcksl1, Gm2518/Taf6l, Gcnt3, Psmb9, Spp1, Trdmt1, Cpe, Rsph9, Prmt1, Pgk1, Serpina1c/Serpina1b/Serpina1a, Klf13, Pkig, Mgp, Pdia4, Ube2b, C4a/C4b, Pglyrp1, Cox7b, Serf2, Cd14, Fabp2, Ddit3, Rnaset2a, Ttc17, Hnrnpa1, Cd74, Clec4b1, Mrps6/Slc5a3, 2900052L18Rik, Abca1, Sprr2j-ps, Ccdc53, Inpp5k, Rrp7a, Clps, Fcgbp, Nme1, Egfl6, Palld, Actb, Urah, Ubl5/Fbxl12, C1s1, Atp5b, H19 |
Downregulated | Dennd1b, Calb1, 1700025G04Rik, Mxd4, Selenbp1, Krtap13, Ocln, Mrps31, Trim35, Micall1, Ctnnbip1, Gzmb, Pcp2, Tssk3, Nle1, C78278, A330105O20Rik/Golga4, Slc16a11, Skap2, Stard8, Acoxl, Prr22, Relt, C2cd2, Cdkn2b, Nanog, Slx1b, Aff4, Aldh3a1, Tmem236, 1700007H22Rik, 1700022H16Rik, Acox3, Ppm1d, Cnn2, Pou3f2, Atp2a2, Mia3, Oog3, Apoe, Lyzl1, 2310034C09Rik, Tmem119, Rpl7, Aatk, Cilp2, 4930542C16Rik, Khdc1b, Ciart, Alox12e, Pck2, C77068, Igbp1b, Incenp, 1110064A23Rik, Kpna1, 4930550L05Rik/Fbxw2, Epcam, Gchfr, Slc22a4, Slc7a3, Arrdc2, Cox6b1, Zfp821/Ccdc7b, Wnt7a, Zmynd19, Gk5, Txndc2, Picalm, Omt2b, Mmaa, Gm8783, Lta, Fmo3, Ankrd33b, D10Wsu159e, Scn4b, Mitf, Chsy1, Sec14l4, Bbip1, Aig1, Dgat2, Nkx2-4, Phyhipl, Ufd, Ccnf, Kap, Efhb, Sdhaf3, Nop58, Il12a, Col15a1, Slurp2, Hnrnpu, 1700022F17Rik, Bglap, Il7r, Casp9, Golph3, Kifap3, Wdr1, 2310065H11Rik/2310057J18Rik, Gm10589/Pik3c2a, Tor4a, C77872, Pin1rt1, Kcnk7, Efnb1, Nf1, Smpd1, Smg5, 1700026H06Rik/Map7, Caml, Angel1, Slc12a4, Elovl6, Eif3f, Paxip1, Ostf1, Dag1, Igfbp5, Mycl, Ggps1, Tm9sf3, Ttll10, Wfdc21, Unc13c, Pygb, D1Ertd799e, Il9r, Nlrp4b, Slc2a13, Ppp5c, Slc3a2, Pds5b, 1700016D08Rik/Cluap1, Klhl32, Selplg, Wdr47, Rfpl4, Kcnd2, Cntn3, Fbxo36, Tmem225, Fam3c, Gm21685/Entpd4, Wnt1, Hbb-bs/Hbb-b2, Ppp1r12b, Tbx3, Ptger4, Rab11a, 1700125H03Rik, Tas1r3, Zxdc, Rgs14, D9Ertd167e, Cited4, Svil, Fxyd4, Nes, Pim3, Cypt12, Apoa1, Elmo2, Tmem159/4930560O18Rik, D6Ertd87e, Ahi1, 1810030O07Rik, LOC105242488/Tjap1, 2310061I04Rik, Ccdc80, Gm19935, Scn1, Col1a1, Trpv4, P4ha1, Col5a1top2A, Ccnb2, Nusap1, Iqgap3, Uhrf1, Aspm, Tubb3, Prc1, Tyms, Kiaa0101 |
DEGs, differentially expressed genes.
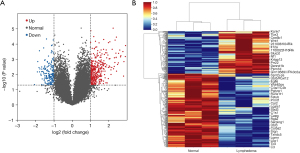
GO and pathway enrichment analyses
We used DAVID to depict the enriched GO terms and signaling pathways of the DEGs. Figure 3 shows the top enrichment terms for the DEGs in the BP, CC, and MF categories. The BP terms were mainly related to immune system processes, the inflammatory response, and the innate immune response. In the CC category, the DEGs were primarily related to the extracellular exosome, extracellular region, and extracellular space components. In the MF category, the DEGs were significantly related to protein binding, extracellular matrix (ECM) structural constituent, and identical protein binding terms. According to the results of pathway analysis, the genes were highly involved in ECM-receptor interactions, Staphylococcus aureus infection, leishmaniasis, pertussis, and tuberculosis (Figure 4).
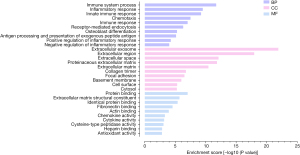
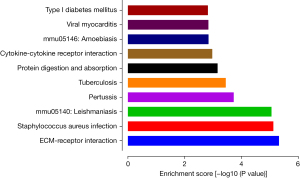
PPI and module analysis
A total of 434 genes/nodes with 1,715 edges were included in the PPI networks. In contrast, 47 genes did not participate in the PPI networks (Figure 5). Based on the MCODE analysis, 13 modules were identified in the network. The top 5 significant modules are shown in Table 2.
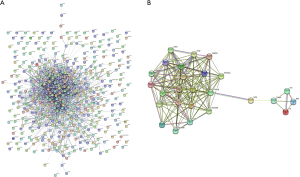
Table 2
Module | Nodes | Edges | Score | Genes |
---|---|---|---|---|
Module 1 | 26 | 334 | 13.360 | Aif1, Alox5ap, Arhgdib, Bgn, C1qc, Cd52, Cd53, Cd74, Coro1a, Cyba, Eln, Fcer1g, Inpp5d, Irf8, Laptm5, Lcp1, Lox, Ly86, Ms4a6b, Ms4a6d, Ncf2, Ncf4, Plek, Rac2, Selp, Thbs1 |
Module 2 | 7 | 36 | 6.000 | Nle1, Gnl2, Nop58, Rpl7, Prmt1, Wdr18, Rpl7l1 |
Module 3 | 16 | 86 | 5.733 | Actb, C1qa, Ccl3, Ccr2, Cd14, Col12a1, Col15a1, Col27a1, Col8a1, Il7r, Lcn2, Ly6c1, Lyn, Mmp9, S100a9, Vim |
Module 4 | 16 | 80 | 5.333 | Selplg, Nanog, Nes, Clec4n, Epcam, Ccl6, Lyz2, Apoe, Ccl7, Tlr2, S100a8, Cybb, Cfp, Fn1, Bglap, Nt5e |
Module 5 | 14 | 52 | 4.000 | Irf7, Sdhd, Psmb8, Atp5b, Ifitm2, Cyc1, Hsp90b1, Irf1, Cox6b1, Psmb9, Cox7b, Il10rb, Ifnar2, Ifitm1 |
Hub gene identification
Based on the MCC method, the connectivity degree values in the PPI network were used to determine the top 10 genes, which included Fc epsilon receptor Ig (Fcer1g), CD53 molecule (Cd53), neutrophil cytosolic factor 4 (Ncf4), Rac family small GTPase 2 (Rac2), lymphocyte antigen 86 (Ly86), lysosomal protein transmembrane 5 (Laptm5), interferon regulatory factor 8 (Irf8), neutrophil cytosolic factor 2 (Ncf2), allograft inflammatory factor 1 (Aif1), and coronin 1A (Coro1a).
Drug-gene interaction analysis
We selected the top module, module 1 (26 genes), for the analysis of drug-gene interactions (Figure 5). The ALOX5AP gene, which is targeted by one existing candidate drug (fiboflapon), was found in module 1.
Discussion
Acquired lymphedema is a prevalent complication among patients who undergo ALND and axillary radiotherapy (20). Acquired lymphedema can be divided into acute acquired lymphedema and delayed acquired lymphedema. Acute acquired lymphedema, which affects numerous patients, often occurs within a few months after surgery, while delayed acquired lymphedema does not occur until a few years later. Patients’ quality of life can be negatively affected by acquired lymphedema because of chronic infections, swelling, secondary malignancies, and unsightly upper limbs. In most cases, acquired lymphedema can be treated with conservative methods, such as combined decongestive therapy, compression therapy, and patient education programs. Recently, the key conservative method of treating acquired lymphedema is complete decongestive therapy (CDT), which involves physical therapy, manual lymphatic drainage, and skin care. Lymphatic fluid accumulation in the tissues was reduced by this method (21). But it can only relieve acquired lymphedema. However, there is still no cure for lymphedema. Prevention including prophylactic use of compression sleeves and upper limb strength excise remains the key measure. Screening high-risk patients is critical for early intervention. Therefore, the current study attempted to reveal the hub genes, important pathways, and potentially effective drugs for acquired lymphedema.
The efficacy of therapeutic strategies for acquired lymphedema is still not ideal, primarily because the pathogenesis is not clear. Recent studies have found that the critical mechanisms involved in acquired lymphedema development might include inflammation, fibrosis, and adipocytokine signaling (22-24). However, few studies have explored the genetic mechanism of and potential targeted drugs for acquired lymphedema.
In this paper, the GSE4333 datasets containing microarray data for female hairless immunocompetent SKH-1 mice were utilized for gene expression analysis. We found a total of 481 DEGs, including 307 upregulated genes and 174 downregulated genes. Select bioinformatics methods, namely, GO term enrichment analysis, signaling pathway enrichment analysis, PPI network construction, hub gene selection, and drug-gene interaction analysis, were used to further characterize these DEGs.
We found interactions among proteins related to the DEGs and potential hub genes involved in the molecular mechanism through PPI network analysis. Then, we identified 10 genes with comparatively high connectivity levels (≥50): Aif1 (degree =90), Fcer1g (degree =88), Rac2 (degree =86), Irf8 (degree =80), Ly86 (degree =72), Ncf4 (degree =70), Ncf2 (degree =64), Laptm5 (degree =56), Cd53 (degree =52), and Coro1a (degree =50). Among them, Cd53, Ncf4, Rac2, Ncf2, and Coro1a were enriched in immune system processes. In addition, LY86, Laptm5, and Irf8 were related to inflammatory responses. The gene Cd53 belongs to the tetraspanin family, which is involved in the regulation of immune cell function (25,26). In our study, immune system processes were the most significantly enriched functional term. This may suggest that Cd53 acts on acquired lymphedema through an immune system process. Meanwhile, Ncf4, Rac2, Ncf2, and Coro1a participate in the same mechanism in the immune system (27-29). The gene Ly86 is also known as MD-1, and some studies have shown that MD-1 is related to inflammatory responses (30); Laptm5 has a positive feedback role in the inflammatory signaling pathway (31); and Irf8 adjusts the transcription of NLR family apoptosis inhibitory proteins related to inflammasome activation (32). In GO enrichment analysis, the annotated BP terms were associated with the inflammatory response. Therefore, the inflammatory response plays an active role in acquired lymphedema. The roles of Fcer1g and Aif1 in the mechanism of acquired lymphedema are still unclear, and further studies are needed.
In the BP annotation category, the DEGs were mostly related to the immune system and the inflammatory response. García Nores et al. suggested that in the lymphedematous region, the constituents of the skin innate immune system change during different phases. One study has shown that T-regulatory cells mediate local immunosuppression in lymphedema (33). Patients with chronic lymphedema can easily develop persistent infections and multiple neoplasms in the lymphedematous limb.
In the CC annotation category, the DEGs were markedly associated with extracellular exosomes and focal adhesion. Regarding extracellular exosomes, research has suggested that cancer exosomes trigger fibroblast-to-myofibroblast differentiation, which has major implications for the mechanisms underlying fibrotic diseases (34). Regarding focal adhesion, mutations in some transcription factors are predominantly associated with lymphedema and increased focal adhesions in human lymphatic endothelial cells (35).
In the MF category, the DEGs were significantly associated with fibronectin binding, protein binding, and ECM structural constituents. The ECM is a noncellular three-dimensional macromolecular network composed of collagens, fibronectin, laminins, several other glycoproteins, and other components. Herrera et al. (36) found that the ECM is a trigger of gradual fibrosis. Recently, additional studies have shown that chronic inflammation-induced fibrosis is important in the pathophysiology of acquired lymphedema. One basic study has shown that fibrosis gradually worsens and is irreversible in mouse tail models of acquired lymphedema (37). Rockson (38) found that inhibition of fibrosis can combat lymphoedema. With regard to protein binding, Leung (39) found that the accumulation and binding of protein molecules might damage the flow of lymph fluid and induce lymphedema and tissue oedemaedema. Regarding fibronectin binding, fibronectin is the basic component of the ECM. Yim et al. (40) found that in Korean breast cancer survivors, serum fibronectin 1 levels are associated with a high risk of lymphoedema.
According to KEGG analysis, the DEGs were highly related to the processes of ECM-receptor interaction and Staphylococcus aureus infection. Evidence has revealed that ECM receptors control the transcription of collagen by translocating to the nucleus (41,42). Some specific areas of ECM receptors contribute to tissue fibrosis by adjusting the production of ECM. Furthermore, lymphoedema associated with fluid accumulation in tissue is an important pathological feature of bacterial infections. However, how bacterial infections can be both the result and cause of lymphatic vessel dysfunction is still unclear. In recent studies, significant inhibition of both lymphatic vessel contraction and lymph velocity was observed in mice with active Staphylococcus aureus infection. When Staphylococcus aureus was cleared and inflammation was addressed, chronic inhibition of lymphatic function remained (43,44). These findings prompted us to focus on the potential mechanisms of fibrosis and bacterial inflammation.
The drug-gene interaction analysis of module 1 revealed that ALOX5AP is targeted by one existing candidate drug, fiboflapon. Fiboflapon is a 5-lipoxygenase-activating protein inhibitor and an effective oral anti-inflammatory drug (45). When the lymphatic system is injured, changes in normal tissue function occur due to inflammation. Subsequently, this can result in the lymphedema phenotype. Jiang et al. (46) investigated the interplay between inflammation and pathological tissue remodelling through inflammatory factors. In addition, Rockson (47) found that the inflammatory response is related to some gene expression pathways associated with acquired lymphedema. Hence, suppression of inflammatory reactions may delay the development of lymphedema. The findings of these studies suggest that anti-inflammatory drugs might be beneficial treatment agents for acquired lymphedema.
To date, the genes related to acquired lymphedema and drugs that can be applied to treat this condition have not been revealed. In a mouse model of acquired lymphedema, we identified 10 hub genes and one existing drug through comprehensive analyses. Among them, Cd53, Ncf4, Rac2, Ncf2, and Coro1a were enriched in immune system processes. Kataru et al. (48) found lymphatic injury could regulate immune response, which could promote tissue fibrosis. Furthermore, LY86, Laptm5, and Irf8 were related to inflammatory responses. A study showed that fluid cumulation by lymphatic injury might act as an initial signaling key to inflammation that eventually brings about the pathologic changes of lymphedema (49). So, immune system processes and immune response play important roles in acquired lymphedema. However, the functions of Fcer1g and Aif1 in the process of acquired lymphedema are still unclear. Besides, the fiboflapon-related drug maybe effective in treating acquired lymphedema. Future studies are needed to verify.
The study still had some limitations. We did not use human samples to verify the results due to the difficulty associated with acquiring humanized lymphedema samples. This kind of dataset did not exist in the GEO database. After searching in PubMed, we concluded that this is the first study to reveal the potential mechanism and therapeutic target of lymphedema using bioinformatic methods. Consequently, our findings provide insights to aid in the discovery of the molecular mechanism of acquired lymphedema.
Conclusions
Using bioinformatics methods, we identified the top 10 hub genes (Fcer1g, Cd53, Ncf4, Rac2, Ly86, Laptm5, Irf8, Ncf2, Aif1, and Coro1a), molecular mechanisms, and potential therapeutic drugs (fiboflapon) for acquired lymphedema.
Acknowledgments
Funding: This work was supported by the Elite Young Scholars Program of Jiangmen Central Hospital (No. J201905 to Xiaoping Li). The funders had no role in the study design, data collection and analysis, decision to publish, or preparation of the manuscript.
Footnote
Reporting Checklist: The authors have completed the STREGA reporting checklist. Available at https://gs.amegroups.com/article/view/10.21037/gs-22-453/rc
Conflicts of Interest: All authors have completed the ICMJE uniform disclosure form (available at https://gs.amegroups.com/article/view/10.21037/gs-22-453/coif). XL reports that this work was supported by the Elite Young Scholars Program of Jiangmen Central Hospital (No. J201905 to XL). The other authors have no conflicts of interest to declare.
Ethical Statement: The authors are accountable for all aspects of the work in ensuring that questions related to the accuracy or integrity of any part of the work are appropriately investigated and resolved.
Open Access Statement: This is an Open Access article distributed in accordance with the Creative Commons Attribution-NonCommercial-NoDerivs 4.0 International License (CC BY-NC-ND 4.0), which permits the non-commercial replication and distribution of the article with the strict proviso that no changes or edits are made and the original work is properly cited (including links to both the formal publication through the relevant DOI and the license). See: https://creativecommons.org/licenses/by-nc-nd/4.0/.
References
- Sung H, Ferlay J, Siegel RL, et al. Global Cancer Statistics 2020: GLOBOCAN Estimates of Incidence and Mortality Worldwide for 36 Cancers in 185 Countries. CA Cancer J Clin 2021;71:209-49. [Crossref] [PubMed]
- Petrek JA, Senie RT, Peters M, et al. Lymphedema in a cohort of breast carcinoma survivors 20 years after diagnosis. Cancer 2001;92:1368-77. [Crossref] [PubMed]
- Lee SH, Min YS, Park HY, et al. Health-related quality of life in breast cancer patients with lymphedema who survived more than one year after surgery. J Breast Cancer 2012;15:449-53. [Crossref] [PubMed]
- Park JE, Jang HJ, Seo KS. Quality of life, upper extremity function and the effect of lymphedema treatment in breast cancer related lymphedema patients. Ann Rehabil Med 2012;36:240-7. [Crossref] [PubMed]
- Pusic AL, Cemal Y, Albornoz C, et al. Quality of life among breast cancer patients with lymphedema: a systematic review of patient-reported outcome instruments and outcomes. J Cancer Surviv 2013;7:83-92. [Crossref] [PubMed]
- Grada AA, Phillips TJ. Lymphedema: Pathophysiology and clinical manifestations. J Am Acad Dermatol 2017;77:1009-20. [Crossref] [PubMed]
- Vignes S. Lymphedema: From diagnosis to treatment. Rev Med Interne 2017;38:97-105. [Crossref] [PubMed]
- Grada AA, Phillips TJ. Lymphedema: Diagnostic workup and management. J Am Acad Dermatol 2017;77:995-1006. [Crossref] [PubMed]
- Tabibiazar R, Cheung L, Han J, et al. Inflammatory manifestations of experimental lymphatic insufficiency. PLoS Med 2006;3:e254. [Crossref] [PubMed]
- Barrett T, Troup DB, Wilhite SE, et al. NCBI GEO: archive for high-throughput functional genomic data. Nucleic Acids Res 2009;37:D885-90. [Crossref] [PubMed]
- Ashburner M, Ball CA, Blake JA, et al. Gene ontology: tool for the unification of biology. The Gene Ontology Consortium. Nat Genet 2000;25:25-9. [Crossref] [PubMed]
- Kanehisa M, Goto S. KEGG: kyoto encyclopedia of genes and genomes. Nucleic Acids Res 2000;28:27-30. [Crossref] [PubMed]
- Edgar R, Domrachev M, Lash AE. Gene Expression Omnibus: NCBI gene expression and hybridization array data repository. Nucleic Acids Res 2002;30:207-10. [Crossref] [PubMed]
- Virtanen P, Gommers R, Oliphant TE, et al. SciPy 1.0: fundamental algorithms for scientific computing in Python. Nat Methods 2020;17:261-72. [Crossref] [PubMed]
- Huang DW, Sherman BT, Tan Q, et al. DAVID Bioinformatics Resources: expanded annotation database and novel algorithms to better extract biology from large gene lists. Nucleic Acids Res 2007;35:W169-75. [Crossref] [PubMed]
- Szklarczyk D, Morris JH, Cook H, et al. The STRING database in 2017: quality-controlled protein-protein association networks, made broadly accessible. Nucleic Acids Res 2017;45:D362-8. [Crossref] [PubMed]
- Kohl M, Wiese S, Warscheid B. Cytoscape: software for visualization and analysis of biological networks. Methods Mol Biol 2011;696:291-303. [Crossref] [PubMed]
- Bader GD, Hogue CW. An automated method for finding molecular complexes in large protein interaction networks. BMC Bioinformatics 2003;4:2. [Crossref] [PubMed]
- Doncheva NT, Morris JH, Gorodkin J, et al. Cytoscape StringApp: Network Analysis and Visualization of Proteomics Data. J Proteome Res 2019;18:623-32. [Crossref] [PubMed]
- Liu YF, Liu JE, Mak YW, et al. Prevalence and predictors of breast cancer-related arm lymphedema over a 10-year period in postoperative breast cancer patients: A cross-sectional study. Eur J Oncol Nurs 2021;51:101909. [Crossref] [PubMed]
- Huang TW, Tseng SH, Lin CC, et al. Effects of manual lymphatic drainage on breast cancer-related lymphedema: a systematic review and meta-analysis of randomized controlled trials. World J Surg Oncol 2013;11:15. [Crossref] [PubMed]
- Shimizu Y, Shibata R, Ishii M, et al. Adiponectin-mediated modulation of lymphatic vessel formation and lymphedema. J Am Heart Assoc 2013;2:e000438. [Crossref] [PubMed]
- Cuadrado GA, de Andrade MFC, Ariga SK, et al. Inflammation Precedes Fat Deposition in an Experimental Model of Lymphedema. Lymphat Res Biol 2021;19:116-25. [Crossref] [PubMed]
- Kataru RP, Wiser I, Baik JE, et al. Fibrosis and secondary lymphedema: chicken or egg? Transl Res 2019;209:68-76. [Crossref] [PubMed]
- Demaria MC, Yeung L, Peeters R, et al. Tetraspanin CD53 Promotes Lymphocyte Recirculation by Stabilizing L-Selectin Surface Expression. iScience 2020;23:101104. [Crossref] [PubMed]
- Dunlock VE. Tetraspanin CD53: an overlooked regulator of immune cell function. Med Microbiol Immunol 2020;209:545-52. [Crossref] [PubMed]
- Chou J, Hsu JT, Bainter W, et al. A novel mutation in NCF2 associated with autoimmune disease and a solitary late-onset infection. Clin Immunol 2015;161:128-30. [Crossref] [PubMed]
- Pick R, Begandt D, Stocker TJ, et al. Coronin 1A, a novel player in integrin biology, controls neutrophil trafficking in innate immunity. Blood 2017;130:847-58. [Crossref] [PubMed]
- Lougaris V, Baronio M, Gazzurelli L, et al. RAC2 and primary human immune deficiencies. J Leukoc Biol 2020;108:687-96. [Crossref] [PubMed]
- Thomas Jennings R, Odkhuu E, Nakashima A, et al. Inflammatory responses increase secretion of MD-1 protein. Int Immunol 2016;28:503-12. [Crossref] [PubMed]
- Glowacka WK, Alberts P, Ouchida R, et al. LAPTM5 protein is a positive regulator of proinflammatory signaling pathways in macrophages. J Biol Chem 2012;287:27691-702. [Crossref] [PubMed]
- Karki R, Lee E, Place D, et al. IRF8 Regulates Transcription of Naips for NLRC4 Inflammasome Activation. Cell 2018;173:920-933.e13. [Crossref] [PubMed]
- García Nores GD, Ly CL, Savetsky IL, et al. Regulatory T Cells Mediate Local Immunosuppression in Lymphedema. J Invest Dermatol 2018;138:325-35. [Crossref] [PubMed]
- Webber J, Steadman R, Mason MD, et al. Cancer exosomes trigger fibroblast to myofibroblast differentiation. Cancer Res 2010;70:9621-30. [Crossref] [PubMed]
- Norden PR, Sabine A, Wang Y, et al. Shear stimulation of FOXC1 and FOXC2 differentially regulates cytoskeletal activity during lymphatic valve maturation. Elife 2020;9:53814. [Crossref] [PubMed]
- Herrera J, Henke CA, Bitterman PB. Extracellular matrix as a driver of progressive fibrosis. J Clin Invest 2018;128:45-53. [Crossref] [PubMed]
- Zhou C, Su W, Han H, et al. Mouse tail models of secondary lymphedema: fibrosis gradually worsens and is irreversible. Int J Clin Exp Pathol 2020;13:54-64. [PubMed]
- Rockson SG. Inhibition of Fibrosis to Combat Lymphedema. Lymphat Res Biol 2020;18:399. [Crossref] [PubMed]
- Leung K. IRDye800CW-Cyclic albumin-binding domain (Ac-RLIEDICLPRWGCLWEDDK-NH2). Molecular Imaging and Contrast Agent Database (MICAD). Bethesda (MD); 2004.
- Yim SY, Ryu E, Lim JY, et al. Serum fibronectin 1 and ApoE levels increase with risk of lymphedema in Korean breast cancer survivors. Support Care Cancer 2015;23:2319-26. [Crossref] [PubMed]
- Chiusa M, Hu W, Liao HJ, et al. The Extracellular Matrix Receptor Discoidin Domain Receptor 1 Regulates Collagen Transcription by Translocating to the Nucleus. J Am Soc Nephrol 2019;30:1605-24. [Crossref] [PubMed]
- Tang HW, Hu Y, Chen CL, et al. The TORC1-Regulated CPA Complex Rewires an RNA Processing Network to Drive Autophagy and Metabolic Reprogramming. Cell Metab 2018;27:1040-1054.e8. [Crossref] [PubMed]
- Jones D, Meijer EFJ, Blatter C, et al. Methicillin-resistant Staphylococcus aureus causes sustained collecting lymphatic vessel dysfunction. Sci Transl Med 2018;10:eaam7964. [Crossref] [PubMed]
- Tang HW, Weng JH, Lee WX, et al. mTORC1-chaperonin CCT signaling regulates m6A RNA methylation to suppress autophagy. Proc Natl Acad Sci U S A 2021;118:e2021945118. [PubMed]
- Rask-Andersen M, Masuram S, Schiöth HB. The druggable genome: Evaluation of drug targets in clinical trials suggests major shifts in molecular class and indication. Annu Rev Pharmacol Toxicol 2014;54:9-26. [Crossref] [PubMed]
- Jiang X, Nicolls MR, Tian W, et al. Lymphatic Dysfunction, Leukotrienes, and Lymphedema. Annu Rev Physiol 2018;80:49-70. [Crossref] [PubMed]
- Rockson SG. The lymphatics and the inflammatory response: lessons learned from human lymphedema. Lymphat Res Biol 2013;11:117-20. [Crossref] [PubMed]
- Kataru RP, Baik JE, Park HJ, et al. Regulation of Immune Function by the Lymphatic System in Lymphedema. Front Immunol 2019;10:470. [Crossref] [PubMed]
- Ly CL, Kataru RP, Mehrara BJ. Inflammatory Manifestations of Lymphedema. Int J Mol Sci 2017;18:171. [Crossref] [PubMed]
(English Language Editor: J. Jones)