Bioinformatics analysis of downstream circRNAs and miRNAs regulated by Runt-related transcription factor 1 in papillary thyroid carcinoma
Introduction
Papillary thyroid carcinoma (PTC) is the most common and least malignant form of thyroid cancer (1). The incidence of PTC has risen rapidly year by year (1). Most PTC patients have a good prognosis; however, the biological characteristics of PTC vary wildly, ranging from non-progressive lesions and refractory lesions to aggressive metastatic cancers (2). Even patients with a low risk of death may have severe or even catastrophic complications, including the invasion of the trachea, esophagus, neck vessels, and recurrent laryngeal nerve (2). Additionally, there is still a lack of effective preoperative judgment strategies for identifying patients with a poor prognosis. Despite an increasing number of observational studies, results on the treatment of PTC are often contradictory, and there is very little randomized controlled trial evidence to support PTC treatment decisions (3). This highlights the current deficiencies in research in terms of both the basic and clinical aspects of PTC.
A previous study has shown that >90% of human genes can be transcribed into ribonucleic acid (RNA), but only 2% are ultimately translated into proteins with biological functions. Most transcribed RNA is non-coding RNA (ncRNA) (4). Circular RNA (circRNA) is a type of closed circular ncRNA molecule that is formed by reverse splicing, which connects the downstream splicing site to the upstream splicing site in the reverse direction (5). As circRNA lacks a 5' cap structure and a 3' end, it is resistant to digestion by ribonuclease, and thus has a long half-life, which is up to 10 times that of linear RNA (5). CircRNA can be divided into the following 3 types based on the sequence types it contains: exon circRNA, intron circRNA, and exon-intron circRNA (5).
At present, the most widely studied circRNA is exon circRNA, which is widely expressed in the cytoplasm. Research has shown that a variety of circRNAs are abnormally expressed in PTC, and these abnormally expressed circRNAs may be used as potential markers for early diagnosis; for example, Du et al. found that circ_0002111 was overexpressed in PTC, and the upregulation of circ_0002111 was related to the tumor-node-metastasis staging and lymph node metastasis of PTC patients (6). Liu et al. found that circ-PRKCI regulated tumor progression and glycolysis by regulating the miR-335/E2F3 axis, thereby creating a carcinogenic effect in PTC (7). Bi et al. found that the overexpression of circRNA_102171 activated the Wnt/β-catenin pathway in a CTNNBIP1-dependent manner to promote PTC progression (8). Such studies suggest that circRNA regulates the malignant biological phenotype of PTC through multiple tumor-related signaling pathways, thereby participating in the occurrence and progression of PTC. Given the importance of circRNAs in the occurrence and development of PTC, it is essential to clarify the expression pattern and mechanism of circRNAs to enable early diagnosis, precisely targeted therapy, and prognosis prediction in PTC.
MicroRNA (miRNA) is a type of RNA molecule, approximately 22 nucleotides in length. Numerous studies have shown that miRNA participates in a variety of tumor biological processes (BPs), including cell proliferation, cell apoptosis, cycle control, cell migration, and invasion (9-12). A previous study has shown that the expression levels of a variety of miRNAs in PTC are significantly dysregulated (13). A Related molecular and cell biology study has shown that abnormally expressed miRNAs affect the critical signaling pathways related to the occurrence and development of tumors (14). Yu et al. found that the expression levels of miR-146b-5p and miR-146b-3p in PTC are elevated and associated with the metastasis of PTC (15). Further mechanism explorations found that miR-146b-5p and miR-146b-3p promote tumor invasion and metastasis by inhibiting the expression of neurofibromatosis type 2 (15). Huang et al. found that miR-222 promotes the invasion and metastasis of PTC by targeting PPP2R2A (16). Pilli et al. found that the combined measurement of the expression of miRNA-95 and miRNA-190 in serum is of great value in the diagnosis of PTC (17). Such findings emphasize the importance of unveiling the expression profile and possible mechanisms of miRNAs in PTC.
Runt-related transcription factor 1 (RUNX1) is an important transcription factor that regulates the differentiation of hematopoietic stem cells into mature blood cells (18). There is increasing evidence that RUNX1 plays a crucial role in solid cancer. Recent research has shown that circRNA plays a regulatory role in the expression of RUNX1 in solid tumors; for example, Hong et al. found that knocking down circ_0000512 in colorectal cancer regulates the expression of RUNX1 by miR-296-5p sponging, thereby inhibiting the proliferation of cells by inducing apoptosis (19). Wang et al. found that circ_0026827 regulates the Beclin1 and RUNX1 signaling pathways by sponging miR-188-3p and promotes osteoblast differentiation (20). Ji et al. found that circFAT1(e2) exerts a tumor suppressor effect by regulating the miR-548g/RUNX1 signal axis in gastric cancer (21). Microarray profiling of circular RNAs and miRNAs has both been reported in PTC. However, it is currently unclear whether RUNX1 as a transcription factor regulates circRNAs and miRNAs downstream.
This study aimed to clarify the expression level of circRNAs and miRNAs in PTC cells after downregulating the expression of RUNX1 via high-throughput sequencing and reveal the possible mechanisms based on a bioinformatics analysis. We present the following article in accordance with the MDAR reporting checklist (available at https://gs.amegroups.com/article/view/10.21037/gs-22-219/rc).
Methods
Clinical samples
PTC tumor tissues and adjacent non-tumor tissues (n=29, 18 females, 11 males, aged 39.2±12.2 years) were acquired from PTC patients at the Zhejiang Provincial People’s Hospital. All the patients signed informed consent forms, and were aged ≥18 and ≤75 years, and did not have any other malignant tumors. The tissues were obtained and stored at −80 ℃ for further experiments. The study was approved by the Ethics Committee of the Zhejiang Provincial People’s Hospital (No. 2022O[041]). The study was conducted in accordance with the Declaration of Helsinki (as revised in 2013).
Data collection, processing, and quality control
The cells were randomly divided into the following 2 groups: the negative control (NC) group (n=3) and Small interfering RNA of RUNX1 (si-RUNX1) group (n=3). The RNA was extracted from the cells with TRIzol reagent. Next, the total RNA was purified with a RNeasy Mini Kit (Qiagen, CA, USA). The ribosomal RNAs were removed by Ribo-Zero rRNA Removal Kits (Illumina, USA) from the total RNAs. The cicrRNAs, miRNAs, and messenger RNAs (mRNAs) were identified using the Illumina platform. The TruSeq Stranded Total RNA Library Prep Kit (Illumina, USA) was used to construct the RNA libraries. The BioAnalyzer 2100 system (Agilent Technologies, USA) was used to evaluate the quality and quantity of the libraries. A total of 10 pmol libraries were denatured to single-stranded deoxyribonucleic acid (DNA) molecules, which were amplified in situ as clusters. The Illumina HiSeq Sequencer was used to sequence 150cycles.
Differential gene analysis
The limma package was used to normalize the experimental data. To confirm there were sufficient genes for the downstream analysis, the threshold for identifying differentially expressed genes (DEGs) was set as a |log2(Foldchange)| >1, and a P value <0.05. To visualize the DEGs, volcano plots and heatmaps were generated using ggplot2 package and heatmap software, respectively.
Cell transfection
For the cell transfection, the TPC-1 cells (a PTC-derived cell line) were cultured in 6-well plates. After reaching 60–70% confluence, miRNA mimics, or corresponding controls, small interfering (si) RNAs and plasmid were transfected using Lipofectamine® 3000. The RUNX1 siRNA and its NC were obtained from the genomeditech Co., Ltd. (Shanghai, China).
Real-time cell proliferation assays
A xCELLigence real time cellular analysis (RTCA DP) multifunctional real-time label-free cell analysis was conducted to detect high-throughput, non-invasive, systemic real-time cell-drug interactions using the Real-time Cell Migration and Cell Invasion Assay (RT-CIM). The cell suspension was prepared as follows: (I) the cells used in the experiment were incubated at 37 ℃ with a CO2 of 5%; (II) the cells were passaged 1 day before the cell experiment; the cell fullness fell between 60–80% for the cell experiment; (III) The cells were removed from the incubator, digested, centrifuged, and prepared. The cell concentration was 5× that required for the experiment (i.e., 104 cells/mL). Next, the cells were added to the Cell Migration and Cell Invasion Assay (CIM) plate as follows: (I) The CIM plate was taken out, the baseline of the RTCA DP was measured, and 100 µL of the well-mixed serum-free cell suspension was added to the upper chamber to ensure the number of cells in each well was 40 k; (II) the 16-well CIM plate was placed on a clean bench and stored at room temperature for 30 min; (III) the 16-well CIM plate was placed on the RTCA Station in the incubator; (IV) the cells were scanned and detected automatically in the system.
RNA isolation and RT-qPCR analysis
The RNA was isolated from the tissue samples and TPC-1 cells using TRIzol® reagent (Invitrogen). PrimeScript RT master mix (Takara Bio Inc.) was used to synthesize the complementary DNA (cDNA). The LightCycler 96 system was used for the qPCR. The cDNA was used as a template for amplification using specific primers. The sequences of the primers used in the qPCR were as follows: glyceraldehyde-3-phosphate dehydrogenase (GAPDH) forward, 5'-GGAGCGAGATCCCTCCAAAAT-3' and reverse, 5'-GGCTGTTGTCATACTTCTCATGG-3'; RUNX1 forward, 5'-GACCCTGCCCATCGCTTTCAAG-3' and reverse, 5'-TCATCATTGCCAGCCATCACAGTG-3'. All the PCR primers were designed and synthesized by Genewiz, Inc. The 2−ΔΔCt method was used to evaluate the data.
Western blotting
Western blotting was conducted in accordance with the manufacturer’s instructions. In brief, total protein was separated by 10% sodium dodecyl-sulfate polyacrylamide gel electrophoresis and then transferred onto a polyvinylidene fluoride membranes. Next, the membranes were incubated with primary antibodies RUNX1 (1:1,000; ab272456; Abcam), and β-actin (1:1,000; ab8226; Abcam) overnight at 4 ℃ and then incubated with the horse radish peroxidase-conjugated secondary antibodies for 1 h at room temperature. Enhanced chemiluminescence (ECL) and the Tanon 4600 imaging system were used to evaluate the proteins.
Migration assays
The TPC-1 cells were placed into the 8.0-µm pore size Transwell chambers for the migration assays. The TPC-1 cells (3×104 cells/well) were seeded in the upper chambers without Matrigel and maintained in serum-free Dulbecco’s Modified Eagle Medium (DMEM). Next, 600 µL of DMEM containing 10% fetal bovine serum, was added to the lower chamber. After incubating for the indicated 48 h, the cells migrated to the lower chamber and were stained with crystal violet. Finally, a light microscope was used to photograph and count the cells in 5 random fields.
CircRNA, miRNA, and mRNA prediction and co-expression network construction
The TargetScan and Circinteractome online databases were used to predict the targets of the circRNAs. The target genes of the miRNAs were predicted using the TargetScan and miRanda databases. Pearson’s correlation coefficients were used to analyze the differential expression of the circRNAs, miRNAs, and mRNAs. The miRNA-mRNA network and the circRNA-miRNA co-expression network were developed based on the results of the differentially expressed circRNAs, miRNAs, and mRNAs. A P value <0.05 for the miRNA-mRNA network and a P value <0.01 for the circRNA-miRNA network were considered significant. A functional enrichment analysis was conducted to identify potential signaling pathways.
Functional enrichment analysis
DEGs were used for the functional enrichment analysis. ClusterProfiler was used to conduct the Gene Ontology (GO) and Kyoto Encyclopedia of Genes and Genomes (KEGG) enrichment analyses. The bar plots and bubble plots were visualized using the GO terms, and were classified into 3 categories: BPs, cellular components (CCs), and molecular functions (MFs). GO terms (top 10 of each category) and KEGG pathways were visualized with bar plots and bubble plots, respectively.
Statistical analysis
SPSS 25.0 was selected to perform the statistical analysis. The statistical data was displayed as the mean ± SD. Student’s t-test was used for the difference analysis in the two groups and one way ANOVA followed Tukey’s test were used for the difference analysis in multiple groups. P<0.05 was considered as significant. All experiments were repeated at least three times.
Results
RUNX1 is abnormally expressed in PTC
First, we evaluated the expression of RUNX1 in PTC tissues and adjacent normal tissues, and found that RUNX1 was more upregulated in primary tumor tissues than normal tissues (see Figure 1A). The RUNX1 expression in the PTC tissues in the N0 subset was lower than that in the N1 subset (see Figure 1B). Additionally, the level of the N1a subset was notably lower than that of the N1b subset (see Figure 1C). The RUNX1 expression was also downregulated in the PTC tissues at T1 + T2 subset vs. T3 + T4 subset and non-extrathyroidal extension (non-ETE) subset vs. ETE subset, respectively, but the RUNX1 expression was no significance in the PTC tissues at I + II subset vs. III + V subset (see Figure 1D-1F). Additionally, the expression level of RUNX1 was lower in the minimal ETE tissues than in extensive ETE tissues (see Figure 1G). The expression levels of RUNX1 in the unifocal and multifocal PTC tissues were also evaluated, and the level in the unifocal PTC tissues was lower than that in the multifocal PTC tissues (see Figure 1H). Notably, RUNX1 expression was no significance in the male groups vs. female groups, respectively (see Figure 1I).
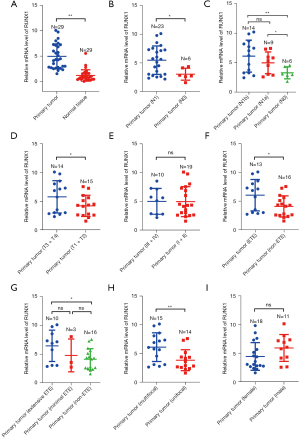
Knockdown of RUNX1 inhibits cell migration and invasion
To further examine the functional role of RUNX1 in PTC progression, a number of functional experiments were conducted. First, we suppressed the expression of RUNX1 via RNA knockdown (see Figure 2A,2B). The results of the real-time cell proliferation assay showed that the downregulation of RUNX1 significantly suppressed tumor proliferation (see Figure 2C). We further validated our results through transwell assays. The results revealed that the downregulation of RUNX1 significantly suppressed the tumor migration and invasion (see Figure 2D,2E). Thus, the downregulation of RUNX1 was able to suppress cell migration and invasion in PTC.
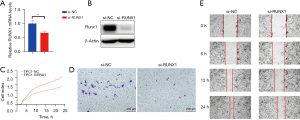
The differential expression and differential analysis of circRNAs
The differential expression of the circRNAs after RUNX1 knockdown was analyzed via high-throughput sequencing, and a total of 10,782 circRNAs were detected. According to the difference and significance level (i.e., a |log2(Foldchange)| >1 and a P value <0.001), a total of 29 differentially expressed circRNAs were identified between the 2 samples, of which 11 were upregulated and 18 were downregulated (see Figure 3A-3C).
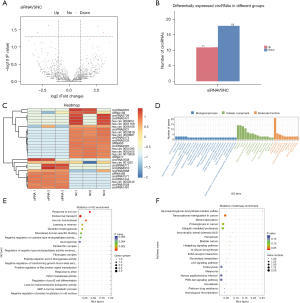
Subsequently, a GO function annotation was performed for the functional analysis of the differentially expressed circRNA target genes. The GO analysis revealed 147 BPs (P<0.05), 27 CCs (P<0.05), and 46 MFs (P<0.05). Based on the 3 GO_function annotations, the number (S gene number) was arranged in descending order from large to small, and the Top 25, Top 15, and Top 10 GO_Terms were selected for graphical display (see Figure 3D). We then drew bubble diagrams for the top 20 signaling pathways with P values <0.05. The results showed that the iron channel-related pathways, endosomal transport, learning and memory pathways had the largest number of differential genes, and the greatest level of difference changes (see Figure 3E).
We also performed a KEGG analysis on the circRNA target genes to lay the foundation for subsequent experiments. The results showed that the circRNA target genes were enriched in a total of 44 signaling pathways, of which 2 pathways (i.e., the glycosaminoglycan synthesis and transcription disorder pathways) had a P value <0.05, and thus met the significant threshold requirements (see Figure 3F).
The differential expression and differential analysis of miRNAs
The differential expression of the miRNAs after RUNX1 knockdown was analyzed via high-throughput sequencing. The differential expression between the 2 samples was selected based on the 2 thresholds for the difference multiple and significance levels (i.e., a |log2(Foldchange)| >1, and a P value <0.1). There were a total of 130 miRNAs, of which 49 were upregulated and 81 were downregulated. Based on the 2 thresholds for the difference multiple and significance levels (i.e., a |log2(Foldchange)| >1 and a P value <0.05), the difference between the 2 samples were selected. There were a total of 70 differentially expressed miRNAs, of which 26 were upregulated and 44 were downregulated; the 2 samples were selected according to the 2 thresholds for difference multiple and significance levels (i.e., a |log2(Foldchange)| >1 and a P value <0.01) There was a total of 20 differentially expressed miRNAs, of which 8 were upregulated and 12 were downregulated (see Figure 4A-4C).
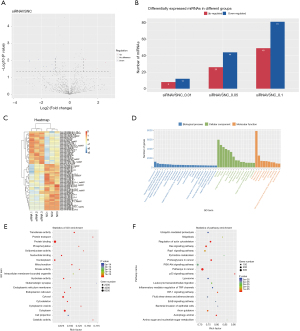
Among all the selected miRNA target genes, we divided the GO annotations corresponding to these genes into 3 categories based on MF, BP, and CC, and the divided the GO functions in each category according to the number of target genes annotated and sorted them from high to low and generates a map. The abscissa is the classification of GO, and the ordinate is the percentage of the target genes. The results revealed 108 BPs (P<0.05), 29 CCs (P<0.05), and 43 MFs (P<0.05). For the 3 GO_functions, we annotated the different GO_Term genes. The numbers (S gene numbers) were arranged in descending order from large to small. The Top 25, Top 15, and Top 10 GO_Terms were selected for graphical display (see Figure 4D). We also drew bubble charts for the top 20 signaling pathways with P values <0.05, and the results showed that the protein binding and cytoplasm pathways had the largest number of differential genes, and the greatest level of difference (see Figure 4E).
Based on the KEGG biological pathway database, we performed a KEGG analysis on the miRNA target genes to lay the foundation for subsequent experiments. The results showed that the miRNA target genes were enriched in multiple signaling pathways, of which 28 pathways had a P value <0.05, and thus met the significant threshold requirements. The notable pathways included the tumor-related pathways, the phosphatidylinositol-3-kinase and protein kinase B pathway, and the glycoprotein, cytoskeleton pathways, such as Ras and Rap1 (see Figure 4F).
The differential expression and differential analysis of the mRNAs
The differential expression of the mRNAs after RUNX1 knockdown was analyzed via high-throughput sequencing. According to the 2 thresholds for difference multiple and significance levels (i.e., a |log2(Foldchange)| >1 and a P value <0.05), a total of 201 mRNAs were differentially expressed, of which 109 were upregulated and 92 were downregulated (see Figure 5A-5C).
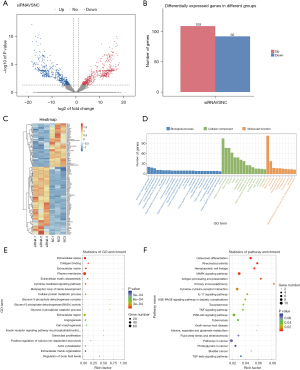
Among all the selected mRNA genes, the GO annotations corresponding to the genes were divided into 3 categories according to MF, BP and CC, and the GO functions in each category were divided according to the number of target genes, annotated and sorted from high to low and a map was generated. The results revealed 280 BPs (P<0.05), 9 CCs (P<0.0), and 9 MFs (P<0.05). For the 3 GO_functions, we annotated the different GO_Term genes. The numbers (S gene numbers) were arranged in descending order from large to small. The Top 25, Top 15, and Top 10 GO_Terms were selected for graphical display (see Figure 5D). We also drew bubble charts for the top 20 signaling pathways with P values <0.05, and the results showed that the number of differential genes in the plasma membrane was the largest, and the level of difference changed the most (see Figure 5E).
Based on the KEGG biological pathway database, we performed a KEGG analysis on the mRNA genes to lay the foundation for subsequent experiments. The results showed that mRNA genes were enriched in multiple signaling pathways, of which a total of 20 pathways had P values <0.05, and thus met the significant threshold requirements. The notable pathways included the osteoclast differentiation, rheumatoid arthritis, hematopoietic cell lineage, and mitogen-activated protein kinase signaling pathways (see Figure 5F).
Construction of a circRNA-miRNA-mRNA ceRNA network
Based on the correlation analysis between the DEGs, we then constructed a competing endogenous RNA (ceRNA) network and conducted a functional enrichment analysis to examine the ceRNA mechanism. The KEGG enrichment results showed that the number of differential genes in tissue homeostasis, postsynaptic density, the positive regulation of endocytosis, the regulation of interferon-beta production, and the establishment of planar polarity was the largest in the ceRNA pathways (see Figure 6A). The KEGG enrichment results showed that the number of differential genes in ubiquitin-mediated proteolysis, Toll-like receptor pathway, tumor necrosis factor pathway, and C-type lectin reception pathway was the largest in the ceRNA pathways (see Figure 6B).
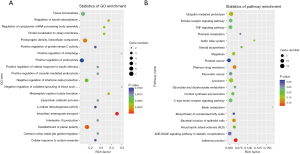
Discussion
PTC is a common but the least malignant form of thyroid cancer. PTC patients have a good prognosis; however, the biological characteristics of PTC vary wildly (2). Pathological factors such as hormones, genetics and environment influence the development of PTC. The molecular mechanisms contribute to the PTC progression are complicated. Numerous genetic alterations that playa fundamental role in the tumorigenesis of PTC have been reported, such as BRAF, RET/PTC, RAS, TRK.
RUNX1 is a critical transcription factor. Research has shown that RUNX1 regulates the differentiation of hematopoietic stem cells into mature blood cells (18). There is increasing evidence that RUNX1 plays a crucial role in solid cancer (19). Recently, research has shown that circRNA plays a regulatory role in the expression of RUNX1 in solid tumors (20-22). However, the downstream ncRNAs of RUNX1 are currently unclear.
In this study, we showed that the knockdown of RUNX1 inhibited cell migration and invasion. We also explored changes in the expression of circRNAs and miRNAs in PTC cells after RUNX1 knockdown via high-throughput sequencing. The results showed that 29 circRNAs were differentially expressed, of which 11 were upregulated and 18 were downregulated. A total of 20 miRNAs were the most significantly differentially expressed, of which, 8 were upregulated and 12 were downregulated. This study innovatively used high-throughput sequencing to explore the regulation of RUNX1 as a transcription factor for downstream circRNAs and miRNAs, and thus provided further evidence of the biological effects of RUNX1 in PTC.
After analyzing the differential genes, we further explored the possible biological functions of the differentially expressed circRNAs and miRNAs in PTC via the enrichment of the GO and KEGG pathways. The GO analysis revealed that the dominantly differential genes were associated with iron channel-related pathways. The KEGG analysis revealed that the most significantly altered genes were associated with glycosaminoglycan synthesis. It has been reported that pathways related to iron metabolism play a vital role in the occurrence and development of various tumors (23). In general, transferrin receptor 1 (TFR1) is essential for regulating iron uptake and cell growth (24). Research has shown that compared to normal cells, the expression of TFR1 in cancer cells is increased (25). Further, TFR1 promotes the proliferation, migration, invasion, and metastasis of cancer cells and is regarded as a potential tumor treatment target (25). Cui et al. found that TfR1 expression in CRC tissues was obviously higher in CRC patients with no lymph node metastasis (no lymph node metastasis vs. lymph node metastasis: 69.8% vs. 47.8%, P=0.002) (26). Additionally, patients with positively expressed TFR1 have a higher survival rate than those with negatively expressed TFR1 (26). A previous study has found that TFR1 is highly expressed in colorectal cancer; however, research has shown that the downregulation of TFR1 promotes cell migration and invasion through the JAK/STAT pathway (26). TFR1 is also highly expressed in pancreatic ductal adenocarcinoma. Jeong reported that TFR1 promotes the mitochondrial respiration and ROS production of human pancreatic cancer cells, which is essential to their tumorigenic growth and survival (27).
The expression of TFR1 also determines the sensitivity of cells to oxidative stress. Iron reductases, especially members of the six-transmembrane epithelial antigen of prostate (STEAP) family, are essential for the absorption and reduction of iron in the endosome. Additionally, the expression of STEAP 1–4 in different types of tumors (e.g., prostate cancer, breast cancer, pancreatic cancer, and glioma) has changed (28-31). STEAP2 drives prostate cancer development by promoting proliferation and metastasis, and modifying the transcription profiles of specific genes (28). Ferritin plays a central role in iron storage (30). In some cancers, ferritin concentration in plasma is very high, which is related to an advanced tumor stage and poor prognosis. Thus, elevated ferritin levels are manifested in inflammatory diseases and cancers (e.g., pancreatic cancer, and colorectal cancer), indicating that ferritin may be the basis for the development of cancer (32).
Iron flux is regulated by the iron pump and its regulator. Additionally, hepcidin might be involved in tumorigenesis (33). The reduction of iron transporter expression on the cell surface is associated with an aggressive phenotype in prostate and breast cancer (31,34). A previous study has also shown that iron homeostasis also plays a vital role in the development of PTC. Zhou et al. found that E4BP4 promotes the proliferation of PTC by regulating iron homeostasis (35). Many previous bioanalytical studies have found that the pathways related to glycosaminoglycan synthesis play a critical role in the occurrence and development of PTC (36-38). Consistent with previous studies, our KEGG analysis determined the largest number of differential genes, such as glycosaminoglycan synthesis pathways, and the difference level with the greatest change. This suggests that the pathways related to glycosaminoglycan synthesis may also be potential targets for PTC treatment.
It may be more meaningful to add functional research on key circRNAs and miRNAs which is a limitation of this study. But in the present study, we just focused on the bioinformatics analysis based on the public database and tried to find out the key molecules that may involve in the PTC progression. Functional experiments will be carried out in our further study.
Conclusions
In summary, we analyzed the effect of knocking down RUNX1 on the levels of circRNA and miRNA in PTC via high-throughput sequencing. We successfully identified the dominantly differential genes that were associated with iron channel-related pathways and glycosaminoglycan synthesis via GO and KEGG enrichment analyses. These key circRNAs or miRNAs combined with RUNX1 may be potential prognostic markers of PTC. In addition, these molecules are also potential targets for the biotherapy. We will carry on the studying the roles of these key biomarkers in the PTC progression.
Acknowledgments
We are thankful for the support of the Zhejiang Provincial Program, which cultivates high-level innovative health talents.
Funding: This study received funding from the Natural Science Foundation of Zhejiang Province (No. LY21H160049), the Medical Science and Technology Project of Zhejiang Province (No. 2021KY482, No. 2020KY008, No. 2020KY030, and No. 2019ZD024), and the Key Research Projects of Traditional Chinese Medicine of Zhejiang Province (No. 2019ZZ004).
Footnote
Reporting Checklist: The authors have completed the MDAR reporting checklist. Available at https://gs.amegroups.com/article/view/10.21037/gs-22-219/rc
Data Sharing Statement: Available at https://gs.amegroups.com/article/view/10.21037/gs-22-219/dss
Conflicts of Interest: All authors have completed the ICMJE uniform disclosure form (available at https://gs.amegroups.com/article/view/10.21037/gs-22-219/coif). The authors have no conflicts of interest to declare.
Ethical Statement: The authors are accountable for all aspects of the work in ensuring that questions related to the accuracy or integrity of any part of the work are appropriately investigated and resolved. The study was conducted in accordance with the Declaration of Helsinki (as revised in 2013), and was approved by the Ethics Committee of the Zhejiang Provincial People’s Hospital (No. 2022O[041]). All the patients signed informed consent forms.
Open Access Statement: This is an Open Access article distributed in accordance with the Creative Commons Attribution-NonCommercial-NoDerivs 4.0 International License (CC BY-NC-ND 4.0), which permits the non-commercial replication and distribution of the article with the strict proviso that no changes or edits are made and the original work is properly cited (including links to both the formal publication through the relevant DOI and the license). See: https://creativecommons.org/licenses/by-nc-nd/4.0/.
References
- Siegel RL, Miller KD, Jemal A. Cancer statistics, 2020. CA Cancer J Clin 2020;70:7-30. [Crossref] [PubMed]
- Cabanillas ME, McFadden DG, Durante C. Thyroid cancer. Lancet 2016;388:2783-95. [Crossref] [PubMed]
- Fagin JA, Wells SA Jr. Biologic and Clinical Perspectives on Thyroid Cancer. N Engl J Med 2016;375:1054-67. [Crossref] [PubMed]
- Wong CM, Tsang FH, Ng IO. Non-coding RNAs in hepatocellular carcinoma: molecular functions and pathological implications. Nat Rev Gastroenterol Hepatol 2018;15:137-51. [Crossref] [PubMed]
- Kristensen LS, Andersen MS, Stagsted LVW, et al. The biogenesis, biology and characterization of circular RNAs. Nat Rev Genet 2019;20:675-91. [Crossref] [PubMed]
- Du G, Ma R, Li H, et al. Increased Expression of hsa_circ_0002111 and Its Clinical Significance in Papillary Thyroid Cancer. Front Oncol 2021;11:644011. [Crossref] [PubMed]
- Liu Y, Chen G, Wang B, et al. Silencing circRNA protein kinase C iota (circ-PRKCI) suppresses cell progression and glycolysis of human papillary thyroid cancer through circ-PRKCI/miR-335/E2F3 ceRNA axis. Endocr J 2021;68:713-27. [Crossref] [PubMed]
- Bi W, Huang J, Nie C, et al. CircRNA circRNA_102171 promotes papillary thyroid cancer progression through modulating CTNNBIP1-dependent activation of β-catenin pathway. J Exp Clin Cancer Res 2018;37:275. [Crossref] [PubMed]
- Shi L, Zhu H, Shen Y, et al. Regulation of E2F Transcription Factor 3 by microRNA-152 Modulates Gastric Cancer Invasion and Metastasis. Cancer Manag Res 2020;12:1187-97. [Crossref] [PubMed]
- Zhao P, Ma YG, Zhao Y, et al. MicroRNA-552 deficiency mediates 5-fluorouracil resistance by targeting SMAD2 signaling in DNA-mismatch-repair-deficient colorectal cancer. Cancer Chemother Pharmacol 2019;84:427-39. [Crossref] [PubMed]
- Jahangiri Moez M, Bjeije H, Soltani BM. Hsa-miR-5195-3P induces downregulation of TGFβR1, TGFβR2, SMAD3 and SMAD4 supporting its tumor suppressive activity in HCT116 cells. Int J Biochem Cell Biol 2019;109:1-7. [Crossref] [PubMed]
- Xiao S, Zhu H, Luo J, et al. miR-425-5p is associated with poor prognosis in patients with breast cancer and promotes cancer cell progression by targeting PTEN. Oncol Rep 2019;42:2550-60. [Crossref] [PubMed]
- Zhu G, Xie L, Miller D. Expression of MicroRNAs in Thyroid Carcinoma. Methods Mol Biol. 2017;1617:261-280. [Crossref] [PubMed]
- Gebert LFR, MacRae IJ. Regulation of microRNA function in animals. Nat Rev Mol Cell Biol 2019;20:21-37. [Crossref] [PubMed]
- Yu C, Zhang L, Luo D, et al. MicroRNA-146b-3p Promotes Cell Metastasis by Directly Targeting NF2 in Human Papillary Thyroid Cancer. Thyroid 2018;28:1627-41. [Crossref] [PubMed]
- Huang Y, Yu S, Cao S, et al. MicroRNA-222 Promotes Invasion and Metastasis of Papillary Thyroid Cancer Through Targeting Protein Phosphatase 2 Regulatory Subunit B Alpha Expression. Thyroid 2018;28:1162-73. [Crossref] [PubMed]
- Pilli T, Cantara S, Marzocchi C, et al. Diagnostic Value of Circulating microRNA-95 and -190 in the Differential Diagnosis of Thyroid Nodules: A Validation Study in 1000 Consecutive Patients. Thyroid 2017;27:1053-7. [Crossref] [PubMed]
- Sood R, Kamikubo Y, Liu P. Role of RUNX1 in hematological malignancies. Blood 2017;129:2070-82. Erratum in: Blood 2018;131:373. [Crossref] [PubMed]
- Hong D, Fritz AJ, Gordon JA, et al. RUNX1-dependent mechanisms in biological control and dysregulation in cancer. J Cell Physiol 2019;234:8597-609. [Crossref] [PubMed]
- Wang L, Wu H, Chu F, et al. Knockdown of circ_0000512 Inhibits Cell Proliferation and Promotes Apoptosis in Colorectal Cancer by Regulating miR-296-5p/RUNX1 Axis. Onco Targets Ther 2020;13:7357-68. [Crossref] [PubMed]
- Ji F, Zhu L, Pan J, et al. hsa_circ_0026827 Promotes Osteoblast Differentiation of Human Dental Pulp Stem Cells Through the Beclin1 and RUNX1 Signaling Pathways by Sponging miR-188-3p. Front Cell Dev Biol 2020;8:470. [Crossref] [PubMed]
- Fang J, Hong H, Xue X, et al. A novel circular RNA, circFAT1(e2), inhibits gastric cancer progression by targeting miR-548g in the cytoplasm and interacting with YBX1 in the nucleus. Cancer Lett 2019;442:222-32. [Crossref] [PubMed]
- Ludwig H, Evstatiev R, Kornek G, et al. Iron metabolism and iron supplementation in cancer patients. Wien Klin Wochenschr 2015;127:907-19. [Crossref] [PubMed]
- Bystrom LM, Rivella S. Cancer cells with irons in the fire. Free Radic Biol Med 2015;79:337-42. [Crossref] [PubMed]
- Zhou L, Zhao B, Zhang L, et al. Alterations in Cellular Iron Metabolism Provide More Therapeutic Opportunities for Cancer. Int J Mol Sci 2018;19:1545. [Crossref] [PubMed]
- Cui C, Cheng X, Yan L, et al. Downregulation of TfR1 promotes progression of colorectal cancer via the JAK/STAT pathway. Cancer Manag Res 2019;11:6323-41. [Crossref] [PubMed]
- Jeong SM, Hwang S, Seong RH. Transferrin receptor regulates pancreatic cancer growth by modulating mitochondrial respiration and ROS generation. Biochem Biophys Res Commun 2016;471:373-9. [Crossref] [PubMed]
- Whiteland H, Spencer-Harty S, Morgan C, et al. A role for STEAP2 in prostate cancer progression. Clin Exp Metastasis 2014;31:909-20. [Crossref] [PubMed]
- Han M, Xu R, Wang S, et al. Six-Transmembrane Epithelial Antigen of Prostate 3 Predicts Poor Prognosis and Promotes Glioblastoma Growth and Invasion. Neoplasia 2018;20:543-54. [Crossref] [PubMed]
- Wang SL, Cao S, Wu R, et al. Serum ferritin predicted prognosis in patients with locally advanced pancreatic cancer. Future Oncol 2015;11:2905-10. [Crossref] [PubMed]
- Shan Z, Wei Z, Shaikh ZA. Suppression of ferroportin expression by cadmium stimulates proliferation, EMT, and migration in triple-negative breast cancer cells. Toxicol Appl Pharmacol 2018;356:36-43. [Crossref] [PubMed]
- Wang W, Knovich MA, Coffman LG, et al. Serum ferritin: Past, present and future. Biochim Biophys Acta 2010;1800:760-9. [Crossref] [PubMed]
- Brookes MJ, Hughes S, Turner FE, et al. Modulation of iron transport proteins in human colorectal carcinogenesis. Gut 2006;55:1449-60. [Crossref] [PubMed]
- Xue D, Zhou CX, Shi YB, et al. Decreased expression of ferroportin in prostate cancer. Oncol Lett 2015;10:913-6. [Crossref] [PubMed]
- Zhou Q, Chen J, Feng J, et al. E4BP4 promotes thyroid cancer proliferation by modulating iron homeostasis through repression of hepcidin. Cell Death Dis 2018;9:987. [Crossref] [PubMed]
- Zhao H, Li H. Network-based meta-analysis in the identification of biomarkers for papillary thyroid cancer. Gene 2018;661:160-8. [Crossref] [PubMed]
- Liang W, Sun F. Identification of key genes of papillary thyroid cancer using integrated bioinformatics analysis. J Endocrinol Invest 2018;41:1237-45. [Crossref] [PubMed]
- Yusof AM, Tieng FYF, Muhammad R, et al. In-depth characterization of miRNome in papillary thyroid cancer with BRAF V600E mutation. Prog Microbes Mol Biol 2020;3:a0000043.
(English Language Editor: L. Huleatt)