Diagnostic accuracy and prognostic value of three-dimensional electrical impedance tomography imaging in patients with breast cancer
Introduction
The incidence of breast cancer has been increasing around the world. In some areas, breast cancer occupies the top cause of death from female malignant tumors, and the population is getting younger (1). Early detection is an important factor affecting prognosis in breast cancer patients. Although conventional imaging methods for early detection of breast cancer, such as ultrasonography and mammography, have made great progress in diagnostic accuracy in recent years, limitations associated with current technologies still exist (2). Some patients are reluctant to undergo mammography due to the painful procedure and X-ray radiation exposure. Further, mammography’s two-dimensional (2D) images show a high number of false negatives, especially for dense breasts (3). For ultrasonography, breast lesions smaller than 1 cm and microscopic calcifications are not easy to display. Moreover, ultrasound results are largely dependent on the experience of the operator (4).
Three-dimensional electrical impedance tomography (3D EIT) is a novel, non-invasive, radiation-free imaging technology based on detecting differences in electrical conductivity between malignant and non-malignant tissues (5). This distinction is attributed to increased cellular water and salt content, altered membrane permeability, and packing density, and cell orientation changes (6). Compared with 2D EIT, 3D EIT provides a 3D conductivity distribution and image reconstruction algorithm, which improves the localization of lesions without multiple investigations (7). In particular, 3D EIT gives information regarding the depth of the detected abnormalities and decreases the masking of deeper objects (8).
Recent advances in computational tools for bioimpedance quantification have allowed EIT to become faster, more reliable, and cheaper (9,10). Over the past several years, few studies have been published on 3D EIT for clinical diagnosis and outcomes for patients with malignant breast lesions. In this study, we aimed to: (I) identify the electrical impedance characteristics of 3D EIT imaging in benign and malignant breast lesions, (II) assess the diagnostic performance of 3D EIT compared with other conventional imaging modalities, and (III) evaluate the prognostic value of 3D EIT associated with other clinicopathological features in patients with breast cancer.
We present the following article in accordance with the STARD reporting checklist (available at https://dx.doi.org/10.21037/gs-21-348).
Methods
Subjects and study design
A total of 645 patients with suspicious breast lesions [Breast Imaging Reporting and Data System (BI-RADS) III, IV, V] between January 2016 and December 2016 at Beijing Chao-Yang Hospital were retrospectively enrolled in this study. The diagnoses of breast lesions were confirmed independently by two pathologists who reviewed pathological slides from biopsies or resected tissues. Inclusion criteria were as follows: (I) 3D EIT was performed before tissue biopsy, or surgical resection, (II) all suspicious breast lesions were pathologically confirmed, and (III) clinical follow-up data were available. Patients who received neoadjuvant chemotherapy before surgery were excluded from this study. The following covariates were measured during the baseline clinical exam and survey: age, menopause status, cancer type, tumor size, vessel embolus, perineural invasion, estrogen receptor (ER) status, progesterone receptor (PR) status, human epidermal growth factor receptor 2 (HER2) status, lymph node metastasis (LNM), tumor stage, and outcomes. The minimum duration of follow-up was 36 months (median, 55 months; range, 36–62 months). All eligible patients provided written informed consent before taking part. This study was approved by the Ethics Review Board of Affiliated Beijing Chao-Yang Hospital, Capital Medical University. The study was conducted in accordance with the Declaration of Helsinki (as revised in 2013).
3D EIT imaging protocol
EIT examinations were performed using electrical impedance computer mammograph MEIK v. 5.6, developed by the Institute of Radio Engineering and Electronics, Russian Academy of Sciences (11). Most participants were examined in the supine position. The breast was levelled frontally, with a bolster placed under the corresponding shoulder and the arm placed behind the neck. When mammary glands were moderately sized or less, they could be examined in a sitting position. Before the procedure, the bilateral breasts and wrists were moistened evenly with water without leaving any water drops. Two gel electrodes were attached to the patient’s opposite wrist at a distance of 1.5–2 cm from each other. The 256-electrode panel was placed against the breast so that the laser marker was positioned on the nipple. A weak alternative current (0.5 mA) with a frequency of 50 kHz was applied to the breast under examination, and 3D conductivity distribution of electric potential was recorded. The signals were then reprocessed into electronic impedance images (12). The sensor was fixed on the mammary gland for not more than 35 seconds. In particular, a measuring system and reconstruction algorithm for breast tissue imaging and breast cancer detection were used. The reconstruction results consisted of electrical impedance images of 7 cross-sectional slices parallel to the plane of the electrodes. The depth of each slice was 8 mm, starting 4 mm from the surface. Each breast was scanned twice to ensure accurate imaging.
Image analysis
The results of 3D EIT imaging were analyzed independently based on visual interpretation and quantitative evaluation of breast tissues and according to the main diagnostic criteria previously reported from Russia (13-15). The breast’s structure, contour, focal changes of electrical conductivity, and bilateral breast conductivity differences were evaluated to analyze the possibility of pathological changes in the patient’s breast. The resulting electrical impedance image represented tissue conductivity along a greyscale from dark to light, indicating low to high conductivity. The reporting system then converted the electrical impedance score into commonly used BI-RADS classification for further interpretation. Normal breasts were characterized by smooth contours of conductivity and an absence of focal abnormalities in the reconstruction image. However, malignant lesions exhibited different electrochemical properties and a different distribution of electrical charges.
Statistical analysis
Sensitivity, specificity, positive and negative predictive value, and diagnostic accuracy were calculated with 95% confidence intervals (CIs). Categorical variables were compared using the Pearson chi-square test or Fisher’s exact test. Continuous variables were compared between groups using independent sample t-test or Mann-Whitney U-test. Progression-free survival (PFS) and overall survival (OS) were calculated using Kaplan-Meier survival analysis. PFS was defined as the time interval between the date of operation and disease progression or death; OS was defined as the time from the date of operation to death from all causes. Univariate and multivariate Cox proportional hazard models were applied to determine whether 3D EIT and other clinicopathological variables were significantly associated with PFS and OS. The relationships between variables and outcomes were summarized by hazard ratios (HRs) and 95% CIs. All statistical analyses were performed using SPSS software (version 20.0). A P value <0.05 was considered statistically significant.
Results
Basic characteristics of the patients
The mean age of the enrolled patients was 40.7±5.2 years, and 198 of the patients (30.7%) were postmenopausal. Table 1 summarizes the histopathological results for all patients. Of 645 enrolled lesions, 272 (42.2%) were malignant, and 373 (57.8%) were benign. The most common malignancies were invasive ductal carcinoma (IDC, n=201), ductal carcinoma in situ (DCIS, n=35), invasive lobular carcinoma (n=25), and mucinous cancer (n=11). Among them, positive 3D EIT imaging was found in 218 patients involving 31 cases of DCIS, 179 cases of invasive cancer, and 8 cases of mucinous cancer. The remaining 54 cases showed negative EIT imaging. Predominant benign lesions were fibroadenomas (n=148), cysts (n=42), papilloma (n=57), and hyperplasia (n=126). Mean lesion size was 18.6±8.4 mm (benign, 12.5±7.3 mm; malignant, 23.2±13.1 mm).
Table 1
Breast lesions | N |
---|---|
Benign (n=373) | |
Cysts | 42 |
Fibroadenoma | 148 |
Papilloma | 57 |
Hyperplasia | 126 |
Malignant (n=272) | |
Ductal carcinoma |
35 |
Invasive ductal carcinoma | 201 |
Invasive lobular carcinoma | 25 |
Mucinous cancer | 11 |
3D EIT and diagnostic value
The accuracy, sensitivity, specificity, positive predictive value, and negative predictive value of 3D EIT, ultrasound, and mammography diagnosis of breast lesions are shown in Table 2. There were no significant differences in the diagnostic accuracy, sensitivity, or specificity between 3D EIT and mammography, ultrasound, or combined mammography and ultrasound (P>0.05). The accuracy and specificity of 3D EIT combined with ultrasound in the diagnosis of breast lesions were higher than ultrasound alone (89.6% vs. 80.1% and 88.5% vs. 71.4%, respectively). Furthermore, the diagnostic accuracy, sensitivity, and specificity of 3D EIT combined with mammography were higher than mammography alone (86.0% vs. 76.8%, 86.4% vs. 74.3%, and 85.8% vs. 75.1%, respectively).
Table 2
Methods | Accuracy | Sensitivity | Specificity | PPV | NPV |
---|---|---|---|---|---|
3D EIT | 77.2% | 80.1% | 75.1% | 70.1% | 83.8% |
MG | 76.8% | 74.3% | 78.9% | 70.8% | 81.3% |
US | 79.3% | 90.6% | 71.4% | 68.9% | 91.5% |
MG + US | 84.7% | 91.5% | 79.8% | 76.5% | 92.9% |
P1 | 0.953 | 0.573 | 0.688 | 0.942 | 0.799 |
P2 | 0.762 | 0.351 | 0.662 | 0.887 | 0.466 |
P3 | 0.312 | 0.339 | 0.618 | 0.512 | 0.414 |
P1 indicates the value of MG in comparison with 3D EIT; P2 indicates the value of US in comparison with 3D EIT; P3 indicates the value of MG + US in comparison with 3D EIT. MG, mammography; US, ultrasound; PPV, positive predictive value; NPV, negative predictive value; 3D EIT, three-dimensional electrical impedance tomography.
We further conducted multiple subgroup analyses. In the group of calcified lesions (n=94, 27 carcinomas), 3D EIT showed moderate sensitivity and specificity of 72.3% and 75.2%, respectively. For 143 lesions smaller than 1 cm, 3D EIT demonstrated sensitivity and specificity of 92.8% and 70.5%, respectively. 3D EIT showed a better performance with sensitivity and specificity of 79.5% and 73.6% for lesions in the dense breast than mammography with 67.2% and 63.4%, respectively. The sensitivity of 3D EIT in IDC diagnosis was 89.1% (179/201), compared to DCIS with 88.6% (31/35). Among the 54 false-negative malignant lesions, 1/3 of the cases were located behind the nipple.
3D EIT and outcomes
Table 3 shows the characteristics of 3D electrical impedance images in different types of benign and malignant breast lesions. We found a significant difference in the electrical conductivity index between benign and malignant lesions, regardless of the affected breast and lesion-focus area. Based on image characteristics, including contour, anatomy, hypoimpedance area, hyperimpedance infiltration, and electrical conductivity index, the positive 3D EIT breast cancer images were classified into 3 different types, including Ia [non-complicated breast cancer (NCBC), 62 cases, Figure 1], Ib [complicated breast cancer (CBC), 131 cases, Figure 2], and Ic [edematous-infiltrative breast cancer (EIBC), 25 cases, Figure 3]. Ia-type imaging was characterized by focal abnormalities, which appeared as a “bright white spot” of high conductivity (0.90±0.03). Ib type was characterized by focal abnormalities accompanied by changed anatomy and hyperimpedance infiltration of surrounding tissues. Ic type mainly manifested as large-area hyperimpedance infiltration (electrical conductivity, 0.15±0.12) accompanied by large differences in bilateral electrical conductivity, which was associated with bigger tumor size (P<0.001), higher tumor stage (P<0.001), and LNM (P=0.012). However, there were no significant differences between 3D EIT imaging classification and other clinicopathological factors such as age, menopause status, tumor type, vessel embolus, perineural invasion, ER status, PR status, HER2 status (Table 4).
Table 3
Characteristics | Subcategories | Normal | Benign | Ia (NCBC) | Ib (CBC) | Ic (EIBC) |
---|---|---|---|---|---|---|
Contour | Deformation | – | – | 0% | 43% | 12% |
Hyperimpedance | – | – | 9% | 72%* | 100% | |
Anatomy | Retained | 100% | 100% | 100% | 15%** | 0% |
Changed | – | – | – | 85% | 100% | |
Hypoimpedance area (electroconductivity index >0.95) | Distinguished on the image | – | – | 60% | 25%** | – |
Not present | 100% | 100% | 40% | 75% | – | |
Hyperimpedance infiltration | Present | – | – | 9% | 83%** | 100% |
Not present | – | – | 91% | 17% | – | |
Breast electroconductivity index | Affected breast | – | 0.43±0.08 | 0.52±0.11 | 0.32±0,12** | 0.15±0.12** |
Healthy breast | 0.46±0.13 | 0.45±0.12 | 0.48±0.10 | 0.45±0.09 | 0.46±0.09 | |
Lesion focus (area) | – | 0.50±0.15 | 0.90±0.03 | 0.65±0.12** | – |
*, reliability of discrepancy between this data and the data of the previous column P<0.05; **, reliability of discrepancy between this data and the data of the previous column P<0.01. Ia: the noncomplicated nodular form of breast cancer; Ib: the nodular form of breast cancer, involving edema, infiltration or abnormalities of vascularization; Ic: edematous-infiltrative form of breast cancer. NCBC, non-complicated breast cancer; CBC, complicated breast cancer; EIBC, edematous-infiltrative breast cancer.
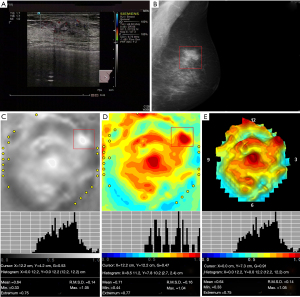
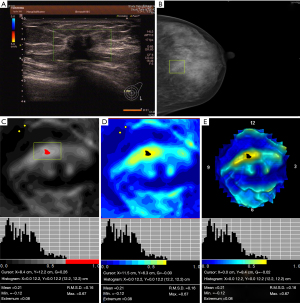
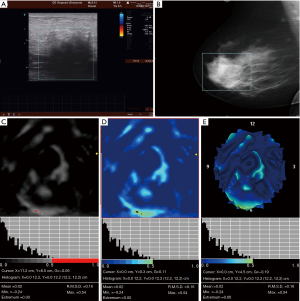
Table 4
Variables | Ia (NCBC) | Ib (CBC) | Ic (EIBC) | P value |
---|---|---|---|---|
Age (years) | 0.414 | |||
≤40 | 28 | 47 | 11 | |
>40 | 34 | 84 | 14 | |
Menopause | 0.454 | |||
Yes | 30 | 51 | 11 | |
No | 32 | 80 | 14 | |
Tumor type | 0.184 | |||
Ductal carcinoma |
15 | 14 | 2 | |
Invasive cancer | 47 | 110 | 22 | |
Mucinous cancer | 0 | 7 | 1 | |
Tumor size (cm) | <0.001 | |||
<2 | 42 | 23 | 0 | |
2–5 | 18 | 86 | 3 | |
>5 | 2 | 22 | 22 | |
Vessel embolus | 0.796 | |||
Present | 14 | 35 | 7 | |
Absent | 48 | 96 | 18 | |
Perineural invasion | 0.693 | |||
Present | 10 | 28 | 5 | |
Absent | 52 | 103 | 20 | |
ER | 0.557 | |||
Positive | 35 | 64 | 14 | |
Negative | 27 | 67 | 11 | |
PR | 0.324 | |||
Positive | 26 | 70 | 12 | |
Negative | 36 | 61 | 13 | |
HER2 | 0.748 | |||
Positive | 20 | 39 | 6 | |
Negative | 42 | 92 | 19 | |
Lymph nodes metastasis | 0.012 | |||
Present | 24 | 72 | 18 | |
Absent | 38 | 59 | 7 | |
Tumor stage | <0.001 | |||
Stage I | 38 | 22 | 0 | |
Stage II | 20 | 89 | 6 | |
Stage III | 4 | 20 | 19 |
NCBC, non-complicated breast cancer; CBC, complicated breast cancer; EIBC, edematous-infiltrative breast cancer; 3D EIT, three-dimensional electrical impedance tomography; ER, estrogen receptor; PR, progesterone receptor; HER2, human epidermal growth factor receptor 2.
After a median follow-up period of 55 months, we found that there was no significant difference in PFS (P=0.65) and OS (P=0.24) between positive and negative EIT groups (Figure 4). Disease progression in the positive 3D EIT group was reported in 38 patients (17.4%), among whom 24 had died. Ic-type breast cancer was significantly associated with shorter 5-year PFS (P=0.006) and 5-year OS (P=0.025) compared with the findings of Ia and Ib imaging. Multivariate analysis showed significance differences in PFS and OS by 3D EIT classification (PFS-HR 2.836, 95% CI: 1.555, 5.172, P=0.012; OS-HR 2.399, 95% CI: 1.035, 5.564, P=0.041), ER status (PFS-HR 0.279, 95% CI: 0.132, 0.590, P=0.004; OS-HR 0.204, 95% CI: 0.074, 0.561, P=0.008), HER2 status (PFS-HR 2.371, 95% CI: 1.226, 4.584, P=0.010; OS-HR 2.746, 95% CI: 1.208, 6.243, P=0.016), and tumor stage (PFS-HR 2.181, 95% CI: 1.336, 4.373, P=0.009; OS-HR 1.648, 95% CI: 1.110, 4.879, P=0.037) (Table 5).
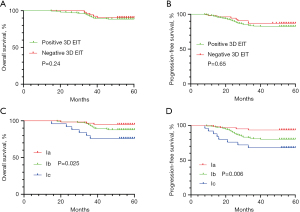
Table 5
Variables | PFS | OS | |||||||||
---|---|---|---|---|---|---|---|---|---|---|---|
Univariate | Multivariate | Univariate | Multivariate | ||||||||
HR (95% CI) | P value | HR (95% CI) | P value | HR (95% CI) | P value | HR (95% CI) | P value | ||||
3D EIT classification (Ic/Ib/Ia) | 2.328 (1.374, 3.944) | 0.006 | 2.836 (1.555, 5.172) | 0.012 | 2.109 (1.088, 4.090) | 0.025 | 2.399 (1.035, 5.564) | 0.041 | |||
Age (≤40/>40 years) | 1.180 (0.611, 2.282) | 0.622 | – | – | 2.602 (0.972, 6.970) | 0.057 | – | – | |||
Tumor size (>5/2–5/<2 cm) | 1.283 (0.815, 2.043) | 0.254 | – | – | 1.512 (0.882, 2.592) | 0.133 | – | – | |||
Vessel embolus (present/absent) | 1.593 (0.815, 3.115) | 0.173 | – | – | 2.136 (0.949, 4.811) | 0.067 | – | – | |||
Perineural invasion (present/absent) | 1.119 (0.513, 2.442) | 0.777 | – | – | 1.411 (0.560, 3.556) | 0.465 | – | – | |||
ER (positive/negative) | 0.374 (0.191, 0.731) | 0.001 | 0.279 (0.132, 0.590) | 0.004 | 0.247 (0.098, 0.621) | 0.002 | 0.204 (0.074, 0.561) | 0.008 | |||
PR (positive/negative) | 0.558 (0.291, 1.070) | 0.079 | – | – | 0.859 (0.481, 2.554) | 0.158 | – | – | |||
HER2 (positive/negative) | 2.519 (1.314, 4.829) | 0.005 | 2.371 (1.226, 4.584) | 0.010 | 2.326 (1.502, 3.600) | <0.001 | 2.746 (1.208, 6.243) | 0.016 | |||
LNM (positive/negative) | 2.304 (1.215, 4.369) | 0.011 | 1.511 (0.768, 2.970) | 0.232 | 2.986 (1.306, 6.824) | 0.015 | 1.786 (0.742, 4.300) | 0.196 | |||
Tumor stage (III/II/I) | 2.267 (1.583, 3.012) | 0.001 | 2.181 (1.336, 4.373) | 0.009 | 1.891 (1.322, 5.023) | 0.012 | 1.648 (1.110, 4.879) | 0.037 |
OS, overall survival; PFS, progression free survival; HR, hazard ratio; 95% CI, 95% confidence interval; 3D EIT, three-dimensional electrical impedance tomography; ER, estrogen receptor; PR, progesterone receptor; HER2, human epidermal growth factor receptor 2; LNM, lymph node metastasis.
Discussion
The application of 3D EIT technology to detect human breast disease has grown in recent years (16,17). The benefits of 3D EIT include speed, low cost, no radiation, and the advantages of 3D visualization, quantitative evaluation, and functional imaging (18). The pathophysiology of breast cancer might be associated with changes in neoplastic cell membrane permeability, which can be revealed through EIT technology (19). An essential difference between functional imaging and structural imaging is that changes in conductivity are visible in early breast cancer, providing a new imaging method for early detection and prospective prediction of breast cancer.
At present, the role of 3D EIT imaging classification and its prognostic value for breast cancer is still limited, and the literature is scarce. This study initially evaluated the clinical detection performance of 3D EIT technology through clinical trials and compared the diagnostic efficiency of traditional breast detection technologies, such as ultrasound and mammography. We found that diagnostic accuracy, specificity, and positive predictive value of 3D EIT, ultrasound, and mammography were not significantly different. However, the accuracy, sensitivity, and specificity of 3D EIT combined with ultrasound or mammography were higher than ultrasound or mammography alone. Therefore, 3D EIT is a good complement to conventional breast cancer detection methods.
Similar results were reported by Raneta et al. (20), who demonstrated that the use of EIT in addition to mammography or ultrasound could improve the sensitivity of these methods and increase the rate of early detection of breast cancer. The sensitivity of ultrasound in the study by Raneta et al. was relatively high because most of the lesions in this group appeared as lumps. Our study, 3D EIT, showed better performance with sensitivity and specificity for screening patients with dense breasts where conventional mammography was the least accurate. As 3D EIT provides more data for quantitative analysis, it relies less on the doctor’s experience, thereby reducing human factors that affect the diagnosis accuracy. Moreover, we demonstrated that 3D EIT had good sensitivity in patients with a 10-mm or smaller tumor size. Diebold et al. also recommended the application of 3D EIT in small tumors, with 85% of lesions <10 mm in size correctly diagnosed (21).
It is worth mentioning that 1/3 of false-negative malignant cases were located in the nipple-areola area. We found that this area showed high conductivity distribution, which was difficult to distinguish from the tumor. Malich et al. also found that the nipple always showed as a bright signal (22). There might be an accumulation of milk or other secretions in the lactiferous duct, resulting in high conductivity in the nipple-areola area (23). For further differential diagnosis, our experience was to (I) assess whether the patient had galactorrhea or nipple discharge; (II) observe whether the surrounding tissue was compressed, deformed, or infiltrated hyperimpedance; and (III) compare the morphology and conductivity distribution of the bilateral nipple-areola areas.
Similar to the findings of Russian studies (24), 3D EIT breast cancer imaging was classified into 3 different types, including Ia, Ib, and Ic, with each subgroup comprising different image characteristics. We compared the 3 imaging classifications with the clinicopathological characteristics of the enrolled breast cancer patients and found that the Ic subtype was significantly associated with larger tumor size, higher tumor stage (stage III), and more LNM. Electrical impedance mammograms from different groups had clear visual distinctions and statistically significant differences in mammary gland conductivity. We suspected that the differences in the imaging of these 3 types of breast cancer might be due to the difference in the surrounding tissues of breast cancer infiltration. NCBC might manifest as surrounding adipose tissue infiltration, while CBC and EIBC might show surrounding breast gland tissue infiltration.
Based on the results, we suspected that different classifications might be related to the prognosis of breast cancer patients. As expected, breast cancer classification of 3D EIT imaging, especially for Ic type, was found to be an independent predictor of a higher rate of disease progression and a lower survival rate. Conversely, Ia type was predictive of a more favorable outcome. To the best of our knowledge, this is the first study to investigate the prognostic role of 3D EIT imaging classification in patients with breast cancer. This may lead to a broader application of this technique.
Although this was a large-scale study, there were still some limits. First, available subjects were limited to a retrospective single-center study. Clinical trials with a prospective design and a large multi-center cohort are needed to expand the use of 3D EIT in the early screening of breast cancer. In addition, we conducted screening and interpreted EIT images under the guidance of Russian experts in the study. More breast surgeons and radiologists should be trained to conduct screening and interpretation of the images.
In conclusion, 3D EIT had good diagnostic performance and prognostic value for estimating the risk of disease progression and survival rates in breast cancer patients. In the future, applying artificial intelligence and deep learning networks to 3D EIT imaging of breast cancer will improve diagnostic efficiency.
Acknowledgments
We thank the team of Russian professor Alexander Y. Karpov from Clinical Hospital No. 9 for providing technical guidance and imaging evaluation support.
Funding: This study was supported by the Project of National Natural Science Foundation of China (grant number 81702587) and the Project of the Science and Technology Commission of Beijing Municipality (grant number Z181100002218001).
Footnote
Reporting Checklist: The authors have completed the STARD reporting checklist. Available at https://dx.doi.org/10.21037/gs-21-348
Data Sharing Statement: Available at https://dx.doi.org/10.21037/gs-21-348
Conflicts of Interest: All authors have completed the ICMJE uniform disclosure form (available at https://dx.doi.org/10.21037/gs-21-348). The authors have no conflicts of interest to declare.
Ethical Statement: The authors are accountable for all aspects of the work in ensuring that questions related to the accuracy or integrity of any part of the work are appropriately investigated and resolved. The study was conducted in accordance with the Declaration of Helsinki (as revised in 2013). The study was approved by institutional ethics board of Beijing Chaoyang Hospital (NO.: 2020-3-16-13) and the participants gave informed consent before taking part.
Open Access Statement: This is an Open Access article distributed in accordance with the Creative Commons Attribution-NonCommercial-NoDerivs 4.0 International License (CC BY-NC-ND 4.0), which permits the non-commercial replication and distribution of the article with the strict proviso that no changes or edits are made and the original work is properly cited (including links to both the formal publication through the relevant DOI and the license). See: https://creativecommons.org/licenses/by-nc-nd/4.0/.
References
- Tao Z, Shi A, Lu C, et al. Breast Cancer: Epidemiology and Etiology. Cell Biochem Biophys 2015;72:333-8. [Crossref] [PubMed]
- Badu-Peprah A, Adu-Sarkodie Y. Accuracy of clinical diagnosis, mammography and ultrasonography in preoperative assessment of breast cancer. Ghana Med J 2018;52:133-9. [Crossref] [PubMed]
- Fiorica JV. Breast Cancer Screening, Mammography, and Other Modalities. Clin Obstet Gynecol 2016;59:688-709. [Crossref] [PubMed]
- Geisel J, Raghu M, Hooley R. The Role of Ultrasound in Breast Cancer Screening: The Case for and Against Ultrasound. Semin Ultrasound CT MR 2018;39:25-34. [Crossref] [PubMed]
- Cherepenin V, Karpov A, Korjenevsky A, et al. A 3D electrical impedance tomography (EIT) system for breast cancer detection. Physiol Meas 2001;22:9-18. [Crossref] [PubMed]
- Malich A, Fritsch T, Anderson R, et al. Electrical impedance scanning for classifying suspicious breast lesions: first results. Eur Radiol 2000;10:1555-61. [Crossref] [PubMed]
- Cherepenin VA, Karpov AY, Korjenevsky AV, et al. Three-dimensional EIT imaging of breast tissues: system design and clinical testing. IEEE Trans Med Imaging 2002;21:662-7. [Crossref] [PubMed]
- Zain NM, Chelliah KK. Breast imaging using electrical impedance tomography: correlation of quantitative assessment with visual interpretation. Asian Pac J Cancer Prev 2014;15:1327-31. [Crossref] [PubMed]
- Zuluaga-Gomez J, Zerhouni N, Al Masry Z, et al. A survey of breast cancer screening techniques: thermography and electrical impedance tomography. J Med Eng Technol 2019;43:305-22. [Crossref] [PubMed]
- Murillo-Ortiz B, Hernández-Ramírez A, Rivera-Villanueva T, et al. Monofrequency electrical impedance mammography (EIM) diagnostic system in breast cancer screening. BMC Cancer 2020;20:876. [Crossref] [PubMed]
- Xu F, Li MX, Jusko HZ, et al. Utilisation of electrical impedance tomography and/or ultrasound and mammography in breast disease diagnosis: a controlled study. Zhonghua Yi Xue Za Zhi 2017;97:1391-5. [PubMed]
- Daglar G, Senol K, Yakut ZI, et al. Effectiveness of breast electrical impedance imaging for clinically suspicious breast lesions. Bratisl Lek Listy 2016;117:505-10. [Crossref] [PubMed]
- Karpov A, Korotkova M, Tsofin Y, et al. Electrical impedance potential mammography for visualization of objects (electrochemical tests). J Phys Conf Ser 2010;224:012032 [Crossref]
- Karpov A, Korotkova M. Diagnostic criteria for mass lesions differentiating in electrical impedance mammography. J Phys Conf Ser 2013;434:012053 [Crossref]
- Karpov A, Kolobanov A, Korotkova M. Diagnostic System in Electrical Impedance Mammography: Background. In: Malik AM. editor. New Perspectives in Breast Imaging. IntechOpen, 2017. doi:
10.5772/intechopen.69195 . Available online: https://www.intechopen.com/chapters/5591010.5772/intechopen.69195 - Murphy EK, Mahara A, Halter RJ. Absolute Reconstructions Using Rotational Electrical Impedance Tomography for Breast Cancer Imaging. IEEE Trans Med Imaging 2017;36:892-903. [Crossref] [PubMed]
- Vairavan R, Abdullah O, Retnasamy PB, et al. A Brief Review on Breast Carcinoma and Deliberation on Current Non Invasive Imaging Techniques for Detection. Curr Med Imaging Rev 2019;15:85-121. [Crossref] [PubMed]
- Akhtari-Zavare M, Latiff LA. Electrical Impedance Tomography as a Primary Screening Technique for Breast Cancer Detection. Asian Pac J Cancer Prev 2015;16:5595-7. [Crossref] [PubMed]
- Zheng B, Lederman D, Sumkin JH, et al. A preliminary evaluation of multi-probe resonance-frequency electrical impedance based measurements of the breast. Acad Radiol 2011;18:220-9. [Crossref] [PubMed]
- Raneta O, Bella V, Bellova L, et al. The use of electrical impedance tomography to the differential diagnosis of pathological mammographic/sonographic findings. Neoplasma 2013;60:647-54. [Crossref] [PubMed]
- Diebold T, Jacobi V, Scholz B, et al. Value of electrical impedance scanning (EIS) in the evaluation of BI-RADS III/IV/V-lesions. Technol Cancer Res Treat 2005;4:93-7. [Crossref] [PubMed]
- Malich A, Boehm T, Facius M, et al. Differentiation of mammographically suspicious lesions: evaluation of breast ultrasound, MRI mammography and electrical impedance scanning as adjunctive technologies in breast cancer detection. Clin Radiol 2001;56:278-83. [Crossref] [PubMed]
- Ng EY, Sree SV, Ng KH, et al. The use of tissue electrical characteristics for breast cancer detection: a perspective review. Technol Cancer Res Treat 2008;7:295-308. [Crossref] [PubMed]
- Sotskova N, Karpov A, Korotkova M, et al. Particularities of electrical impedance images in different forms of growth of infiltrative breast cancer. In: Scharfetter H, Merwa R. editors. 13th International Conference on Electrical Bioimpedance and the 8th Conference on Electrical Impedance Tomography. IFMBE Proceedings, vol 17. Berlin, Heidelberg: Springer, 2007.