A pre-operative MRI-based brain metastasis risk-prediction model for triple-negative breast cancer
Introduction
It is well accepted that triple-negative breast cancer (TNBC) has a worse short-term prognosis than other breast cancer subtypes (1). The 2-year mark after the initial diagnosis of TNBC represents a unique milestone (1). In this 2-year period, the mortality rate of TNBC shows a steep upward trend, then the rate would gradually approach other subtypes after this milestone. Thus, the initial 2-year intervention is of great importance to the prognosis improvement of TNBC patients.
Brain metastasis (BM) is one of the most common and severe negative events of TNBC, and usually occurs within 2 years of diagnosis (2). Apart from lethal metastasis, BM of TNBC is also a clinical indication of a non-ideal systemic treatment response (3). Thus, BM has attracted considerable attention in relation to TNBC prognosis. For example, a BM monitoring system was developed to enable early detection and achieve a better response (4,5). However, existing studies have mainly discussed passive post-operative monitoring, and the use of active pre-operative risk stratification methods is rare.
In clinical practice, the magnetic resonance imaging (MRI) as a routine examination of the breast cancer have concluded many radiological signs include T2 hyperintensity and rim enhancement that greatly helped the TNBC diagnosis (6). Recently, studies have shown that pre-operative MRI data predicts breast cancer prognosis based on radiomics analysis (7,8). Thus, we sought to build an MRI data-based pre-operative BM risk-prediction model and improve TNBC patient prognosis. Our study included TNBC cases with available BM status data from a local center. The quantitative pre-operative MRI features were extracted and used to train the machine-learning model.
We present the following article in accordance with the TRIPOD reporting checklist (available at https://dx.doi.org/10.21037/gs-21-537).
Methods
Cohort
A total of 643 patients with breast cancer were enrolled in the study, all of these patients were treated surgically in our hospital and had postoperative pathological reports. The initial diagnosis dates of patients fell between May 2012 and June 2018. The China Anti-Cancer Association’s guidelines on the clinical diagnosis and treatment of breast cancer were used to guide individual treatment. To be eligible for inclusion in this study, the following primary screening criteria had to be met for each patient: (I) An original immunohistochemical examination had to confirm the triple-negative status of the estrogen receptor (ER), progesterone receptor (PR), and human epidermal growth factor receptor 2 (Her2); (II) pre-operative fat-suppressed T2-weighted MRI had to be available; (III) 24-month post-operative follow-up records detailing outcomes had to be available; (IV) a standard brain MRI follow-up record for BM monitoring had to be available; (V) each patient had to have positive MRI evidence of BM, have undergone surgical resection, and have a pathology report that supported the diagnosis of BM. Additionally, the following secondary imaging screening criteria had to be met: (I) there had to be no image degradation, such as motion artifacts; and (II) the BM had to be a single-lesion type. After the secondary screening, propensity score matching (PSM) was used to perform baseline matching between BM TNBC cases and non-BM TNBC cases based on age (rounded). The method for training and test data set separation are detailed later in this paper in the section that describes the machine-learning model. All procedures performed in this study involving human participants were in accordance with the Declaration of Helsinki (as revised in 2013). The study was approved by ethics board of the Sixth Hospital of Wuhan (No. WHSHIRB-K-2021012) and informed consent was taken from all the patients.
Pre-operative MRI scan
A 1.5-Tesla magnetic resonance scanner at our local center was used to obtain fat-suppressed T2-weighted MRI. The original Digital Imaging and COmmunications in Medicine (DICOM) files were downloaded directly from the local hospital’s picture archiving and communication system (PACS) system, and Neuroimaging Informatics Technology Initiative (NIfTI) format conversion and anonymization was achieved with dcm2niiX (version: v1.0.20190902). The anonymized NIfTI files were used for the subsequent analysis.
Tumor lesion description and region of interest (ROI) segmentation
Two board-certificated radiologists, both experts in breast cancer diagnosis, participated in the radiographic reading and ROI segmentation. In the radiographic reading, the radiologists evaluated each lesion’s length of the long axis and shape (oval/irregular). The two radiologists independently finished the contour in the ROI segmentation using Slicer software (version: 4.11.20200930). The radiologists were able to browse all the available radiological studies, but were blinded to patients’ BM status. The intraclass correlation coefficient (ICC) of the final ROI volume was calculated to evaluate consistency concordance.
Radiomics feature extraction
The Cancer Imaging Phenomics Toolkit (version v.1.8.0.Beta) was used to extract radiomics features from the collected fat-suppressed T2-weighted MRI. Feature categories included morphology, histogram, intensity, and texture. The 2-dimensional (2D) lattice extraction mode was applied. After 0-value based dimension reduction and z-score normalization, a feature matrix was used as input to train the machine-learning model.
Training and evaluating the performance of the machine-learning model
Orange Data Mining (version 3.27.1) was used to train the machine-learning model and evaluate its performance. Classical modeling machine-learning algorithms, including random forest (RF), support vector machine (SVM), K-nearest neighbor (KNN), least absolute shrinkage and selection operator (LASSO) regression, Naive Bayes, and Neural network, were included. The default parameters were used, and 0.5 was set as the prediction threshold. The test and score module of Orange software was used to evaluate performance, and a 10-fold cross-validation was used for benchmark testing. The performance measurements included the area under the curve (AUC) for the receiver operating characteristic (ROC) curve, precision, accuracy, sensitivity, and specificity. In the sensitivity and specificity calculation, the target event was set as BM.
Statistical analysis
SPSS software (version 22.0) was used for the statistical analysis and PSM. Due to the insignificance of the Kolmogorov-Smirnov normality test, a non-parametric test method was used to compare differences between the long axis diameters of the BM Group. A Chi-square test or Fisher’s exact test was used to compare the categorical variables of the BM Group. A P<0.05 was considered statistically significant.
Results
Clinical and radiographic characteristics of the study cohort
Based on the 643 collected breast cancer cases, the screening and PSM process generated 84 TNBC cases (comprising 42 pairs) with and without BM during the initial post-operative period of 24 months. The cohort selection process is summarized in Figure 1. Patients’ clinical and radiographic characteristics are summarized in Table 1. No clinical or radiographic differences were found between the BM groups. Patients’ ages at diagnosis were not compared due to the PSM design.
Table 1
Items | Brain metastasis group (n=42) | Non-brain metastasis group (n=42) | P |
---|---|---|---|
Clinical characteristics | |||
Marital status | >0.05 | ||
Married | 36 | 34 | |
Single, widowed, divorced, separated | 6 | 8 | |
Original TNBC side | >0.05 | ||
Left | 23 | 24 | |
Right | 19 | 18 | |
Histological grade | >0.05 | ||
II | 6 | 12 | |
III | 36 | 30 | |
Radiographic features | |||
TNBC length of the long axis (mm) | 27.1 (26.5–27.6) | 26.9 (26.1–27.5) | >0.05 |
TNBC shape | >0.05 | ||
Oval | 31 | 31 | |
Irregular | 11 | 11 |
Radiomics feature analysis
The 84 TNBC cases (comprising 42 pairs) were subjected to a radiomics feature analysis. The primary workflow is summarized in Figure 2. In relation to the concordance evaluation, the ROI volume concordance between the two radiologists was satisfactory (ICC =0.86). Thus, we selected the ROIs contoured by the senior radiologist. In relation to the lattice radiomics feature extraction, 3,854 quantitative features were extracted. The feature categories included morphology, intensity, histogram, gray-level co-occurrence matrix, gray-level run length matrix, and local binary pattern. After dimension reduction, 2,480 lattice radiomics features were kept and entered into the machine-learning model for training. Figure 3 shows the most relevant BM lattice radiomics features (only the top 50 features according to the information gain ratio ranking are shown).
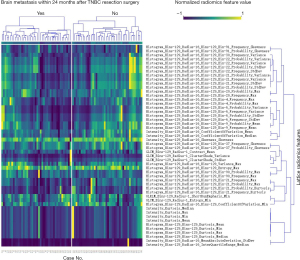
Machine-learning TNBC BM risk-prediction model
The training of the machine-learning models was based on the 2,480 kept lattice radiomics features. Table 2 summarizes the 10-fold cross-validation performance of the generated models. Among the 6 classical machine-learning algorithms, the Naïve Bayes method had the highest AUC (0.878, 95% confidence interval: 0.743–0.911) and accuracy (0.786). The Naïve Bayes model had higher specificity than sensitivity, and thus was more suitable for identifying low-risk BM TNBC cases. Table 3 shows the model confusion matrix. All the generated models are available upon reasonable request (via email) and can be imported into Orange software for exploration.
Table 2
Machine learning algorithm | AUC (95% confidence interval) | Accuracy | Precision | Sensitivity (%) | Specificity (%) | |
---|---|---|---|---|---|---|
Brain metastasis | Non-brain metastasis | |||||
Naive Bayes | 0.878 (0.743–0.911) | 0.786 | 0.800 | 0.773 | 76.2 | 81.0 |
LASSO regression | 0.739 (0.607–0.809) | 0.667 | 0.652 | 0.684 | 71.4 | 61.9 |
Support vector machine | 0.702 (0.592–0.797) | 0.631 | 0.617 | 0.649 | 69.0 | 57.1 |
Random forest | 0.695 (0.585–0.791) | 0.643 | 0.658 | 0.630 | 59.5 | 69.0 |
Neural network | 0.690 (0.580–0.786) | 0.702 | 0.698 | 0.707 | 71.4 | 69.0 |
K-nearest neighbors | 0.647 (0.535–0.748) | 0.607 | 0.600 | 0.615 | 64.3 | 57.1 |
Table 3
Actual brain metastasis status | Predicted brain metastasis status | ||
---|---|---|---|
Yes | No | Sum | |
Yes | 32 (76.2%) | 10 (23.8%) | 42 |
No | 8 (19.0%) | 34 (81.0%) | 42 |
Sum | 40 | 44 | 84 |
Discussion of the machine-learning models
Our discussion focuses on the Naïve Bayes model, as it achieved the highest performance. A nomogram was plotted to depict the features’ absolute weights based on the whole cohort (see Figure 4). The entropy feature that belonged to the gray-level co-occurrence matrix category contributed most to the prediction ability of the model, which mainly described the disorder level of the image. Additionally, the lattice radiomics features of histogram and intensity contributed considerably to the model.
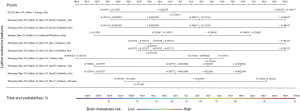
Discussion
BM is a critical clinical milestone in the course of TNBC. The accepted law of BM in TNBC included common, lethal, and unique. In 2008, Lin et al. examined 116 cases of metastatic TNBC and found that the overall prognosis of BM was poor (the median survival time was only 4.9 months after the diagnosis of BM) (1). It has been reported that the age- and race-adjusted rate of death of BM was 3.4 times higher than that of other primary metastasis types (9). However, the dismal prognosis of TNBC patients with BM is not simply due to the BM lesion itself. Indeed, death, as a direct consequence, is uncommon in TNBC patients with BM; rather, unsatisfied systemic control is the main cause of death. The BM could also harbor novel genetic alterations compared to the original lesion (10). Thus, BM in TNBC patients is a unique event that should be considered a particular clinical scenario, and more importantly, as a treatment response marker. To predict this marker, our study established a BM risk-prediction model based on the radiomics features extracted from pre-operative MRI. The data of 84 TNBC cases (comprising 42 pairs), with and without BM within 24 months after the primary breast cancer resection surgery, were collected. Next, 2,480 filtrated lattice radiomics features were extracted. The model that used the Naïve Bayes algorithm had the highest performance (AUC =0.878, accuracy =0.786, sensitivity =76.2%, and specificity =81.0% when BM was the target event in 10-fold verification mode). The interpretative analysis of the model showed that texture, histogram, and intensity features contributed significantly to the prediction ability of the model. Morphological features were not included.
The known BM risk factors for breast cancers include younger age, disease staging, histological subtype, and breast cancer gene 1/2 mutation status (11-15). Due to the considerable heterogeneity of each group, these risk factors cannot be directly used in clinical practice. Given that BM is related to overall treatment response, Gabani et al. developed a TNBC BM risk-assessment tool based on posttreatment lymph node status and grade change (16); however, this model was based on a relatively specific cohort that had undergone neoadjuvant therapy, surgery, and radiotherapy treatment. Besides, the after-surgery prediction could be relatively late. Other BM risk-prediction models have been based on immunohistochemical marker combinations, alternative splicing sites, and chromosome amplification status (17-19). However, a lack of commonly accepted real-world diagnosis power reports and non-routine examination requirements are barriers to the comprehensive clinical verification of these models. Consequently, central nervous symptoms are still the primary and sometimes only available tool by which most clinicians identify BM in TNBC patients. In well-known ESME-MBC trial cohort, the majority (70.7%) of the BM in TNBC patients were found in symptom-initiated procedure, which clearly showed the screening role of clinical symptom-based diagnosis and the decision-making role of the MRI examination (20). But the MRI as an objective examination in lesion detection requires less clinical experience than symptom-based diagnosis. This clinical dilemma apparently called for a better data interpretation method for the BM prediction in TNBC.
In recent years, radiomics analysis, which is a quantitative imaging analysis method, has emerged. By extracting a large number of features, radiomics workflows can significantly enhance clinicians’ understandings of medical images. In the field of breast cancer, previous studies have shown that radiomics analysis can be applied in early screening, subtype prediction, immune infiltration pattern differentiation, and treatment response prediction (7,21-25). Braman et al. used peritumoral radiomics characteristics to predict the tumor microenvironmental status and treatment response of HER-2 positive breast cancer (26). The previous study also showed the ability of three-dimensional radiomics feature in predicting TNBC systemic recurrence (27). However, BM risk stratification based on such a method remains rare. Our study conducted further research on this topic. In 2016, Bae et al. reported that pretreatment MRI feature peritumoral edema was the only radiographic feature associated with worse recurrence-free survival in TNBC (28). As the distant recurrence of TNBC was represented by brain and lung metastasis, our study indicated the feasibility of MRI-based BM risk prediction. Unlike the peritumoral edema used in the above study, in which there was a moderate overall agreement between radiologists, radiomics feature extraction is almost completely robust, as it relies on a mathematical definition. Tumor ROI segmentation represented the primary variation. Tumor ROI segmentation can be controlled by signal thresholding on MRI and was supported by our study’s satisfied ROI volume concordance (ICC =0.86). It should also be noted that, similar to the previous study, our BM risk-prediction model and high-correlation feature list did not include morphological features.
The present study had some limitations. First, its retrospective nature may have caused related bias. Second, the internal validation was based on data from a local, single center, and this limited cohort may not comprehensively reflect the real-world performance of the model. Finally, the radiomics analysis method used in this study required manual ROI segmentation, and the subjective contour criteria could have introduced bias; however, this limitation should be acceptable given the ICC results of our study.
Conclusions
Our study established a pre-operative TNBC BM risk-prediction model using 84 cases from a local hospital. This imaging-based non-invasive model should help in early BM risk stratification and benefit prognosis.
Acknowledgments
Funding: None.
Footnote
Reporting Checklist: The authors have completed the TRIPOD reporting checklist. Available at https://dx.doi.org/10.21037/gs-21-537
Data Sharing Statement: Available at https://dx.doi.org/10.21037/gs-21-537
Conflicts of Interest: All authors have completed the ICMJE uniform disclosure form (available at https://dx.doi.org/10.21037/gs-21-537). The authors have no conflicts of interest to declare.
Ethical Statement: The authors are accountable for all aspects of the work in ensuring that questions related to the accuracy or integrity of any part of the work are appropriately investigated and resolved. All procedures performed in this study involving human participants were in accordance with the Declaration of Helsinki (as revised in 2013). The study was approved by the ethics board of the Sixth Hospital of Wuhan (No. WHSHIRB-K-2021012) and informed consent was taken from all the patients.
Open Access Statement: This is an Open Access article distributed in accordance with the Creative Commons Attribution-NonCommercial-NoDerivs 4.0 International License (CC BY-NC-ND 4.0), which permits the non-commercial replication and distribution of the article with the strict proviso that no changes or edits are made and the original work is properly cited (including links to both the formal publication through the relevant DOI and the license). See: https://creativecommons.org/licenses/by-nc-nd/4.0/.
References
- Lin NU, Claus E, Sohl J, et al. Sites of distant recurrence and clinical outcomes in patients with metastatic triple-negative breast cancer: high incidence of central nervous system metastases. Cancer 2008;113:2638-45. [Crossref] [PubMed]
- Rostami R, Mittal S, Rostami P, et al. Brain metastasis in breast cancer: a comprehensive literature review. J Neurooncol 2016;127:407-14. [Crossref] [PubMed]
- Witzel I, Laakmann E, Weide R, et al. Treatment and outcomes of patients in the Brain Metastases in Breast Cancer Network Registry. Eur J Cancer 2018;102:1-9. [Crossref] [PubMed]
- Cheun JH, Jung J, Lee ES, et al. Intensity of metastasis screening and survival outcomes in patients with breast cancer. Sci Rep 2021;11:2851. [Crossref] [PubMed]
- Cagney DN, Martin AM, Catalano PJ, et al. Implications of Screening for Brain Metastases in Patients With Breast Cancer and Non-Small Cell Lung Cancer. JAMA Oncol 2018;4:1001-3. [Crossref] [PubMed]
- Angelini G, Marini C, Iacconi C, et al. Magnetic resonance (MR) features in triple negative breast cancer (TNBC) vs receptor positive cancer (nTNBC). Clin Imaging 2018;49:12-6. [Crossref] [PubMed]
- Park H, Lim Y, Ko ES, et al. Radiomics Signature on Magnetic Resonance Imaging: Association with Disease-Free Survival in Patients with Invasive Breast Cancer. Clin Cancer Res 2018;24:4705-14. [Crossref] [PubMed]
- Huang SY, Franc BL, Harnish RJ, et al. Exploration of PET and MRI radiomic features for decoding breast cancer phenotypes and prognosis. NPJ Breast Cancer 2018;4:24. [Crossref] [PubMed]
- Hung MH, Liu CY, Shiau CY, et al. Effect of age and biological subtype on the risk and timing of brain metastasis in breast cancer patients. PLoS One 2014;9:e89389 [Crossref] [PubMed]
- Sperduto PW, Mesko S, Li J, et al. Estrogen/progesterone receptor and HER2 discordance between primary tumor and brain metastases in breast cancer and its effect on treatment and survival. Neuro Oncol 2020;22:1359-67. [Crossref] [PubMed]
- Barnholtz-Sloan JS, Sloan AE, Davis FG, et al. Incidence proportions of brain metastases in patients diagnosed (1973 to 2001) in the Metropolitan Detroit Cancer Surveillance System. J Clin Oncol 2004;22:2865-72. [Crossref] [PubMed]
- Tsukada Y, Fouad A, Pickren JW, et al. Central nervous system metastasis from breast carcinoma. Autopsy study. Cancer 1983;52:2349-54. [Crossref] [PubMed]
- Arvold ND, Oh KS, Niemierko A, et al. Brain metastases after breast-conserving therapy and systemic therapy: incidence and characteristics by biologic subtype. Breast Cancer Res Treat 2012;136:153-60. [Crossref] [PubMed]
- Song Y, Barry WT, Seah DS, et al. Patterns of recurrence and metastasis in BRCA1/BRCA2-associated breast cancers. Cancer 2020;126:271-80. [Crossref] [PubMed]
- Slimane K, Andre F, Delaloge S, et al. Risk factors for brain relapse in patients with metastatic breast cancer. Ann Oncol 2004;15:1640-4. [Crossref] [PubMed]
- Gabani P, Weiner AA, Hernandez-Aya LF, et al. Treatment response as predictor for brain metastasis in triple negative breast cancer: A score-based model. Breast J 2019;25:363-72. [Crossref] [PubMed]
- Klimov S, Rida PC, Aleskandarany MA, et al. Novel immunohistochemistry-based signatures to predict metastatic site of triple-negative breast cancers. Br J Cancer 2017;117:826-34. [Crossref] [PubMed]
- Gong S, Song Z, Spezia-Lindner D, et al. Novel Insights Into Triple-Negative Breast Cancer Prognosis by Comprehensive Characterization of Aberrant Alternative Splicing. Front Genet 2020;11:534. [Crossref] [PubMed]
- Qian J, Chen H, Ji X, et al. A 3q gene signature associated with triple negative breast cancer organ specific metastasis and response to neoadjuvant chemotherapy. Sci Rep 2017;7:45828. [Crossref] [PubMed]
- Gobbini E, Ezzalfani M, Dieras V, et al. Time trends of overall survival among metastatic breast cancer patients in the real-life ESME cohort. Eur J Cancer 2018;96:17-24. [Crossref] [PubMed]
- Zhou J, Lu J, Gao C, et al. Predicting the response to neoadjuvant chemotherapy for breast cancer: wavelet transforming radiomics in MRI. BMC Cancer 2020;20:100. [Crossref] [PubMed]
- Wei W, Cao S, Liu J, et al. Fibroblast growth factor receptor 4 as a prognostic indicator in triple-negative breast cancer. Transl Cancer Res 2020;9:6881-8. [Crossref]
- Zhang X, Liang M, Yang Z, et al. Deep Learning-Based Radiomics of B-Mode Ultrasonography and Shear-Wave Elastography: Improved Performance in Breast Mass Classification. Front Oncol 2020;10:1621. [Crossref] [PubMed]
- Fan M, Yuan W, Zhao W, et al. Joint Prediction of Breast Cancer Histological Grade and Ki-67 Expression Level Based on DCE-MRI and DWI Radiomics. IEEE J Biomed Health Inform 2020;24:1632-42. [Crossref] [PubMed]
- Yu H, Meng X, Chen H, et al. Correlation Between Mammographic Radiomics Features and the Level of Tumor-Infiltrating Lymphocytes in Patients With Triple-Negative Breast Cancer. Front Oncol 2020;10:412. [Crossref] [PubMed]
- Braman N, Prasanna P, Whitney J, et al. Association of Peritumoral Radiomics With Tumor Biology and Pathologic Response to Preoperative Targeted Therapy for HER2 (ERBB2)-Positive Breast Cancer. JAMA Netw Open 2019;2:e192561 [Crossref] [PubMed]
- Koh J, Lee E, Han K, et al. Three-dimensional radiomics of triple-negative breast cancer: Prediction of systemic recurrence. Sci Rep 2020;10:2976. [Crossref] [PubMed]
- Bae MS, Shin SU, Ryu HS, et al. Pretreatment MR Imaging Features of Triple-Negative Breast Cancer: Association with Response to Neoadjuvant Chemotherapy and Recurrence-Free Survival. Radiology 2016;281:392-400. [Crossref] [PubMed]