A nomogram for survival prediction in 275,812 U.S. patients with breast cancer: a population-based cohort study based on the SEER database
Introduction
Breast cancer is one of the most lethal malignant tumors and has become the second leading cause of female death (1). It is estimated that about 287,850 females have suffered from breast cancer and approximately 43,250 patients have died from the cancer in 2022 so far (1). With advancements in surgery, chemotherapy, radiation, and hormone therapy, the survival of breast cancer has been improved in the past few decades (2-4). The improvement of multimodal therapies is significant for the survival of cancer patients, with a 5-year relative survival over 90% in developed countries (5). However, these women still have a poor prognosis worldwide.
Nomograms, a statistic-based tool, can assess the risk of clinicopathological features by quantifying the biological and clinical variables of patients with cancer (6). To date, nomograms have been widely applied in the personalized prediction of cancer, such lung, cervical, prostate, and hepatocellular carcinomas (7-11). To the best of our knowledge, several studies have reported risk factors associated with breast cancer (12-17). However, the nomogram based on significant factors that influence the survival of breast cancer in a large population has been rarely explored. Huang et al. (18) reported a nomogram to assess the overall mortality risk in young breast cancer patients. Johnson et al. (19) mentioned that the incidence of breast cancer in adolescents and young adults is relatively low. It is of clinical significance to establish a model to predict the mortality risk of all breast cancer patients.
Herein, we attempted to investigate the predictive factors for the death of breast cancer patients, and develop and validate a nomogram based on the Surveillance, Epidemiology, and End Results (SEER) database, with the aim of reliably predicting the 3- and 5-year survival of breast cancer patients. In addition, we further explored the predictive performance of our nomogram in different ethnicities. We present the following article in accordance with the TRIPOD reporting checklist (available at https://gs.amegroups.com/article/view/10.21037/gs-22-321/rc).
Methods
Study design and population
In this retrospective cohort study, data on patients with breast cancer were extracted from the SEER database, a public registry from the National Cancer Institute (NCI), which covers approximately 27.8% of the U.S. cancer population (20). A total of 275,812 breast cancer patients aged 18 years and over were included. These patients were diagnosed as breast cancer via histopathological examination between 1975 and 2015. The study was carried out in accordance with the Declaration of Helsinki (as revised in 2013).
Study variables
Basic information on patients was collected, including the age at diagnosis (20–39, 40–59, 60–79, or ≥80 years), year of diagnosis, race (American Indian, Asian, Black, Hispanic, and White), marital status (single, married, divorced/separated, or widowed), vital status (alive or dead), American Joint Committee on Cancer (AJCC) T stage (Tis & T1, T2, T3, or T4), N stage (N0, N1, N2, or N3), grade (I, II, III, or IV), type of reporting source item (hospital inpatients or others), regional nodes positive (no or yes), tumor size (<4 or ≥4 cm), laterality (right or left), first malignant primary indicator (no or yes), and number of malignant tumors (1, 2, or ≥3). The outcomes of our study were the 3- and 5-year survival rates of breast cancer patients. The follow-up duration was 5 years. The follow-up was terminated when the death occurred during the follow-up period.
Establishment and validation of the nomogram
All eligible patients were randomly classified as two groups in a random manner, namely the training set (n=193,069) and validation set (n=82,743). Univariate and multivariate Cox regression analyses were used to assess the predictors of cancer mortality using the training set. The regression model was recalculated using significant variables from the multivariate Cox regression analysis. The nomogram was drawn to predict the 3- or 5-year survival of patients.
Harrell’s concordance index (C-index) was utilized to assess the performance of the nomogram (no predictive power: C=0.50, low predictive power: 0.51–0.70, moderate predictive power: 0.71–0.90, and high predictive power: >0.90) (21). The discriminative ability of the nomogram was evaluated using the receiver operating characteristic (ROC) and calibration curves.
Statistical analysis
Normal distributed measurement data were compared through one-way analysis of variance and shown as the mean ± standard deviation (), and those with skewed distribution were compared via the rank-sum test and presented as the median and quartile [M (Q1, Q3)]. The χ2 test or Fisher’s exact test was utilized for comparisons between enumeration data that were expressed as number and proportion [n (%)]. R software (version 4.0.2, The R Foundation for Statistical Computing, Vienna, Austria) and SAS software (version 9.4, SAS Institute Inc., USA) were used for statistical analysis. Two-sided P<0.05 was deemed statistically significant.
Results
The characteristics of patients with breast cancer
Totally 275,812 women with breast cancer who were registered in the SEER database were enrolled in this study. Among them, 193,069 cases were in the training set, with a mean survival time of 42.22±21.36 months. The ages at diagnosis were 20–39 (n=9,329, 4.83%), 40–59 (n=79,750, 41.31%), 60–79 (n=89,615, 46.42%), and ≥80 (n=14,375, 7.45%) years. In terms of marital status, 58.87% (n=113,668) of cases were married, 15.42% (n=29,768) were single, 13.13% (n=25,347) were widowed, and 12.58% (n=24,286) were divorced or separated.
The comparison of the baseline characteristics of women with breast cancer between the training and validation sets is shown in Table 1. There were no differences between the two sets regarding age at diagnosis (20–39, 40–59, 60–79, or ≥80 years), year of diagnosis (2010, 2011, 2012, 2013, 2014, or 2015), race (American Indian, Asian, Black, Hispanic, and White), marital status (single, married, divorced/separated, or widowed), AJCC T stage (Tis & T1, T2, T3, or T4), N stage (N0, N1, N2, or N3), grade (I, II, III, or IV), type of reporting source item (hospital inpatients or others), regional nodes positive (no or yes), tumor size (<4 or ≥4 cm), laterality (right or left), first malignant primary indicator (no or yes), number of malignant tumors (1, 2, or ≥3), and vital status (alive or dead), all with P>0.05.
Table 1
Variables | Total (n=275,812) | Groups | Statistics | P | |
---|---|---|---|---|---|
Training set (n=193,069) | Validation set (n=82,743) | ||||
Age at diagnosis (years), n (%) | Z=1.070 | 0.285 | |||
20–39 | 13,381 (4.85) | 9,329 (4.83) | 4,052 (4.90) | ||
40–59 | 113,673 (41.21) | 79,750 (41.31) | 33,923 (41.00) | ||
60–79 | 128,145 (46.46) | 89,615 (46.42) | 38,530 (46.57) | ||
≥80 | 20,613 (7.47) | 14,375 (7.45) | 6,238 (7.54) | ||
Year of diagnosis, n (%) | Z=−1.684 | 0.092 | |||
2010 | 42,881 (15.55) | 29,905 (15.49) | 12,976 (15.68) | ||
2011 | 44,062 (15.98) | 30,759 (15.93) | 13,303 (16.08) | ||
2012 | 45,105 (16.35) | 31,568 (16.35) | 13,537 (16.36) | ||
2013 | 46,833 (16.98) | 32,832 (17.01) | 14,001 (16.92) | ||
2014 | 47,397 (17.18) | 33,296 (17.25) | 14,101 (17.04) | ||
2015 | 49,534 (17.96) | 34,709 (17.98) | 14,825 (17.92) | ||
Race, n (%) | χ2=3.244 | 0.518 | |||
American Indian | 1,467 (0.53) | 1,042 (0.54) | 425 (0.51) | ||
Asian | 23,857 (8.65) | 16,777 (8.69) | 7,080 (8.56) | ||
Black | 28,900 (10.48) | 20,267 (10.50) | 8,633 (10.43) | ||
Hispanic | 30,243 (10.97) | 21,089 (10.92) | 9,154 (11.06) | ||
White | 191,345 (69.38) | 133,894 (69.35) | 57,451 (69.43) | ||
Marital status, n (%) | χ2=6.373 | 0.095 | |||
Single | 42,591 (15.44) | 29,768 (15.42) | 12,823 (15.50) | ||
Married | 162,606 (58.96) | 113,668 (58.87) | 48,938 (59.14) | ||
Separated/divorced | 34,694 (12.58) | 24,286 (12.58) | 10,408 (12.58) | ||
Widowed | 35,921 (13.02) | 25,347 (13.13) | 10,574 (12.78) | ||
T stage, n (%) | Z=0.267 | 0.790 | |||
Tis & T1 | 168,672 (61.15) | 118,114 (61.18) | 50,558 (61.10) | ||
T2 | 83,921 (30.43) | 58,670 (30.39) | 25,251 (30.52) | ||
T3 | 16,301 (5.91) | 11,488 (5.95) | 4,813 (5.82) | ||
T4 | 6,918 (2.51) | 4,797 (2.48) | 2,121 (2.56) | ||
N stage, n (%) | Z=−0.692 | 0.489 | |||
N0 | 186,438 (67.60) | 130,400 (67.54) | 56,038 (67.73) | ||
N1 | 65,622 (23.79) | 46,094 (23.87) | 19,528 (23.60) | ||
N2 | 14,851 (5.38) | 10,386 (5.38) | 4,465 (5.40) | ||
N3 | 8,901 (3.23) | 6,189 (3.21) | 2,712 (3.28) | ||
Tumor size (cm), n (%) | χ2=0.485 | 0.486 | |||
<4 | 9,388 (3.40) | 6,602 (3.42) | 2,786 (3.37) | ||
≥4 | 26,6424 (96.60) | 186,467 (96.58) | 79,957 (96.63) | ||
Grade, n (%) | Z=−1.055 | 0.292 | |||
I | 63,996 (23.20) | 44,701 (23.15) | 19,295 (23.32) | ||
II | 122,430 (44.39) | 85,699 (44.39) | 36,731 (44.39) | ||
III | 88,632 (32.13) | 62,154 (32.19) | 26,478 (32.00) | ||
IV | 754 (0.27) | 515 (0.27) | 239 (0.29) | ||
Type of reporting source item, n (%) | χ2=0.001 | 0.986 | |||
Hospital inpatients | 263,759 (95.63) | 184,631 (95.63) | 79,128 (95.63) | ||
Others | 12,053 (4.37) | 8,438 (4.37) | 3,615 (4.37) | ||
Regional nodes positive, n (%) | χ2=0.988 | 0.320 | |||
No | 189,198 (68.60) | 132,328 (68.54) | 56,870 (68.73) | ||
Yes | 86,614 (31.40) | 60,741 (31.46) | 25,873 (31.27) | ||
Laterality, n (%) | χ2=1.076 | 0.300 | |||
Left | 139,797 (50.69) | 97,983 (50.75) | 41,814 (50.53) | ||
Right | 136,015 (49.31) | 95,086 (49.25) | 40,929 (49.47) | ||
First malignant primary indicator, n (%) | χ2=0.784 | 0.376 | |||
No | 39,445 (14.30) | 27,537 (14.26) | 11,908 (14.39) | ||
Yes | 236,367 (85.70) | 165,532 (85.74) | 70,835 (85.61) | ||
Malignant tumors, n (%) | Z=0.437 | 0.662 | |||
1 | 210,697 (76.39) | 147,513 (76.40) | 63,184 (76.36) | ||
2 | 53,762 (19.49) | 37,699 (19.53) | 16,063 (19.41) | ||
≥3 | 11,353 (4.12) | 7,857 (4.07) | 3,496 (4.23) | ||
Vital status, n (%) | χ2=0.415 | 0.519 | |||
Alive | 249,773 (90.56) | 174,887 (90.58) | 74,886 (90.50) | ||
Dead | 26,039 (9.44) | 18,182 (9.42) | 7,857 (9.50) | ||
Survival time (months), | 42.26±21.37 | 42.22±21.36 | 42.35±21.39 | t=−1.450 | 0.148 |
Predictors of the mortality of breast cancer patients in the training set
The results of univariate Cox regression analysis are listed in Table 2. There were significant differences in age at diagnosis, race, marital status, T stage, N stage, tumor size, grade, type of reporting source item, regional nodes positive, first malignant primary indicator, and the number of malignant tumors (all P<0.001).
Table 2
Variables | β | S.E | χ2 | P | HR | 95% CI | |
---|---|---|---|---|---|---|---|
Lower | Upper | ||||||
Age at diagnosis (years) | |||||||
20–39 | Ref | ||||||
40–59 | −0.439 | 0.037 | 139.328 | <0.001 | 0.645 | 0.600 | 0.694 |
60–79 | 0.017 | 0.036 | 0.215 | 0.643 | 1.017 | 0.948 | 1.091 |
≥80 | 1.202 | 0.038 | 1,015.921 | <0.001 | 3.325 | 3.088 | 3.580 |
Year of diagnosis | |||||||
2010 | Ref | ||||||
2011 | −0.013 | 0.021 | 0.375 | 0.540 | 0.987 | 0.947 | 1.029 |
2012 | −0.046 | 0.023 | 3.918 | 0.048 | 0.955 | 0.912 | 0.999 |
2013 | −0.037 | 0.026 | 2.042 | 0.153 | 0.964 | 0.917 | 1.014 |
2014 | −0.031 | 0.030 | 1.087 | 0.297 | 0.970 | 0.915 | 1.028 |
2015 | −0.044 | 0.038 | 1.330 | 0.249 | 0.957 | 0.888 | 1.031 |
Race | |||||||
Asian | Ref | ||||||
White | 0.465 | 0.041 | 130.719 | <0.001 | 1.591 | 1.470 | 1.723 |
Hispanic | 0.488 | 0.034 | 204.199 | <0.001 | 1.628 | 1.523 | 1.741 |
American Indian | 0.729 | 0.096 | 57.237 | <0.001 | 2.073 | 1.716 | 2.503 |
Black | 0.928 | 0.038 | 597.119 | <0.001 | 2.531 | 2.349 | 2.726 |
Marital status | |||||||
Married | Ref | ||||||
Separated/divorced | 0.396 | 0.023 | 296.106 | <0.001 | 1.486 | 1.420 | 1.555 |
Single | 0.443 | 0.021 | 433.584 | <0.001 | 1.557 | 1.493 | 1.623 |
Widowed | 1.029 | 0.018 | 3,130.847 | <0.001 | 2.797 | 2.698 | 2.900 |
T stage | |||||||
Tis & T1 | Ref | ||||||
T2 | 0.833 | 0.017 | 2,424.379 | <0.001 | 2.300 | 2.225 | 2.378 |
T3 | 1.391 | 0.024 | 3,333.377 | <0.001 | 4.019 | 3.834 | 4.213 |
T4 | 2.220 | 0.026 | 7,186.235 | <0.001 | 9.204 | 8.744 | 9.689 |
N stage | |||||||
N0 | Ref | ||||||
N1 | 0.775 | 0.017 | 2,028.604 | <0.001 | 2.171 | 2.099 | 2.245 |
N2 | 1.332 | 0.024 | 3,126.498 | <0.001 | 3.787 | 3.615 | 3.968 |
N3 | 1.858 | 0.025 | 5,660.915 | <0.001 | 6.410 | 6.107 | 6.728 |
Tumor size (cm) | |||||||
<4 | Ref | ||||||
≥4 | 1.015 | 0.065 | 246.547 | <0.001 | 2.760 | 2.432 | 3.133 |
Grade | |||||||
I | Ref | ||||||
II | 0.411 | 0.024 | 293.627 | <0.001 | 1.508 | 1.439 | 1.581 |
III | 1.079 | 0.023 | 2,175.440 | <0.001 | 2.942 | 2.812 | 3.079 |
IV | 1.278 | 0.099 | 165.585 | <0.001 | 3.588 | 2.954 | 4.359 |
Type of reporting source item | |||||||
Hospital inpatients | Ref | ||||||
Other | −0.190 | 0.039 | 23.731 | <0.001 | 0.827 | 0.766 | 0.893 |
Regional nodes positive | |||||||
No | Ref | ||||||
Yes | 1.024 | 0.015 | 4,726.128 | <0.001 | 2.785 | 2.705 | 2.867 |
Laterality | |||||||
Left | Ref | ||||||
Right | −0.023 | 0.015 | 2.455 | 0.117 | 0.977 | 0.949 | 1.006 |
First malignant primary indicator | |||||||
No | Ref | ||||||
Yes | −0.491 | 0.018 | 730.276 | <0.001 | 0.612 | 0.590 | 0.634 |
Malignant tumors | |||||||
1 | Ref | ||||||
2 | 0.481 | 0.017 | 807.050 | <0.001 | 1.618 | 1.565 | 1.672 |
≥3 | 0.854 | 0.027 | 970.342 | <0.001 | 2.348 | 2.225 | 2.478 |
S.E, standard error; HR, hazard ratio; CI, confidence interval.
Multivariate Cox regression analysis was used to assess the predictive factors of the mortality of patients with breast cancer, and the details are listed in Table 3. The findings showed that compared with women aged 20–39 years, the risk of cancer mortality in women aged 60–79 years [hazard ratio (HR) =1.484, 95% confidence interval (CI): 1.380 to 1.596] and ≥80 years (HR =3.872, 95% CI: 3.575 to 4.195) was higher, while the risk of cancer mortality in those aged 40–59 years was lower (HR =0.856, 95% CI: 0.796 to 0.922). The mortality risks of White (HR =1.456, 95% CI: 1.362 to 1.558), Hispanic (HR =1.462, 95% CI: 1.349 to 1.583), American Indian (HR =1.843, 95% CI: 1.526 to 2.227), and Black (HR =1.924, 95% CI: 1.785 to 2.074) patients were higher than Asian patients, respectively. Compared with married cases, the mortality risks of single, divorced/separated, or widowed patients were higher, with HR values of 1.285 (95% CI: 1.228 to 1.344), 1.352 (95% CI: 1.295 to 1.411), and 1.530 (95% CI: 1.469 to 1.593), respectively. Breast cancer patients with tumor size ≥4 cm had a high mortality risk compared to those with tumor size <4 cm (HR =1.322, 95% CI: 1.163 to 1.503). The first malignant primary indicator (HR =1.064, 95% CI: 1.011 to 1.120), T stage (T2, HR =1.608, 95% CI: 1.551 to 1.667; T3, HR =2.328, 95% CI: 2.210 to 2.453; T4, HR =4.110, 95% CI: 3.879 to 4.354), N stage (N2, HR =1.512, 95% CI: 1.315 to 1.738; N3, HR =2.189, 95% CI: 1.902 to 2.519), and tumor grade (II, HR =1.167, 95% CI: 1.112 to 1.224; III, HR =2.037, 95% CI: 1.942 to 2.136; IV, HR =2.411, 95% CI: 1.983 to 2.931) were associated with the mortality of women with breast cancer. Patients with positive regional nodes had a higher risk of death than in patients without (HR =1.596, 95% CI: 1.401 to 1.819). When the number of malignant tumors was 2 (HR =1.577, 95% CI: 1.510 to 1.646) or ≥3 (HR =2.223, 95% CI: 2.077 to 2.380), patients had a higher risk of death.
Table 3
Variables | β | S.E | χ2 | P | HR | 95% CI | |
---|---|---|---|---|---|---|---|
Lower | Upper | ||||||
Age at diagnosis (years) | |||||||
20–39 | Ref | ||||||
40–59 | −0.155 | 0.037 | 17.099 | <0.001 | 0.856 | 0.796 | 0.922 |
60–79 | 0.395 | 0.037 | 112.671 | <0.001 | 1.484 | 1.380 | 1.596 |
≥80 | 1.354 | 0.041 | 1,103.175 | <0.001 | 3.872 | 3.575 | 4.195 |
Race | |||||||
Asian | Ref | ||||||
White | 0.376 | 0.034 | 120.320 | <0.001 | 1.456 | 1.362 | 1.558 |
Hispanic | 0.379 | 0.041 | 86.893 | <0.001 | 1.462 | 1.349 | 1.583 |
American Indian | 0.612 | 0.096 | 40.232 | <0.001 | 1.843 | 1.526 | 2.227 |
Black | 0.654 | 0.038 | 291.673 | <0.001 | 1.924 | 1.785 | 2.074 |
Marital status | |||||||
Married | Ref | ||||||
Separated/divorced | 0.250 | 0.023 | 116.634 | <0.001 | 1.285 | 1.228 | 1.344 |
Single | 0.301 | 0.022 | 191.052 | <0.001 | 1.352 | 1.295 | 1.411 |
Widowed | 0.425 | 0.021 | 418.100 | <0.001 | 1.530 | 1.469 | 1.593 |
Tumor size (cm) | |||||||
<4 | Ref | ||||||
≥4 | 0.279 | 0.066 | 18.150 | <0.001 | 1.322 | 1.163 | 1.503 |
First malignant primary indicator | |||||||
No | Ref | ||||||
Yes | 0.062 | 0.026 | 5.636 | 0.018 | 1.064 | 1.011 | 1.120 |
Regional nodes positive | |||||||
No | Ref | ||||||
Yes | 0.468 | 0.067 | 49.407 | <0.001 | 1.596 | 1.401 | 1.819 |
T stage | |||||||
Tis & T1 | Ref | ||||||
T2 | 0.475 | 0.018 | 665.191 | <0.001 | 1.608 | 1.551 | 1.667 |
T3 | 0.845 | 0.027 | 1,010.133 | <0.001 | 2.328 | 2.210 | 2.453 |
T4 | 1.413 | 0.029 | 2,299.684 | <0.001 | 4.110 | 3.879 | 4.354 |
N stage | |||||||
N0 | Ref | ||||||
N1 | 0.078 | 0.068 | 1.332 | 0.249 | 1.082 | 0.947 | 1.236 |
N2 | 0.413 | 0.071 | 33.834 | <0.001 | 1.512 | 1.315 | 1.738 |
N3 | 0.784 | 0.072 | 119.696 | <0.001 | 2.189 | 1.902 | 2.519 |
Grade | |||||||
I | Ref | ||||||
II | 0.154 | 0.024 | 40.042 | <0.001 | 1.167 | 1.112 | 1.224 |
III | 0.711 | 0.024 | 851.166 | <0.001 | 2.037 | 1.942 | 2.136 |
IV | 0.880 | 0.100 | 77.918 | <0.001 | 2.411 | 1.983 | 2.931 |
Malignant tumors | |||||||
1 | Ref | ||||||
2 | 0.455 | 0.022 | 431.758 | <0.001 | 1.577 | 1.510 | 1.646 |
≥3 | 0.799 | 0.035 | 529.101 | <0.001 | 2.223 | 2.077 | 2.380 |
S.E, standard error; HR, hazard ratio; CI, confidence interval.
The predictive performance of the nomogram
The variables with statistical significance obtained from the multivariate Cox regression analysis were utilized to construct a nomogram to predict the 3- and 5-year survival rates of breast cancer patients, including age at diagnosis, marital status, race, T stage, N stage, grade, regional nodes positive, first malignant primary indicator, tumor size, and number of malignant tumors (Figure 1). The C-index of our nomogram using the training set was 0.782, with a standard error of 0.002. The C-index in the validation set was 0.778, with a standard error of 0.003. The ROC curves on the 3- and 5-year survival prediction were shown in Figure 2. The area under the curve (AUC) values of the nomogram for predicting the 3- and 5-year survival of breast cancer were 0.770 and 0.756, respectively. There were no differences between the training and validation sets (Z=0.383, P=0.998), indicating that the nomogram had good predictive power. The calibration curves of the nomogram for predicting the 3- and 5-year survival rates of breast cancer patients are shown in Figure 3 (the training set) and Figure 4 (the validation set).
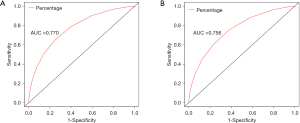
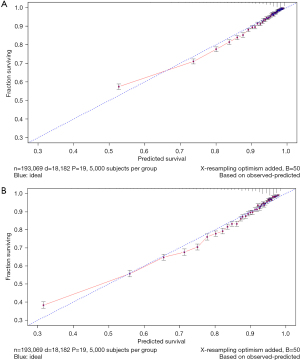
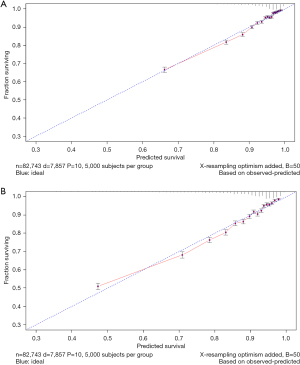
The predictive effect of the nomogram in different races
The patients from the validation set were classified into 5 risk subgroups based on race. As shown in Table 4, the C-index values of our nomogram in the Asian, White, Hispanic, American Indian, and Black groups were 0.816, 0.775, 0.773, 0.734, and 0.750, respectively. The predictive effect of the nomogram in Asian cases was superior to that in White (Z=3.927, P<0.001), Hispanic (Z=3.196, P=0.001), American Indian (Z=2.253, P=0.024), and Black (Z=5.407, P<0.001) cases with breast cancer.
Table 4
Race | C-index | S.E | Z | P |
---|---|---|---|---|
Asian | 0.816 | 0.010 | – | – |
White | 0.775 | 0.003 | 3.927 | <0.001 |
Hispanic | 0.773 | 0.009 | 3.196 | 0.001 |
American Indian | 0.734 | 0.035 | 2.253 | 0.024 |
Black | 0.750 | 0.007 | 5.407 | <0.001 |
S.E, standard error.
Discussion
In the present study, 275,812 patients with breast cancer were screened from the SEER database. We found that the age at diagnosis, marital status, race, T stage, N stage, grade, regional nodes positive, first malignant primary indicator, tumor size, and number of malignant tumors were independent predictors for the death of patients with breast cancer. A nomogram that contained the predictive factors associated with breast cancer death was established. The C-indexes of the nomogram for the training and validation sets were 0.782 and 0.778, respectively. Our findings indicated that our nomogram had predictive ability for predicting the 3- and 5-year survival rates of breast cancer patients.
Previous studies have reported that nomograms play an important role in the personalized prediction of cancer (22-25), which helps clinicians optimize the therapeutic protocols of patients on the basis of individual information. The nomogram is a visual tool to effectively reflect the results of Cox regression analysis. The most valuable aspect of a nomogram is in predicting the outcomes, and the length of the lines can be used to indicate the impact of different variables on the outcomes, as well as the effects of various values of these variables on the outcomes. The larger the C-index, the more accurate the predictive effectiveness of the nomogram (26). However, the predictive power of the nomogram is limited in predicting the mortality risk of breast cancer patients, especially for different ethnicities. In this study, we developed a nomogram according to the related predictive factors from the general information and tumor characteristics of patients in the SEER database to estimate the 3- and 5-year survival rates of patients with breast cancer, and further assessed the predictive performance of the nomogram for the survival of breast cancer patients with different ethnicities.
The predictive factors were considered in the nomogram on the basis of our findings obtained from the univariate and multivariate analyses. We discovered that the risk of cancer death in patients aged 40–59, 60–79, and >80 years was higher than that in the 20–39 years age group. It was suggested that the risk of dying from breast cancer gradually increased with the increase of age. This may be related to less aggressive treatments, poor endurance of stress, impaired compensatory mechanisms, or other chronic diseases in the elderly, whereas younger patients may often exhibit more aggressive biological behaviors of malignant tumors (27). Cases with malignant tumors had a higher risk of death than cases with in situ tumors. The occurrence of lymph node metastases in patients resulted in a higher risk of death than in patients without lymph node metastases. Early evidence showed that lymph node metastases is a common clinical feature in the progression of breast cancer (28-30). Compared with patients with grade I tumors, the mortality risks of those with grade II, III, and IV tumors were higher, with HR values of 1.167, 2.037, and 2.411, respectively. Our results found that tumor size ≥4 cm could increase the mortality risk of breast cancer. When the number of malignant tumors was over 2, patients had a higher risk of death. It was indicated that high tumor grade, large tumor size, and a large number of tumors were associated with cancer death.
Validation of the nomogram was carried out through randomly selecting 275,812 patients with breast cancer. The nomogram showed greater discrimination for the prediction of the survival time of patients, and the C-index for the validation set was 0.778, indicating that our nomogram had good effectiveness in predicting the prognosis of breast cancer patients. Furthermore, patients in the validation set were further classified into different ethnicities. Ethnicity is an independent risk factor of the occurrence of breast cancer and affects the prognosis of breast cancer. In the current study, we discovered that the predictive performance of the nomogram in Asian patients was superior to that in other ethnicities, which may suggest that the prognosis of breast cancer is racially heterogeneous. Hence, the specific race-based risk and prognostic prediction model was more precise and personalized for outcome prediction.
This was a population-based study that covered U.S. cancer registry data of high quality. The SEER database incorporates relatively complete follow-up information for breast cancer, indicating that the death-related information is reliable. Nonetheless, some limitations should be noted. More information on systemic therapy, such as targeted therapy records and chemotherapy protocols, was not available in the SEER database, and the predictive factors were only limited to this database. Additionally, this nomogram was developed using retrospective data, and further studies with external validation in large and prospective cohorts are needed.
Conclusions
In summary, we established and validated a nomogram based on the SEER database to predict the 3- and 5-year survival of breast cancer patients. Our nomogram had the effectiveness for predicting survival, especially in the Asian population, which may provide clinicians with useful guidelines for the individualized treatment of patients.
Acknowledgments
Funding: None.
Footnote
Reporting Checklist: The authors have completed the TRIPOD reporting checklist. Available at https://gs.amegroups.com/article/view/10.21037/gs-22-321/rc
Conflicts of Interest: All authors have completed the ICMJE uniform disclosure form (available at https://gs.amegroups.com/article/view/10.21037/gs-22-321/coif). The authors have no conflicts of interest to declare.
Ethical Statement: The authors are accountable for all aspects of the work in ensuring that questions related to the accuracy or integrity of any part of the work are appropriately investigated and resolved. The study was conducted in accordance with the Declaration of Helsinki (as revised in 2013).
Open Access Statement: This is an Open Access article distributed in accordance with the Creative Commons Attribution-NonCommercial-NoDerivs 4.0 International License (CC BY-NC-ND 4.0), which permits the non-commercial replication and distribution of the article with the strict proviso that no changes or edits are made and the original work is properly cited (including links to both the formal publication through the relevant DOI and the license). See: https://creativecommons.org/licenses/by-nc-nd/4.0/.
References
- Siegel RL, Miller KD, Fuchs HE, et al. Cancer statistics, 2022. CA Cancer J Clin 2022;72:7-33. [Crossref] [PubMed]
- Kubo M. Adjuvant endocrine treatment for estrogen receptor (ER)-positive/HER2-negative breast cancer. Chin Clin Oncol 2020;9:33. [Crossref] [PubMed]
- Hanson SE, Lei X, Roubaud MS, et al. Long-term Quality of Life in Patients With Breast Cancer After Breast Conservation vs Mastectomy and Reconstruction. JAMA Surg 2022;157:e220631. [Crossref] [PubMed]
- Nitz U, Gluz O, Graeser M, et al. De-escalated neoadjuvant pertuzumab plus trastuzumab therapy with or without weekly paclitaxel in HER2-positive, hormone receptor-negative, early breast cancer (WSG-ADAPT-HER2+/HR-): survival outcomes from a multicentre, open-label, randomised, phase 2 trial. Lancet Oncol 2022;23:625-35. [Crossref] [PubMed]
- Katanoda K, Matsuda T. Five-year relative survival rate of breast cancer in the USA, Europe and Japan. Jpn J Clin Oncol 2014;44:611. [Crossref] [PubMed]
- Balachandran VP, Gonen M, Smith JJ, et al. Nomograms in oncology: more than meets the eye. Lancet Oncol 2015;16:e173-80. [Crossref] [PubMed]
- Gao J, Ren Y, Guo H, et al. A new method for predicting survival in stage I non-small cell lung cancer patients: nomogram based on macrophage immunoscore, TNM stage and lymphocyte-to-monocyte ratio. Ann Transl Med 2020;8:470. [Crossref] [PubMed]
- Luo LM, Wang Y, Lin PX, et al. The clinical outcomes, prognostic factors and nomogram models for primary lung cancer patients treated with stereotactic body radiation therapy. Front Oncol 2022;12:863502. [Crossref] [PubMed]
- Yi J, Liu Z, Wang L, et al. Development and validation of novel nomograms to predict the overall survival and cancer-specific survival of cervical cancer patients with lymph node metastasis. Front Oncol 2022;12:857375. [Crossref] [PubMed]
- Han Y, Wen X, Chen D, et al. Survival analysis and a novel nomogram model for progression-free survival in patients with prostate Cancer. J Oncol 2022;2022:6358707. [Crossref] [PubMed]
- Luo D, Li H, Yu H, et al. Predictive value of preoperative and postoperative peripheral lymphocyte difference in hepatitis B virus-related hepatocellular cancer patients: Based on the analysis of dynamic nomogram. J Surg Oncol 2020;122:1553-68. [Crossref] [PubMed]
- Rudolph A, Song M, Brook MN, et al. Joint associations of a polygenic risk score and environmental risk factors for breast cancer in the breast cancer association consortium. Int J Epidemiol 2018;47:526-36. [Crossref] [PubMed]
- Ma X, Liu C, Xu X, et al. Biomarker expression analysis in different age groups revealed age was a risk factor for breast cancer. J Cell Physiol 2020;235:4268-78. [Crossref] [PubMed]
- Schembre SM, Jospe MR, Giles ED, et al. A Low-Glucose Eating Pattern Improves Biomarkers of Postmenopausal Breast Cancer Risk: An Exploratory Secondary Analysis of a Randomized Feasibility Trial. Nutrients 2021;13:4508. [Crossref] [PubMed]
- Sun YS, Zhao Z, Yang ZN, et al. Risk factors and preventions of breast cancer. Int J Biol Sci 2017;13:1387-97. [Crossref] [PubMed]
- James FR, Wootton S, Jackson A, et al. Obesity in breast cancer--what is the risk factor? Eur J Cancer 2015;51:705-20. [Crossref] [PubMed]
- Kim SE, Bachorik AE, Bertrand KA, et al. Differences in breast cancer screening practices by diabetes status and race/ethnicity in the United States. J Womens Health (Larchmt) 2022;31:848-55. [Crossref] [PubMed]
- Huang X, Luo Z, Liang W, et al. Survival Nomogram for Young Breast Cancer Patients Based on the SEER Database and an External Validation Cohort. Ann Surg Oncol 2022; Epub ahead of print. [Crossref] [PubMed]
- Johnson RH, Anders CK, Litton JK, et al. Breast cancer in adolescents and young adults. Pediatr Blood Cancer 2018;65:e27397. [Crossref] [PubMed]
- Chihara D, Oki Y, Fanale MA, et al. Stage I non-Hodgkin lymphoma: no plateau in disease-specific survival? Ann Hematol 2019;98:1169-76. [Crossref] [PubMed]
- Harrell FE Jr, Lee KL, Mark DB. Multivariable prognostic models: issues in developing models, evaluating assumptions and adequacy, and measuring and reducing errors. Stat Med 1996;15:361-87. [Crossref] [PubMed]
- Xu X, Wang H, Du P, et al. A predictive nomogram for individualized recurrence stratification of bladder cancer using multiparametric MRI and clinical risk factors. J Magn Reson Imaging 2019;50:1893-904. [Crossref] [PubMed]
- Dong D, Tang L, Li ZY, et al. Development and validation of an individualized nomogram to identify occult peritoneal metastasis in patients with advanced gastric cancer. Ann Oncol 2019;30:431-8. [Crossref] [PubMed]
- Tang XR, Li YQ, Liang SB, et al. Development and validation of a gene expression-based signature to predict distant metastasis in locoregionally advanced nasopharyngeal carcinoma: a retrospective, multicentre, cohort study. Lancet Oncol 2018;19:382-93. [Crossref] [PubMed]
- Cambier S, Sylvester RJ, Collette L, et al. EORTC nomograms and risk groups for predicting recurrence, progression, and disease-specific and overall survival in non-muscle-invasive stage Ta-T1 urothelial bladder cancer patients treated with 1-3 years of maintenance bacillus Calmette-Guérin. Eur Urol 2016;69:60-9. [Crossref] [PubMed]
- Huang YQ, Liang CH, He L, et al. Development and validation of a radiomics nomogram for preoperative prediction of lymph node metastasis in colorectal cancer. J Clin Oncol 2016;34:2157-64. [Crossref] [PubMed]
- Chen M, Cao J, Zhang B, et al. A nomogram for prediction of overall survival in patients with node-negative gallbladder cancer. J Cancer 2019;10:3246-52. [Crossref] [PubMed]
- Ginter PS, Karagiannis GS, Entenberg D, et al. Tumor Microenvironment of metastasis (TMEM) doorways are restricted to the blood vessel endothelium in both primary breast cancers and their lymph node metastases. Cancers (Basel) 2019;11:1507. [Crossref] [PubMed]
- Golden JA. Deep Learning algorithms for detection of lymph node metastases from breast cancer: Helping Artificial Intelligence Be Seen. JAMA 2017;318:2184-6. [Crossref] [PubMed]
- Weigelt B, Peterse JL, van 't Veer LJ. Breast cancer metastasis: markers and models. Nat Rev Cancer 2005;5:591-602. [Crossref] [PubMed]
(English Language Editor: C. Betlazar-Maseh)