Identification of immune-related biomarkers for predicting neoadjuvant chemotherapy sensitivity in HER2 negative breast cancer via bioinformatics analysis
Introduction
Breast cancer (BC) ranks number one among all female malignancies, with 281,550 new diagnoses in 2021, accounting for 30% of all female cancer cases (1). With recent advances in medical treatments, the mortality rate of BC patients continues to decrease, and in 2021, the mortality rate was 15% (1). One of the key treatment strategies for BC is neoadjuvant chemotherapy (NAC), which refers to chemotherapy before surgery. NAC aims to reduce the tumor size, thereby facilitating surgery in otherwise inoperable patients or patients for whom breast-conserving surgery is not an option (2,3). In recent years, increasing numbers of BC patients have undergoing NAC with favorable results (4).
Human epidermal growth factor receptor 2 (HER2) is an important therapeutic target for breast cancer (5). HER2 is a specific antigen expressed on breast cancer tumor cells. Combined with anti-HER2 drugs such as trastuzumab, it can mediate antibody-dependent cell-mediated cytotoxicity (ADCC) effect and kill tumor cells (6). In the past 10 years, anti-HER-2 target therapy has significantly improved the prognosis of breast cancer, and about 75% of metastatic HER2-positive patients who undergo NAC can achieve pathological complete response (pCR) (7). However, about 70–80% of BC patients are HER2-negative, and the response of these patients to NAC is much lower than that of HER2 positive patients (8,9). For HER2-negative patients, including intracavitary and triple-negative breast cancer (TNBC) patients, in the reason of the lack of specific therapeutic targets for breast cancer cells by traditional anthracycline and paclitaxel drugs, conventional chemotherapy regimens can only achieve pCR in about 20–40% of patients (10-12). The low pCR rate of HER2-negative BC patients necessitates the search for novel biomarkers to identify which patients are likely to acquire pCR after NAC. Cancer immunotherapy is a treatment that identifies and attacks cancer cells by manipulating the immune system (13). There have been some breakthroughs in a variety of solid malignant tumors and hematological malignant tumors (13). Immunotherapy in BC patients mainly aims to block antibodies against programmed death 1 (PD-1)/programmed death ligand 1 (PD-L1), and this has been shown to achieve strong local tumor control and lasting response in BC patients (14). While immunotherapy is suitable for a portion of TNBC patients, at present, there are no effective immunotherapy drugs for the treatment of luminal BCs (15,16). For patients with HER-negative BC, study has shown that the expression of maternal embryonic leucine zipper kinase (MELK) in breast cancer is related to immune cell infiltration and pCR after NAC (17).
From a previous study, pCR predictors such as prediction analysis of microarray 50 (PAM50), integrated clustering (intcluster), phosphatidylinositol-4,5-bisphosphate 3-kinase catalytic subunit alpha (PI3KCA) mutation or trastuzumab risk model (TRAR) are useful molecular tools to evaluate treatment response and prognosis (18). These predictive tools have limit investigate with tumor immunity. Therefore, present study wants to further explore the relationship between immunity and the prediction of NAC treatment effect in breast cancer, and analyze whether the expression of immune genes can predict pCR in BC patients with NAC. We identified differentially expressed genes (DEGs) between the 51 patients with pCR and the 108 patients with residual disease (RD), and constructed a prediction model to determine the accuracy of predicting the NAC sensitive in BC patients. We present the following article in accordance with the STARD reporting checklist (available at https://gs.amegroups.com/article/view/10.21037/gs-22-234/rc).
Methods
Research process
The raw data of GSE163882 was downloaded from the Gene Expression Omnibus (GEO) database. We compared pCR and RD patients to obtain the DEGs. Functional enrichment analyses were then conducted on these DEGs. We intersect the DEGs and immune-related genes to obtain the hub immune biomarkers, and then use the linear fitting model to construct a prediction model to predicting the NAC sensitive in BC patients. Finally, the single sample gene set enrichment analysis (ssGSEA) algorithm was used to analyze immune cell invasion in BC patients.
Raw data
The GSE163882 dataset, containing 222 BC patients treated with NAC, was downloaded from the GEO database. There were 159 HER2 negative and 63 HER2 positive patients. The data from the 159 HER2 negative patients were used for subsequent analyses. The list of immune-related genes was obtained from ImPort share data (https://www.immport.org/shared/home). The study was conducted in accordance with the Declaration of Helsinki (as revised in 2013).
Identification of the differentially expressed genes between pathological complete response samples and residual disease samples
Among the 159 HER2 negative BC patients, 51 reached pCR and 108 reached RD after NAC. The pCR and RD patients were compared to obtain the DEGs using the “limma” package in R software, with the threshold set to log2 fold change |logFC| >1 and false discovery rate (FDR) <0.05. pCR means no residual invasive tumor cells were found in the surgical specimens of primary breast; RD means there's still residual invasive tumor cells residual in the surgical specimens of primary breast.
Functional enrichment analysis
After obtaining the DEGs, the “clusterProfiler” package in R software was used to analyze the functional enrichment of the DEGs. Enrichment function analysis including Gene Ontology (GO) and Kyoto Encyclopedia of Genes and Genomes (KEGG) were performed.
Construction of the protein-protein interaction (PPI) network
The PPI network of DEGs was constructed through the STRING online website (https://string-db.org/) and visualized using the Cytoscape software.
The expression of immune-related differentially expressed genes in breast cancer and the construction of a single- and multi-index diagnostic model
The intersection of the DEGs and immune-related genes was used to obtain the immune-related DEGs. The expression of these immune-related DEGs was compared between RD and pCR patients. The ROC curves of the expression levels of these immune-related DEGs were constructed using the “pROC” package and linearly combined to construct a multi-index diagnostic model using the “glm” R function.
Single sample gene set enrichment analysis (ssGSEA) of immune cell abundance in breast cancer patients
The “GSEABase” and “GSVA” packages were used to identify the abundance of 28 types of immune cells in pCR and RD patients. Furthermore, the relationship between immune cells and immune-related DEGs in BC patients was analyzed.
Statistical analysis
The R software was used for all data analysis in the present study. Differential analysis of pCR and RD BC patients was performed using the limma package. Wilcoxon non-parametric tests and Spearman’s method were used to compare the differences and correlation between the two groups. A P value <0.05 (two-sided) was considered statistically significant.
Results
The differentially expressed genes between pathological complete response and residual disease patients
Compared with RD patients, a total of 45 DEGs were identified in pCR patients, of which, 17 were upregulated and 28 were downregulated (Figure 1A,1B).
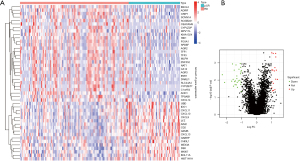
Functional enrichment analysis
The results of the GO functional enrichment analysis of the DEGs indicated that antimicrobial humoral response, antimicrobial humoral immune response mediated by antimicrobial peptide, lymphocyte chemotaxis, lung epithelial cell differentiation, and lung cell differentiation were the top five biological processes (BPs). Apical plasma membrane, apical part of the cell, chloride channel complex, specific granule lumen, and Golgi medial cisterna were the top five cell components (CC). Chemokine activity, chemokine receptor binding, cytokine activity, CXCR chemokine receptor binding, and dystroglycan binding were the top five molecular functions (MFs) (Figure 2A,2B).
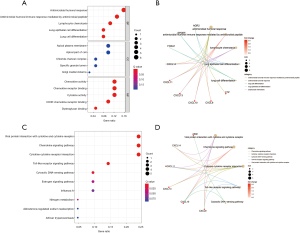
KEGG analysis indicated that viral protein interaction with cytokine and cytokine receptors, chemokine signaling pathway, cytokine-cytokine receptor interaction, Toll-like receptor signaling pathway, cytosolic DNA-sensing pathway, estrogen signaling pathway, influenza A, nitrogen metabolism, aldosterone-regulated sodium reabsorption, and African trypanosomiasis were the top 10 enriched KEGG signaling pathways (Figure 2C,2D).
Protein-protein interaction network of the differentially expressed genes and the immune-related differentially expressed genes
The PPI network constructed with the 45 DEGs resulted in a total of 30 nodes and 56 edges. The top nodes were anterior gradient 2, protein disulphide isomerase family member (AGR2), C-X-C motif chemokine ligand 10 (CXCL10), CXCL9, and estrogen receptor 1 (ESR1) (Figure 3A,3B). Based on the intersection of the immune-related genes and the DEGs, 9 hub immune-related DEGs were obtained (Figure 3C).

The expression of the 9 immune-related genes in pathological complete response and residual disease patients
Among the 9 immune-related DEGs, CXCL9, CXCL10, CXCL11, CXCL13, granzyme B (GZMB), indoleamine 2,3-dioxygenase 1 (IDO1), and lysozyme (LYZ) were highly expressed in pCR patients, while CXCL14 and ESR1 were lowly expressed in pCR patients compared to RD patients (Figure 4A-4I).
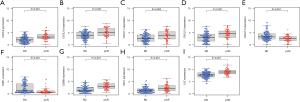
The diagnostic value of the 9 immune-related DEGs in pathological complete response breast cancer patients (ROC curve)
At the single-gene expression level, the AUC values of the ROC curve for pCR patient diagnosis were as follows: CXCL9 =0.722; CXCL10 =0.690; CXCL11 =0.654; CXCL13 =0.707; CXCL14 =0.659; ESR1 =0.682; GZMB =0.760; IDO1 =0.716; and LYZ =0.764 (Figure 5A-5I).
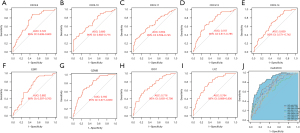
At the multi-gene expression model (model = −6.222−0.280* CXCL9 +0.114* CXCL10 −0.324* CXCL11 −0.004* CXCL13 −0.367* CXCL14 −0.179* ESR1 +0.405* GZMB +0.459* IDO1 +0.769* LYZ), the AUC value of the ROC curve in the diagnosis of pCR patients was 0.844 (Figure 5J).
The relationship between immune cells and immune-related genes
Among the 28 immune cell types examined, 15 immune cell types showed high-infiltration in pCR patients compared to RD patients, including activated CD8 T cells, effector memory CD8 T cells, activated CD4 T cells, T follicular helper cells, gamma delta T cells, type 1 T helper cells, type 2 T helper cells, regulatory T cells, activated B cells, immature B cells, memory B cells, myeloid derived suppressor cells, natural killer T cell, activated dendritic cells, and plasmacytoid dendritic cells. Meanwhile, CD56 dim natural killer cells showed low infiltration in pCR patients (Figure 6A).
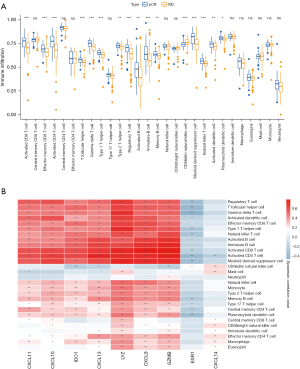
There was a positive correlation between the expression levels of the 9 immune-related genes and the abundance of immune cell infiltration, especially with CXCL9, CXCL10, CXCL11, CXCL13, CXCL14, IDO1, LYZ and GZMB. However, ESR1 was negatively correlated with immune cell infiltration levels (Figure 6B).
Discussion
In patients with stage II or III BC, preoperative NAC has several advantages. First, chemotherapy can shrink and downgrade the tumor, enabling surgery or breast-conserving surgery in patients who would otherwise be unsuitable for such treatments. Second, the follow-up treatment plan can be formulated according to the efficacy of chemotherapy, which can be used as a marker of prognosis (3,19). High pCR rates can be achieved with anti-HER2-targeted therapy in HER2-positive BC patients. However, HER2-negative is the most common pathological type of BC, especially estrogen receptor (ER) positive BC, which is associated with poorer response to chemotherapy (20). Hence, for HER2-negative patients, there is an urgent need to identify the biomarkers which respond to chemotherapy, and find effective HER2-negative diagnostic markers to identify which patients are likely to acquire pCR. This has important guiding significance for the selection of subsequent treatment options.
Epigenetics plays an important role in the pathogenesis of BC drug resistance. For example, changes in gene expression levels and DNA methylation silencing key genes can inhibit related signaling pathways, leading to drug resistance in BC (21,22). There is much evidence to show that cytokines play an important role in the treatment of drug resistance in BC. For instance, interleukin (IL)-6, IL-8, and tumor necrosis factor-α (TNF-α) can significantly regulate epithelial-mesenchymal transformation (EMT)-mediated drug resistance (23-26). In the present study, a total of 45 DEGs were screened from pCR and RD patients, including 17 upregulated and 28 downregulated genes. The results of functional enrichment analysis indicated that viral protein interaction with cytokine and cytokine receptors, chemokine signaling pathways, cytokine-cytokine receptor interaction, and Toll-like receptor signaling pathways are related to the most abundant DEGs. To further investigate the roles of key immune-related genes in these signaling pathways, 9 key immune genes were obtained by intersecting immune-related genes and the DEGs. The results indicated that CXCL9, CXCL10, CXCL11, CXCL13, GZMB, IDO1, and LYZ were highly expressed in pCR patients compared to RD patients, while CXCL14 and ESR1 were lowly expressed in pCR patients. A study by Chen et al. demonstrated that high expression of CXCL9 can not only inhibit the proliferation and apoptosis of BC cells, but can also reduce the IC50 of taxanes, which indicates that CXCL9 plays an important role in the resistance of chemotherapeutic drugs (27). Our results showed that CXCL9 is highly expressed in BCs with pCR, which is consistent with Chen’s report. Dai et al. demonstrated in vitro that CXCL10 was correlated with the NAC response on account of the hypoxia inducible factor-1α (HIF1α)/interleukin 17 (IL-17A)/CXCL10 pathway affecting the sensitivity of BC cells to paclitaxel (28). The results of our study also revealed that CXCL10 is highly expressed in pCR patients, indicating that a higher expression level of CXCL10 may result in pCR in BC patients. Several studies have shown that CXCL11, CXCL13, and CXCL14 biomarkers are closely related to chemotherapeutic resistance in BC (29-33). ESR1 encodes estrogen receptor α (ERα) and plays an important role in the tumorigenesis of BC (34). The vast majority of BCs are ER positive and endocrine sensitive but not sensitive to chemotherapy (35,36). Our results showed that the expression of ESR1 is low in pCR BCs, suggesting that high expression of ESR1 may inhibit the sensitivity of tumor cells to chemotherapeutic drugs. The combined results of above previous studies and this current report suggests that the 9 hub immune-related genes identified in the present study may play an important role in the chemotherapeutic resistance of BC cells. Indeed, a linear model for predicting pCR in BC patients following NAC was established based on these hub genes. The model has high diagnostic value (AUC =0.844) and has the potential to be used in practical clinical applications.
The tumor microenvironment, including cancer-associated fibroblast (CAFs), mesenchymal cells, tumor-associated macrophages (TAMs), and endothelial cells, plays an important role in the occurrence and development, recurrence and metastasis, and chemotherapeutic resistance of BC (37,38). Macrophages are one of the most important types of immune cells in the TME stroma of BCs. Several in vitro and in vivo studies have shown that TAMs affect the response of tumor cells to paclitaxel, doxorubicin, and cyclophosphamide (39,40). The mechanisms may involve TAM-derived IL-10 increasing the resistance of BC cells to paclitaxel (41). However, in the present study, no significant difference was found in the abundance of TAMs between RD and pCR patients, as calculated by the ssGSEA algorithm. Nonetheless, the abundance of macrophages was positively correlated with the expression levels of key immune genes in this research (except ESR1), suggesting that the immune-related genes screened in this study may be potential biomarkers for chemotherapeutic drug resistance in BC. In vitro study by Gomes-Santos et al. demonstrated that exercise training in mice promoted the infiltration of CD8+T cells and made refractory breast cancer sensitive to treatment through the causal effect of the CXCL9/CXCL11/C-X-C motif chemokine receptor 3 (CXCR3) pathway. Exercise training can also normalize the tumor vascular system, reshape tumor microenvironment, and enhance CD8+T cell-mediated anti-tumor activity through CXCR3 (33). In this study, the results of the immune cell infiltration analysis showed that activated CD8 T cells, effector memory CD8 T cells, and activated CD4 T immune cells were highly infiltrated in pCR compared to RD patients. Although tumor immunotherapy has made great achievements in non-small cell lung cancer, its effect on epithelial solid tumors, including breast cancer, has been limited (42,43). This current investigation was limited by the lack of experimental verification of the key genes identified. In the future, further gene functional verification experiments should be performed investigations should continue to identify novel and effective targets for the diagnosis and treatment of BC.
Conclusions
In summary, this study ultimately identified 9 immune-related biomarkers as potential tools for assessing the sensitivity to NAC in BC patients, which will help clinicians predict a patient’s likely response to chemotherapy based on model scores.
Acknowledgments
Funding: This work was supported by Scientific Research and Technology Development Plan of Baise City (No. 202209+14).
Footnote
Reporting Checklist: The authors have completed the STARD reporting checklist. Available at https://gs.amegroups.com/article/view/10.21037/gs-22-234/rc
Conflicts of Interest: All authors have completed the ICMJE uniform disclosure form (available at https://gs.amegroups.com/article/view/10.21037/gs-22-234/coif). The authors have no conflicts of interest to declare.
Ethical Statement: The authors are accountable for all aspects of the work in ensuring that questions related to the accuracy or integrity of any part of the work are appropriately investigated and resolved. The study was conducted in accordance with the Declaration of Helsinki (as revised in 2013).
Open Access Statement: This is an Open Access article distributed in accordance with the Creative Commons Attribution-NonCommercial-NoDerivs 4.0 International License (CC BY-NC-ND 4.0), which permits the non-commercial replication and distribution of the article with the strict proviso that no changes or edits are made and the original work is properly cited (including links to both the formal publication through the relevant DOI and the license). See: https://creativecommons.org/licenses/by-nc-nd/4.0/.
References
- Siegel RL, Miller KD, Fuchs HE, et al. Cancer Statistics, 2021. CA Cancer J Clin 2021;71:7-33. [Crossref] [PubMed]
- Montemurro F, Nuzzolese I, Ponzone R. Neoadjuvant or adjuvant chemotherapy in early breast cancer? Expert Opin Pharmacother 2020;21:1071-82. [Crossref] [PubMed]
- Korde LA, Somerfield MR, Carey LA, et al. Neoadjuvant Chemotherapy, Endocrine Therapy, and Targeted Therapy for Breast Cancer: ASCO Guideline. J Clin Oncol 2021;39:1485-505. [Crossref] [PubMed]
- Loibl S, Poortmans P, Morrow M, et al. Breast cancer. Lancet 2021;397:1750-69. [Crossref] [PubMed]
- Leon-Ferre RA, Hieken TJ, Boughey JC. The Landmark Series: Neoadjuvant Chemotherapy for Triple-Negative and HER2-Positive Breast Cancer. Ann Surg Oncol 2021;28:2111-9. [Crossref] [PubMed]
- Ferraro E, Drago JZ, Modi S. Implementing antibody-drug conjugates (ADCs) in HER2-positive breast cancer: state of the art and future directions. Breast Cancer Res 2021;23:84. [Crossref] [PubMed]
- Loibl S, Gianni L. HER2-positive breast cancer. Lancet 2017;389:2415-29. [Crossref] [PubMed]
- Torrisi R, Marrazzo E, Agostinetto E, et al. Neoadjuvant chemotherapy in hormone receptor-positive/HER2-negative early breast cancer: When, why and what? Crit Rev Oncol Hematol 2021;160:103280. [Crossref] [PubMed]
- Burstein HJ. Systemic Therapy for Estrogen Receptor-Positive, HER2-Negative Breast Cancer. N Engl J Med 2020;383:2557-70. [Crossref] [PubMed]
- Pusztai L, Yau C, Wolf DM, et al. Durvalumab with olaparib and paclitaxel for high-risk HER2-negative stage II/III breast cancer: Results from the adaptively randomized I-SPY2 trial. Cancer Cell 2021;39:989-98.e5. [Crossref] [PubMed]
- Liu M, Liu S, Yang L, et al. Comparison between nab-paclitaxel and solvent-based taxanes as neoadjuvant therapy in breast cancer: a systematic review and meta-analysis. BMC Cancer 2021;21:118. [Crossref] [PubMed]
- Boland MR, Al-Maksoud A, Ryan ÉJ, et al. Value of a 21-gene expression assay on core biopsy to predict neoadjuvant chemotherapy response in breast cancer: systematic review and meta-analysis. Br J Surg 2021;108:24-31. [Crossref] [PubMed]
- Kennedy LB, Salama AKS. A review of cancer immunotherapy toxicity. CA Cancer J Clin 2020;70:86-104. [Crossref] [PubMed]
- Sugie T. Immunotherapy for metastatic breast cancer. Chin Clin Oncol 2018;7:28. [Crossref] [PubMed]
- Keenan TE, Tolaney SM. Role of Immunotherapy in Triple-Negative Breast Cancer. J Natl Compr Canc Netw 2020;18:479-89. [Crossref] [PubMed]
- Franzoi MA, Romano E, Piccart M. Immunotherapy for early breast cancer: too soon, too superficial, or just right? Ann Oncol 2021;32:323-36. [Crossref] [PubMed]
- Oshi M, Gandhi S, Huyser MR, et al. MELK expression in breast cancer is associated with infiltration of immune cell and pathological compete response (pCR) after neoadjuvant chemotherapy. Am J Cancer Res 2021;11:4421-37. [PubMed]
- Chica-Parrado MR, Godoy-Ortiz A, Jiménez B, et al. Resistance to Neoadjuvant Treatment in Breast Cancer: Clinicopathological and Molecular Predictors. Cancers (Basel) 2020;12:2012. [Crossref] [PubMed]
- Burstein HJ, Curigliano G, Thürlimann B, et al. Customizing local and systemic therapies for women with early breast cancer: the St. Gallen International Consensus Guidelines for treatment of early breast cancer 2021. Ann Oncol 2021;32:1216-35. [Crossref] [PubMed]
- Bartsch R. ASCO 2020: highlights in breast cancer. Memo 2021;14:58-61. [Crossref] [PubMed]
- Garcia-Martinez L, Zhang Y, Nakata Y, et al. Epigenetic mechanisms in breast cancer therapy and resistance. Nat Commun 2021;12:1786. [Crossref] [PubMed]
- Deblois G, Tonekaboni SAM, Grillo G, et al. Epigenetic Switch-Induced Viral Mimicry Evasion in Chemotherapy-Resistant Breast Cancer. Cancer Discov 2020;10:1312-29. [Crossref] [PubMed]
- Morel AP, Lièvre M, Thomas C, et al. Generation of breast cancer stem cells through epithelial-mesenchymal transition. PLoS One 2008;3:e2888. [Crossref] [PubMed]
- Mani SA, Guo W, Liao MJ, et al. The epithelial-mesenchymal transition generates cells with properties of stem cells. Cell 2008;133:704-15. [Crossref] [PubMed]
- Fernando RI, Castillo MD, Litzinger M, et al. IL-8 signaling plays a critical role in the epithelial-mesenchymal transition of human carcinoma cells. Cancer Res 2011;71:5296-306. [Crossref] [PubMed]
- Asiedu MK, Ingle JN, Behrens MD, et al. TGFbeta/TNF(alpha)-mediated epithelial-mesenchymal transition generates breast cancer stem cells with a claudin-low phenotype. Cancer Res 2011;71:4707-19. [Crossref] [PubMed]
- Chen D, Bao C, Zhao F, et al. Exploring Specific miRNA-mRNA Axes With Relationship to Taxanes-Resistance in Breast Cancer. Front Oncol 2020;10:1397. [Crossref] [PubMed]
- Dai H, Sheng X, Wang Y, et al. HIF1alpha Regulates IL17 Signaling Pathway Influencing Sensitivity of Taxane-Based Chemotherapy for Breast Cancer. Front Cell Dev Biol 2021;9:729965. [Crossref] [PubMed]
- Xu Y, Zhang Z, Zhang L, et al. Novel module and hub genes of distinctive breast cancer associated fibroblasts identified by weighted gene co-expression network analysis. Breast Cancer 2020;27:1017-28. [Crossref] [PubMed]
- Su P, Peng Z, Xu B, et al. Establishment and validation of an individualized macrophage-related gene signature to predict overall survival in patients with triple negative breast cancer. PeerJ 2021;9:e12383. [Crossref] [PubMed]
- Qiu P, Guo Q, Yao Q, et al. Characterization of Exosome-Related Gene Risk Model to Evaluate the Tumor Immune Microenvironment and Predict Prognosis in Triple-Negative Breast Cancer. Front Immunol 2021;12:736030. [Crossref] [PubMed]
- Liu Y, Zhang J, Sun X, et al. Down-regulation of miR-29b in carcinoma associated fibroblasts promotes cell growth and metastasis of breast cancer. Oncotarget 2017;8:39559-70. [Crossref] [PubMed]
- Gomes-Santos IL, Amoozgar Z, Kumar AS, et al. Exercise Training Improves Tumor Control by Increasing CD8+ T-cell Infiltration via CXCR3 Signaling and Sensitizes Breast Cancer to Immune Checkpoint Blockade. Cancer Immunol Res 2021;9:765-78. [Crossref] [PubMed]
- Karsono R, Haryono SJ, Karsono B, et al. ESR1 PvuII polymorphism: from risk factor to prognostic and predictive factor of the success of primary systemic therapy in advanced breast cancer. BMC Cancer 2021;21:1348. [Crossref] [PubMed]
- Mayayo-Peralta I, Faggion B, Hoekman L, et al. Ribociclib Induces Broad Chemotherapy Resistance and EGFR Dependency in ESR1 Wildtype and Mutant Breast Cancer. Cancers (Basel) 2021;13:6314. [Crossref] [PubMed]
- Allouchery V, Beaussire L, Perdrix A, et al. Circulating ESR1 mutations at the end of aromatase inhibitor adjuvant treatment and after relapse in breast cancer patients. Breast Cancer Res 2018;20:40. [Crossref] [PubMed]
- Mehraj U, Dar AH, Wani NA, et al. Tumor microenvironment promotes breast cancer chemoresistance. Cancer Chemother Pharmacol 2021;87:147-58. [Crossref] [PubMed]
- Bahrami A, Hassanian SM, Khazaei M, et al. The Therapeutic Potential of Targeting Tumor Microenvironment in Breast Cancer: Rational Strategies and Recent Progress. J Cell Biochem 2018;119:111-22. [Crossref] [PubMed]
- Paulus P, Stanley ER, Schäfer R, et al. Colony-stimulating factor-1 antibody reverses chemoresistance in human MCF-7 breast cancer xenografts. Cancer Res 2006;66:4349-56. [Crossref] [PubMed]
- Olson OC, Kim H, Quail DF, et al. Tumor-Associated Macrophages Suppress the Cytotoxic Activity of Antimitotic Agents. Cell Rep 2017;19:101-13. [Crossref] [PubMed]
- Yang C, He L, He P, et al. Increased drug resistance in breast cancer by tumor-associated macrophages through IL-10/STAT3/bcl-2 signaling pathway. Med Oncol 2015;32:352. [Crossref] [PubMed]
- Wang M, Herbst RS, Boshoff C. Toward personalized treatment approaches for non-small-cell lung cancer. Nat Med 2021;27:1345-56. [Crossref] [PubMed]
- Bagati A, Kumar S, Jiang P, et al. Integrin αvβ6-TGFβ-SOX4 Pathway Drives Immune Evasion in Triple-Negative Breast Cancer. Cancer Cell 2021;39:54-67.e9. [Crossref] [PubMed]
(English Language Editor: J. Teoh)