Identification of differentially expressed genes and signaling pathways in papillary thyroid cancer: a study based on integrated microarray and bioinformatics analysis
Introduction
Papillary thyroid carcinoma (PTC) is a typical indolent cancer, and in the last 30 years, a rapidly increasing incidence of PTC has been observed throughout the world without sex or ethnicity bias. In the United States, the incidence of PTC disease has increased rapidly from 3.4 to 12.5 in 100,000 people, an increase of 3.7 times (1). Experts increasingly argue that low-risk papillary thyroid microcarcinomas (PTMCs) have contributed primarily to this increasing incidence, and active surveillance should be sufficient to manage those cancers; however, 10–15% of patients with PTMC will suffer from tumor relapse (2), and approximately 5% of PTMC patients will experience some metastasis (3). The dilemma of treating PTC is how to differentiate indolent cancer from aggressive cancer. Based on the above analysis, it is of great significance to identify and develop new genes in tumor biology, as well as genes with therapeutic and prognostic effects.
PTC has multiple histopathological variants, and each condition also has different molecular changes, including somatic mutations, such as BRAF (4) and TERT (5), as well as gene rearrangements, such as RET/PTC1, AKAP9/BRAF and NTRK1/TMP3 (6). The most frequent missense somatic mutation of PTC is BRAFV600E, which, substitutes an A for a T at nucleotide 1799, changing valine to glutamic acid at amino acid 600 (4). The prognostic effect of BRAFV600E is somewhat divergent: some studies indicate the poor prognosis of BRAFV600E (7-9), but other studies have not shown a correlation (10-12). There are some TERT promoter mutations in approximately 11% of PTCs (5), which are major indicators of poor prognosis. Coexistence of TERT promoter mutations and BRAFV600E has a more obvious and prominent correlation with aggressive behavior (13). A large meta-analysis identified miR-2861, miR-1271, miR-623, miR-451, miR-222, miR-221, miR-199b-5p, miR-181b, and miR-151. Comparison and analysis of 146b, miR-135b, miR-130b, miR-34b, miR-21 and let-7e with PTC identified the presence of at least one aggressive characteristic with a particularly obvious correlation (14). Abnormally expressed long noncoding RNAs (lncRNAs) have been reported to have a functional role in carcinogenesis (15). In PTC, the lncRNAs PTCSC2 (16), ANRIL (17), and MALAT1 (18) were demonstrated to be oncogenes that act by altering chromatin structure or by regulating the expression of protein-coding genes. Many research studies have compared and analyzed the mutation characteristics and gene expression of PTC tumors with surrounding non-tumor thyroid tissue genes in detail. Studies of some specific genes have shown that thyroid cancer is different from normal tissues, and many drivers have been identified. However, studies describing the core molecular features of PTC that distinguish it from normal thyroid tissue through integrated analysis of Gene Expression Omnibus (GEO) microarray data from a large volume of samples are still very rare. Our study attempted to verify the differentially expressed genes in a large clinical samples from department of head and neck surgery, Fudan University Shanghai Cancer Center (FUSCC) which might make the results more stable and reliable. Furthermore, we adopted Robust Multichip Average (RMA) algorithm normalization to preprocess the matrix file and platform. RMA is a quantitative normalization algorithm and it can unify the data between different groups, reduce the intra-group differences and eliminate the non-experimental differences of measurement.
We present the following article in accordance with the MDAR reporting checklist (available at http://dx.doi.org/10.21037/gs-20-673).
Methods
Microarray data
We completed an effective search in the GEO database (https://www.ncbi.nlm.nih.gov/geo/) to find PTC microarray data. The keywords in the search process were as follows: papillary thyroid carcinoma, papillary thyroid carcinoma, thyroid cancer, and thyroid tumor. The following criteria were used to screen the dataset and ensure relevant data were recorded: (I) the sample includes normal thyroid tissue and papillary thyroid carcinoma tissue; (II) the core aim of the research is to complete analysis of the expression profile based on the array method; (III) the species is limited to Homo sapiens; (IV) access to raw data is allowed. Then, the gene expression profiles of GSE33630, GSE35570, GSE60542 and GSE29265 were downloaded. The GSE33630 dataset was used to perform an effective analysis of multiple contents, including gene expression of 45 cases of normal thyroid (N), 49 cases of PTC and 11 cases of anaplastic thyroid carcinoma (ATC). ATC is not within the scope of our study, so our study included 45 cases of normal thyroid (N) and 49 cases of papillary thyroid carcinoma (PTC). The GSE35570 dataset includes 65 PTC patients and 51 normal thyroid samples, which were all included in our study. A total of 92 samples were included in GSE60542. LNM samples [23], recurrence samples [1], normal lymph node samples [4], and pleural metastasis samples [1] were excluded. Therefore, we included 33 cancer samples and 30 normal samples. GSE29265: Twenty PTCs and 9 ATCs and surrounding tissues (N) were matched and hybridized with the Affymetrix U133 Plus 2.0 array. PTC was collected from the Chernobyl Organization Bank (post-Chernobyl PTC, n=10) and Ambroise Paré Hospital (sporadic PTC, n=10). We only chose the 10 sporadic PTCs and matched adjacent tissues. The platform for the four categories of datasets is the GPL570, [HG-U133_Plus_2] Affymetrix Human Genome U133 Plus 2.0 array, covering 157 papillary thyroid carcinoma specimens and 136 normal samples. Series matrix files and platforms are downloaded as CEL files. The main content of the dataset is shown in Table 1.
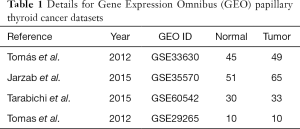
Full table
DEG identification and integration
Processing of the downloaded matrix file and platform was completed with R Project version 4.0.2 and the annotation package. The ID corresponding to the probe name was converted into the international standard name of the gene (that is, the gene symbol) and deployed to a csv file. Preprocessing was based on RMA algorithm normalization in the Affy software package (http://www.bioconductor.org/packages/release/bioc/html/affy.html). The limma software package (http://www.bioconductor.org/packages/release/bioc/html/limma.html) was used to complete the DEG analysis of papillary thyroid cancer (PTC) and normal thyroid samples from four microarray datasets. The traditional t-test process was completed, and DEG statistical significance identification with a P value <0.05 and log2 (FC) >1 was performed. The heatmaps were produced by heatmap 2 in the gplots package (https://cran.r-project.org/web/packages/gplots/). Venn analysis (Venn) was used to integrate the DEG changes that often appear in the dataset.
GO and KEGG pathway enrichment analyses of DEGs
The visualization, annotation and integrated discovery database (DAVID) contains the core content of high-throughput gene function analysis (https://david.ncifcrf.gov/). Pathway and functional enrichment of the protein encoded by each candidate gene was carried out, and then, the DAVID database was used to realize gene annotation. Gene ontology (GO) analysis is mainly used to effectively identify the biological characteristics of DEGs. GO annotation was performed on DEGs using the DAVID online tool. GO analysis included three different categories: biological process (BP), cellular component (CC), and molecular function (MF). Fold enrichment was calculated for each GO term using the formula Fold Enrichment = (k/n)/(M/N). The enrichment analysis process of the “Kyoto Encyclopedia of Genes and Genomes” (KEGG) approach was completed so that the functional content of DEGs could be obtained. The KOBAS online analysis database was used to complete KEGG pathway analysis processing of DEGs (online available: http://kobas.cbi.pku.edu.cn/). During this process, we compared and analyzed in detail the significant upregulation and downregulation of DEGs based on the integrated microarray PTC data, and a false discovery rate (FDR) of <0.05 was considered to be statistically significant.
Protein-protein interaction (PPI) network integration
The mutual function of proteins can provide a background for the molecular mechanisms of cellular processes. A search tool was used to effectively search the PPI network constructed by the gene database (STRING, version 10.5, URL: https://string-db.org/) and was visualized using Cytoscape (version 3.7.1, https://cytoscape.org/download.html). Each node is a molecule, protein, or gene, and each connection between the nodes acts as a molecule to identify the internal pathways and functions of the DEG-encoded proteins in PTC. The protein in the central node may be an important candidate gene with an important function in physiological regulation or a core protein.
Tissue samples
The study was conducted in accordance with the Declaration of Helsinki (as revised in 2013). All of the tissue specimens were obtained for the present study with informed consent from the patients, and the use of the human specimens was approved by the Institutional Ethics Committee of FUSCC. The ID of ethical approval was 050432-4-1911D. All procedures performed in our study were consistent with the ethical standards of our institutional research committee. Biopsy samples of adjacent tissues and thyroid cancer tissues were collected. The distance between the adjacent tissues and the edge of the tumor was 1 cm; relevant experts evaluated the samples and found no obvious tumor cells. We only collected papillary thyroid carcinoma; other pathological types, including follicular thyroid carcinoma, medullary thyroid carcinoma, ATC, non-epithelial tumors and secondary tumors, were not included. In addition, variants of papillary thyroid carcinoma (classic, encapsulated, follicular, diffuse sclerosing, tall cell, etc., according to Pathology of Endocrine Tumors Update: World Health Organization New Classification 2017) were not differentiated in our research.
RNA extraction and quantitative real-time PCR (qPCR)
In accordance with the instructions of the manufacturer, Life Technology Company in Carlsbad, California, the effective extraction of total RNA was completed by relying on TRIzol reagent. RNA measurement was based on a SmartSpec Plus spectrophotometer (Bio-Rad, Hercules, CA, USA). The assessment of purity relied on the A260/A280 ratio. Then, reverse transcription (RT) reactions were performed. Oligo dT primers were used to realize the RT reaction process of mRNA. The YBR Green PCR kit (Toyobo, Osaka, Japan) was used to perform qPCR on an Applied Biosystems 7300 real-time PCR system (Applied Biosystems, Foster City, CA). The main reference target of mRNA was β-actin. The following primers were used: ENTPD1-forward 5'-CAGCCTTGGGAGGAGATAAA-3' and ENTPD1-reverse 5'-GAGAGAGGTGTGGACAATGGTT-3', THRSP-forward 5'-CTTCTCCAGCCTCCATCACA-3' and THRSP-reverse 5'-TCCGCCGACCTCATCAA-3', KLK10-forward 5'-GGTCTTCTACCCTGGCGTG-3' and KLK10-reverse 5'-CTTGGAGGGTCTCGTCACAG-3', ADAMTS9-forward 5'-TGGATACACCGCAAACGACT-3' and ADAMTS9-reverse 5'-GTTCACC GTTGGACCGCT-3', MIR31HG-forward 5'-AGAGCCCTCAATCACCCACT-3' and MIR31HG-reverse 5'-ACCAACTCCACACTTTACGTCAT-3', SCARA5-forward 5'-AAAGCTATGTACCTACACACCGT-3' and SCARA5-reverse 5'-CCGCCGTTTGTGACATGGA-3', EPHB1-forward 5'-CTTTCTGTCCGTGTCTTCTTCA-3' and EPHB1-reverse 5'-CACGCTGTTCTCAGGCTCAT-3', CHRDL1-forward 5'-CTTATGGGTTGGTTTACTGCG-3' and CHRDL1-reverse 5'-CACAGGAGAAAGGCAATGA AC-3', LOC440934-forward 5'-GCCGTAGTGGTTGACGAAGA-3' and LOC440934-reverse 5'-TGGGGCATCTTGCTGTCTT-3', and FOXP2-forward 5'-GCATTGGATGACCGAAGCA-3' and FOXP2-reverse 5'-CCAGATTTAGAGG TTTGGGAGA-3'.
Statistical analysis
All data are representative of each assay repeated independently at least three times. Quantitative data are presented as the mean ± standard deviation (SD). R project software (version 4.0.2) was used to conduct all statistical tests and produce the resulting graphics. We use the Jarque-Bera test to examine the normal distribution characteristics of the quantitative data. Furthermore, we checked the qPCR data using the Jarque-Bera test. The Bayes test was used to screen the differentially expressed genes (DEGs) between PTC and normal tissues. The Wilcoxon signed-rank test was used to screen the DEGs between PTC and normal tissues. P values <0.05 were considered significant.
Results
Microarray data information and identification of DEGs in PTC
The GEO and ArrayExpress databases were searched for PTC-relevant mRNA microarray data using the following phrases: [(thyroid OR papillary OR follicular) AND (tumor OR tumor OR cancer OR carcinoma) AND (mRNA)]. After the initial search, we found four DNA microarray datasets that were combined to complete the effective identification of DEGs, including GSE29265, GSE33630, GSE35570 and GSE60542. All four datasets were generated by the same platform: GPL570, Affymetrix Human Genome U133 Plus 2.0 Array. This platform includes 47,000 transcripts from 38,500 human genes based on the NCBI human genome, Washington University EST trace repository, UniGene database, RefSeq, dbEST, and GenBank assembly. Combining the microarray data from multiple studies could effectively expand the sample size and improve the statistical power of detecting DEGs, which may provide a more distinct picture of tumorigenesis and the development of PTC.
After a normalization and standardization process, the content of Figure 1 was obtained. The limma software package was used to screen the GSE33630 dataset (corrected logFC >1, P value <0.05) and collect 1,813 DEGs. There were 816 upregulated and 997 downregulated genes. In general, 1,615 DEGs were selected from the GSE60542 dataset, including 823 downregulated and 792 upregulated genes. A total of 4,668 DEGs were selected from the GSE35570 dataset, including 2,247 downregulated and 2,421 upregulated genes. A total of 1,200 DEGs were selected from the expression profile dataset GSE29265, including 523 downregulated and 677 upregulated genes. The cluster heatmaps of each dataset are shown in Figure 2. The q-q plot provides a visual comparison of the sample quantiles to the corresponding theoretical quantiles and is used to test whether the data conform to a normal distribution. The data approximate a straight line and a normal distribution.
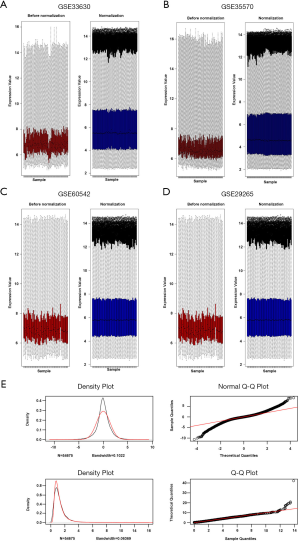
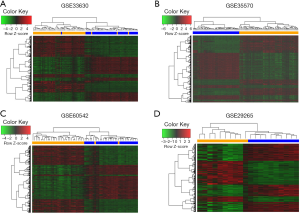
Following the normalization of RMA and analysis by ebayes, we identified 590 consistently expressed genes by Venn analysis (Figure 3). We used the same screening criteria to calculate four groups of datasets with DEGs and then calculated them by Venn to find the overlapping genes. We found that GSE35570 had the most unique genes in terms of total numbers, which may be related to the sample age, sex, and clinical stage. This represents a limitation of the study, which will be improved and explored in the future. There were 263 downregulated and 327 upregulated genes (Table 2).
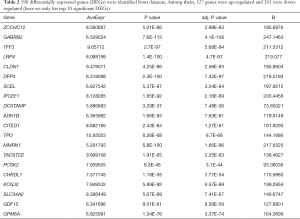
Full table
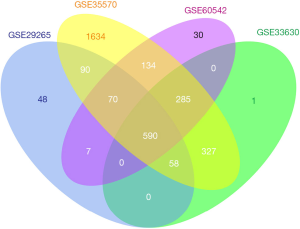
Gene ontology analysis
Gene ontology describes the concept and function of genes. Data processing and analysis were completed using the DAVID online analysis tool. P<0.05 was used as the cutoff criterion. DEGs included three functional groups (Figure 4): (I) biological stage; (II) MF; and (III) CCs. Tables 3 and 4 show the core results of the GO enrichment analysis of DEGs in PTC. The most enriched GO terms were extracellular exosome (GO:0070062), cell adhesion (GO:0070062), positive regulation of gene expression (GO:0010628), and extracellular matrix (ECM) organization (GO:0030198).
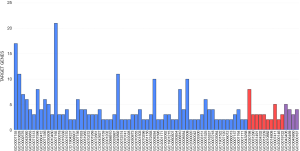
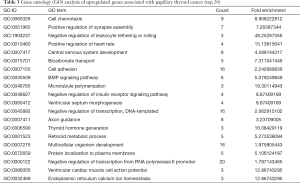
Full table
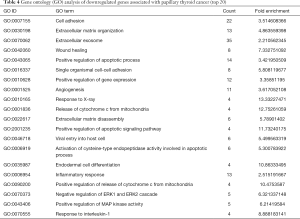
Full table
Identification of DEG-regulated pathways
We used the KEGG online analysis database (http://www.kegg.jp/kegg/kegg2.html) to complete processing of the gene microarray and realize the effective identification of DEGs (Table 5). The signal transduction process of DEGs was enriched in cell adhesion molecules (CAMs) (P<0.001), transcriptional misregulation in cancer (P=0.0144), and tyrosine metabolism (P<0.001) (Figures 5,6). In addition, using the STRING database (http://string-db.org) for analysis, a PPI network of DEG expression in PTC was generated. There were 165 nodes and 117 edges in the PPI network. The constructed DEG network is shown in Figure 7.
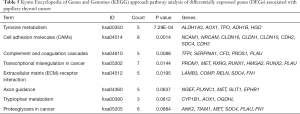
Full table
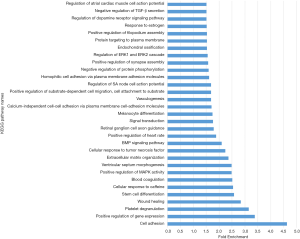
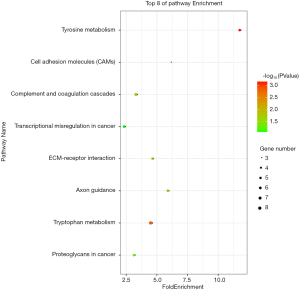
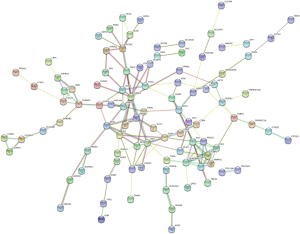
Clinical validation of candidate genes by qRT-PCR analysis
To verify the calculated data, ten DEGs, including five upregulated (ENTPD1, THRSP, KLK10, ADAMTS9, MIR31HG) and five downregulated (SCARA5, EPHB1, CHRDL1, LOC440934, FOXP2) genes, were randomly selected for adjacent noncancerous tissue samples and q-PCR in 50 pairs of PTCs. The 2−ΔΔCt function was used to calculate differential gene expression. The log2 (2−ΔΔCt) value was used to complete the relative expression mapping of the 10 identified genes in Figure 8 through the R project (version 3.3). The results were consistent with the integrated data (Figure 8).
Discussion
In this study, we integrated four GEO datasets, GSE33630, GSE35570, GSE60542 and GSE29265, including 136 normal samples and 157 PTC specimens. A total of 590 DEGs were consistently expressed in the four datasets, of which 327 were upregulated and 263 were downregulated. Ten DEGs, including five upregulated (ENTPD1, THRSP, KLK10, ADAMTS9, MIR31HG) and five downregulated (SCARA5, EPHB1, CHRDL1, LOC440934, FOXP2) genes, were randomly selected for q-PCR in our own tissue samples to validate the integrated data. Among the upregulated DEGs verified by q-PCR, the extracellular nucleotidase ENTPD1/CD39 is of great significance during the conversion of immunostimulatory adenosine triphosphate (ATP) to immunosuppressive adenosine (19). Overexpression of tumoral ENTPD1 in hepatocellular carcinoma was related to increased tumor recurrence and shortened overall survival and was considered an independent predictor of poor outcome after radical resection (20). LncRNA MIR31HG expression in PTC is also significantly upregulated. MIR31HG is the host gene of miR-31 and serves as the initial intron of MIR31HG (21). This lncRNA is known as an oncogenic lncRNA and is upregulated in various cancers, including breast cancer (22), cervical cancer (23) and laryngeal cancer (24). MIR31HG has also been shown to have prognostic value; it exhibits effects on cell proliferation and invasion in pancreatic ductal adenocarcinoma, and its expression and function are suppressed by miR-193b (25). MIR31HG is significantly upregulated in oral cancer and is significantly related to poor clinical results. It has the function of a HIF-1α coactivator and is also a subunit of HIF-1. HIF-1 relies on the activation of target gene expression in core steps to drive the cell’s response to hypoxia, including mitochondrial function, angiogenesis, metabolism reprogramming, invasion and metastasis (26). To date, no study has investigated the prognostic and functional roles of ENTPD1 and MIR31HG in PTC. Further study should explore the diagnostic and prognostic value of these DEGs in PTC.
GO enrichment analysis of the DEGs in PTC indicated that the most enriched GO terms were endothelial and immune cells or alterations in the structure and composition of the ECM (27-30). Exosomes can also transfer oncogene transcripts into recipient cells (26). For example, exosome-enclosed miRNAs can alter the behavior of recipient cells by regulating gene expression (31-33), which could increase the metastatic potential in poorly differentiated cells. Studies of exosomes in PTC are currently quite limited since the DEGs in PTC were enriched in this area, and further investigation should be promising.
In this study, the KEGG pathway analysis method showed abnormally expressed genes and tyrosine metabolism, CAMs, complement and coagulation cascades, transcriptional misregulation and ECM-receptor interaction pathways. These are the main pathways of cancer. Similar to tyrosine metabolism, tyrosine phosphorylation of proteins serves as an exquisite switch to control several key oncogenic signaling pathways, including the MAPK pathway, which is the major dysregulated pathway in PTC (34). Among the five DEGs in this pathway, ALDH1A3 plays an important role in tyrosine metabolism and is responsible for oxidizing the intermediate aldehyde forms of tyrosine into its corresponding acid (35). Researchers have extensively studied the core functions of CAMs. The adhesion of tumor cells in the vasculature of specific organs is an important process in the metastatic cascade reaction stage (36). The complement system is mainly composed of a cascade of serine proteases encoded by genes from the same source as coagulation proteins (37), which represent the core content of inflammation, and inflammation occurs throughout the process (38). At the same time, complement activation can effectively regulate the adaptive immune response (39) and may also act in the response of tumor T cells (40). Transcriptional misregulation, including misregulation of transcription factors, cofactors, chromatin regulators and noncoding RNAs, has long been known to contribute to tumorigenesis and progression (41). For example, the protein encoded by one of the DEGs, RUNX1, in combination with many promoters and enhancers, is considered to be relevant in the occurrence of breast cancer and leukemia (42,43). The ECM is a complex grid structure of large molecules. It can interact with cell signal transduction receptors and can also act as a reservoir for signal molecules or growth factors (44). In short, all of these pathways have been proven to be classic cancer processes. Our analysis shows that the selected DEGs play an important role in PTC tumorigenesis and cancer progression.
Our study has the following limitations. First, the clinical information and gene mutation information of these patients were not included for validation, and the analysis of cancer and para-cancer differential genes may be erroneous, requiring further verification. Second, the GSE33630 dataset was from the Chernobyl Tissue Bank in Ukraine, and it is not known whether these PTC patients had received radiation, which might induce bias. In addition, we did identify DEGs, but we do not yet know the roles of these genes, and we will further study them.
In conclusion, 590 DEGs were identified in PTC by integrated microarray analysis. The KEGG analysis presented here suggests that the DEGs were enriched in transcriptional misregulation in cancer, transcriptional misregulation in cancer, proteoglycans in cancer, and tyrosine metabolism. Functional studies of PTC should focus on these pathways.
Acknowledgments
Funding: This study was funded by Shanghai Municipal Health Commission (20204Y0266).
Footnote
Reporting Checklist: The authors have completed the MDAR reporting checklist. Available at http://dx.doi.org/10.21037/gs-20-673
Data Sharing Statement: Available at http://dx.doi.org/10.21037/gs-20-673
Conflicts of Interest: All authors have completed the ICMJE uniform disclosure form (http://dx.doi.org/10.21037/gs-20-673). The authors have no conflicts of interest to declare.
Ethical Statement: The authors are accountable for all aspects of the work in ensuring that questions related to the accuracy or integrity of any part of the work are appropriately investigated and resolved. The study was conducted in accordance with the Declaration of Helsinki (as revised in 2013). The study was approved by ethics board of Fudan University Shanghai Cancer Center (No.: 050432-4-1911D) and individual consent for this retrospective analysis was waived.
Open Access Statement: This is an Open Access article distributed in accordance with the Creative Commons Attribution-NonCommercial-NoDerivs 4.0 International License (CC BY-NC-ND 4.0), which permits the non-commercial replication and distribution of the article with the strict proviso that no changes or edits are made and the original work is properly cited (including links to both the formal publication through the relevant DOI and the license). See: https://creativecommons.org/licenses/by-nc-nd/4.0/.
References
- Increasing Diagnosis of Subclinical Thyroid Cancers Leads to Spurious Improvements in Survival Rates. Cancer 2015;121:1793-9. [Crossref] [PubMed]
- Biologic and clinical perspectives on thyroid cancer. N Engl J Med 2016;375:1054-67. [Crossref] [PubMed]
- Differential clinicopathological risk and prognosis of major papillary thyroid cancer variants. J Clin Endocrinol Metab 2016;101:264-74. [Crossref] [PubMed]
- High prevalence of BRAF mutations in thyroid cancer: Genetic evidence for constitutive activation of the RET/PTC-RAS-BRAF signaling pathway in papillary thyroid carcinoma. Cancer Res 2003;63:1454-7. [PubMed]
- TERT promoter mutations in thyroid cancer. Endocr Relat Cancer 2016;23:R143-R155. [Crossref] [PubMed]
- Molecular rearrangements in papillary thyroid carcinoma. Clin Chim Acta 2010;411:301-8. [Crossref] [PubMed]
- BRAF mutation predicts a poorer clinical prognosis for papillary thyroid cancer. J Clin Endocrinol Metab 2005;90:6373-9. [Crossref] [PubMed]
- BRAF(V600E) mutation and outcome of patients with papillary thyroid carcinoma: a 15year median follow-up study. J Clin Endocrinol Metab 2008;93:3943-9. [Crossref] [PubMed]
- The BRAF(V600E) mutation is an independent, poor prognostic factor for the outcome of patients with low-risk intrathyroid papillary thyroid carcinoma: single-institution results from a large cohort study. J Clin Endocrinol Metab 2012;97:4390-8. [Crossref] [PubMed]
- Type and prevalence of BRAF mutations are closely associated with papillary thyroid carcinoma histotype and patients’ age but not with tumour aggressiveness. Virchows Arch 2005;446:589-95. [Crossref] [PubMed]
- BRAF mutation in papillary thyroid carcinoma in a Japanese population: its lack of correlation with high-risk clinicopathological features and diseasefree survival of patients. Endocr J 2009;56:89-97. [Crossref] [PubMed]
- BRAFV600E mutation does not mean distant metastasis in thyroid papillary carcinomas. J Clin Endocrinol Metab 2012;97:E1745-E1749. [Crossref] [PubMed]
- BRAF V600E and TERT promoter mutations cooperatively identify the most aggressive papillary thyroid cancer with highest recurrence. J Clin Oncol 2014;32:2718-26. [Crossref] [PubMed]
- MicroRNA expression and association with clinicopathologic features in papillary thyroid cancer: a systematic review. Thyroid 2015;25:1322-9. [Crossref] [PubMed]
- MicroRNA-29 can regulate expression of the long non-coding RNA gene MEG3 in hepatocellular cancer. Oncogene 2011;30:4750-6. [Crossref] [PubMed]
- Genetic predisposition to papillary thyroid carcinoma: involvement of FOXE1, TSHR, and a novel lincRNA gene, PTCSC2. J Clin Endocrinol Metab 2015;100:E164-E172. [Crossref] [PubMed]
- Long non-coding RNA ANRIL promotes the invasion and metastasis of thyroid cancer cells through TGF-β/Smad signaling pathway. Oncotarget 2016;7:57903-18. [Crossref] [PubMed]
- MALAT1 promotes the proliferation and invasion of thyroid cancer cells via regulating the expression of IQGAP1. Biomed Pharmacother 2016;83:1-7. [Crossref] [PubMed]
- High expression of CD39/ENTPD1 in malignant epithelial cells of human rectal adenocarcinoma. Tumour Biol 2015;36:9411-9. [Crossref] [PubMed]
- Overexpression of CD39 in hepatocellular carcinoma is an independent indicator of poor outcome after radical resection. Medicine (Baltimore) 2016;95:e4989. [Crossref] [PubMed]
- miR-31 and its host gene lncRNA LOC554202 are regulated by promoter hypermethylation in triple negative breast cancer. Mol Cancer 2012;11:5. [Crossref] [PubMed]
- Different gene expression patterns in invasive lobular and ductal carcinomas of the breast. Mol Biol Cell 2004;15:2523-36. [Crossref] [PubMed]
- Elevated Expression Levels of Long Non-Coding RNA, Loc554202, Are Predictive of Poor Prognosis in Cervical Cancer. Tohoku J Exp Med 2017;243:165-72. [Crossref] [PubMed]
- Long non-coding RNA LOC554202 promotes laryngeal squamous cell carcinoma progression through regulating miR-31. J Cell Biochem 2018;119:6953-60. [Crossref] [PubMed]
- Long noncoding RNA MIR31HG exhibits oncogenic property in pancreatic ductal adenocarcinoma and is negatively regulated by miR-193b. Oncogene 2016;35:3647-57. [Crossref] [PubMed]
- Long noncoding RNA LncHIFCAR/MIR31HG is a HIF-1α co-activator driving oral cancer progression. Nat Commun 2017;8:15874. [Crossref] [PubMed]
- Glioblastoma microvesicles transport RNA and proteins that promote tumour growth and provide diagnostic biomarkers. Nat Cell Biol 2008;10:1470-6. [Crossref] [PubMed]
- Heparanase regulates secretion, composition, and function of tumor cell-derived exosomes. J Biol Chem 2013;288:10093-9. [Crossref] [PubMed]
- Tumor-derived microvesicles induce, expand and up-regulate biological activities of human regulatory T cells (Treg). PLoS One 2010;5:e11469. [Crossref] [PubMed]
- Secretion of active membrane type 1 matrix metalloproteinase (MMP-14) into extracellular space in microvesicular exosomes. J Cell Biochem 2008;105:1211-8. [Crossref] [PubMed]
- Stromal fibroblast-derived miR-409 promotes epithelial-to-mesenchymal transition and prostate tumorigenesis. Oncogene 2015;34:2690-9. [Crossref] [PubMed]
- Exosomes from drug-resistant breast cancer cells transmit chemoresistance by a horizontal transfer of microRNAs. PLoS One 2014;9:e95240. [Crossref] [PubMed]
- Exosomes derived from hypoxic leukemia cells enhance tube formation in endothelial cells. J Biol Chem 2013;288:34343-51. [Crossref] [PubMed]
- Protein tyrosine phosphatases in cancer: friends and foes!. Prog Mol Biol Transl Sci 2012;106:253-306. [Crossref] [PubMed]
- ALDH1A3, a metabolic target for cancer diagnosis and therapy. Int J Cancer 2016;139:965-75. [Crossref] [PubMed]
- Cancer cell adhesion and metastasis: selectins, integrins, and the inhibitory potential of heparins. Int J Cell Biol 2012;2012:676731. [Crossref] [PubMed]
- Evolution of enzyme cascades from embryonic development to blood coagulation. Trends Biochem Sci 2002;27:67-74. [Crossref] [PubMed]
- Inflammation and cancer. Nature 2002;420:860-7. [Crossref] [PubMed]
- Regulation of humoral immunity by complement. Immunity 2012;37:199-207. [Crossref] [PubMed]
- The role of the complement system in cancer. J Clin Invest 2017;127:780-9. [Crossref] [PubMed]
- Transcriptional regulation and its misregulation in disease. Cell 2013;152:1237-51. [Crossref] [PubMed]
- RUNX1 and RUNX1-ETO: roles in hematopoiesis and leukemogenesis. Front Biosci (Landmark Ed) 2012;17:1120-39. [Crossref] [PubMed]
- Runx1 is associated with breast cancer progression in MMTV-PyMT transgenic mice and its depletion in vitro inhibits migration and invasion. J Cell Physiol 2015;230:2522-32. [Crossref] [PubMed]
- Targeting ECM Disrupts Cancer Progression. Front Oncol 2015;5:224. [Crossref] [PubMed]