Development and validation of a nomogram for prediction of lymph node metastasis in early-stage breast cancer
Introduction
As the cancer with the first morbidity and the second mortality among women worldwide, breast cancer has always remained a key field of cancer research (1). With the development of medical image processing technology and ultrasonic medicine, more and more breast cancer patients could be diagnosed in early-stage (2,3). Effective early treatments could significantly improve the prognosis of patients with early-stage breast cancer. Lymph node metastasis (LNM) status was recognized to be one of the most important prognostic factors in determining the stages and predicting the survival of invasive breast cancer patients (4). Sentinel lymph node biopsy (SLNB) can effectively assess axillary status in early clinically node-negative breast cancer (5,6). SLNB was recommended as a standard treatment/procedure for early-stage breast cancer patients who did not have nodal metastases with lower rates of morbidity and lymphedema than axillary lymph node dissection (ALND) (7). However, there remains 4.6% to 16.7% false negative rates of SLNB (8,9). Therefore, an appropriate prediction tool is urgently needed to evaluate LNM risk.
Many studies so far have identified some clinicopathologic features of the breast cancer as risk factors that may predict the LNM risk, such as tumor size, histologic grade, age of diagnosis, the status of hormone receptor (HR) and human epidermal growth factor receptor 2 (HER2) (10-12). A number of predictive models have been developed, including nomograms and scoring systems, which could combine statistically significant factors (13-16). Nomogram model is a kind of digital model characterized by the ability to predict the probability of a result event individually based on characteristics of patients and disease (17). For example, preoperative nomograms estimating the risk of positive surgical margins and LNM could help clinicians identify patients who might benefit more from more extensive surgery (13,18). Postoperative nomograms estimating recurrence, cancer-specific survival, benefit of adjuvant therapies and the effect of treatment on quality of life might assist patients and physicians in making decision in all aspects (19-21).
Herein, utilizing a huge population-based training cohort derived from the Surveillance, Epidemiology, and End Results (SEER) database, which contained 30 percent or more American, we developed a nomogram to predict LNM of T1 or T2 breast cancer patients, which was further confirmed by a validation cohort in which some samples from a Chinese institutional database. We present the following article in accordance with the TRIPOD and STROBE reporting checklists (available at http://dx.doi.org/10.21037/gs-20-782).
Methods
Patients
The patients were gathered from the SEER database (SEER 18 registry database using SEER*Stat 8.3.6.1 software) according to the following criterions: female, age at diagnosis (older than 20 years), year of diagnosis (2010–2015), race, site recode (breast), primary site of tumor, laterality, histological type, grade, American Joint Committee on Cancer (AJCC) stage, tumor size (T1–2), all lymph node (LN) stages, without distant metastasis (M0) and breast subtype. Patients had only one primary breast cancer were included. And patients whose important information was unknown or unavailable were excluded. The flow chart for cases selection was shown in Figure 1. Part of patients in validation set was comprised from the First Affiliated Hospital of Nanjing Medical University for medical records from June 2016 to June 2019 to identify patients with histologically confirmed breast cancer. Exclusion criteria included: (I) male gender; (II) tumor size larger than 5 cm; (III) previous breast cancer history; (IV) previous neoadjuvant therapy for breast carcinoma; (V) bilateral breast cancer; (VI) with distant metastasis. The study was conducted in accordance with the Declaration of Helsinki (as revised in 2013). The study was approved by the Ethical Committee of the First Affiliated Hospital of Nanjing Medical University (No. 2019-SRFA-197) and individual consent for this retrospective analysis was waived.
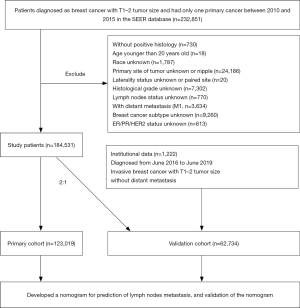
Statistical analysis
All patients from SEER were randomly divided into primary cohort and validation cohort in a 2:1 ratio. And data from the First Affiliated Hospital of Nanjing Medical University was included in the validation cohort. We performed Chi square test to examine the difference of demographics and tumor characteristics between patients with positive LNM or negative in primary cohort and validation one. Univariate and multivariate binary logistic regression analysis were performed to assess risk factors for LNM in early-stage breast cancer in the primary cohort. Univariate analysis incorporated all variables including age at diagnosis, race, primary site of tumor, laterality, histological type, grade, tumor size and subtype. And multivariate analysis included variables with statistical significance in univariate analysis. A nomogram was subsequently drawn according to the regression coefficient of each variables that were statistically significant in the multivariate analysis. Different variable values can get corresponding different integrals on the integration line at the top of the nomogram, then added the points of all variables to get the total score. The predicted risk of LNM can be obtained on the prediction line at the bottom of the nomogram through the total point line.
The model performance was evaluated by the predictive accuracy for individual outcomes (discriminating ability) and accuracy of point estimates of the LNM (calibration) (22). To quantify the discrimination of the LNM nomogram, Harrell’s C-index was measured. The receiver-operating characteristic (ROC) curves and the areas under the ROC curve (AUC) value were done to assess the sensitivity and specificity of the LNM nomogram in the primary and validation sets. The AUC value ranges from 0 to 1, and it is generally accepted that a considerable discrimination values of AUC is between 0.7 and 0.9; AUC values exceeding 0.9 represent good discrimination (23). Calibration curves were used to evaluate the calibration of the LNM nomogram. Bootstrap resampling (1,000 resamples) was used for this plot. External validation was conducted by a data from validation cohorts. Then the C-index and ROC curve of the validation group were derived on the basis of the regression analysis.
Based on the nomogram model, we figured up the total score of all patients and determined the best cut-off point value by using Youden’s index (24). According to the cut-off point, patients were divided into two groups in both cohorts. Univariate logistic regression analysis was performed using the total point as an independent factor.
The software IBM SPSS Statistics (version 25) and the programming language R (version 3.6.1, http://www.R-project.org) were applied to perform all statistical analyses. All reported P values were two-sided, and differences were considered statistically significant at P<0.05.
Results
Characteristics of patients in primary and validation cohorts
The demographic and clinical characteristics of T1–2 breast cancer patients in primary and validation cohorts were described in Table 1 and details of the institutional data were shown in Table S1. LNM was present in 32,512 of 123,019 patients (26.4%) in the primary set and 16,470 of 62,734 patients (26.3%) in the validation set. In the correlation analysis, significant differences were detected in all variables except laterality in the primary set. Patients with LNM presented a higher percent of younger women (<40 years old) and black person in both cohorts. Besides, patients with positive LN tented to exhibit unfavorable prognostic factors, such as worse differentiation (grade 3) and larger tumor size (T2) (P<0.001, for two factors). We also found that the primary site of breast cancer was significantly related to LNM (P<0.001). Breast cancer subtype of HR+/HER2+ (LN positive 12.8% vs. LN negative 9.2%) and HER2+ (LN positive 5.4% vs. LN negative 3.4%) were more common in patients with LNM in the primary cohort.

Full table
Independent significant factors in the primary cohort
Univariable logistic regression analysis found that age, race, primary site of tumor, histological type, laterality, grade, tumor size and breast cancer subtype were risk factors for LNM in early breast cancer in the primary cohort. Multivariable logistic regression analysis was performed to further identify the seven variables mentioned above except laterality were independent risk factors for LNM (Table 2). Compared with patients whose age at diagnosis was older than 40 years old, patients diagnosed at other ages were less prone to have positive LN [age: 40–49, odds ratio (OR) =0.814, P<0.001; age: 50–59, OR =0.693, P<0.001; age: 60–69, OR =0.573, P<0.001; age: ≥70, OR =0.444, P<0.001]. We also found that the black patients were more likely to occur LNM than white patients (OR =1.159, P<0.001). As for the primary site of tumor, the site of inner quadrant (OR =0.612, P<0.001) and overlapping (OR =0.865, P<0.001) were less likely to have positive LN but the site of central portion (OR =1.303, P<0.001) and axillary tail (OR =1.382, P<0.001) were more apt to LNM, compared to outer quadrant. Histological type also had influence to LNM. Invasive ductal carcinoma was more prone to have LNM. Besides, the patients who had higher tumor grade were more likely to have positive LN (grade 2 vs. grade 1, OR =1.740, P<0.001; grade 3 vs. grade 1, OR =2.119, P<0.001). The rate of LNM in lager tumor size was higher than small ones (T2 vs. T1, OR =3.464, P<0.001). In addition, the results showed that triple-negative breast cancer (TNBC) (P<0.001) patients had lower LNM rate compared to HR+/HER2− patients. And compared with HR+/HER2− patients, there was no significant difference in HR+/HER2+ patients (P=0.540). Same result was shown in HR−/HER2+ patients (P=0.565).
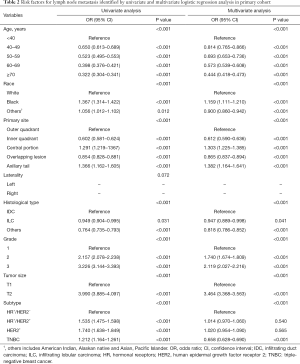
Full table
Development of nomogram for LNM prediction
We next developed a nomogram combining the significant predictive factors from the analysis of multiple factors (Figure 2). Seven variables including age of diagnosis, race, primary site of tumor, histological type, grade, tumor size and breast cancer subtype were contained in the nomogram. Besides, Table 3 exhibited the point assignments and predictive scores for each variable in the nomogram model. By calculating the total points for each variable, the likelihood of LN positivity in a particular patient can be predicted.
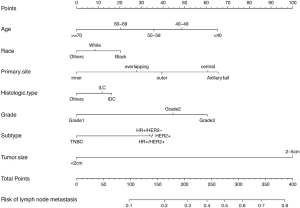
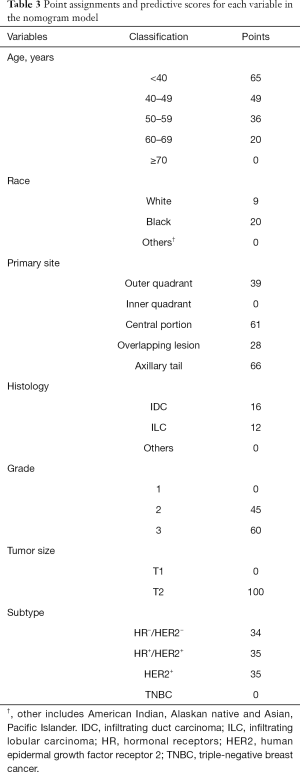
Full table
Performance and validation of nomogram for LNM prediction
The calibration curve of the nomogram for the prediction of LNM demonstrated good agreement in primary cohort (Figure 3A) and validation cohort (Figure 3B). The C-index for the predictive nomogram was 0.720 (95% CI: 0.717–0.723) for the primary cohort, and similar C-index of 0.718 was found in the validation cohort. We performed ROC analysis to verify the predictive values of the nomogram model. The AUC values of the nomogram were 0.720 (95% CI: 0.716–0.723) and 0.718 (95% CI: 0.714–0.723), respectively in the primary (Figure 4A) and validation cohorts (Figure 4B).
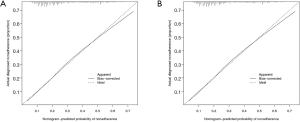
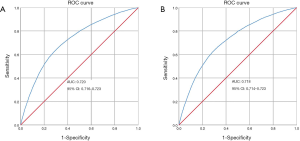
Stratifying patient risk based on the nomogram
We next summed all patient total points according the LNM nomogram. Then we identified the best cut-off point as 189 based on the Youden’s index in the primary cohort. The patients were stratified into two groups: low risk group (total point ≤189) and high-risk group (total point >189) in both cohorts. Logistic regression analysis found that patients in high-risk group were more prone to have LNM than those in low-risk group (OR =4.126, P<0.001) in primary cohort (Table 4). Same outcome was shown in the validation cohort (OR =4.029, P<001).

Full table
Discussion
LNM is an important risk factor for the prognosis of breast cancer, underscoring the importance of accurate nodal status. We established and validated a nomogram based on clinic-pathologic signature for predicting LNM in particular patient with T1–2 breast cancer. Incorporating clinical risk factors into an easy-to-use nomogram promotes the individualized prediction of total LNM in the small breast cancer, thereby assisting doctors in formulating suitable individual treatments.
Our study found that age at diagnosis, race, primary site, histologic type, histologic grade, tumor size and tumor subtype were related to LNM in T1–2 breast cancer. Young breast cancer patients are a unique group, who are usually characterized with more aggressive tumors and worse prognosis (25). We also found that the risk of LN involvement decreases with women’s age from youngest to oldest. Younger patients (age <40 years old) were more likely to have LNM than other age groups, and same results were shown in other researches (14,26). Moreover, increasing tumor histologic grade was significantly associated with an increased risk of LNM. Tumor size showed a positive correlation with the LN involvement. The incidence of LNM was more presented in the larger tumor. Women with a tumor size >2 cm had a significantly higher risk of LN metastasis than those with tumor ≤2 cm (27). Race also had a significant influence on LNM. Blacks were the most prone to LNM, followed by whites, and other races (including American Indian, Alaskan native and Asian, Pacific Islander) last.
Our study observed a lower frequency of LNM in inner quadrant location tumors. The similar result was published by another study (28). This was hypothesized to result from increased lymphatic flow through the axillary nodes rather than the internal mammary nodes as compared to more medial tumors. However, others considered that tumors from inner breast quadrants tend to have less favorable prognosis (29,30). Their “hidden” nature makes physical examination and imaging detection difficult, which leads to under staging and undertreatment.
We discovered that HER2-positive tumors were associated with more LN positivity than HER2-negative tumors in primary cohort (18.2% vs. 12.6%). Lu et al. confirmed that the lowest probability of node metastasis was in estrogen receptor (ER)−/HER2− cancers (31). Similarly, in our study, triple negative tumors had the lowest probability of LNM, while HR−/HER2+ tumors had the highest probability, which was in agreement with the findings of previous study (26). Reyal et al. hypothesized that the process of axillary LNM was mainly related to intrinsic biological characteristics in the ER−/HER2− breast cancer subgroup, while tumor size, growth rate and lympho-vascular invasion were the main determinants in both the ER positive or the HER2 positive breast cancer subgroups (32).
Compared with other researches, the present study was the first to develop a simple nomogram to predict overall LNM in T1–2 breast cancer patients using SEER database. Previous studies had developed plentiful nomograms and scoring models (13-16,33-36). Many models have focused on calculating the risk of sentinel axillary node (14,15,35) or additional non-sentinel node disease axillary LNs (13,34), but few models predicted total LNM. Our nomogram can be widely used with less limitation. The variables involved in the nomogram were available before surgery. Our model was developed form SEER database which contains many centers in America. Moreover, our validation cohort contained some patients from First Affiliated Hospital of Nanjing Medical University. Few studies included race as a risk factor in the prediction model, while our study identified race as an independent risk factor for LNM.
We could discuss the clinical utility of our nomogram in different scenarios. SLNB is widely accepted as a vital method to make a reliable assessment of the axilla status in early-stage breast cancer. However, SLNB success decreased with increasing body mass, tumor location other than the upper outer quadrant and grade 3 tumors (37). Our study also found that outer quadrant and grade 3 were independent risk factors for LNM. Therefore, variable false-negative rate of SLNB varied with patients with different clinicopathologic features. What’s more, SLNB after new adjuvant therapy (NAT) could increase false negative rates and TNBC patients showed a significantly higher SLNB false negative rate than non-TNBC patients with NAT before SLNB (38). Additionally, SLNB is still an invasive procedure and could produce complications, including pain, paraesthesia and lymphedema (39). Thus, incorporating our prediction nomogram with other medical examination results could help comprehend lymph status better. SLNB might be exempted in patients who are predicted to have a lower possibility of LNM in the light of our nomogram. Although validation studies have confirmed the satisfactory precision of the previous models, their accuracy is often reduced outside the centers of initial development (40). Our validation cohort, however, contained some patients from a Chinese institutional database. Few studies included race as a risk factor in the prediction model, yet our study identified race as an independent risk factor for LNM which indicated that our nomogram could be used broadly.
One of the advantages of our study lied in the considerable number of breast cancer patients in the SEER database, which ensured the strength and objectivity of our conclusions. Nevertheless, there are some shortcomings in the present study that cannot be neglected. Frist of all, our Chinese institutional data was relatively small, thus we added these patients into the validation cohort, which could cause some biases. And, most of the cases may depend on the SEER data patients’ group because the number of our institutional data was less compared with the SEER data patients. Second, the presence of lymphovascular invasion (LVI) as an important predictor for LN involvement is well accepted in the previous studies (41,42). However, our study cannot obtain such vital information from SEER database. Moreover, we could not tell whether patients had received NAT or not in that NAT can modify lymphatic drainage patterns due to fibrosis and downstage (43). In addition, our study was a retrospective study, and election bias or information bias can could be scarcely avoided.
Conclusions
We developed and validated a nomogram based on clinic-pathologic signature to predict the LNM in individual patients with small breast cancer. The AUC value of the present nomogram for predicting LNM was 0.720, which showed good performance for the evaluation of LN status. Applying the nomogram to the validation cohort still provided good discrimination. The calibration curve shows that the nomogram prediction is consistent with the actual LN metastasis rate, which could assist doctors in formulating suitable individual treatments.
Acknowledgments
We wish to acknowledge Dr. Xin Wang and Dr. Yiqiu Liu for their linguistic assistance during the preparation of this manuscript.
Funding: This work was supported by National Natural Science Foundation of China (No. 81773102), and Key International Cooperation of National Natural Science Foundation of China (No. 81920108029).
Footnote
Reporting Checklist: The authors have completed the TRIPOD and STROBE reporting checklists. Available at http://dx.doi.org/10.21037/gs-20-782
Data Sharing Statement: Available at http://dx.doi.org/10.21037/gs-20-782
Conflicts of Interest: All authors have completed the ICMJE uniform disclosure form (available at http://dx.doi.org/10.21037/gs-20-782). The authors have no conflicts of interest to declare.
Ethical Statement: The authors are accountable for all aspects of the work in ensuring that questions related to the accuracy or integrity of any part of the work are appropriately investigated and resolved. The study was conducted in accordance with the Declaration of Helsinki (as revised in 2013). The study was approved by the Ethical Committee of the First Affiliated Hospital of Nanjing Medical University (No. 2019-SRFA-197) and individual consent for this retrospective analysis was waived.
Open Access Statement: This is an Open Access article distributed in accordance with the Creative Commons Attribution-NonCommercial-NoDerivs 4.0 International License (CC BY-NC-ND 4.0), which permits the non-commercial replication and distribution of the article with the strict proviso that no changes or edits are made and the original work is properly cited (including links to both the formal publication through the relevant DOI and the license). See: https://creativecommons.org/licenses/by-nc-nd/4.0/.
References
- Siegel RL, Miller KD, Jemal A. Cancer statistics, 2019. CA Cancer J Clin 2019;69:7-34. [Crossref] [PubMed]
- Jacklyn G, McGeechan K, Irwig L, et al. Trends in stage-specific breast cancer incidence in New South Wales, Australia: insights into the effects of 25 years of screening mammography. Breast Cancer Res Treat 2017;166:843-54. [Crossref] [PubMed]
- Vale DB, Filho CC, Shinzato JY, et al. Downstaging in opportunistic breast cancer screening in Brazil: a temporal trend analysis. BMC Cancer 2019;19:432. [Crossref] [PubMed]
- Choi HY, Park M, Seo M, et al. Preoperative Axillary Lymph Node Evaluation in Breast Cancer: Current Issues and Literature Review. Ultrasound Q 2017;33:6-14. [Crossref] [PubMed]
- Lyman GH, Giuliano AE, Somerfield MR, et al. American Society of Clinical Oncology guideline recommendations for sentinel lymph node biopsy in early-stage breast cancer. J Clin Oncol 2005;23:7703-20. [Crossref] [PubMed]
- Veronesi U, Paganelli G, Viale G, et al. Sentinel-lymph-node biopsy as a staging procedure in breast cancer: update of a randomised controlled study. Lancet Oncol 2006;7:983-90. [Crossref] [PubMed]
- Lyman GH, Somerfield MR, Bosserman LD, et al. Sentinel Lymph Node Biopsy for Patients with Early-Stage Breast Cancer: American Society of Clinical Oncology Clinical Practice Guideline Update. J Clin Oncol 2017;35:561-4. [Crossref] [PubMed]
- Veronesi U, Viale G, Paganelli G, et al. Sentinel lymph node biopsy in breast cancer: ten-year results of a randomized controlled study. Ann Surg 2010;251:595-600. [Crossref] [PubMed]
- Zavagno G, De Salvo GL, Scalco G, et al. A Randomized clinical trial on sentinel lymph node biopsy versus axillary lymph node dissection in breast cancer: results of the Sentinella/GIVOM trial. Ann Surg 2008;247:207-13. [Crossref] [PubMed]
- Patani NR, Dwek MV, Douek M. Predictors of axillary lymph node metastasis in breast cancer: a systematic review. Eur J Surg Oncol 2007;33:409-19. [Crossref] [PubMed]
- Kolarik D, Pecha V, Skovajsova M, et al. Predicting axillary sentinel node status in patients with primary breast cancer. Neoplasma 2013;60:334-42. [Crossref] [PubMed]
- Tseng HS, Chen LS, Kuo SJ, et al. Tumor characteristics of breast cancer in predicting axillary lymph node metastasis. Med Sci Monit 2014;20:1155-61. [Crossref] [PubMed]
- Van Zee KJ, Manasseh DM, Bevilacqua JL, et al. A nomogram for predicting the likelihood of additional nodal metastases in breast cancer patients with a positive sentinel node biopsy. Ann Surg Oncol 2003;10:1140-51. [Crossref] [PubMed]
- Bevilacqua JL, Kattan MW, Fey JV, et al. Doctor, what are my chances of having a positive sentinel node? A validated nomogram for risk estimation. J Clin Oncol 2007;25:3670-9. [Crossref] [PubMed]
- Chen JY, Chen JJ, Yang BL, et al. Predicting sentinel lymph node metastasis in a Chinese breast cancer population: assessment of an existing nomogram and a new predictive nomogram. Breast Cancer Res Treat 2012;135:839-48. [Crossref] [PubMed]
- Barranger E, Coutant C, Flahault A, et al. An axilla scoring system to predict non-sentinel lymph node status in breast cancer patients with sentinel lymph node involvement. Breast Cancer Res Treat 2005;91:113-9. [Crossref] [PubMed]
- Kattan MW, Scardino PT. Evidence for the usefulness of nomograms. Nat Clin Pract Urol 2007;4:638-9. [Crossref] [PubMed]
- Shin HC, Han W, Moon HG, et al. Nomogram for predicting positive resection margins after breast-conserving surgery. Breast Cancer Res Treat 2012;134:1115-23. [Crossref] [PubMed]
- Wang Y, Xu L, Du T, et al. A Nomogram Predicting Recurrence and Guiding Adjuvant Radiation for Thymic Carcinoma After Resection. Ann Thorac Surg 2018;106:257-63. [Crossref] [PubMed]
- Brockman JA, Alanee S, Vickers AJ, et al. Nomogram Predicting Prostate Cancer-specific Mortality for Men with Biochemical Recurrence After Radical Prostatectomy. Eur Urol 2015;67:1160-7. [Crossref] [PubMed]
- Lubelski D, Alentado V, Nowacki AS, et al. Preoperative Nomograms Predict Patient-Specific Cervical Spine Surgery Clinical and Quality of Life Outcomes. Neurosurgery 2018;83:104-13. [Crossref] [PubMed]
- Kim Y, Margonis GA, Prescott JD, et al. Nomograms to Predict Recurrence-Free and Overall Survival After Curative Resection of Adrenocortical Carcinoma. JAMA Surg 2016;151:365-73. [Crossref] [PubMed]
- Qiu PF, Liu JJ, Wang YS, et al. Risk factors for sentinel lymph node metastasis and validation study of the MSKCC nomogram in breast cancer patients. Jpn J Clin Oncol 2012;42:1002-7. [Crossref] [PubMed]
- Li C, Chen J, Qin G. Partial Youden index and its inferences. J Biopharm Stat 2019;29:385-99. [Crossref] [PubMed]
- Collins LC, Marotti JD, Gelber S, et al. Pathologic features and molecular phenotype by patient age in a large cohort of young women with breast cancer. Breast Cancer Res Treat 2012;131:1061-6. [Crossref] [PubMed]
- Houvenaeghel G, Lambaudie E, Classe JM, et al. Lymph node positivity in different early breast carcinoma phenotypes: a predictive model. BMC Cancer 2019;19:45. [Crossref] [PubMed]
- Howland NK, Driver TD, Sedrak MP, et al. Lymph node involvement in immunohistochemistry-based molecular classifications of breast cancer. J Surg Res 2013;185:697-703. [Crossref] [PubMed]
- Siotos C, McColl M, Psoter K, et al. Tumor Site and Breast Cancer Prognosis. Clin Breast Cancer 2018;18:e1045-52. [Crossref] [PubMed]
- Bao J, Yu KD, Jiang YZ, et al. The effect of laterality and primary tumor site on cancer-specific mortality in breast cancer: a SEER population-based study. PLoS One 2014;9:e94815 [Crossref] [PubMed]
- Hwang KT, Kim J, Kim EK, et al. Poor Prognosis of Lower Inner Quadrant in Lymph Node-negative Breast Cancer Patients Who Received No Chemotherapy: A Study Based on Nationwide Korean Breast Cancer Registry Database. Clin Breast Cancer 2017;17:e169-84. [Crossref] [PubMed]
- Lu X, Lu X, Wang ZC, et al. Predicting features of breast cancer with gene expression patterns. Breast Cancer Res Treat 2008;108:191-201. [Crossref] [PubMed]
- Reyal F, Rouzier R, Depont-Hazelzet B, et al. The molecular subtype classification is a determinant of sentinel node positivity in early breast carcinoma. PLoS One 2011;6:e20297 [Crossref] [PubMed]
- Cho J, Han W, Lee JW, et al. A scoring system to predict nonsentinel lymph node status in breast cancer patients with metastatic sentinel lymph nodes: a comparison with other scoring systems. Ann Surg Oncol 2008;15:2278-86. [Crossref] [PubMed]
- Canavese G, Bruzzi P, Catturich A, et al. A risk score model predictive of the presence of additional disease in the axilla in early-breast cancer patients with one or two metastatic sentinel lymph nodes. Eur J Surg Oncol 2014;40:835-42. [Crossref] [PubMed]
- Dihge L, Bendahl PO, Ryden L. Nomograms for preoperative prediction of axillary nodal status in breast cancer. Br J Surg 2017;104:1494-505. [Crossref] [PubMed]
- Zhang J, Li X, Huang R, et al. A nomogram to predict the probability of axillary lymph node metastasis in female patients with breast cancer in China: A nationwide, multicenter, 10-year epidemiological study. Oncotarget 2017;8:35311-25. [Crossref] [PubMed]
- Goyal A, Newcombe RG, Chhabra A, et al. Factors affecting failed localisation and false-negative rates of sentinel node biopsy in breast cancer--results of the ALMANAC validation phase. Breast Cancer Res Treat 2006;99:203-8. [Crossref] [PubMed]
- Han A, Moon HG, Kim J, et al. Reliability of sentinel lymph node biopsy after neoadjuvant chemotherapy in breast cancer patients. J Breast Cancer 2013;16:378-85. [Crossref] [PubMed]
- McLaughlin SA, Wright MJ, Morris KT, et al. Prevalence of lymphedema in women with breast cancer 5 years after sentinel lymph node biopsy or axillary dissection: patient perceptions and precautionary behaviors. J Clin Oncol 2008;26:5220-6. [Crossref] [PubMed]
- Dingemans SA, de Rooij PD, van der Vuurst de Vries RM, et al. Validation of Six Nomograms for Predicting Non-sentinel Lymph Node Metastases in a Dutch Breast Cancer Population. Ann Surg Oncol 2016;23:477-81. [Crossref] [PubMed]
- de Oliveira Filho HR, Doria MT, Piato JR, et al. Criteria for prediction of metastatic axillary lymph nodes in early-stage breast cancer. Rev Bras Ginecol Obstet 2015;37:308-13. [Crossref] [PubMed]
- Chas M, Boivin L, Arbion F, et al. Clinicopathologic predictors of lymph node metastasis in breast cancer patients according to molecular subtype. J Gynecol Obstet Hum Reprod 2018;47:9-15. [Crossref] [PubMed]
- Garcia-Tejedor A, Falo C, Quetglas C, et al. Feasibility, accuracy and prognosis of sentinel lymph node biopsy before neoadjuvant therapy in breast cancer. A prospective study. Int J Surg 2017;39:141-7. [Crossref] [PubMed]