Identification of markers associated with brain metastasis from breast cancer through bioinformatics analysis and verification in clinical samples
Introduction
Breast cancer (BC) is one of the most common neoplasms, and the incidence of BC ranks first among female malignant tumors (1). It is estimated that there were 271,270 new BC cases in the USA in 2019, and the estimated number of deaths was as high as 42,260 (2). BC’s incidence is dramatically increasing year by year, showing a clear younger trend, posing a serious threat to women’s physical and mental health. BC is highly heterogeneous, and different molecular subtypes of BC have different clinical characteristics and prognosis, which increases the difficulty of clinical diagnosis and treatment (3). At present, BC’s clinical treatment mainly includes chemotherapy, radiotherapy, surgery, and targeted therapy. With the continuous advancements in medical standards, BC’s curative effect has been significantly improved, and quality of life has improved dramatically for patients (4). However, due to the low awareness of cancer prevention among the general population, many patients are already at the advanced stages of BC when they are diagnosed. The prognosis of advanced BC is poor, and is usually accompanied by cancer metastasis. Even if patients with advanced BC have been cured, the recurrence rate of breast carcinoma is high. Recurrence and metastasis are the main causes of BC related death (1). Therefore, periodical checks for BC and early treatment can effectively reduce mortality. For clinicians, discovering more accurate diagnostic methods has become a critical priority.
BC is the second most common cause of brain metastasis after lung cancer (1). With the improvements in diagnosis and treatment, BC patients’ survival time with recurrence and metastasis has been significantly prolonged, which significantly increases the chances of brain metastasis in patients. The incidence of brain metastasis in BC patients is increasing year by year. BC cases with brain metastasis account for 15–30% of BC patients with metastasis (5). The current treatment plan is not effective for BC patients with brain metastasis, and patients have an extremely poor prognosis. BC patients with brain metastases who do not accept any treatment have an overall survival (OS) 1–2 months. After active treatment, patients’ median OS time generally does not exceed 2 years (1,6). Previous research has shown that the major risk factors for BC with brain metastasis are age, hormone receptor expression [estrogen receptor (ER), progesterone receptor (PR)], human epidermal growth factor receptor 2 (HER2), extracranial metastasis, number of brain metastases, histological grade, and pathological stage (7,8).
Unfortunately, the results of drug treatments for targets such as ER, PR, and HER2 have failed to prevent the occurrence of brain metastases (4,9,10) effectively. HER2-directed antibodies poorly penetrate the blood brain barrier and appear to provide an unclear benefit for brain metastasis patients (11). Some studies have suggested an increased propensity for central nervous system metastasis among triple negative BC (TNBC), for which targeted therapies are ineffective (10,12,13). Patient survival time and the incidence of metastasis still cannot be effectively improved. Therefore, patients with BC brain metastases need better molecular therapeutic targets.
In the present study, we applied univariate Cox regression analysis to analyze the association between metastasis-associated genes (MAGs) and brain metastasis from BC. Weighted gene co-expression network analysis (WGCNA) was performed to screen the genes which were associated with brain metastases from BC. The least absolute shrinkage and selection operator (LASSO) was used to identify key genes associated with oncology metastasis, and a nomogram was constructed for predicting tumor metastasis. Finally, 2 key genes were screened from MAGs and were further verified in clinical samples. We present the following article in accordance with the MDAR reporting checklist (available at http://dx.doi.org/10.21037/gs-20-767).
Methods
Data collection
The gene expression matrix of 24 BC with brain metastasis samples and primary BC samples from the GSE14690 dataset (14) was obtained from the Gene Expression Omnibus (GEO) database (doi: 10.1186/bcr2603). The raw gene expression data of BC and normal control samples were obtained from The Cancer Genome Atlas (TCGA)-BRCA. An external dataset from TCGA was used as a verification group.
WGCNA of GSE14690 dataset
In the WGCNA algorithm, the weighted gene co-expression network construction’s premise is that the connection of the gene network should obey the scale-free distribution. In the present study, the weighting coefficient (P) was selected to infinitely approach the scale-free network distribution. The selection of the soft threshold should satisfy the following conditions: the correlation coefficient between the log(k) of the number of connected nodes and the log[p(k)] of the occurrence probability of the node should reach at least 0.8. In this study, there were 5 main steps in the construction of the gene co-expression network:
(I) Calculate the similarity matrix between genes.
The correlation coefficient between gene i and gene j was Sij, and the similarity matrix S=[Sij].
(II) Define the adjacency function.
The soft threshold was defined for describing the association between any 2 genes. The adjacency coefficient aij was obtained by exponentially weighting each gene pair’s correlation coefficient to the power of β, and β was defined as the soft threshold. The soft threshold was set as 0.8, and the similarity matrix was converted to an adjacency matrix.
(III) Calculate the degree of dissimilarity between nodes and transform the adjacency matrix into a topological matrix.
To make the module more in line with biological characteristics, WGCNA uses a topological overlap measure (TOM) to calculate the degree of the correlation between 2 genes in WGCNA.
In the above formula, lij represents the sum of the product of the adjacency coefficients of the nodes connected to both genes i and j, ki represents the sum of the adjacency coefficients of the nodes individually connected to the gene i, and kj represents the sum of the adjacency coefficients of the nodes individually connected to the gene j. After the adjacency matrix was converted to a topological matrix, the degree of dissimilarity between nodes was measured by
(IV) Identify gene modules through cluster analysis.
To ensure that the genes in the modules were highly correlated, hierarchical clustering of genes based on the dissimilarity of the TOM matrix was performed in WGCNA to construct a hierarchical clustering tree. The static tree cut method was used in this study.
(V) Explore the association between modules and phenotypes.
The module eigengene (ME) was calculated to detect the module’s overall level of gene expression. For a certain gene, the correlation between the expression of the gene in all samples and the module’s characteristic value was used to measure the importance of this gene in the module (module membership, MM). Here, the relationship between the module and the phenotype was evaluated by calculating the correlation coefficient between the module’s characteristic value and the phenotype variable. Also, according to different phenotype groups, the t-test was used to calculate the difference in each gene expression between the different groups to obtain different P values, and the P values were log10 transformed to obtain gene significance (GS). The average of the GS of each gene in a certain gene module was calculated to get the significance of a certain module (module significance, MS).
Construction of LASSO Cox proportional hazards regression models
The genes from the modules with most MS were then analyzed through LASSO Cox proportional hazards regression models to screen MAGs. All of the samples in LASSO Cox proportional hazards regression models were from the TCGA-BRCA database. The sample composition of the Cox proportional hazards model was:
In the formula above, n was the sample size, Ti and Ci respectively were the survival time and censorship time of the individual i, and δi was the event variable (δi=1 indicated that the sample had reached the end of the study, and δi=0 indicated that the sample was still being followed up).
The regression coefficients were estimated using the partial likelihood function estimation method, and the following formula calculated the log partial likelihood function:
Then the LASSO method was applied to the Cox proportional hazards model, and the following formula calculated the partial likelihood function with penalty:
The parameter λ was used to adjust the model. When λ was small, there were too many variables in the model, and the model was not sparse enough, resulting in a high possibility of overfitting. When λ increased, the regression coefficient with low correlation was compressed to 0, and the corresponding variable exited the model so that the effect of a variable, feature selection, and estimation could be realized. However, if λ were too large, it would lead to a substantial deviation in estimating the large regression coefficient. Therefore, the λ corresponding to the minimum partial likelihood function was selected to construct the model, and the following formula calculated the risk score of the patient in the model:
Where βi was the non-zero LASSO coefficient corresponding to the minimum λ value, and xi was the gene expression level corresponding to the non-zero LASSO coefficient.
The 10-fold cross-validation method was used to test the accuracy of algorithms and models. The dataset was randomly divided into 10 parts, 9 of which were used as training data in turn, and the remaining 1 was set as test data. The average value of the results of 10 verifications was used to estimate the accuracy of the algorithm to obtain a higher accuracy rate and reduce the risk of model overfitting. The glmnet package from R software was used to construct a multi-gene prognostic prediction model for BC. The function glmnet was used to generate the results of model fitting. The function cv.glmnet was used to perform 10-fold cross-validation of the model corresponding to each λ to calculate the value of the logarithmic partial likelihood function corresponding to each λ. The model with the λ value corresponding to the smallest logarithmic partial likelihood function value was selected.
The construction and evaluation of the nomogram
The factors which were related to cancer metastasis were integrated to construct the nomogram of TCGA data through R software. The returned samples were obtained by bootstrap self-extraction and validated by calculation. The C-index of the prediction model was then calculated. The capacity was further evaluated and quantified by calculating the level to which the C-index and baseline time proposed by the nomogram in the standard curve. Finally, a receiver operating characteristic (ROC) curve of brain metastasis from BC was generated to evaluate the nomogram’s accuracy.
Gene set enrichment analysis (GSEA)
In order to detect the potential molecular mechanisms of our screened MAGs, GSEA (15,16) was conducted to screen enriched terms predicted to be associated with the Kyoto Encyclopedia of Genes and Genomes (KEGG) pathway in C2, and a gene set that contained genes was annotated by the same Gene Ontology (GO) terms in C5. Both P<0.05 and a false discovery rate (FDR) <0.05 were considered statistically significant.
Immunohistochemistry (IHC)
All of the 103 human primary BC surgical tissue specimens in the present study were available as formal in fixed-paraffin embedded (FFPE) tumor blocks, and the information of all the clinical samples was collected. Written informed consent was obtained from all participants. Patients were diagnosed with cerebral metastases from BC, as determined by computed tomography (CT) and magnetic resonance imaging (MRI). The primary antibody against DLG3 was obtained from Abcam (ab254634, 1:1,000), and the primary antibody against GFI1 was obtained from Sabbiotech (#47575, 1:200). After the addition of the primary antibody, sections were incubated at 4 °C overnight. After washing 3 times with phosphate-buffered saline (PBS), sections were treated with an immunohistochemistry kit according to the manufacturer’s instructions (G1211, Servicebio). The color was developed by 3'-diaminobenzidine (DAB). Two pathologists who were blinded to the protocol evaluated the immunostaining. For DLG3, scores were determined by staining intensity in tissue samples (0, none; 1, weak; 2, moderate; 3, strong) multiplied by the percentage of cell staining (positive cells ≤25% of the cells: 1; 26–50%: 2; 51–75%: 3; ≥75%: 4). The scope of this value was 0–12. The median value of scores was used to determine the cut-off. Tissue samples with scores above the cut-off value were considered to express the indicated gene and vice versa highly. All procedures performed in this study involving human participants were in accordance with the Declaration of Helsinki (as revised in 2013) and the study was approved by the ethics committee of Tianjin Medical University General Hospital (IRB2020-WZ-118). Written informed consent was obtained from each participant included in the study.
Survival analysis
A Student’s t-test was used for paired data. ANOVA examined continuous variables. Categorical variables were analyzed through either the Fisher’s exact test or χ2 test. Kaplan-Meier analysis was used to evaluate the model’s prognostic prediction effect when the test set, validation set, and total set were divided into high-risk groups and low-risk groups. The log-rank test was used to test the statistical significance of survival curve differences. Univariate and multivariate Cox regression analysis was used to verify whether the risk coefficient was an independent predictor of BC OS. ROC curves were used to evaluate the accuracy of survival analysis by using the survival ROC package in R. The Kruskal-Wallis test was performed to detect the relationship between clinical characteristics and risk scores.
Results
WGCNA construction and key module identification
The gene expression profiling and clinical features were integrated as the input dataset; then the dendrogram was constructed (Figure 1A). After 3 outlier samples were discarded, WGCNA was constructed based on the 1,488 most variable genes. The soft threshold power was set as 9 to make R2=0.808, ensuring the co-expression network was a scale-free network (Figure 1B). After the soft-thresholding was determined, the co-expression network was constructed. The minimum number was set to 50. Dynamic tree shearing was used to divide the modules, and the abline was set as 0.25. The modules of similar special genes were merged in the gene cluster dendrogram, then 2 modules were obtained (Figure 2A). In the gene cluster dendrogram, each color represented a different module. Analysis of relevance between key genes and clinical features was constructed in a heat map (Figure 2B). The grey module and turquoise module’s correlation coefficient was the highest, indicating that the genes in these 2 modules were most related to tumor metastasis. The genes in these 2 modules were selected for further research.
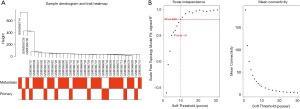
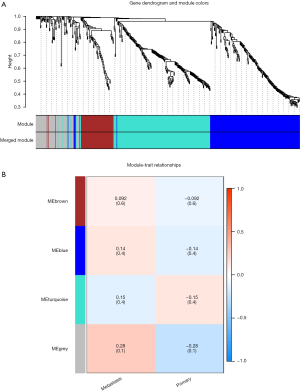
Identification of key genes and survival analysis
LASSO was performed for the subsequent selection of the 52 genes (Figure 3). Ten-fold cross-validation mean was used to assess λ. When λ=0.024, the error rate reached the minimum and the MAGs were screened. Kaplan-Meier survival analysis was performed to evaluate the prognostic prediction effect of the identified MAGs when the test set, validation set, and total set were divided into high-risk groups and low-risk groups. The risk scores and survival time of the samples are shown (Figure 4).
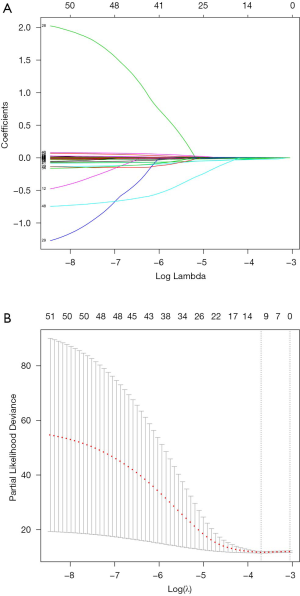
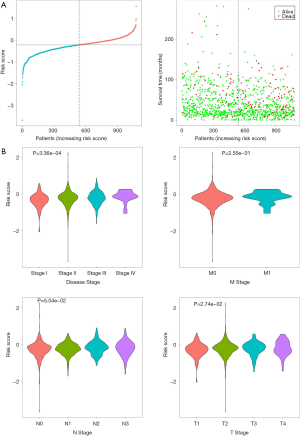
We detected the association between clinical characteristics and risk scores using the Kruskal-Wallis test. As shown in Figure 4, the disease stage and T stage were significantly related to risk scores. In the training set, test and total set (Figure 5), P<0.01 indicated that the survival curve difference was statistically significant. The ROC curve was then used to evaluate the accuracy of the survival analysis. In the training set, test and total set, the AUC was 0.731, 0.651, and 0.667, respectively, indicating that the prediction effect was good. To further verify the prediction effect of the MAGs, another external dataset from TCGA was used as a verification group. The samples were divided into low-risk groups and high-risk groups based on risk scores. The survival analysis results showed that the risk scores were significantly related to the survival time of patients. The area under the ROC curve was 0.601, indicating that our results were reliable. Moreover, the risk scores were significantly associated with the histological grade of the patients (Figure S1).
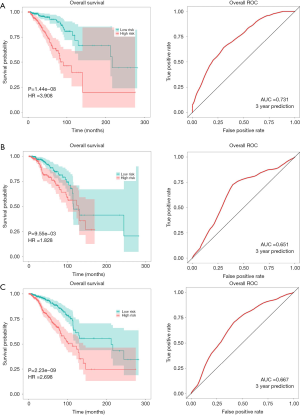
Establishment and evaluation of clinical predictive models
Univariate and multivariate Cox regression was performed based on MAGs and clinical information to establish the nomogram. Considering that in the multivariate Cox regression, only age and MAGs were the significant factors (Figure 6), these 2 factors were applied in the establishment of the nomogram. Factors were given scores in the nomogram. The corresponding score was added to get a total score according to each prognostic factor (Figure 7). The model’s predictive power was evaluated and quantified by measuring the degree of fit between the baseline time predicted through the nomogram and the C-index. It was observed from the 3- and 5-year metastasis calibration curves illustrated in Figure 7 that the nomogram had a good predictive ability for metastasis. Also, the risk scores of postoperative metastasis of patients were calculated, and an ROC curve was plotted (Figure 7). For the ROC curve, the area under the curve was 0.737, indicating that the nomogram had good predictive accuracy for metastasis.
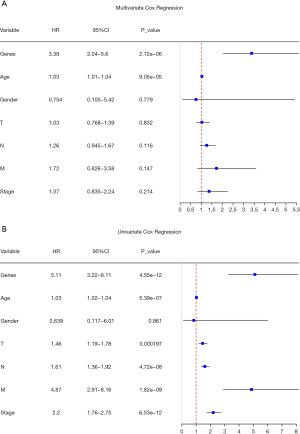
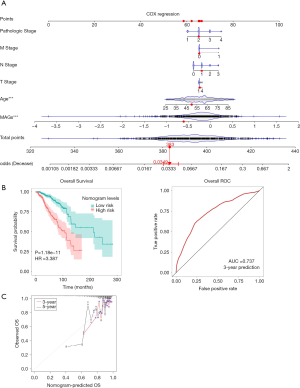
GSEA analysis for MAGs
To investigate the biological characteristics of the MAGs, a GSEA assay was performed. The most significant KEGG pathways were proteoglycans in cancer, RAP1 signaling pathway, and ERBB signaling pathway (Figure 8A). The most significant biological process terms were nervous system process, positive regulation of peptidyl-tyrosine phosphorylation, and positive regulation of ERK1 and ERK2 cascade (Figure 8B). The most significant cell component terms were receptor complex, intrinsic component of plasma, and membrane Golgi apparatus (Figure 8C). The most significant molecular function terms were transmembrane receptor protein tyrosine kinase activity, protein tyrosine kinase activity, and transmembrane signaling receptor activity (Figure 8D).
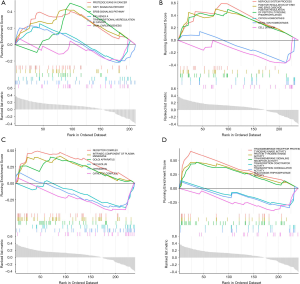
Verification of key genes in clinical samples
The 103 patients were all female, ranging in age from 25 to 77 years old, with median age at initial BC diagnosis of 49 years old. The relevance of clinical information and gene expression was further analyzed. In the 103 BC patients, high expression of DLG3 in primary breast tumor was significantly related to lymph node (LN) metastasis and histological grade (Table 1). The results of univariate and multivariate analysis of clinicopathological factors for OS and recurrence-free survival (RFS) also revealed that high expression of DLG3 in primary breast tumor was significantly related to brain metastasis from BC (Table 2). The median time to OS was 60 months [95% confidence interval (CI): 41.785, 78.215] in the low DLG3 expression group (n=32) and 36 months (95% CI: 25.126, 46.874) in the high DLG3 expression group (n=71) of BC tissues (P<0.001). The median time to RFS for the DLG3 low expression group (n=32) was 50 months (95% CI: 24.749, 75.251), and 24 months (95% CI: 19.908, 28.092) for the DLG3 high expression group (n=71; P<0.001). To further evaluate the predictive effect of DLG3 expression on metastasis, IHC was performed to detect the expression of DLG3 in BC samples and normal samples. As shown in Figure 9, DLG3 was significantly overexpressed in tumor samples (P<0.001). Moreover, high expression of DLG3 was significantly related to short survival time. The expression of another key MAG, GFI1, was also detected by IHC in tumor and normal tissues. The expression of GFI1 was also overexpressed in BC samples (Figure 10), and high expression of GFI1 was significantly related to high expression of DLG3 (χ2=14.264, P<0.001).
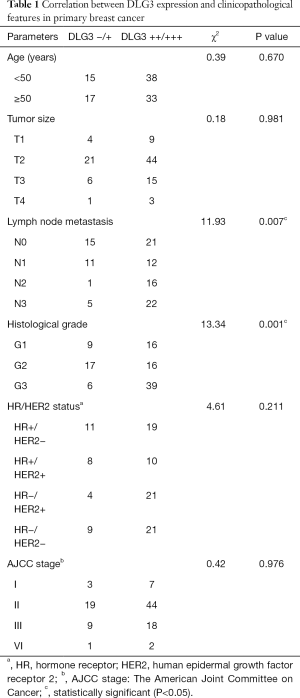
Full table
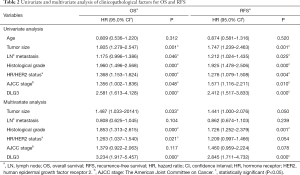
Full table
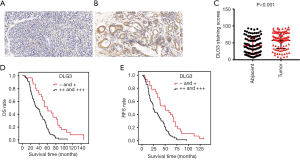
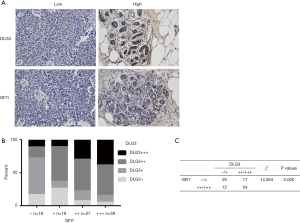
Discussion
Brain metastasis affects approximately 10% of cancer patients (17-19). Even minor lesions can result in neurological dysfunction, and the median survival time of brain metastasis patients is very short (19). The 2 main sources of brain metastasis are adenocarcinoma of the lung or breast. In BC, long-term remission is usually required before long-term recurrence (20,21), which indicates that BC cells initially lack sufficient ability to grow in distant organs, but develop under the selective pressure of the microenvironment of different organs. BC metastasis often spreads widely in the same organ, earlier than in other organs, while brain metastasis is often a late event (17). Therefore, early prevention is important for brain metastasis from BC.
Previous studies have identified many risk factors for brain metastasis. However, the results are often inconsistent and the conclusions are quite different. Moreover, some studies have obvious selection bias, which leads to low reliability of the conclusions (22,23). Other studies included fewer eligible cases, which is very important for brain metastasis from BC (24,25). Age is also one of the most widely reported factors, but is still controversial (17). In a retrospective study including 219 BC patients, Evans et al. (26), reported that the incidence of brain metastasis from BC under 40 years old was higher than that in BC patients over 60 years old (43%: 8%). Research has also found that age and race were not significant risk factors that affected the survival of brain metastasis from BC (17). In the present study, the GEO dataset GSE14690 and the TCGA-BRCA dataset which had large sample sizes were used, and an external TCGA dataset was used for further verification. Our results support age as an independent risk factor. The expression of special biomarkers is another major factor influencing BC brain metastasis. According to the current research, these markers were mainly concentrated in ER, PR, andHER2 (27-29).
BC is very heterogeneous, and 4 intrinsic BC subtypes have been proposed: luminal A/B (HR positive and HER2 negative or positive), HER2 positive (HR negative and HER2 positive), and TNBC (HR negative and HER2 negative) (30,31). TNBC confers a high risk of death after brain metastases regardless of patient race and age (32). Some studies have confirmed that HER2+ tumors have a higher risk of developing cerebral metastasis in metastatic BC, and have a significantly higher incidence of brain metastasis after treatment with trastuzumab (33,34). Improvements in systemic therapies and new molecular targets for the treatment of BC with brain metastasis are urgently needed. In this study, HR/HER2 status (molecular subtypes) in the univariate analysis of clinicopathological factors for OS and RFS was statistically significant (P<0.05) (Table 2). However, compared with MAGs, the weighting and relative importance of subtypes were not very attractive in the multivariate analysis. This is consistent with the data obtained by the LASSO Cox regression model and the nomogram.
In recent years, precision medicine has always been based on the genetic background of patients. The development of next-generation sequencing (NGS) has produced exponentially increasing biological data. The analysis of this type of high-throughput data is an important way of finding new biomarkers and establishing robust prediction models (35). Since the Cox regression model requires that the number of follow-up cases in the multivariate analysis should be more than 10 times the number of covariates, the Cox proportional hazards model cannot be directly applied to the high-dimensional gene expression levels measured by microarray and NGS data. In addition, the expression levels of genes are often highly correlated, especially genes originating from the same co-expressed gene module in WGCNA. Such collinear data is not suitable for direct application of the Cox proportional hazards model for analysis. In order to solve the above problems, in 1996 Tibshirani proposed the LASSO method. This method is a compressed estimation method of a linear model. It minimizes the sum of squared residuals under the constraint that the sum of the absolute value of each coefficient is less than a constant, so that some regression coefficients are compressed (36), and a sparse model is obtained which can effectively select variables for high-dimensional and collinear data (37). Tibshirani applied the basic assumptions of variable selection and constrained contraction in the LASSO method to the Cox proportional hazards regression model, which provided a robust model while reducing the estimated variance, and was more accurate than the stepwise selection method in screening prognostic-related genes. Here, we screened MAGs through WGCNA first, and then LASSO Cox proportional hazards regression models were constructed for further selection. Moreover, an external dataset was used for verification to ensure that the prediction model was accurate. We identified 2 key MAGs, DLG3 and GFI1, which were strongly associated with brain metastasis from BC, though have rarely been reported in the literature.
Discs large homolog 3 (DLG3) is in the membrane-associated guanylate kinase (MAGUK) superfamily, whose members contain PDZ (PSD-95/DLG/ZO-1) and SH3 domains. It plays important roles in different polarized cell types and in the establishment and maintenance of apical cell junctions and tight junctions of epithelial cells and neuronal synapses (38-40). In some studies, MIAT promoted the methylation of CpG islands in the DLG3 promoter and inhibited DLG3 expression. Furthermore, DLG3 silencing has been shown to inhibit BC progression via activation of the Hippo signaling pathway (41). In pancreatic ductal adenocarcinoma, the downregulation of DLG3 resulted in Golgi complex fragmentation and reduced cancer-promoting chemokines (42).
It has been reported that DLG3 was overexpressed in BC, and high expression of DLG3 was associated with decreased survival time of patients with BC (43). However, the role of DLG3 in brain metastases from BC is still unknown. Our results also revealed that high expression of DLG3 was significantly related to high expression of GFI1. This correlation suggests that there might be an interaction between these 2 genes, though more studies on this topic are still required.
Conclusions
Datasets were downloaded from the public databases GEO and TCGA.WGCNA was performed to construct LASSO Cox proportional hazards regression models for screening key MAGs. A nomogram, which had better sensitivity and specificity for brain metastasis from BC, was constructed. The potential mechanisms of MAGs were detected by GSEA. The increased expression of the key MAGs DLG3 and GFI1 were detected by IHC in BC samples, and expression was also closely related to brain metastasis from BC.
Acknowledgments
Funding: This study was supported by the Health Science and Technology Project of Tianjin Health Commission (RC20197, KJ20180).
Footnote
Reporting Checklist: The authors have completed the MDAR reporting checklist. Available at http://dx.doi.org/10.21037/gs-20-767
Data Sharing Statement: Available at http://dx.doi.org/10.21037/gs-20-767
Conflicts of Interest: All authors have completed the ICMJE uniform disclosure form (available at http://dx.doi.org/10.21037/gs-20-767). The authors have no conflicts of interest to declare.
Ethical Statement: The authors are accountable for all aspects of the work in ensuring that questions related to the accuracy or integrity of any part of the work are appropriately investigated and resolved. The study was approved by the ethics committee of Tianjin Medical University General Hospital (IRB2020-WZ-118). Written informed consent was obtained from each participant included in the study. All procedures performed in this study involving human participants were in accordance with the Declaration of Helsinki (as revised in 2013).
Open Access Statement: This is an Open Access article distributed in accordance with the Creative Commons Attribution-NonCommercial-NoDerivs 4.0 International License (CC BY-NC-ND 4.0), which permits the non-commercial replication and distribution of the article with the strict proviso that no changes or edits are made and the original work is properly cited (including links to both the formal publication through the relevant DOI and the license). See: https://creativecommons.org/licenses/by-nc-nd/4.0/.
References
- DeSantis C, Siegel R, Bandi P, et al. Breast cancer statistics, 2011. CA Cancer J Clin 2011;61:409-18. [Crossref] [PubMed]
- Siegel RL, Miller KD, Jemal A. Cancer statistics, 2019. CA Cancer J Clin 2019;69:7-34. [Crossref] [PubMed]
- Veronesi U, Boyle P, Goldhirsch A, et al. Breast cancer. Lancet 2005;365:1727-41. [Crossref] [PubMed]
- Woolston C. Breast cancer. Nature 2015;527:S101. [Crossref] [PubMed]
- Bos PD, Zhang XH, Nadal C, et al. Genes that mediate breast cancer metastasis to the brain. Nature 2009;459:1005-9. [Crossref] [PubMed]
- Cardoso F, van't Veer LJ, Bogaerts J, et al. 70-Gene Signature as an Aid to Treatment Decisions in Early-Stage Breast Cancer. N Engl J Med 2016;375:717-29. [Crossref] [PubMed]
- Achrol AS, Rennert RC, Anders C, et al. Brain metastases. Nat Rev Dis Primers 2019;5:5. [Crossref] [PubMed]
- Mustafa DAM, Pedrosa RMSM, Smid M, et al. T lymphocytes facilitate brain metastasis of breast cancer by inducing Guanylate-Binding Protein 1 expression. Acta Neuropathol 2018;135:581-99. [Crossref] [PubMed]
- Miller JA, Kotecha R, Ahluwalia MS, et al. Overall survival and the response to radiotherapy among molecular subtypes of breast cancer brain metastases treated with targeted therapies. Cancer 2017;123:2283-93. [Crossref] [PubMed]
- Krishnan M, Krishnamurthy J, Shonka N. Targeting the Sanctuary Site: Options when Breast Cancer Metastasizes to the Brain. Oncology (Williston Park) 2019;33:683730 [PubMed]
- Stemmler HJ, Schmitt M, Willems A, et al. Ratio of trastuzumab levels in serum and cerebrospinal fluid is altered in HER2-positive breast cancer patients with brain metastases and impairment of blood-brain barrier. Anticancer Drugs 2007;18:23-8. [Crossref] [PubMed]
- Kennecke H, Yerushalmi R, Woods R, et al. Metastatic behavior of breast cancer subtypes. J Clin Oncol 2010;28:3271-7. [Crossref] [PubMed]
- Dawood S, Lei X, Litton JK, et al. Incidence of brain metastases as a first site of recurrence among women with triple receptor-negative breast cancer. Cancer 2012;118:4652-9. [Crossref] [PubMed]
- Da Silva L, Simpson PT, Smart CE, et al. HER3 and downstream pathways are involved in colonization of brain metastases from breast cancer. Breast Cancer Res 2010;12:R46. [Crossref] [PubMed]
- Mootha VK, Lindgren CM, Eriksson KF, et al. PGC-1alpha-responsive genes involved in oxidative phosphorylation are coordinately downregulated in human diabetes. Nat Genet 2003;34:267-73. [Crossref] [PubMed]
- Subramanian A, Tamayo P, Mootha VK, et al. Gene set enrichment analysis: a knowledge-based approach for interpreting genome-wide expression profiles. Proc Natl Acad Sci U S A 2005;102:15545-50. [Crossref] [PubMed]
- Weil RJ, Palmieri DC, Bronder JL, et al. Breast cancer metastasis to the central nervous system. Am J Pathol 2005;167:913-20. [Crossref] [PubMed]
- El Kamar FG, Posner JB. Brain metastases. Semin Neurol 2004;24:347-62. [Crossref] [PubMed]
- Lassman AB, DeAngelis LM. Brain metastases. Neurol Clin 2003;21:1-23. vii. [Crossref] [PubMed]
- Karrison TG, Ferguson DJ, Meier P. Dormancy of mammary carcinoma after mastectomy. J Natl Cancer Inst 1999;91:80-5. [Crossref] [PubMed]
- Schmidt-Kittler O, Ragg T, Daskalakis A, et al. From latent disseminated cells to overt metastasis: genetic analysis of systemic breast cancer progression. Proc Natl Acad Sci U S A 2003;100:7737-42. [Crossref] [PubMed]
- Witzel I, Oliveira-Ferrer L, Pantel K, et al. Breast cancer brain metastases: biology and new clinical perspectives. Breast Cancer Res 2016;18:8. [Crossref] [PubMed]
- Custódio-Santos T, Videira M, Brito MA. Brain metastasization of breast cancer. Biochim Biophys Acta Rev Cancer 2017;1868:132-47. [Crossref] [PubMed]
- Wu Q, Li J, Zhu S, et al. Breast cancer subtypes predict the preferential site of distant metastases: a SEER based study. Oncotarget 2017;8:27990-6. [Crossref] [PubMed]
- Medeiros B, Allan AL. Molecular Mechanisms of Breast Cancer Metastasis to the Lung: Clinical and Experimental Perspectives. Int J Mol Sci 2019;20:2272. [Crossref] [PubMed]
- Evans AJ, James JJ, Cornford EJ, et al. Brain metastases from breast cancer: identification of a high-risk group. Clin Oncol (R Coll Radiol) 2004;16:345-9. [Crossref] [PubMed]
- Tallet A, Kirova Y. Brain metastases from breast cancer: prognostic factors and tailored management. Bull Cancer 2013;100:63-7. [Crossref] [PubMed]
- Sperduto PW, Kased N, Roberge D, et al. Effect of tumor subtype on survival and the graded prognostic assessment for patients with breast cancer and brain metastases. Int J Radiat Oncol Biol Phys 2012;82:2111-7. [Crossref] [PubMed]
- Takahashi H, Isogawa M. Management of breast cancer brain metastases. Chin Clin Oncol 2018;7:30. [Crossref] [PubMed]
- Goldhirsch A, Wood WC, Coates AS, et al. Strategies for subtypes--dealing with the diversity of breast cancer: highlights of the St. Gallen International Expert Consensus on the Primary Therapy of Early Breast Cancer 2011. Ann Oncol 2011;22:1736-47. [Crossref] [PubMed]
- Perou CM, Sorlie T, Eisen MB, et al. Molecular portraits of human breast tumours. Nature 2000;406:747-52. [Crossref] [PubMed]
- Anders CK, Deal AM, Miller CR. The prognostic contribution of clinical breast cancer subtype, age, and race among patients with breast cancer brain metastases. Cancer 2011;117:1602-11. [Crossref] [PubMed]
- Musolino A, Ciccolallo L, Panebianco M. Multifactorial central nervous system recurrence susceptibility in patients with HER2-positive breast cancer: epidemiological and clinical data from a population-based cancer registry study. Cancer 2011;117:1837-46. [Crossref] [PubMed]
- Heitz F, Rochon J, Harter P. Cerebral metastases in metastatic breast cancer: disease-specific risk factors and survival. Ann Oncol 2011;22:1571-81. [Crossref] [PubMed]
- Collins FS, Varmus H. A new initiative on precision medicine. N Engl J Med 2015;372:793-5. [Crossref] [PubMed]
- Tibshirani R. Regression shrinkage and selection via the lasso: a retrospective. J Roy Stat Soc B 1996;58:267-88. [Crossref]
- Gui J, Li H. Penalized Cox regression analysis in the high-dimensional and low-sample size settings, with applications to microarray gene expression data. Bioinformatics 2005;21:3001-8. [Crossref] [PubMed]
- Thomas U, Phannavong B, Muller B, et al. Functional expression of rat synapse-associated proteins SAP97 and SAP102 in Drosophila dlg-1 mutants: effects on tumor suppression and synaptic bouton structure. Mech Dev 1997;62:161-74. [Crossref] [PubMed]
- Hanada N, Makino K, Koga H, et al. NE-dlg, a mammalian homolog of Drosophila dlg tumor suppressor, induces growth suppression and impairment of cell adhesion: possible involvement of down-regulation of beta-catenin by NE-dlg expression. Int J Cancer 2000;86:480-8. [Crossref] [PubMed]
- Kakunaga S, Ikeda W, Itoh S, et al. Nectin-like molecule-1/TSLL1/SynCAM3: a neural tissue-specific immunoglobulin-like cell-cell adhesion molecule localizing at non-junctional contact sites of pre-synaptic nerve terminals, axons and glia cell processes. J Cell Sci 2005;118:1267-77. [Crossref] [PubMed]
- Liu J, Li J, Li P, et al. Loss of DLG5 promotes breast cancer malignancy by inhibiting the Hippo signaling pathway. Sci Rep 2017;7:42125. [Crossref] [PubMed]
- Li D, Hu X, Yu S, et al. Silence of lncRNA MIAT-mediated inhibition of DLG3 promoter methylation suppresses breast cancer progression via the Hippo signaling pathway. Cell Signal 2020;73:109697 [Crossref] [PubMed]
- Liu J, Li P, Wang R, et al. High expression of DLG3 is associated with decreased survival from breast cancer. Clin Exp Pharmacol Physiol 2019;46:937-43. [Crossref] [PubMed]
(English Language Editors: C. Betlazar-Maseh and J. Chapnick)