Salivary biomarkers-assisted ultrasound-based differentiation of malignant and benign thyroid nodules
Introduction
The incidence of papillary thyroid cancer (PTC) has gradually been increasing over the past decades and it is currently the 6th leading cancer among the female population (1). In China, PTC is the most prevalent type of cancer among females under the age of 30 (2). The majority of thyroid nodules are benign, while only 5% are cancerous (2). Although PTC is less aggressive compared to other cancers, some patients are still at risk of metastasis and dissemination (3). In clinical practice, total thyroidectomy is the standard treatment method; however, PTC with metastases often requires an extended surgical resection. Therefore, early identification, diagnosis, and treatment are critical.
Ultrasonography (US) is currently the most common method of examining the properties of thyroid nodules (4). In 2009, Park et al. (5) established a thyroid imaging reporting and data system (TI-RADS) criteria, and based on ultrasound features, they developed 6 classifications to distinguish between thyroid benign and malignant nodules. Despite TI-RADS achieving significant clinical value, it has inherent shortcomings including experience dependence, poor reproducibility, and high heterogeneity among sonographers (6), specifically in the differentiation of borderline tumors. Based on previous reports, the TI-RADS 4B nodule has a 10–50% probability of malignancy (7). These patients often require invasive fine-needle aspiration biopsy, which markedly increases their burden and suffering. Besides, biopsy might also yield non-diagnostic or false-negative results (8). Therefore, more convenient tests are necessary to help in diagnosis and differentiation.
Among the current techniques, tumor markers are frequently used as adjuncts in the screening and diagnosis of malignant tumors including pancreatic cancer, lung cancer, liver cancer, and so on (9,10). Nonetheless, due to the low malignancy, serum biomarkers of thyroid cancers and their diagnostic value are usually restricted. Increasing recent studies have shown that salivary biomarkers are substantially increased in many cancers including breast cancer, pancreatic cancer, and parotid tumors, indicating their significant diagnostic value (11). Among these, certain biomarkers even exhibit higher expression levels in saliva, thereby making them more advantageous in examining the severity and outcomes of tumors (12). This research sought to evaluate the predictive value of salivary biomarkers and develop a diagnostic model combining with US to distinguish benign from malignant thyroid tumors. We present the following article in accordance with the STARD reporting checklist (available at https://gs.amegroups.com/article/view/10.21037/gs-21-864/rc).
Methods
Patients
Between July and December 2020, we enrolled 86 patients with thyroid nodules from Ruijin Rehabilitation Hospital. The experimental protocol was approved by the Shanghai Ruijin Rehabilitation Hospital Ethics Committee. Informed consent was waived for this non-interventional, observational study by the Ethics Committees, in which the patient data used were kept strictly confidential. The study was conducted in accordance with the Declaration of Helsinki (as revised in 2013). Among them, 42 individuals had benign thyroid tumors (BTT), whereas 44 patients had PTC. After hospitalization, the patients underwent thyroidectomy and were diagnosed through postoperative pathology. Either BTT or PTC was confirmed through post-operative pathological diagnosis by a team of specialists. All participants had salivary and serological assays comprising tumor markers and thyroid hormones. The inclusion criteria were as follows: (I) patients underwent thyroidectomy and were confirmed through postoperative pathological diagnosis; (II) complete clinical information; (III) the diagnosis of malignant cases restricted to PTC and benign tumors included thyroid adenoma and thyroid cyst. The exclusion criteria were as follows: (I) co-morbidity with other malignancy; (II) pregnant women or minors under 18 years old; (III) diagnosed with chronic thyroid inflammation or other thyroid disorder.
Data collection
The clinical characteristics of the participants included age, gender, body mass index (BMI), serum and salivary tumor markers, serum and salivary thyroid hormones, US reports, and so on. Tumor markers included carcinoembryonic antigen (CEA), alpha-fetoprotein (AFP), neuron-specific enolase (NSE), carbohydrate antigen 125 (CA125), carbohydrate antigen 153 (CA153), carbohydrate antigen 19-9 (CA199), carbohydrate antigen 242 (CA242), carbohydrate antigen 724 (CA724), squamous cell carcinoma antigen (SCC), and calcitonin (CT). Thyroid hormones included triiodothyronine (T3), thyroxine (T4), free-triiodothyronine (FT3), free-thyroxine (FT4), thyroid-stimulating hormone (TSH), thyroglobulin (TG), anti-thyroglobulin antibody (A-TG), and thyroid peroxidase antibody (A-TPO). None of those salivary biomarkers were secreted by salivary glands, but derived from serum after absorption and concentration.
The patient diagnosis was performed through surgical resection and postoperative pathological analysis. Thyroid US was executed by 2 experienced sonographers and was scored using TI-RADS criteria. After admission, venous blood samples were drawn from the antecubital vein. The passive drool technique was used to collect saliva samples after cleaning of the oral cavity in the morning after fasting (13); notably, approximately 5 mL of non-stimulated saliva was collected during this step. For the detection of biomarkers, the specimens were examined using the chemiluminescence method (i2000 chemiluminescence immunoassay analyzer; Abbott, Chicago, IL, USA). Also, salivary biomarkers were determined using serum marker reagents (14).
Research process
First, serum and salivary tumor markers, as well as thyroid hormone levels were measured and compared between the BTT and PTC groups. Subsequently, the risk factors for thyroid malignant tumors were determined using logistic regression analysis. Then, the receiver operating characteristic (ROC) curve was used to establish the cut-off values for these risk factors to differentiate between benign and malignant thyroid tumors. Also, sensitivity, specificity, positive predictive value (PPV), negative predictive value (NPV), area under the curve (AUC), and Youden index were determined. Thereafter, the diagnostic value of US was evaluated for each TI-RADS grade. The PTC grade with the lowest prediction accuracy was selected to develop a salivary biomarker diagnostic model using K-nearest neighbor (KNN) and support vector machine (SVM) machine learning algorithms. In the ultimate diagnostic models, patients were classified first by the US, then the borderline cases between benign and malignant were further confirmed using a salivary biomarker diagnostic model.
Statistical analysis
The R language (4.0.2; https://cran.r-project.org/bin/windows/base/old/4.0.2/) was employed for data analysis and graphing. Data were presented by mean ± standard deviation (SD). The Mann-Whitney U test was performed for continuous variables. The chi-square test was used to establish the differences between subgroups. P<0.05 indicated a statistically significant difference. Further, the ROC curve, AUC, and Youden index were used to determine the predictive value of biomarkers. Machine learning algorithms were conducted to construct the multi-biomarkers-based diagnostic model, including KNN and SVM.
Results
Comparison of biomarker distributions in serum and saliva between PTC and BTT
A total of 86 patients were enrolled, among them, 44 had PTC, while 42 had BTT (Figure 1). Initially, the gender and age distributions of the 2 participant groups were evaluated, as a result, no statistically significant difference was found. Subsequently, the serum and saliva biomarkers were analyzed in the PTC and BTT groups, where tumor markers including CEA, AFP, NSE, CA125, CA153, CA199, CA242, CA724, SCC, and CT, and thyroid hormones including T3, T4, FT3, FT4, TSH, TG, A-TG, and A-TPO were identified. Among tumor indicators, serum AFP distribution was significantly different between benign and malignant thyroid tumors (P<0.05), whereas the distribution of other tumor markers was not statistically different both in saliva and serum. As for thyroid hormones, salivary T3, FT3, and FT4 had a considerably larger distribution in BTT than that in PTC (P<0.05). Additionally, BTT exhibited a higher level of T4 than PTC and the difference was statistically insignificant (P=0.053). In serum thyroid hormone, only TG revealed a considerably higher expression in the BTT group than that in the PTC group (P=0.008). The results are shown in Table 1.
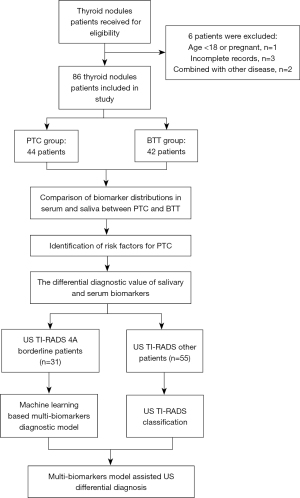
Table 1
Variables | Serum biomarkers | Salivary biomarkers | |||||
---|---|---|---|---|---|---|---|
PTC (n=44) | BTT (n=42) | P value | PTC (n=44) | BTT (n=42) | P value | ||
Gender | 0.307 | – | |||||
Male | 8 | 12 | – | – | |||
Female | 35 | 31 | – | – | |||
Age, years | 43.19±13.73 | 44.30±14.75 | 0.717 | – | – | – | |
CEA | 24.13±98.89 | 7.55±18.09 | 0.499 | 62.44±117.74 | 54.23±76.91 | 0.840 | |
AFP | 0.85±0.51 | 1.05±0.70 | 0.075 | 0.85±0.51 | 1.05±0.70 | 0.214 | |
NSE | 3.89±0.64 | 4.58±0.51 | 0.106 | 0.58±0.30 | 0.54±0.15 | 1.000 | |
CA125 | 14.2±5.97 | 16.09±8.32 | 1.000 | 128.89±218.99 | 162.18±265.91 | 0.171 | |
CA153 | 9.12±7.54 | 6.78±5.19 | 0.439 | 4.88±5.87 | 9.67±17.55 | 0.430 | |
CA199 | 10.89±7.93 | 8.96±4.96 | 0.535 | 389.69±380.96 | 455.32±379.99 | 0.227 | |
CA242 | 14.39±10.84 | 11.98±15.83 | 0.148 | 152.61±69.67 | 181.63±46.72 | 0.025 | |
CA724 | 2.04±1.78 | 2.05±2.01 | 0.950 | 18.02±35.91 | 21.74±31.7 | 0.081 | |
SCC | 0.84±0.71 | 2.18±2.16 | 0.503 | 69.48±36.45 | 72.35±37.21 | 0.719 | |
CT | 4.32±2.1 | 4.60±2.59 | 0.828 | 40.51±62.21 | 40.3±53.84 | 0.843 | |
T3 | 1.84±0.46 | 1.80±0.35 | 0.937 | 1.68±0.31 | 1.91±0.38 | 0.045* | |
T4 | 108.47±25.26 | 110.30±21.49 | 0.519 | 6.62±0.91 | 7.41±1.59 | 0.089 | |
FT3 | 4.93±1.24 | 4.87±0.70 | 0.623 | 1.98±0.32 | 2.29±0.51 | 0.027* | |
FT4 | 17.57±3.46 | 18.00±2.69 | 0.464 | 0.82±0.41 | 1.12±0.49 | 0.016* | |
TSH | 1.92±1.15 | 1.64±1.16 | 0.168 | 0.01±0.01 | 0.01±0.01 | 0.111 | |
TG | 81.45±149.50 | 189.31±210.37 | 0.008* | 0.05±0.02 | 0.15±0.35 | 0.809 | |
A-TG | 252.09±725.63 | 88.24±322.94 | 0.923 | 10.50±1.67 | 11.03±2.45 | 0.420 | |
A-TPO | 35.52±59.77 | 34.56±100.08 | 0.687 | 7.68±6.82 | 13.75±18.54 | 0.260 |
*, P<0.05. PTC, papillary thyroid carcinoma; BTT, benign thyroid tumor; CEA, carcinoembryonic antigen; AFP, alpha fetoprotein; NSE, neuron-specific enolase; CA125, carbohydrate antigen 125; CA153, carbohydrate antigen 153; CA199, carbohydrate antigen 19-9; CA242, carbohydrate antigen 242; CA724, carbohydrate antigen 724; SCC, squamous cell carcinoma antigen; CT, calcitonin; T3, triiodothyronine; T4, thyroxine; FT3, free-triiodothyronine; FT4, free-thyroxine; TSH, thyroid-stimulating hormone; TG, thyroglobulin; A-TG, anti-thyroglobulin antibody; A-TPO, thyroid peroxidase antibody.
Regression analysis of risk factors for benign and malignant thyroid tumors
The risk factors for distinguishing benign and malignant thyroid nodules were examined in this section. First, univariate regression analysis was performed including age, gender, US TI-RADS classification, and several biomarkers with significant differences including salivary T3, salivary FT3, salivary FT4, and serum TG. Consequently, data indicated that lower levels of salivary FT3, salivary FT4, serum TG, and higher US grades were associated with an increased risk of malignancy (P<0.05) (Table 2). In the multivariate logistic regression analysis, salivary FT3 and the US grade were independent risk factors of PTC (P<0.05). The salivary FT3 was negatively associated with the increased risk of PTC, whereas US grades were positively associated, which was broadly consistent with the trends presented above (Table 3).
Table 2
Factors | Regression coefficient | OR | 95% CI | P value |
---|---|---|---|---|
Age | −0.012 | 0.989 | 0.945–1.034 | 0.616 |
Gender | −1.478 | 0.228 | 0.052–1.007 | 0.051 |
Salivary T3 | −1.949 | 0.142 | 0.020–1.013 | 0.052 |
Salivary FT3 | −2.140 | 0.118 | 0.017–0.824 | 0.031 |
Salivary FT4 | −1.588 | 0.204 | 0.043–0.981 | 0.047 |
Serum TG | −0.006 | 0.994 | 0.989–0.999 | 0.027 |
US | 2.266 | 9.643 | 2.362–39.365 | 0.002 |
OR, odds ratio; CI, confidence interval; US, ultrasound; T3, triiodothyronine; FT3, free-triiodothyronine; FT4, free-thyroxine; TG, thyroglobulin.
Table 3
Factors | Regression coefficient | OR | 95% CI | P value |
---|---|---|---|---|
Salivary FT3 | –2.372 | 0.093 | 0.011–0.828 | 0.033 |
US | 3.036 | 20.815 | 3.369–128.620 | 0.001 |
OR, odds ratio; CI, confidence interval; US, ultrasound; FT3, free-triiodothyronine.
The differential diagnostic value of salivary and serum biomarkers
The ROC curves were plotted for candidate biomarkers and their combination for differential diagnosis of benign and malignant nodules (Figure 2). The candidate diagnostic indicators included salivary T3, salivary FT3, salivary FT4, serum TG, and US. The diagnostic values of each index were subsequently determined. As a consequence, the sensitivity, specificity, AUC, and cut-off of salivary T3 were 68.18%, 66.67%, 0.679, and 1.710, respectively. The diagnostic values of salivary FT3 were 72.73%, 59.09%, 0.695, and 1.995, respectively; those of salivary FT4 were 66.67%, 77.27%, 0.714, and 0.955, respectively, while those of serum TG were 67.5%, 64.29%, 0.671, and 32.25, respectively. In contrast, the US had a sensitivity of 59.09% and a specificity of 83.33%, respectively (Table 4).
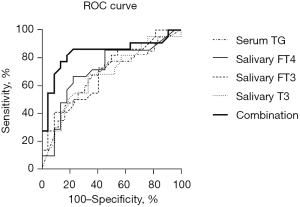
Table 4
Biomarkers | Sensitivity (%) | Specificity (%) | PPV (%) | NPV (%) | Youden index (%) | AUC | Cut-off value | 95% CI |
---|---|---|---|---|---|---|---|---|
Salivary T3 | 68.18 | 66.67 | 66.67 | 68.18 | 34.85 | 0.679 | 1.710 | 0.517–0.840 |
Salivary FT3 | 72.73 | 59.09 | 68.42 | 64.00 | 31.82 | 0.695 | 1.995 | 0.540–0.851 |
Salivary FT4 | 66.67 | 77.27 | 70.83 | 73.68 | 43.94 | 0.714 | 0.955 | 0.555–0.874 |
Serum TG | 67.50 | 64.29 | 67.50 | 64.29 | 31.79 | 0.671 | 32.25 | 0.552–0.789 |
US | 59.09 | 83.33 | 78.95 | 72.00 | 42.42 | – | – | – |
Combination | 86.36 | 77.27 | 79.17 | 85.00 | 63.63 | 0.836 | 0.335 | 0.706–0.966 |
PPV, positive predictive value; NPV, negative predictive value; AUC, area under the curve; CI, confidence interval.
Machine learning-based multi-biomarkers diagnostic model in TI-RADS borderline patients
First, the distributions of US TI-RADS grades and their respective diagnostic accuracy rates were analyzed. The US grades higher than 4A were classified as malignancy, while grade 4A and below was classed as benign. Based on Table 4, the sensitivity and specificity of US was 59.09% and 83.33% in differentiation of benign and malignant tumor, respectively. Among the TI-RADS US grades, grade 4A had the majority of the patients (31 cases, 36.05%). Meanwhile, 4A also had the poorest diagnostic accuracy (48.39%) compared to other grades (higher than 60%) (Table 5). After excluding 4A patients, the sensitivity and specificity of US increased to 92.86% and 74.07%, respectively. Considering that TI-RADS 4A patients accounted for the majority of US diagnoses and with the lowest diagnostic accuracy, a multi-biomarkers diagnostic model was adopted to improve the diagnostic effect of US in this subgroup.
Table 5
Grades | No. (%) | BTT | PTC | Accuracy, % |
---|---|---|---|---|
3 | 22 (25.58) | 20 | 2 | 90.91 |
4A | 31 (36.05) | 15 | 16 | 48.39 |
4B | 24 (27.91) | 5 | 19 | 79.17 |
4C | 6 (6.98) | 2 | 4 | 66.67 |
5 | 3 (3.49) | 0 | 3 | 100.00 |
TI-RADS, thyroid imaging reporting and data system; PTC, papillary thyroid carcinoma; BTT, benign thyroid tumor.
In the diagnosis of 4A patients, salivary FT3 used alone had a sensitivity, specificity, and AUC of 100.00%, 66.67%, and 0.854%, respectively. Thereafter, SVM and KNN algorithms were used to develop a prediction model which enrolled salivary T3, FT3, FT4, and serum TG. Consequently, the sensitivity and specificity of the SVM model reached 87.50% and 86.67%, respectively. The sensitivity and specificity of the KNN model reached 87.50% and 100.00%, respectively (Table 6).
Table 6
Biomarkers | Sensitivity (%) | Specificity (%) | PPV (%) | NPV (%) | Youden index (%) |
---|---|---|---|---|---|
Traditional method | |||||
US | 59.09 | 83.33 | 78.95 | 72.00 | 42.42 |
US (without 4A) | 92.86 | 74.07 | 66.93 | 78.79 | 90.91 |
Logistic | 86.36 | 77.27 | 79.17 | 85.00 | 63.63 |
TI-RADS 4A group | |||||
4A_FT3 | 100.00 | 66.67 | 66.67 | 76.19 | 100.00 |
4A_SVM | 87.50 | 86.67 | 74.17 | 87.50 | 86.67 |
4A_KNN | 87.50 | 100.00 | 87.50 | 100.00 | 88.24 |
Combination | |||||
US + 4A_FT3 | 95.45 | 71.43 | 66.88 | 77.78 | 93.75 |
US + SVM | 90.91 | 78.57 | 69.48 | 81.63 | 89.19 |
US + KNN | 90.91 | 83.33 | 74.24 | 85.11 | 89.74 |
US, ultrasound; TI-RADS, thyroid imaging reporting and data system; PPV, positive predictive value; NPV, negative predictive value; SVM, support vector machine; KNN, K-nearest neighbour.
Multi-biomarkers model-assisted US differential diagnosis
Finally, US TI-RADS grades and a multi-biomarkers diagnostic model for 4A patients were combined as a multi-index diagnostic model. In this diagnostic model, the patients were first evaluated by the US, then, a multi-biomarker model was utilized to verify benign and malignant lesions in those with 4A grade. The results revealed that US + salivary FT3 in 4A patients exhibited a sensitivity of 95.45% and a specificity of 71.43%. The US+SVM prediction model had a sensitivity of 90.91% and a specificity of 78.57%. The US + KNN prediction model had the best sensitivity and specificity performance of 90.91% and 83.33%, respectively (Table 6).
Discussion
For the first time, the present study examined salivary tumor markers and thyroid hormone profiles in individuals with benign and malignant thyroid tumors. We found that salivary T3, FT3, and FT4 levels were significantly different in benign and malignant tumors. Subsequently, we performed univariate and multivariate logistic regression to evaluate salivary FT3 and US as independent risk variables for predicting benign and malignant cancers. Consequently, patients of 4A accounted for the greatest proportion (36.05%) among all participants; nevertheless, it had the lowest diagnostic accuracy (48.39%), which influenced the overall diagnostic efficiency. As a result, a multi-biomarker model primarily based on salivary indicators was constructed to verify the diagnosis of 4A patients, where several machine learning algorithms were employed. The diagnostic performance of the KNN model was efficient, with a sensitivity of 87.50% and a specificity of 100.00%. Finally, we combined US and 4A diagnostic models, where patients with 4A grades received multi-biomarkers diagnostic models for validation, and other patients directly received US diagnoses. As a consequence, the US + KNN model had the best diagnostic efficacy, with a sensitivity and specificity of 90.91% and 83.33%, respectively, compared to that of 59.09%, 83.33% for the US alone. In this regard, salivary biomarkers could improve the diagnostic efficacy of borderline thyroid nodules in US examination with a potential application in the differentiation of thyroid benign and malignant nodules.
Currently, US is the primary screening and diagnostic technique for the distinction of benign and malignant thyroid nodules. A meta-analysis examined US elastography for the detection of benign and malignant thyroid cancers. After pooling 5,481 nodules from 4,468 patients, the average sensitivity and specificity of US identification approached 0.79 and 0.77, respectively (15), suggesting the high potential of US in differentiating benign from malignant tumors. Notably, the TI-RADS classification is the extensively used evaluation method in ultrasonography. Horvath (16) and Kwak (17) found that TI-RADS may be used to increase diagnostic accuracy, decrease needle biopsies, and eventually improve thyroid nodule treatment. In the TI-RADS system, thyroid nodules are classified into 6 classes, among which the probability of malignancy was 0 in the category below 3; 5% in category 3; 5–10% in category 4A; 10–50% in 4B; 50–85% in 4C; higher than 85% in category 5; and 100% in category 6 (7).
In clinical practice, it is often difficult to distinguish benign from malignant tumors in patients with borderline nodules including 4A grade; this, therefore, requires further needle biopsies to confirm the diagnosis, hence increasing patient suffering and impairing the effective performance of the medical system. As a result, auxiliary strategies are essential to augment the diagnostic accuracy of US. Additionally, borderline patients account for a significant fraction of the population. Among the 86 patients who participated in this work, 4A patients as borderline cases accounted for 36.05% (the highest percentage). At the same time, the diagnosis of category 4A patients was also difficult with a malignancy probability of 51.61%. Other studies on the predictive accuracy of various TI-RADS classifications have reported similar findings (18) in a malignant rate of 9.5% in 4A patients. Nonetheless, herein, we found a malignancy rate of 48% for category 4B individuals. This disparity may reflect regional differences in the subjective diagnosis of US physicians. However, the US examination frequently exhibited low prediction accuracy among the boundary nodules between benign and malignant classifications. Notably, needle biopsy is a critical adjunct to the US with high diagnostic accuracy for the ultimate confirmation of high-risk patients. Nevertheless, it has a number of limitations, including high cost, risk of bleeding, and so on, which hamper its applicability in mass screening. Thus, a simple and non-invasive auxiliary detection tool is immediately required in clinical practice.
Serum biomarkers are used in the diagnosis and treatment of liver cancer, pancreatic cancer, lung cancer, and other malignancies (19,20), and also offer diagnostic potential in the detection of thyroid carcinoma. Giovanella et al. (21) discovered the value of serum TG in detecting differentiated thyroid cancer. Elsewhere, Gul et al. (22) discovered that low serum FT3, FT4, and TSH levels were linked to an increased risk of thyroid cancer. Many other indicators have also been uncovered including interleukin (IL)-8 (23), Leptin (24), and so on. Nonetheless, the application of serum biomarkers is hindered by the low diagnostic efficacity of PTC. Moreover, our findings of poor diagnostic value of serum biomarkers indicated similar outcomes. Therefore, we used salivary biomarkers for investigation.
Besides serum tumor indicators, studies on salivary biomarkers have intensified in recent years. Salivary biomarkers have been used to diagnose pancreatic cancer, breast cancer, and other types of cancers (11,25,26). Several studies have shown that numerous salivary indicators have greater concentrations than those in serum. This is potentially attributed to a one-way filtering process between the salivary glands and blood, resulting in constant biomarker concentrations in saliva. Because thyroid cancer is less aggressive compared to other types of cancer, its blood biomarkers change insignificantly. In contrast, highly concentrated salivary biomarkers may have a significant diagnostic capability for thyroid nodule evaluation. Herein, salivary tumor biomarkers were also higher than serum tumor biomarkers. However, they lacked considerable diagnostic significance. This might be due to the small sample size; therefore, this should be confirmed in future research with a larger sample size.
Furthermore, previous research has reported a link between thyroid illness and the salivary glands. Saliva contains a greater quantity of iodine than that in serum (27). Salivary glands, stomach, and thyroid share a sodium iodide transporter (NIS) capable of concentrating iodine ions (28). As such, when I131 radiation is used as radiotherapy after thyroid surgery, the salivary glands usually develop additional damage (29). Consequently, salivary biomarkers were used in the detection of thyroid disorders. Guo et al. (30) noted a significant correlation between elevated salivary iodine levels and the presence of thyroid nodules. Nonetheless, no research has been performed to establish the correlation between salivary biomarkers and thyroid cancer. Therefore, we examined the expression of tumor markers and thyroid hormones in saliva and discovered saliva T3, FT3, FT4, and blood TG levels as potential biomarkers that distinguish benign from malignant thyroid nodules.
As previously stated, the major difficulty in US thyroid nodule identification is the low differential predictive value of benign-malignant borderline nodules. In this work, 2 machine learning algorithms, SVM and KNN, were used to improve differential diagnosis in category 4A. Researchers have previously employed machine learning to help in PTC diagnosis; however, most of them focused on the analysis of US images (2,31). Also, these investigations require labeling and identification of many US photographs by specialists, which demands high development costs. The objective of this study was to develop a low-cost, simple, and easy-to-promote saliva indicator program to supplement the US examination and consequently improve the prediction accuracy of thyroid nodules.
We confirmed that the distribution of salivary biomarkers is significantly different between benign and malignant nodules, hence making diagnosis feasible. By integrating with the US, a KNN-assisted salivary biomarker-based diagnostic model could distinguish PTC from BTT with a sensitivity and specificity of up to 90.91% and 83.33%, respectively, compared to 59.09% and 83.33%, respectively, for US analysis alone. Therefore, we believe that using salivary biomarkers combined with the US can significantly improve the accuracy of thyroid nodule identification, specifically in borderline cases. As a convenient, rapid, and non-invasive detection method, salivary biomarkers have the potential to significantly improve the prediction accuracy in the differential diagnosis of thyroid tumors, reduce the number of fine-needle aspiration biopsy needed, thereby reducing patient suffering, and increasing the efficiency. This minimizes the medical resource consumption, making them a feasible option for thyroid nodule screening.
This research has compelling limitations. First, it was a single-center study, suggesting a possibility of sample bias. Secondly, the sample size was inadequate, with 86 overall cases and 31 cases in 4A grade. In the future, we will enroll additional cases and centers in this project. Moving forward, we will focus on 4A borderline patients and assess the predictive value of salivary biomarker-based diagnostic models.
Conclusions
In conclusion, our findings indicated that salivary biomarkers showed great potential for identifying benign and malignant thyroid cancers, which might enhance the US prediction accuracy considerably. Moreover, we found that salivary FT3 is an independent risk factor for PTC and may be utilized as a diagnostic biomarker. This means that salivary biomarkers have extensive application potential; therefore, additional research into their diagnostic value is required.
Acknowledgments
We thank home-for-researchers for its linguistic assistance during the preparation of this manuscript. Thanks for the anonymous reviewers for their valuable comments and suggestions that helped improve the quality of our manuscript.
Funding: This study was financially supported by the National Natural Science Fund of China (82100639, 81970526, 81900550, and 81770599). The funding body had no role in the design of the study and collection, analysis, interpretation of data or preparation of the manuscript.
Footnote
Reporting Checklist: The authors have completed the STARD reporting checklist. Available at https://gs.amegroups.com/article/view/10.21037/gs-21-864/rc
Data Sharing Statement: Available at https://gs.amegroups.com/article/view/10.21037/gs-21-864/dss
Conflicts of Interest: All authors have completed the ICMJE uniform disclosure form (available at https://gs.amegroups.com/article/view/10.21037/gs-21-864/coif). The authors have no conflicts of interest to declare.
Ethical Statement: The authors are accountable for all aspects of the work in ensuring that questions related to the accuracy or integrity of any part of the work are appropriately investigated and resolved. The study was conducted in accordance with the Declaration of Helsinki (as revised in 2013). The experimental protocol was approved by the Shanghai Ruijin Rehabilitation Hospital Ethics Committee. Informed consent was waived for this non-interventional, observational study by the Ethics Committees, in which the patient data used were kept strictly confidential.
Open Access Statement: This is an Open Access article distributed in accordance with the Creative Commons Attribution-NonCommercial-NoDerivs 4.0 International License (CC BY-NC-ND 4.0), which permits the non-commercial replication and distribution of the article with the strict proviso that no changes or edits are made and the original work is properly cited (including links to both the formal publication through the relevant DOI and the license). See: https://creativecommons.org/licenses/by-nc-nd/4.0/.
References
- Siegel RL, Miller KD, Jemal A. Cancer statistics, 2019. CA Cancer J Clin 2019;69:7-34. [Crossref] [PubMed]
- Li X, Zhang S, Zhang Q, et al. Diagnosis of thyroid cancer using deep convolutional neural network models applied to sonographic images: a retrospective, multicohort, diagnostic study. Lancet Oncol 2019;20:193-201. [Crossref] [PubMed]
- Thompson AM, Turner RM, Hayen A, et al. A preoperative nomogram for the prediction of ipsilateral central compartment lymph node metastases in papillary thyroid cancer. Thyroid 2014;24:675-82. [Crossref] [PubMed]
- Song G, Xue F, Zhang C. A Model Using Texture Features to Differentiate the Nature of Thyroid Nodules on Sonography. J Ultrasound Med 2015;34:1753-60. [Crossref] [PubMed]
- Park JY, Lee HJ, Jang HW, et al. A proposal for a thyroid imaging reporting and data system for ultrasound features of thyroid carcinoma. Thyroid 2009;19:1257-64. [Crossref] [PubMed]
- Melany M, Chen S. Thyroid Cancer: Ultrasound Imaging and Fine-Needle Aspiration Biopsy. Endocrinol Metab Clin North Am 2017;46:691-711. [Crossref] [PubMed]
- Russ G. Risk stratification of thyroid nodules on ultrasonography with the French TI-RADS: description and reflections. Ultrasonography 2016;35:25-38. [Crossref] [PubMed]
- Chernyavsky VS, Shanker BA, Davidov T, et al. Is one benign fine needle aspiration enough? Ann Surg Oncol 2012;19:1472-6. [Crossref] [PubMed]
- Luo G, Jin K, Deng S, et al. Roles of CA19-9 in pancreatic cancer: Biomarker, predictor and promoter. Biochim Biophys Acta Rev Cancer 2021;1875:188409. [Crossref] [PubMed]
- Tzartzeva K, Singal AG. Testing for AFP in combination with ultrasound improves early liver cancer detection. Expert Rev Gastroenterol Hepatol 2018;12:947-9. [Crossref] [PubMed]
- Wang X, Kaczor-Urbanowicz KE, Wong DT. Salivary biomarkers in cancer detection. Med Oncol 2017;34:7. [Crossref] [PubMed]
- Liu J, Duan Y. Saliva: a potential media for disease diagnostics and monitoring. Oral Oncol 2012;48:569-77. [Crossref] [PubMed]
- Agha-Hosseini F, Mirzaii-Dizgah I, Rahimi A. Correlation of serum and salivary CA15-3 levels in patients with breast cancer. Med Oral Patol Oral Cir Bucal 2009;14:e521-4. [Crossref] [PubMed]
- Dyckhoff G, Warta R, Gonnermann A, et al. Carbohydrate antigen 19-9 in saliva: possible preoperative marker of malignancy in parotid tumors. Otolaryngol Head Neck Surg 2011;145:772-7. [Crossref] [PubMed]
- Sun J, Cai J, Wang X. Real-time ultrasound elastography for differentiation of benign and malignant thyroid nodules: a meta-analysis. J Ultrasound Med 2014;33:495-502. [Crossref] [PubMed]
- Horvath E, Majlis S, Rossi R, et al. An ultrasonogram reporting system for thyroid nodules stratifying cancer risk for clinical management. J Clin Endocrinol Metab 2009;94:1748-51. [Crossref] [PubMed]
- Kwak JY, Han KH, Yoon JH, et al. Thyroid imaging reporting and data system for US features of nodules: a step in establishing better stratification of cancer risk. Radiology 2011;260:892-9. [Crossref] [PubMed]
- Fernández Sánchez J. TI-RADS classification of thyroid nodules based on a score modified regarding the ultrasound criteria for malignancy. Rev Argent Radiol 2014;78:138-48.
- Hasan S, Jacob R, Manne U, et al. Advances in pancreatic cancer biomarkers. Oncol Rev 2019;13:410. [Crossref] [PubMed]
- Chatterjee R, Mitra A. An overview of effective therapies and recent advances in biomarkers for chronic liver diseases and associated liver cancer. Int Immunopharmacol 2015;24:335-45. [Crossref] [PubMed]
- Giovanella L, Clark PM, Chiovato L, et al. Thyroglobulin measurement using highly sensitive assays in patients with differentiated thyroid cancer: a clinical position paper. Eur J Endocrinol 2014;171:R33-46. [Crossref] [PubMed]
- Gul K, Ozdemir D, Dirikoc A, et al. Are endogenously lower serum thyroid hormones new predictors for thyroid malignancy in addition to higher serum thyrotropin? Endocrine 2010;37:253-60. [Crossref] [PubMed]
- Kobawala TP, Patel GH, Gajjar DR, et al. Clinical utility of serum interleukin-8 and interferon-alpha in thyroid diseases. J Thyroid Res 2011;2011:270149. [Crossref] [PubMed]
- Hedayati M, Yaghmaei P, Pooyamanesh Z, et al. Leptin: a correlated Peptide to papillary thyroid carcinoma? J Thyroid Res 2011;2011:832163. [Crossref] [PubMed]
- Porto-Mascarenhas EC, Assad DX, Chardin H, et al. Salivary biomarkers in the diagnosis of breast cancer: A review. Crit Rev Oncol Hematol 2017;110:62-73. [Crossref] [PubMed]
- Zhang L, Farrell JJ, Zhou H, et al. Salivary transcriptomic biomarkers for detection of resectable pancreatic cancer. Gastroenterology 2010;138:949-57.e1-7.
- Ross GR, Fabersani E, Russo M, et al. Effect of Excess Iodide Intake on Salivary Glands in a Swiss Albino Mice Model. Biomed Res Int 2017;2017:6302869. [Crossref] [PubMed]
- Wapnir IL, van de Rijn M, Nowels K, et al. Immunohistochemical profile of the sodium/iodide symporter in thyroid, breast, and other carcinomas using high density tissue microarrays and conventional sections. J Clin Endocrinol Metab 2003;88:1880-8. [Crossref] [PubMed]
- Hyer S, Kong A, Pratt B, et al. Salivary gland toxicity after radioiodine therapy for thyroid cancer. Clin Oncol (R Coll Radiol) 2007;19:83-6. [Crossref] [PubMed]
- Guo W, Pan Z, Zhang Y, et al. Saliva Iodine Concentration in Children and Its Association with Iodine Status and Thyroid Function. J Clin Endocrinol Metab 2020;105:dgaa471.
- Xia J, Chen H, Li Q, et al. Ultrasound-based differentiation of malignant and benign thyroid Nodules: An extreme learning machine approach. Comput Methods Programs Biomed 2017;147:37-49. [Crossref] [PubMed]
(English Language Editor: J. Jones)