A nomogram for predicting overall-specific survival in thyroid cancer patients with total thyroidectomy: a SEER database analysis
Introduction
The incidence of thyroid cancer (TC) has increased by 211% from 1975 to 2013, with papillary TC (PTC) being the most common type of TC. Those aged 15–79 years are most commonly diagnosed with TC. Approximately 10% of PTC patients exhibit recurrences including lymph node recurrence and lung metastasis, or even death. South Korea had the greatest increase globally, from 12.2 cases per 100,000 in 1993–1997 to 59.9 cases per 100,000 in 2003–2007 (1). Although the prevalence rate of TC is significantly higher than the mortality rate, there has been a significant increase in the mortality rate since the 1980s, with an annual rate of 1.1% [95% confidence interval (CI): 0.6–1.6] (2).
The current TC evaluation system is the American Joint Committee on Cancer (AJCC) TNM staging system, which includes T staging (representing the size of the primary tumor and the scope of invasion), N staging (describing regional lymph node metastasis), and M staging (illustrating distant metastasis) in clinical practice (3). This can be used to predict the prognosis of patients and guide specific treatment methods. With the advancement of precision medicine, the TMN staging system cannot provide comprehensive treatment guidance and outcome prediction for a single patient because it lacks many other important clinical factors, such as age, sex, and tissue type, which can have a significant impact on patient outcomes.
The nomogram chart is a statistically-based graphical tool that plays a significant role in many fields, and is more commonly used for predictive modeling in clinical practice. A more accurate predictive model based on the analysis of big data expressed through graphics can be obtained, which is convenient for clinicians (4). At present, there is no tool for predicting a single TC patient after total thyroidectomy. In the present study, based on the Surveillance, Epidemiology, End Results (SEER) database (5), we analyzed the 3- or 5-year mortality of TC patients with total thyroidectomy, which can provide a basis for follow up and treatment of TC cases after surgery. Although, some nomograms have been constructed for predicting the survival of TC patients. However, our study is the first time to highlight the effect of race on thyroid cancer prognosis and we add it into the nomogram as an independent risk factor for predicting TC overall survival (OS).
We present the following article in accordance with the TRIPOD reporting checklist (available at https://dx.doi.org/10.21037/gs-21-520).
Methods
Data source
In the present study, eligible data were collected from the SEER dataset [The study was conducted in accordance with the Declaration of Helsinki (as revised in 2013)], which is the largest population-based cancer registry of the USA. The SEER database was launched in 1973 and is supported and managed by the National Cancer Institute. The database collects information on patient demographics, clinical data, tumor features, tumor stage, treatment, and overall survival (OS) for approximately 30% of the US population. The data input process of SEER is conducted by well-trained staff with strict quality control. Data from 1975 to 2009 were deleted due to a lack of adequate surgical stage information. The inclusion criteria for screening data in this study were as follows: (I) tumor located in the thyroid; (II) patients without detailed information about surgical stage, pathological type, marital status, survival time, and surgery; (III) patients diagnosed with TC only; (IV) known cause of mortality; and (V) definite AJCC tumor classification (7th edition).
Clinical characteristics
The following information was obtained from the SEER dataset: patient demographics, tumor characteristics, therapeutic patterns, as well as survival results. Age, race, year of diagnosis, and marital status were used in the analysis of patient demographics. Tumor stage, grade, histological classification, as well as surgery information, were analyzed for tumor characteristics. Patients were categorized into different groups according to age (<20, 21–30, 31–40, 41–50, 51–60, 61–70, and >70 years). Marital status was classified as married or unmarried. Race was categorized as white, black, and other (including Asian/Pacific Islanders, and American Indians/Alaskan natives). Tumor size also was divided into 4 groups (cm): <1.0, 1.1–2, 2.1–4.0, and >4.0. The AJCC 7th surgical-pathological staging classification was utilized for TNM stage. The ICD-0-3 site/histology validation list and the World Health Organization histological classification were employed to identify PTC (8050/3), follicular TC (8330/3), medullary TC (8510/3), and anaplastic TC (8021/3). These 4 types are the main pathological types of TC (6). Tumor grade was classified as G1–G4, indicating well differentiation, moderate differentiation, poor differentiation, and undifferentiation, respectively. Sex was classified as female and male. In addition, radioisotopes, chemotherapy, extrathyroidal extension, and lymphadenectomy were all divided into 2 groups, namely the treatment group and the untreated group.
Statistical analysis
Kaplan-Meier method was used to generate various stage with different therapies OS curves, along with log-rank test to test statistical significance. The relationship between TC and survival outcome was evaluated using a Cox proportional hazard regression model with a hazard ratio (HR) and corresponding 95% CI. Modeling a nomogram using R 3.5.0 software (https://www.r-project.org/) and SPSS version 22 (Chicago, IL, USA) was used for statistical analysis. P<0.05 was considered statistically significant.
Results
Patient characteristics
Figure 1 shows the process of data exclusion. In Table 1, a total of 11,614 postoperative patients with TC were randomly allocated to the modeling group and the verification group at a ratio of 7:3 by R software. Age, sex, race, tissue type, grade, TMN stage, tumor size, marital status, and different treatments were not statistically significant among these variables. Based on the distribution of the data, 7,405 (63.8%) were married and 4,209 (36.2%) were unmarried. Extrathyroidal extension had an important impact on TC recurrence. Additionally, 1,989 (17.1%) had the extrathyroidal extension and 9,625 (82.9%) did not have. In addition, 10,747 (92.5%) patients had PTC, 788 (6.8%) had FTC, 64 (0.6%) had MTC, and 15 (0.1%) had ATC. There were 8,942 (77%) females and 2,672 (23%) males.
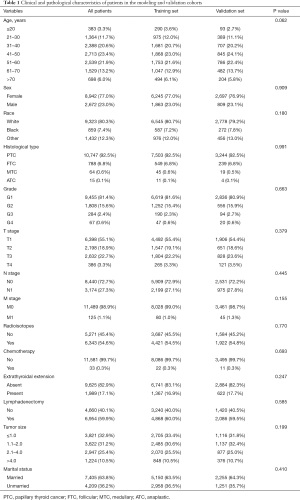
Full table
Single-factor and multifactor Cox analyses
Survival analysis of different treatment methods (lymphadenectomy and radiotherapy) in different stages found that TC patients with lymph node resection at early stage or radiotherapy at late stage had a better outcome on survival (Figure 2). In Table 2, from the univariate Cox analysis in OS, the differences of all variables were significant in statistics science except lymphadenectomy, radiotherapy and marital status (all P<0.05). Table 3 showed that most factors, with the exception of extrathyroidal extension and chemotherapy, were found to be statistically significant in the multivariate Cox analysis for OS. Although M staging did not reach statistical significance (P=0.11) in this study, it is still significant to evaluate tumor metastasis in clinic. Male patients with TC had worse OS compared with female patients (HR: 1.627, 95% CI: 1.160–2.282, P=0.005).

Full table
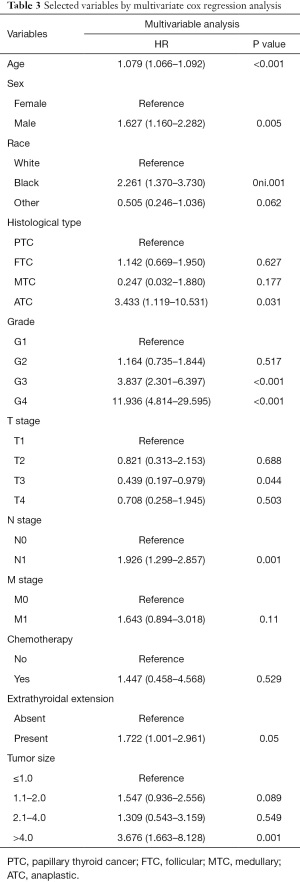
Full table
Nomogram development and verification
Using multivariate COX analysis, we selected age, histological type, grade, tumor size, stage TMN, and sex to construct the nomogram mode (see in Figure 3). Each factor corresponded to a specific score, and then the value of all the scores were added to obtain the corresponding 3- or 5-year survival rate. The C-index of the modeling group was 0.867, and the C-index of the verification group was 0.892. Figure 4 showed excellent calibration.
Discussion
In the present study, the characteristics of TC patients after total thyroidectomy in the SEER dataset were analyzed. We performed single-factor and multifactor analyses to screen out independent risk factors for nomogram modeling. Furthermore, internal verification further strengthened the feasibility of the model. Each patient had great individual differences. TC patients were no longer solely satisfied with the guidance of TMN staging on the prognosis and review of patients, because TMN staging did not include lots of important clinical factors, such as age, gender, tumor size, histology, etc. The model in the present study includes multiple factors to further provide detailed predictions and follow-up plans for each patient. We can also check out the proportion of the risk factors in nomogram to understand how to improve the survival rate of patients with TC, such as age and tumor grade. Which means early diagnosis and early treatment can reduce the first onset age and tumor grade, so that the patients can get longer survival.
A nomogram chart was used to assign points to each value level of each influencing factor according to the contribution of every factor in the model to the outcome variable, and then scores were added to obtain the total score (7). Finally, the predicted value of an individual’s outcome was calculated using the total score and the probability of the outcome. The nomogram transformed the complex regression equation into a visualized graph, making the results of the prediction model more readable, and facilitating the evaluation of the patient. This model is receiving increasing attention in medical research and clinical practice because of its intuitive and easy-to-understand characteristics via the simple pictures. The nomogram chart had been used to predict a variety of diseases, such as breast cancer (8,9), prostate cancer (10,11), and colon cancer (12,13).
PTC, FTC, ATC, and MTC are the 4 main pathological subtypes related to the prognosis of TC. Whether in the thyroid itself or in local regional lymph nodes, PTC was a prognostic factor, which meant an increased risk of recurrence. Namely, we might need to operate a new model and predict OS (14,15). According to the manifestation and treatment of the disease, it was found that the most important factor for prognosis was patient age. Compared with elderly patients with the same disease stage, younger patients had a lower recurrence rate and disease-specific mortality (16). The incidence of TC in both men and women has shown a steady increase in most countries. At the same time, the incidence of TC among women is higher than for men (17). As a result, tumor size, grade, and TMN stage all were vital prognostic factors (18,19). In the present study, we also found that age, histological type, grade, tumor size, TMN stage, and sex were independent risk factors for OS according to the multivariate Cox regression.
The advantages of the present study include that it is a population-based study with a large sample size and adjustment for the patient’s clinical demographic characteristics. However, the study has some limitations. First, we built a model from retrospective research in the SEER database. This process has all the effects of the bias of the retrospective research. Second, retrospective analysis limited the choice of variables. For example, calcitonin plays an essential role in predicting postoperative TC and the role of calcitonin in modeling is not analyzed in this article (20). Then, the research was only based on a US population, and there was a lack of research on data from other countries. Finally, and most importantly, the study lacked external verification. In the future, it will be necessary to conduct prospective verification on a larger patient population to further improve authenticity of the predictive model in our study and to ensure clinical practicability.
We established a postoperative prediction model for TC through a variety of variables, providing a basis for different predictions and follow-up plans for each patient.
Acknowledgments
Funding: This study was funded by Ningbo Natural Science Foundation of China under Grant No. 2019A610274; Ningbo Natural Science Foundation of China under Grant No. 202003N4250; Ningbo Natural Science Foundation of China under Grant No. 2019A610266.
Footnote
Reporting Checklist: The authors have completed the TRIPOD reporting checklist. Available at https://dx.doi.org/10.21037/gs-21-520
Conflicts of Interest: All authors have completed the ICMJE uniform disclosure form (available at https://dx.doi.org/10.21037/gs-21-520). The authors have no conflicts of interest to declare.
Ethical Statement: The authors are accountable for all aspects of the work in ensuring that questions related to the accuracy or integrity of any part of the work are appropriately investigated and resolved. The study was conducted in accordance with the Declaration of Helsinki (as revised in 2013).
Open Access Statement: This is an Open Access article distributed in accordance with the Creative Commons Attribution-NonCommercial-NoDerivs 4.0 International License (CC BY-NC-ND 4.0), which permits the non-commercial replication and distribution of the article with the strict proviso that no changes or edits are made and the original work is properly cited (including links to both the formal publication through the relevant DOI and the license). See: https://creativecommons.org/licenses/by-nc-nd/4.0/.
References
- Vaccarella S, Dal Maso L, Laversanne M, et al. The Impact of Diagnostic Changes on the Rise in Thyroid Cancer Incidence: A Population-Based Study in Selected High-Resource Countries. Thyroid 2015;25:1127-36. [Crossref] [PubMed]
- Lim H, Devesa SS, Sosa JA, et al. Trends in Thyroid Cancer Incidence and Mortality in the United States, 1974-2013. JAMA 2017;317:1338-48. [Crossref] [PubMed]
- Tam S, Boonsripitayanon M, Amit M, et al. Survival in Differentiated Thyroid Cancer: Comparing the AJCC Cancer Staging Seventh and Eighth Editions. Thyroid 2018;28:1301-10.
- Iasonos A, Schrag D, Raj GV, et al. How to build and interpret a nomogram for cancer prognosis. J Clin Oncol 2008;26:1364-70. [Crossref] [PubMed]
- Doll KM, Rademaker A, Sosa JA. Practical Guide to Surgical Data Sets: Surveillance, Epidemiology, and End Results (SEER) Database. JAMA Surg 2018;153:588-9. [Crossref] [PubMed]
- Torre LA, Bray F, Siegel RL, et al. Global cancer statistics, 2012. CA Cancer J Clin 2015;65:87-108. [Crossref] [PubMed]
- Park SY. Nomogram: An analogue tool to deliver digital knowledge. J Thorac Cardiovasc Surg 2018;155:1793. [Crossref] [PubMed]
- Han L, Zhu Y, Liu Z, et al. Radiomic nomogram for prediction of axillary lymph node metastasis in breast cancer. Eur Radiol 2019;29:3820-9. [Crossref] [PubMed]
- Wang J, Li Y, Fu W, et al. Prognostic nomogram based on immune scores for breast cancer patients. Cancer Med 2019;8:5214-22. [Crossref] [PubMed]
- Brockman JA, Alanee S, Vickers AJ, et al. Nomogram Predicting Prostate Cancer-specific Mortality for Men with Biochemical Recurrence After Radical Prostatectomy. Eur Urol 2015;67:1160-7. [Crossref] [PubMed]
- Gandaglia G, Ploussard G, Valerio M, et al. A Novel Nomogram to Identify Candidates for Extended Pelvic Lymph Node Dissection Among Patients with Clinically Localized Prostate Cancer Diagnosed with Magnetic Resonance Imaging-targeted and Systematic Biopsies. Eur Urol 2019;75:506-14. [Crossref] [PubMed]
- Frasson M, Flor-Lorente B, Rodríguez JL, et al. Risk Factors for Anastomotic Leak After Colon Resection for Cancer: Multivariate Analysis and Nomogram From a Multicentric, Prospective, National Study With 3193 Patients. Ann Surg 2015;262:321-30. [Crossref] [PubMed]
- Sjoquist KM, Renfro LA, Simes RJ, et al. Personalizing Survival Predictions in Advanced Colorectal Cancer: The ARCAD Nomogram Project. J Natl Cancer Inst 2018;110:638-48. [Crossref] [PubMed]
- Wada N, Duh QY, Sugino K, et al. Lymph node metastasis from 259 papillary thyroid microcarcinomas: frequency, pattern of occurrence and recurrence, and optimal strategy for neck dissection. Ann Surg 2003;237:399-407. [Crossref] [PubMed]
- Grogan RH, Kaplan SP, Cao H, et al. A study of recurrence and death from papillary thyroid cancer with 27 years of median follow-up. Surgery 2013;154:1436-46; discussion 1446-7. [Crossref] [PubMed]
- Rossi RL, Majlis S, Rossi RM. Thyroid cancer. Surg Clin North Am 2000;80:571-80. [Crossref] [PubMed]
- La Vecchia C, Malvezzi M, Bosetti C, et al. Thyroid cancer mortality and incidence: a global overview. Int J Cancer 2015;136:2187-95. [Crossref] [PubMed]
- Wang Z, Zhang Q, Ye H, et al. Tumor size is an independent predictor of mortality risk in differentiated thyroid cancer patients with t4 disease. Endocr Pract 2020;26:499-507. [Crossref] [PubMed]
- Wen Q, Yu Y, Yang J, et al. Development and Validation of a Nomogram for Predicting Survival in Patients with Thyroid Cancer. Med Sci Monit 2019;25:5561-71. [Crossref] [PubMed]
- Meijer JA, le Cessie S, van den Hout WB, et al. Calcitonin and carcinoembryonic antigen doubling times as prognostic factors in medullary thyroid carcinoma: a structured meta-analysis. Clin Endocrinol (Oxf) 2010;72:534-42. [Crossref] [PubMed]
(English Language Editor: R. Scott)