The accuracy of clinical 3D printing in reconstructive surgery: literature review and in vivo validation study
Introduction
A growing number of studies demonstrate the utility of 3D printing for preoperative planning, creating intraoperative guidance devices, improving patient education and medical training, and designing patient-specific implants (1-3). Moreover, 3D printing may improve surgical efficiency and accuracy (4), leading to reduced operating time and subsequent health economic benefits (5). However, 3D printing has not yet been incorporated as part of routine clinical practice due to several significant shortcomings (6).
The main limitations of 3D printing are high cost, lengthy production time and perceived inaccuracy of the models (6). Recently, the cost and production time of 3D printing have reduced significantly by the introduction of affordable 3D printers and free software suites (2,7,8). Encouraged by this, Chae et al. have published the Peninsula 3D printing technique using a desktop 3D printer and an open-source 3D software (2). However, there is a relative paucity of studies investigating the accuracy of 3D printing in the literature (9).
Error can be introduced at any stage of 3D printing, but mainly during image acquisition and segmentation (Figure 1) (6,10-12). Most studies have examined the accuracy of 3D printing techniques by comparing linear measurements of the original object to the final 3D-printed model (13-31).
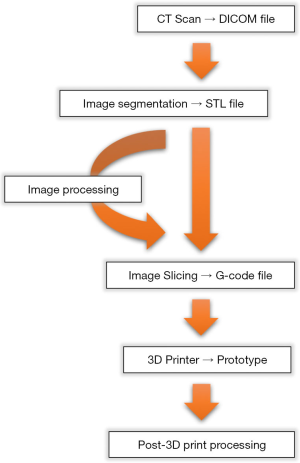
The current study reviews of all published studies where accuracy of image-guided 3D printing of cadaveric specimens is evaluated. Subsequently, an in vivo validation study of the published Peninsula 3D printing technique (2) using the chicken radius bone (Gallus gallus domesticus) is conducted.
Methods
Literature review
A review was undertaken reviewing the published English literature from 1950 to 2018 from well-established databases, such as PubMed, Medline, Web of Science and Embase using search terms, such as “3D printing”, “additive manufacturing”, “rapid prototyping”, “stereolithography”, “CT”, “MRI”, “accuracy”, “precision”, “validation”, “evaluation” and “comparison”. Secondary references found through bibliographic linkage were also retrieved.
Inclusion criteria
Only studies using cadaveric specimens where their direct measurements could be obtained as gold standard were included. Anatomical structures were chosen over generically-shaped phantoms since they pose greater challenge in dimensional assessment and the findings will be more relevant to clinical application. Only studies using CT as imaging source were included since an overwhelming majority of medical 3D printing has been conducted using it (9).
Exclusion criteria
Studies were excluded if authors indirectly deduce the accuracy of 3D printing by analysing the clinical placement of implants or screws using 3D-printed surgical guides since this introduces potentially confounding clinical variables (32-38). Studies using qualitative (39) or volumetric assessment (40), instead of linear measurements, to report 3D printing accuracy were excluded (41-43).
Validation study
The chicken radius bone was chosen for this study, instead of other bones such as the humerus, femur, tibia or fibula, since its length and shape closely resembles the human proximal phalanx (range of 37–44 vs. 32–45 mm, respectively) (Figure 2) (44). Furthermore, it is readily available from the butchers intact in large numbers, compared to other parts of the chicken that are normally discarded or damaged during preparation. This validation study was approved by the Human Research Ethics Committee at Peninsula Health.
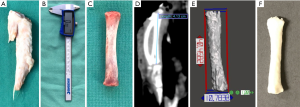
In vitro measurement
The entire defeathered chicken wing was wrapped in cling wrap, placed inside a radiolucent polypropylene-based standard household container, and CT scanned. Then, the radius was dissected out and its articular cartilages removed. Maximum length of the bone was measured using a digital Vernier caliper (Kincrome, Scoresby, VIC, Australia). Out of 50 chicken wings scanned, 11 were removed from the study due to detection of their unrecognised midshaft fractures from the CT scan, which can compromise reliability of the caliper measurements.
CT image acquisition
CT scanning was performed using Siemens SOMATOM Definition AS scanner (Siemens AG, Erlangen, Germany) and hand/wrist scanning protocol. The scan parameters were as follows: tube voltage of 120 kV, tube current exposure time product of 35 mA, single collimation width of 0.6 mm and slice thickness of 0.6 mm. The images were then reconstructed using the bone window and exported in Digital Imaging and COmmunication in Medicine (DICOM) format (1,054 images). The length of each chicken radius was measured from the CT scan using OsiriX software (Pixmeo, Geneva, Switzerland) and recorded to two decimal places (Figure 2).
Image segmentation
The DICOM files were reconstructed into 3D images using free, open-source 3D Slicer software (Surgical Planning Laboratory, Boston, MA, USA) as previously reported and exported in standard tessellation language (STL) format (2,45,46). Briefly, using “ThresholdEffect” function, a range was set from the Hounsfield unit-derived values in order to automatically segment chicken radius from the surrounding soft tissue. Using “PaintEffect” tool, minor surface artefacts were manually removed. Minor surface artefacts were defined as irregularities that did not change overall structure or shape, and which not associated with continuous lines or borders. Each radius was labelled and exported separately. The length of each bone was measured from its 3D-reconstructed image, or STL file, using Autodesk MeshMixer software (Autodesk Inc., San Rafael, CA, USA).
3D printing
Each STL file was converted into a 3D printer-friendly G-code file using Cura software (Ultimaker, Geldermalsen, The Netherlands). The bones were 3D-printed in thermoplastic polylactic acid (PLA) filament using a desktop, fused filament fabrication (FFF) Ultimaker 3 Extended 3D printer (Ultimaker, Geldermalsen, The Netherlands).
Statistical analysis
All measurements were repeated three times and the average was recorded in millimetres to two decimal places. Comparative analysis was performed using Stata statistical software package (StataCorp, College Station, TX, USA). The mean lengths were analysed using one-way ANOVA technique. Using direct caliper measurements as the gold standard, the difference was measured and analysed using unpaired two-sample t-test with unequal variances, with Welch approximation. P value of less than 0.05 was accepted as statistically significant.
Results
Literature review
Characteristics of the published 3D printing validation studies
There were 28 comparison studies within 19 papers which examined the accuracy of medical 3D printing between 1994 and 2016 (Table 1 and Figure 3). Majority of investigators use multidetector CT (MDCT) over cone-beam CT (CBCT) as imaging modality (24 vs. 4 studies). Cadaveric skull and mandible are most commonly used as specimens (16 and 10 studies, respectively). This is most likely due to the fact that 3D printing has been most extensively studied for application in cranio-maxillofacial surgery. Relatively consistent quality of CT images has been acquired as evidenced by a narrow range of CT layer thickness used (0.5–2.5 mm). Majority of papers have reported 3D printing in-house, rather than outsourcing to an external company (14 vs. 5 papers). Encouragingly, this appears to be a growing trend (median year of publication: 2011.5 vs. 2009 respectively). Linear measurements were made more commonly using a caliper than automated CMM (22 vs. 6 studies).
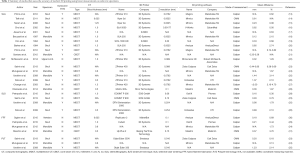
Full table
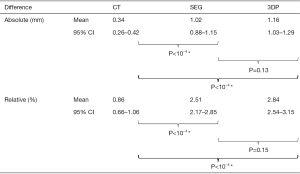
Published accuracy of 3D printing
Stereolithography and binder jet technique (BJT) were most commonly investigated (10 and 7 studies, respectively), followed by newer selective laser sintering (SLS) (4 studies), FFF (4 studies) and PolyJet technology (PJT) (3 studies) (Table 1).
Stereolithography
Stereolithography (SLA) is the earliest 3D printing technology described and is considered the gold standard in 3D biomodel production (47). However, it is slow, relatively expensive and requires significant post-production manual processing (2). Most studies used MDCT over CBCT (8 vs. 2 studies) at slice thickness of 0.5–1.5 mm, and cadaveric skull and mandible as specimens (8 and 2 studies, respectively) (13-21). Highest z-resolution of SLA 3D printers ranged between 0.00177 and 0.0762 mm. Using MDCT, the mean absolute difference between the original specimen and its 3D-printed model was 0.46 mm (range, 0.12–0.85 mm) and relative difference 1.06% (0.08–2.74%). Using CBCT, the mean difference was 0.35–0.39 mm and 0.74–0.82%.
BJT
BJT, also known as powder bed technique, describes a process where a printer head ejects binder material and coloured dye simultaneously onto a bed of powder and fuses them layer-by-layer into a plaster model (48). Major benefits of BJT are that it forgoes support structures and can produce models in multiple colours and materials. However, the final product is brittle and requires extensive post-production manual processing (2). Similar to SLA, most studies used MDCT over CBCT (6 vs. 1 studies) at slice thickness of 0.5–1.5 mm, and cadaveric skull, mandible and upper limb bones as specimens (3, 3, and 1 studies, respectively) (22-28). Highest z-resolution of BJT 3D printers ranged between 0.0762 mm and 0.12 mm. Using MDCT, the mean difference was 1.05 mm (0.32–2.00 mm) and 1.78% (0.38–3.14%). Using CBCT, the mean difference was 0.63 mm and 1.87%.
PJT
PJT, also known as multijet modelling, is similar to SLA but the liquid photopolymer is immediately cured by the ultraviolet (UV) light preventing time-consuming post-production processing (49). A major benefit of PJT is its high resolution; however, its surface finish is still inferior to SLA and the printers remain expensive (2). All studies of PJT used MDCT at slice thickness of 0.6 mm, and cadaveric mandible and skull as specimens (2 and 1 studies, respectively) (23-25). Highest z-resolution of PJT 3D printers was 0.016 mm. Using MDCT, the mean difference was 0.72 mm (0.20–1.23 mm) and 0.82% (0.13–2.14%).
FFF
FFF is the most affordable consumer-grade 3D printing technology where a melted filament of thermoplastic material is extruded in a layer-by-layer fashion (50,51). Major advantages of FFF is its cost and convenience; however, it almost inevitably requires simultaneous production of support structures and hence, yields the lowest quality of surface finish (2). Most studies used MDCT over CBCT (3 vs. 1 studies) at slice thickness of 0.5–1.0 mm, and cadaveric mandible, skull, and vertebra as specimens (2, 1, and 1 studies, respectively) (24,29-31). Highest z-resolution of FFF 3D printers ranged between 0.1 mm and 0.15 mm. Using MDCT, the mean difference was 0.20 mm (0.18–0.21 mm) and 0.95% (0.44–1.73%). Using CBCT, the mean difference was 0.37 mm and 3.76%.
SLS
SLS is a technique where powdered forms of thermoplastic, metal, glass or ceramic material are sintered by high-power laser beams in a layer-by-layer manner (52). Similar to BJT, SLS foregoes support structures and is capable of producing delicate structures with smooth surface finishes. However, high-powered laser of SLS 3D printers requires expert handling for safety and hence, they are related to high cost (2). In contrast to SLA and BJT, all studies of SLS used MDCT at slice thickness of 0.5–1.0 mm, and cadaveric skull and mandible and upper limb bones as specimens (3 and 1 studies, respectively) (23,25,26,29). Highest z-resolution of SLS 3D printers ranged between 0.06 mm and 0.254 mm. Using MDCT, the mean difference was 0.72 mm (0.16–0.93 mm) and 1.25% (0.30–2.10%).
Validation study
Image segmentation took an average of 7.5 minutes per bone (4.2–13.4 minutes). 3D printing took an average of 28.8 minutes per model (25–37 minutes). Mean length of chicken radius bones from direct caliper measurements (i.e., the gold standard) was 39.54 mm (36.64–43.75 mm). Mean length from CT scans was 39.32 mm (36.10–43.30 mm), producing a difference of 0.22 mm from the gold standard (P=1.00). Mean length from image segmentation was 40.56 mm (37.18–44.96 mm), producing a difference of 1.02 mm from the gold standard (P=0.22). Mean length from 3D-printed models was 40.70 mm (37.17–45.00 mm), producing a difference of 1.16 mm from the gold standard (P=0.10).
In order to calculate mean absolute and relative difference, measurements from the DICOM images of CT scans (CT group), image segmentation (SEG group) and 3D-printed models (3DP group) are subtracted from the gold standard for each bone (Figure 3). Mean absolute difference from the CT group was 0.34 mm (standard deviation: 0.26–0.42 mm); SEG group: 1.02 mm (SD: 0.88–1.15 mm); and 3DP group: 1.16 mm (SD: 1.03–1.29 mm). Mean absolute difference from the CT group was significantly smaller than both SEG (P<10−4) and 3DP groups (P<10−4). However, there was no statistical significance between the SEG and 3DP groups (P=0.13). Mean relative difference from CT group was 0.86% (SD: 0.66–1.06%); SEG group 2.51% (SD: 2.17–2.85%) and 3DP group 2.84% (2.54–3.15%). Similarly, mean relative difference of the CT group was significantly smaller than both SEG (P<10−4) and 3DP groups (P<10−4), but there was no statistical significance between latter two groups (P=0.15).
Discussion
Despite a plethora of studies demonstrating numerous utility of 3D printing in surgery (1-3), there is a relative paucity of studies assessing its accuracy in a systematic manner. Current review of 28 comparison studies in 19 papers demonstrates that all 3D printing techniques using conventional MDCT have acceptable accuracy for clinical application with mean difference of less than 1–2 mm (1–2%): SLA: 0.46 mm (1.06%), BJT: 1.05 mm (1.78%), PJT: 0.72 mm (0.82%), FFF: 0.20 mm (0.95%) and SLS 0.72 mm (1.25%). However, it is worth noting that studies have used varying approaches, making them difficult to compare directly.
Some investigators have used CBCT as imaging source, which has lower cost, lower radiation and higher spatial resolution for bony micro-architecture than MDCT, but it is prone to motion artefact and is limited by small field of view (average of 6 cm × 6 cm) making it ideal for dental imaging (53). A wide variety of software programs has been used for image segmentation that differ in basic algorithm for creating 3D-reconstructed images. Some software suites are difficult to obtain for widespread application due to their high cost, such as Mimics (Materialise NV, Leuven, Belgium), or are limited to their local institution, such as V-Works (CyberMed, Seoul, South Korea). Similarly, 3D printers of the same technique can differ in accuracy amongst different brands and different models within the same brand.
Errors can be introduced at all stages of medical 3D printing (Figure 1), but occurs most frequently and most significantly during image acquisition and segmentation (6,12,54). In comparison to MRI, CT is associated with less geometric distortion (55) and, therefore, greater accuracy (20), especially when 3D printing bones (56). The greatest discrepancy can occur from incorrect selection of CT slice thickness when exporting DICOM images (16). CT images are routinely acquired in slice thickness of 0.6–2.0 mm, ideal for 3D printing; however, these are only stored temporarily and discarded after being converted to thicker 5.0 mm images to reduce storage cost (57). As scanners continue to evolve, errors from other aspects of CT, such as image noise, beam hardening, motion artefact, metal artefact and gantry tilt distortion, have significantly improved (58).
When evaluating the accuracy of 3D printing, it is important to differentiate it from describing the z-resolution, which indicates the thinnest layer of 3D print that can be deposited per layer. However, this does not correlate with the overall accuracy of the final model, since increasing the number of layers that needs to be 3D-printed increases the potential for error and artefact (59).
Image segmentation involves partitioning DICOM images into multiple regions that correspond to an anatomical structure of interest and produces its digital 3D reconstruction (58). Due to its reliance on operator expertise for selecting appropriate threshold values and manual processing, image segmentation is highly susceptible to inter-operator variability and is potentially the most significant source of inaccuracy (11,12,60). In place of time-consuming traditional manual segmentation methods (61), semi-automated segmentation techniques have been developed, such as global thresholding (62), edge detection (63) and region growing (64). For 3D printing bones, global thresholding is the most commonly used technique (65,66) and is also employed in our validation study. Despite its benefits, it still requires additional manual editing (12,67). Recent improvements in artificial intelligence and machine learning capabilities pose interesting potential in this field (68). Furthermore, the size of each triangular mesh produced during 3D reconstruction, called triangulation algorithm, can impact accuracy of the final model (69).
Inaccuracy can still be introduced during post-segmentation image processing to further smooth or trim 3D images. However, this has improved significantly in recent times with software advancements and segmentation techniques (70). Slicing software and 3D printers are often packaged together and are generally considered precise by industry standards (71). The surface of 3D-printed models can be damaged during post-print processing to remove support structures, especially with FFF 3D printers. However, these superficial blemishes rarely significantly compromise the overall accuracy (58). Nonetheless, in our study, the models were 3D-printed in an upright position to minimise the amount of support structures that would need to be removed. Altogether, the errors encountered at all the steps combine and influence each other, making the sum of all errors not additive, but multiplicative (9,25). As a result, when assessing 3D printing, the whole process from imaging to fabrication must be validated together.
The results from current validation study using chicken radius is comparable to previously published reports. Interestingly, amongst the studies using FFF 3D printers, our mean absolute and relative differences compare higher than the published values (1.16 vs. 0.20 mm and 2.84% vs. 0.95%, respectively). One of the reasons for this discrepancy may be from soft tissue artefact. In our study, the bones are scanned as chicken wings and then dissected out afterwards. In other studies, the cadaveric specimens are scanned after being dissected, which would yield superior contrast and spatial resolution at bone-to-air interface. Moreover, unlike other expensive, proprietary image segmentation programs, such as Mimics (Materialise NV, Leuven, Belgium), Vitrea (Vital Images, Minnetonka, MN, USA) and OsiriX MD (Pixmeo, Geneva, Switzerland), we have used 3D Slicer used, which has not yet been approved by the US Food and Drugs Administration (FDA) for routine clinical application, albeit only for research purposes (72).
Nevertheless, one of the major limitations of this validation study is that the findings cannot be directly translated to 3D printing anatomical structures that are different in size to chicken radius, such as human femur or humerus. The mean absolute difference found in our study may be altered with other bones in the body with different soft tissue artefacts and their unique anatomical characteristics affecting image segmentation. The mean relative difference may not be translatable since the degree of inaccuracy between different human bones may not linearly correlate with their size. This study is limited to commentary on the accuracy of 3D printing in small bones, and thus direct comparison to large bones is limited. Many of the sources of error, however, will exist in the case of small and large bones, and will exist whether done experimentally or in the clinical setting. In the experimental setting of this study, there is an inherent reduction of error by removing all soft tissue from the scanned bones, and a reduction in the motion artefact that would occur clinically, and thus it could be hypothesized that more of the error could be attributed to image segmentation.
Conclusions
There have been 28 comparison studies in 19 papers which demonstrate that all 3D printing techniques using conventional MDCT have acceptable accuracy for clinical application, with a mean difference from original anatomical specimens of less than 1–2 mm (1–2%): SLA: 0.46 mm (1.06%), BJT: 1.05 mm (1.78%), PJT: 0.72 mm (0.82%), FFF: 0.20 mm (0.95%), and SLS 0.72 mm (1.25%). The current validation study of the Peninsula 3D printing technique produced accuracy similar to the published studies (1.16 mm, 2.84%). There was a statistically significant difference (P<10−4) between the CT group and the latter SEG and 3DP groups indicating that most of the error is introduced during image segmentation stage.
Acknowledgments
We would like to acknowledge the help of Dr Vicky Tobin, our research coordinator, for her support with study design, data collection and analysis.
Funding: None.
Footnote
Conflicts of Interest: All authors have completed the ICMJE uniform disclosure form (available at https://dx.doi.org/10.21037/gs-21-264). WMR serves as an unpaid editorial board member of Gland Surgery from Mar 2018 to Feb 2023. The other authors have no conflicts of interest to declare.
Ethical Statement: The authors are accountable for all aspects of the work in ensuring that questions related to the accuracy or integrity of any part of the work are appropriately investigated and resolved.
Open Access Statement: This is an Open Access article distributed in accordance with the Creative Commons Attribution-NonCommercial-NoDerivs 4.0 International License (CC BY-NC-ND 4.0), which permits the non-commercial replication and distribution of the article with the strict proviso that no changes or edits are made and the original work is properly cited (including links to both the formal publication through the relevant DOI and the license). See: https://creativecommons.org/licenses/by-nc-nd/4.0/.
References
- Kamali P, Dean D, Skoracki R, et al. The Current Role of Three-Dimensional Printing in Plastic Surgery. Plast Reconstr Surg 2016;137:1045-55. [Crossref] [PubMed]
- Chae MP, Rozen WM, McMenamin PG, et al. Emerging Applications of Bedside 3D Printing in Plastic Surgery. Front Surg 2015;2:25. [Crossref] [PubMed]
- Gerstle TL, Ibrahim AMS, Kim PS, et al. A plastic surgery application in evolution: three-dimensional printing. Plast Reconstr Surg 2014;133:446-51. [Crossref] [PubMed]
- Sallent A, Vicente M, Reverté MM, et al. How 3D patient-specific instruments improve accuracy of pelvic bone tumour resection in a cadaveric study. Bone Joint Res 2017;6:577-83. [Crossref] [PubMed]
- Erickson DM, Chance D, Schmitt S, et al. An opinion survey of reported benefits from the use of stereolithographic models. J Oral Maxillofac Surg 1999;57:1040-3. [Crossref] [PubMed]
- Martelli N, Serrano C, van den Brink H, et al. Advantages and disadvantages of 3-dimensional printing in surgery: A systematic review. Surgery 2016;159:1485-500. [Crossref] [PubMed]
- Anderson JR, Thompson WL, Alkattan AK, et al. Three-dimensional printing of anatomically accurate, patient specific intracranial aneurysm models. J Neurointerv Surg 2016;8:517-20. [Crossref] [PubMed]
- Naftulin JS, Kimchi EY, Cash SS. Streamlined, Inexpensive 3D Printing of the Brain and Skull. PLoS One 2015;10:e0136198 [Crossref] [PubMed]
- George E, Liacouras P, Rybicki FJ, et al. Measuring and Establishing the Accuracy and Reproducibility of 3D Printed Medical Models. Radiographics 2017;37:1424-50. [Crossref] [PubMed]
- Mitsouras D, Liacouras P, Imanzadeh A, et al. Medical 3D Printing for the Radiologist. Radiographics 2015;35:1965-88. [Crossref] [PubMed]
- Huotilainen E, Jaanimets R, Valášek J, et al. Inaccuracies in additive manufactured medical skull models caused by the DICOM to STL conversion process. J Craniomaxillofac Surg 2014;42:e259-65. [Crossref] [PubMed]
- Smith EJ, Anstey JA, Venne G, et al. Using additive manufacturing in accuracy evaluation of reconstructions from computed tomography. Proc Inst Mech Eng H 2013;227:551-9. [Crossref] [PubMed]
- Primo BT, Presotto AC, de Oliveira HW, et al. Accuracy assessment of prototypes produced using multi-slice and cone-beam computed tomography. Int J Oral Maxillofac Surg 2012;41:1291-5. [Crossref] [PubMed]
- Taft RM, Kondor S, Grant GT. Accuracy of rapid prototype models for head and neck reconstruction. J Prosthet Dent 2011;106:399-408. [Crossref] [PubMed]
- Nizam A, Gopal RN, Naing L, et al. Dimensional accuracy of the skull models produced by rapid prototyping technology using stereolithography apparatus. Archives of Orofacial Science 2006;1:60-6.
- Choi JY, Choi JH, Kim NK, et al. Analysis of errors in medical rapid prototyping models. Int J Oral Maxillofac Surg 2002;31:23-32. [Crossref] [PubMed]
- Asaumi J, Kawai N, Honda Y, et al. Comparison of three-dimensional computed tomography with rapid prototype models in the management of coronoid hyperplasia. Dentomaxillofac Radiol 2001;30:330-5. [Crossref] [PubMed]
- Bouyssié JF, Bouyssié S, Sharrock P, et al. Stereolithographic models derived from x-ray computed tomography. Reproduction accuracy. Surg Radiol Anat 1997;19:193-9. [PubMed]
- Ono I, Gunji H, Suda K, et al. Method for preparing an exact-size model using helical volume scan computed tomography. Plast Reconstr Surg 1994;93:1363-71. [Crossref] [PubMed]
- Barker TM, Earwaker WJ, Lisle DA. Accuracy of stereolithographic models of human anatomy. Australas Radiol 1994;38:106-11. [Crossref] [PubMed]
- Santana RR, Lozada J, Kleinman A, et al. Accuracy of cone beam computerized tomography and a three-dimensional stereolithographic model in identifying the anterior loop of the mental nerve: a study on cadavers. J Oral Implantol 2012;38:668-76. [Crossref] [PubMed]
- McMenamin PG, Quayle MR, McHenry CR, et al. The production of anatomical teaching resources using three-dimensional (3D) printing technology. Anat Sci Educ 2014;7:479-86. [Crossref] [PubMed]
- Salmi M, Paloheimo KS, Tuomi J, et al. Accuracy of medical models made by additive manufacturing (rapid manufacturing). J Craniomaxillofac Surg 2013;41:603-9. [Crossref] [PubMed]
- Murugesan K, Anandapandian PA, Sharma SK, et al. Comparative evaluation of dimension and surface detail accuracy of models produced by three different rapid prototype techniques. J Indian Prosthodont Soc 2012;12:16-20. [Crossref] [PubMed]
- Ibrahim D, Broilo TL, Heitz C, et al. Dimensional error of selective laser sintering, three-dimensional printing and PolyJet models in the reproduction of mandibular anatomy. J Craniomaxillofac Surg 2009;37:167-73. [Crossref] [PubMed]
- Silva DN, Gerhardt de Oliveira M, Meurer E, et al. Dimensional error in selective laser sintering and 3D-printing of models for craniomaxillary anatomy reconstruction. J Craniomaxillofac Surg 2008;36:443-9. [Crossref] [PubMed]
- Chang PS, Parker TH, Patrick CW Jr, et al. The accuracy of stereolithography in planning craniofacial bone replacement. J Craniofac Surg 2003;14:164-70. [Crossref] [PubMed]
- Olszewski R, Szymor P, Kozakiewicz M. Accuracy of three-dimensional, paper-based models generated using a low-cost, three-dimensional printer. J Craniomaxillofac Surg 2014;42:1847-52. [Crossref] [PubMed]
- Petropolis C, Kozan D, Sigurdson L. Accuracy of medical models made by consumer-grade fused deposition modelling printers. Plast Surg (Oakv) 2015;23:91-4. [Crossref] [PubMed]
- Ogden KM, Aslan C, Ordway N, et al. Factors Affecting Dimensional Accuracy of 3-D Printed Anatomical Structures Derived from CT Data. J Digit Imaging 2015;28:654-63. [Crossref] [PubMed]
- Maschio F, Pandya M, Olszewski R. Experimental Validation of Plastic Mandible Models Produced by a "Low-Cost" 3-Dimensional Fused Deposition Modeling Printer. Med Sci Monit 2016;22:943-57. [Crossref] [PubMed]
- Schweizer A, Mauler F, Vlachopoulos L, et al. Computer-Assisted 3-Dimensional Reconstructions of Scaphoid Fractures and Nonunions With and Without the Use of Patient-Specific Guides: Early Clinical Outcomes and Postoperative Assessments of Reconstruction Accuracy. J Hand Surg Am 2016;41:59-69. [Crossref] [PubMed]
- Hu Y, Yuan ZS, Spiker WR, et al. A comparative study on the accuracy of pedicle screw placement assisted by personalized rapid prototyping template between pre- and post-operation in patients with relatively normal mid-upper thoracic spine. Eur Spine J 2016;25:1706-15. [Crossref] [PubMed]
- Gan Y, Ding J, Xu Y, et al. Accuracy and efficacy of osteotomy in total knee arthroplasty with patient-specific navigational template. Int J Clin Exp Med 2015;8:12192-201. [PubMed]
- Stumpel LJ. Deformation of stereolithographically produced surgical guides: an observational case series report. Clin Implant Dent Relat Res 2012;14:442-53. [Crossref] [PubMed]
- Ozan O, Turkyilmaz I, Ersoy AE, et al. Clinical accuracy of 3 different types of computed tomography-derived stereolithographic surgical guides in implant placement. J Oral Maxillofac Surg 2009;67:394-401. [Crossref] [PubMed]
- Ersoy AE, Turkyilmaz I, Ozan O, et al. Reliability of implant placement with stereolithographic surgical guides generated from computed tomography: clinical data from 94 implants. J Periodontol 2008;79:1339-45. [Crossref] [PubMed]
- Van Assche N, van Steenberghe D, Guerrero ME, et al. Accuracy of implant placement based on pre-surgical planning of three-dimensional cone-beam images: a pilot study. J Clin Periodontol 2007;34:816-21. [Crossref] [PubMed]
- Fasel JH, Beinemann J, Schaller K, et al. A critical inventory of preoperative skull replicas. Ann R Coll Surg Engl 2013;95:401-4. [Crossref] [PubMed]
- Khalil W. Validation of cone beam computed tomography-based tooth printing using different three-dimensional printing technologies. Oral Surg Oral Med Oral Pathol Oral Radiol 2016;121:307-15. [Crossref] [PubMed]
- El-Katatny I, Masood SH, Morsi YS. Error analysis of FDM fabricated medical replicas. Rapid Prototyp J 2010;16:36-43. [Crossref]
- Berry E, Brown JM, Connell M, et al. Preliminary experience with medical applications of rapid prototyping by selective laser sintering. Med Eng Phys 1997;19:90-6. [Crossref] [PubMed]
- Kragskov J, Sindet-Pedersen S, Gyldensted C, et al. A comparison of three-dimensional computed tomography scans and stereolithographic models for evaluation of craniofacial anomalies. J Oral Maxillofac Surg 1996;54:402-11; discussion 411-2. [Crossref] [PubMed]
- Garrido Varas CE, Thompson TJ. Metric dimensions of the proximal phalanges of the human hand and their relationship to side, position, and asymmetry. Homo 2011;62:126-43. [Crossref] [PubMed]
- Chae MP, Hunter-Smith DJ, Chung RD, et al. 3D-printed, patient-specific, DIEP template for preoperative planning autologous breast reconstruction: prospective case series in 20 patients. J Plast Reconstr Aesthet Surg 2017; [Crossref]
- Chae MP, Hunter-Smith DJ, Rostek M, et al. Enhanced Preoperative Deep Inferior Epigastric Artery Perforator Flap Planning with a 3D-Printed Perforasome Template: Technique and Case Report. Plast Reconstr Surg Glob Open 2018;6:e1644 [Crossref] [PubMed]
- Hull CW. Apparatus for production of three-dimensional objects by stereolithography. US Patent No. 4,575,330. March 11, 1986.
- Sachs EM, Haggerty JS, Cima MJ, et al. Three-dimensional printing techniques. US Patent No. 5,204,055. April 20, 1993.
- lmquist TA, Smalley DR. Thermal stereolithography. US Patent No. 5,141,680. August 25, 1992.
- Watson RA. A low-cost surgical application of additive fabrication. J Surg Educ 2014;71:14-7. [Crossref] [PubMed]
- Cohen A, Laviv A, Berman P, et al. Mandibular reconstruction using stereolithographic 3-dimensional printing modeling technology. Oral Surg Oral Med Oral Pathol Oral Radiol Endod 2009;108:661-6. [Crossref] [PubMed]
- Deckard C. Method and apparatus for producing parts by selective sintering. US Patent No. 4,863,538. September 5, 1989.
- Posadzy M, Desimpel J, Vanhoenacker F. Cone beam CT of the musculoskeletal system: clinical applications. Insights Imaging 2018;9:35-45. [Crossref] [PubMed]
- Huotilainen E, Paloheimo M, Salmi M, et al. Imaging requirements for medical applications of additive manufacturing. Acta Radiol 2014;55:78-85. [Crossref] [PubMed]
- Wang D, Doddrell DM, Cowin G. A novel phantom and method for comprehensive 3-dimensional measurement and correction of geometric distortion in magnetic resonance imaging. Magn Reson Imaging 2004;22:529-42. [Crossref] [PubMed]
- Liang X, Lambrichts I, Sun Y, et al. A comparative evaluation of Cone Beam Computed Tomography (CBCT) and Multi-Slice CT (MSCT). Part II: On 3D model accuracy. Eur J Radiol 2010;75:270-4. [Crossref] [PubMed]
- Fleischmann D, Rubin GD, Paik DS, et al. Stair-step artifacts with single versus multiple detector-row helical CT. Radiology 2000;216:185-96. [Crossref] [PubMed]
- Winder J, Bibb R. Medical rapid prototyping technologies: state of the art and current limitations for application in oral and maxillofacial surgery. J Oral Maxillofac Surg 2005;63:1006-15. [Crossref] [PubMed]
- Favero CS, English JD, Cozad BE, et al. Effect of print layer height and printer type on the accuracy of 3-dimensional printed orthodontic models. Am J Orthod Dentofacial Orthop 2017;152:557-65. [Crossref] [PubMed]
- Stoor P, Suomalainen A, Lindqvist C, et al. Rapid prototyped patient specific implants for reconstruction of orbital wall defects. J Craniomaxillofac Surg 2014;42:1644-9. [Crossref] [PubMed]
- Fasel JHD, Beinemann J. Computer science tools for manual editing of computed tomographic images: impact on the quality of 3D printed models. Surg Sci 2014;5:439-43. [Crossref]
- Otsu N. A threshold selection method from gray-level histograms. I IEEE Trans Syst Man Cybern 1979;9:62-6. [Crossref]
- Canny J. A computational approach to edge detection. IEEE Trans Pattern Anal Mach Intell 1986;8:679-98. [Crossref] [PubMed]
- Adams R, Bischof L. Seeded region growing. IEEE Trans Pattern Anal Mach Intell 1994;16:641-7. [Crossref]
- van Eijnatten M, Koivisto J, Karhu K, et al. The impact of manual threshold selection in medical additive manufacturing. Int J Comput Assist Radiol Surg 2017;12:607-15. [Crossref] [PubMed]
- Rathnayaka K, Momot KI, Noser H, et al. Quantification of the accuracy of MRI generated 3D models of long bones compared to CT generated 3D models. Med Eng Phys 2012;34:357-63. [Crossref] [PubMed]
- Gelaude F, Vander Sloten J, Lauwers B. Accuracy assessment of CT-based outer surface femur meshes. Comput Aided Surg 2008;13:188-99. [Crossref] [PubMed]
- Gassman EE, Powell SM, Kallemeyn NA, et al. Automated bony region identification using artificial neural networks: reliability and validation measurements. Skeletal Radiol 2008;37:313-9. [Crossref] [PubMed]
- Fedorov A, Beichel R, Kalpathy-Cramer J, et al. 3D Slicer as an image computing platform for the Quantitative Imaging Network. Magn Reson Imaging 2012;30:1323-41. [Crossref] [PubMed]
- Karron DB. SpiderWeb algorithm for surface construction in noisy volume data. Proc of SPIE 1992;1808:462-76. [Crossref]
- van Eijnatten M, van Dijk R, Dobbe J, et al. CT image segmentation methods for bone used in medical additive manufacturing. Med Eng Phys 2018;51:6-16. [Crossref] [PubMed]
- Di Prima M, Coburn J, Hwang D, et al. Additively manufactured medical products - the FDA perspective. 3D Print Med 2016;2:1.