Chemotherapeutic efficacy of cucurmosin for pancreatic cancer as an alternative of gemcitabine: a comparative metabolomic study
Introduction
Pancreatic cancer is a malignant tumor with insidious onset, extremely low early diagnosis rate, extremely aggressive, and insensitive to traditional radiotherapy and chemotherapy and targeted therapy (1). It is the third leading cause of cancer-related death in the United States and the fourth in Japan (2). The incidence of pancreatic cancer has been also increasing worldwide. There has been very limited progress in the treatment of pancreatic cancer over the last few decades, with its 5-year survival rate increasing from 2.5% in 1975–1977 to 8% in 2010–2018 (3). In the absence of promising results for single-agent or combination chemotherapy in the last decades, prognosis remains poor. With multi-drug systemic treatment, the median survival rate is about 8 months (2,4). It is estimated that by 2020, there will be about 420,000 new cases and 410,000 deaths of pancreatic cancer in the world every year (5). It is therefore projected to become the second leading cause of cancer mortality before 2030 due to improving therapies for other cancers compared with those for pancreatic cancer (6). At present, only 10–20% of clinical cases of pancreatic tumors are resectable, but up to 80% of patients undergoing resection develop disease recurrence even after adjuvant treatment. In patients with borderline resectable/unresectable pancreatic cancer, administration of chemotherapy may increase the chance of resection and, consequently, improve survival outcomes (7,8). Since gemcitabine was approved for pancreatic adenocarcinoma treatment by Food and Drug Administration (FDA) of the USA in 1997, it is still the main drug served to all stages of chemotherapy for pancreatic cancer (9,10). Unfortunately, the chemotherapy effect of gemcitabine is not so ideal and only 12% patients are sensitive to gemcitabine (11). Its short plasma half-life and poor targeting may restrict its focal and effective action. Furthermore, pancreatic cancer has been becoming less sensitive to gemcitabine, and thus chemotherapy resistance would limit its efficacy better display. In order to improve the clinical efficacy of chemotherapy, researchers have carried out numbers of studies on the combination of gemcitabine-based chemotherapeutic drugs in the treatment of pancreatic cancer. These studies have found that paclitaxel, platinum, capecitabine and tegafur and other cytotoxic drugs demonstrated good therapeutic effects in combination with gemcitabine (12,13). However, some other researchers have pointed out that these schemes cannot improve the effect of chemotherapy, but will enhance the toxic damage to the patient’s body (14,15). Therefore, the search for other drugs with high efficiency and low side effects for the treatment of pancreatic cancer has become of high importance for improving the prognosis and enhancing the quality of life of the patients with pancreatic cancer.
Ribosomal inactivated protein (RIP) is a class of toxic proteins widely found in plants, and about 110 species of plants containing RIP have been found so far (16,17). In the past, it was believed that the mechanism of RIP antitumor was cell necrosis caused by the cytotoxicity of the drug itself (18). However, in recent years, many kinds of RIPs have been reported to be able to induce apoptosis of tumor cells, and thus it was believed that inducing apoptosis is an important mechanism of anti-tumor function of RIP (19-21). Cucurmosin (CUS) is a new RIP extracted from cucurbit, and previous studies found that CUS shows significant cytotoxicity to tumors such as chronic myeloid leukemia K562, melanoma B16, pancreatic cancer cell strains (BxPC-3 and SW-1990) (22,23). Moreover, CUS demonstrated obvious inhibition to tumor proliferation in mice models (22), however, the specific mechanism, especially its metabolic changes responsible for the therapeutic effect still keep unknown. In this study, we conducted the therapeutic effect experiments with different doses of CUS on mice with pancreatic cancer to compare with gemcitabine from the perspective of metabolomics. We aimed to provide an effective reference for the treatment of pancreatic cancer with an alternative medicine of gemcitabine and clarify their biochemical mechanism and potential side effects, and hopefully serve to improve the survival rate of pancreatic cancer patients in the future.
We present the following article in accordance with the ARRIVE reporting checklist (available at http://dx.doi.org/10.21037/gs-20-202).
Methods
Experimental materials
Experiments were performed under a project license [NO.: SYXK(Fujian) 2016-0006] granted by the Ethics Committee of the Fujian Medical University Union Hospital, in compliance with the principles of the National Institutes of Health guide for the care and use of Laboratory animals. Adult male nude mice (4–6 weeks old, weighing 18–20 g) were obtained from Shanghai Slack Laboratory Animals Co. Ltd. [license number SCXK (Shanghai) 2007-0005] and fed on standard SPF-grade laboratory conditions including a relative humidity of 60%±10% and a temperature of 24±2 °C. Human pancreatic cancer cell line Panc-1 (Catalog NO. TCHu) was purchased from Shanghai Biocell Bank, and further conserved and passaged. CUS was isolated and purified from the sarcocarp of Cucurbita moschata (pumpkin), and the protein was quantified by BCA Protein Assay Kit.
Establishment of subcutaneous xenograft model of pancreatic cancer
Pancreatic cancer cell line was authenticated with short tandem repeat test and mycoplasma culture. The Panc-1 cells were cultured in a humidified incubator (Thermo Scientific, USA) at 37 °C and 5% CO2 with RPMI 1640 medium (Gibco, USA) supplemented with 10% fetal bovine serum and 80 U/mL gentamicin. The cells were digested by 0.125% trypsinogen (Life Technologies, USA) for the passage once every 2–3 days, keeping the cells in the logarithmic growth phase. When the Panc-1 cells in logarithmic growth phase were 2×107 cells/flask, they were digested with 0.25% trypsin and harvested into a centrifuge tube. The cells in trypsin solution were centrifuged at 1,500 g for 10 min. The supernatant was discarded, and the cells (2×107 cells) were washed for 3 times with phosphate buffered saline (PBS) and resuspended in 0.2 mL culture medium.
After skin degerming, the cell suspension (2×107 cells in 0.2 mL culture medium) was subcutaneously injected into the right shoulder back of the nude mouse with a sterile syringe, followed by normal feeding. When a tumor with a size of 1 cm in diameter was formed in the injection position of the mouse, the mouse was executed by a mercy killing, and the tumor was excised under aseptic conditions and cut into pieces with a size of about 1 mm3. Consequently, the tumor granules were pushed subcutaneously into the right shoulder back of a nude mouse by a 16-gauge trocar.
Drug intervention on the subcutaneous xenograft model of pancreatic cancer
On the 15th, 18th, 21st, 24th, 27th and 30th day after the surgeries, the mice subcutaneously implanted by pancreatic tumors were intraperitoneally administrated by the different drugs, and the mice were randomly divided into 5 groups according to their intervention strategy. Control (C) group (n=12): the healthy nude mice intraperitoneally injected with sterile saline (10 mL/kg); pancreatic cancer (PANC) group (n=9): the xenograft mice intraperitoneally injected with sterile saline (10 mL/kg); high-dose CUS (CUS-H) group (n=10): the xenograft mice intraperitoneally injected with high-dose CUS (1.0 mg/kg); low-dose CUS (CUS-L) group (n=10): the xenograft mice intraperitoneally injected with low-dose CUS (0.5 mg/kg); gemcitabine (G) group (n=9): the xenograft mice intraperitoneally injection with gemcitabine (50 mg/kg). The high dose (1.0 mg/kg) of CUS was based on its safety concentration and a lower dose (0.5 mg/kg) was used as the comparison.
Sample collection and preparation
On the 33th day after the surgeries, 1 mL of orbital blood was collected by exsanguination under isoflurane anesthesia and stored in 1.5 mL Eppendorf tubes. After standing for 60 min on ice, serum was obtained through a 10-min centrifugation at 10,000 g and 4 °C. The supernate was collected and immediately snap frozen by liquid nitrogen and stored at −80 °C. After sacrifice of the mice by cervical dislocation, the tissues were collected for the histological observation.
A serum sample of 400 µL was taken out and mixed with 200 µL of a deuterated phosphate buffer solution (90 mM, pH 7.4) containing 0.9% NaCl (normal saline), and mixed by an oscillator. After centrifugation at 10,000 g for 10 min at 4 °C, 550 µL of the supernatant was transferred into a 5-mm nuclear magnetic resonance (NMR) tube (ST500, NORELL, Inc., Morganton, North Carolina, USA) for NMR sampling. The serum samples include 12 cases of healthy control group (C), 9 cases of PANC group, 10 cases of CUS-H group, 10 cases of CUS-L group and 9 cases of gemcitabine group.
NMR sampling and processing
1H NMR spectra were acquired on a 600 MHz Bruker Avance III spectrometer (Bruker Corporation, Kalsruhe, Germany) equipped with a 5 mm CPBBO probe operating at 600.13 MHz and 295 K. The 1H NMR spectra detection was carried out using a standard water-suppressed CPMG pulse sequence. For each serum sample, 16 free induction decays (FIDs) were collected into 32 K data points over a spectral width of 12,000 Hz with a relaxation delay of 4.0 s and an acquisition time of 2.73 s. Spin-echo loop time (2nτ) was set as 70 ms. All the obtained 1H NMR spectra were processed with MestReNova (version: 9.0, Mestrelan Research, Santiago de Compostella, Spain) and assigned with ChenomX NMR Suit 8.0 (ChenomX Inc., Canada). To boost the signal-to-noise ratio, all FIDs were multiplied by an exponential weighting function equivalent to a 1 Hz line-broadening. In order to make the spectra more unified, the manual phase rectifications and baseline corrections were conducted by using MestReNova. The chemical shifts were referenced to the double-peak of endogenic lactate at δ 1.33 for metabolites identification (24). Next, the spectral regions δ 9.0–0.5 of the processed NMR spectra were segmented into scatter integral regions of δ 0.002 with a removal of spectral region δ 6.00–5.65 and δ 5.20–4.67 to eliminate the impacts of residual water signal and urea signal commonly. All of the data were normalized to the total sum of the spectrum to make the data directly comparable with each other. Then, the integrated data were imported into SIMCA 14.1 for pattern recognition and multivariate statistical analysis.
Statistical analysis and pattern recognition
Multivariate statistical analysis was performed on SIMCA 14.1 software (Umetrics, Umea, Sweden). The pattern recognition methods commonly used in metabonomics include principal component analysis (PCA), partial least square analysis (PLS-DA) and orthogonal partial least square analysis (OPLS-DA). PCA can intuitively present the relationship between samples (distribution results), such as whether there is clustering or outlier. PLS-DA and OPLS-DA, which can be classified as supervised multivariate statistical analysis, was conducted in Parato-scaling approach for better extraction and maximization of the metabonomic differences between different groups. Then, the X-matrix interpretation rate R2X and the Y-matrix interpretation rate R2Y and the prediction rate Q2 were obtained by 9 cross-validation methods to evaluate the model quality. In order to avoid over-fitting of the established model, the responsive permutation experiment was used to randomly change the categorical variable Y 200 times, thus obtaining different random R2 and Q2 values. At the same time, the correlation coefficient (r) and variable importance for the projection (VIP) value of the model were obtained from OPLS-DA, which is used to obtain meaningful differential metabolites.
The relative concentrations of the assigned metabolites were calculated by integrating their characteristic signals in the NMR spectra. The results of the univariate statistical analysis were evaluated using a fold-change value and a Student’s t-test, in which the t-test test value was converted to a P value to obtain a significant change in metabolites.
In this study, a four-dimensional volcano plot was used to identify the discriminative metabolites between pair-wise groups, which is a scatter plot of absolute correlation coefficient value and the VIP value from the multivariate analysis were brought as two additional variables represented by color and dot size, respectively.
Metabolic pathways and interactions analysis
In order to understand the metabolic processes and details involved in the occurrence and treatment of pancreatic cancer, pathways enrichment analysis and pathway topology analysis were performed on the differential metabolites by using pathway database including KEGG (https://www.kegg.jp/kegg/pathway.html), HMDB (http://www.hmdb.ca/), and MetaboAnalyst (https://www.metaboanalyst.ca/MetaboAnalyst/faces/home.xhtml) (25-27).
Histopathology of the tissues
The selected tissue samples from the different groups were fixed in 10% formalin solution. Paraffin embedding and standard procedures followed, and 4-µm sections were cut and stained with hematoxylin and eosin. All slices were randomly double-blind confirmed histopathologically by experienced pathologists.
Results
Establishment of mouse model with pancreatic cancer and drug treatment
The subcutaneous xenograft mouse model with pancreatic cancer was successfully established in 38 nude mice. An infection case occurred in the PANC group, and no obvious tumor was formed in one mouse in G group, which were excluded from the study. A typical mouse with pancreatic tumor was shown in the supplemental materials (Figure S1A), and the tumor usually possessed a size of 1.5 cm in diameter (Figure S1B). When the mouse was euthanized, 4-µm sections of the tumor were cut and stained with hematoxylin and eosin. The successful establishment of pancreatic tumor was confirmed by histopathological analysis by the experienced pathologists (Figure S1C,D). Their growth curves of body weight and the tumor in each group were displayed in Figure S2. After the mice with pancreatic cancer were treated with the different intervention regimens for 15 days, different inhibition effects of the tumors were demonstrated in the mice. Compared with PANC group, the inhibition rates of CUS-H, CUS-L and G to the pancreatic tumors were 43.5%, 33.7%, and 76.1%, respectively (Table S1).
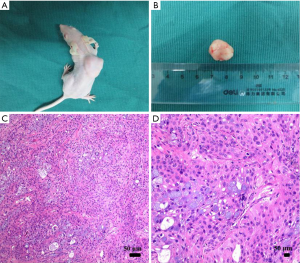
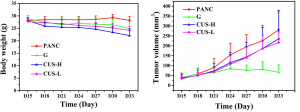
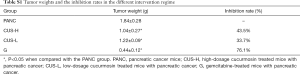
Full table
1H NMR spectral characteristics of mice serum following occurrence and management of pancreatic cancer
The average 1H NMR spectra of serum from the different groups were shown in Figure 1. 1H NMR spectra provided the metabolic variations in the serum following the occurrence of pancreatic cancer and the corresponding metabolic responses to the treatment with gemcitabine and CUS. The resonance assignments were performed based on the HMDB database, ChenomX and published literatures (28-30), and the NMR spectral details of the identified metabolites were tabulated in Table 1. The relative concentration of each metabolite could be obtained from the integral area of the corresponding characteristic signals in the NMR spectra (Table 1). Thus, the comparative analysis between the pair-wise groups could be carried out by the univariate statistical approach, but the results may be misleading or weakened due to the possible overlapping of the signals and synergistic actions and interaction of the different metabolites. The additional multivariate statistical analysis will strengthen the objective evaluation of the metabolic similarity and differences between the different pair-wise groups.
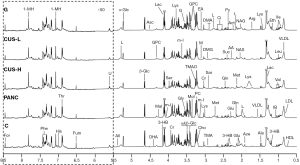
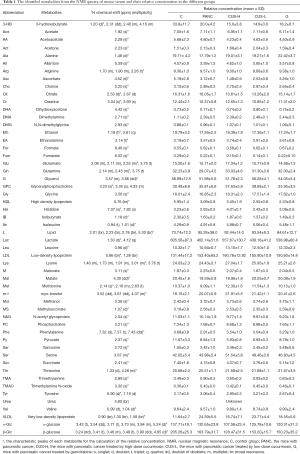
Full table
Statistical analysis of metabolomic characteristics of serum following occurrence and management of pancreatic cancer
PCA is a pattern recognition method to simplify the complex relationship between variables, which could give an overview of all serum samples and examine the intrinsic variation within the groups. As revealed in PCA the scores plot (Figure 2A), the first two principal components (PC1 and PC2) could explain 76.5% of the total variance. Although a certain of overlap existed between the five groups, C, CUS-H and G were closed to each other but away from the PANC group, indicating that the serum metabolomes of the three groups were similar with each other. CUS-L groups also demonstrate a metabolic difference from PANC groups although they quite mix with each other. PLS-DA further maximized the systematic variance and to eliminate the interference information and thus highlighting the separation between the groups in the PLS-DA scores plot (Figure 2B) though a moderate overlap could still be observed between them. These results showed that CUS could regulate the metabolic profile of the mice with pancreatic cancer, and its high dose takes a similar therapeutic effect with gemcitabine.
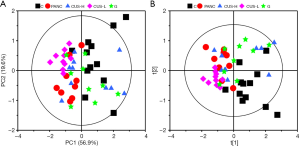
In order to identify the metabolites responsible for the occurrence and management of pancreatic cancer, OPLS-DA was carried out on the NMR data from the pair-wise groups (i.e., groups C vs. PANC, groups C vs. CUS-H, groups C vs. CUS-L, and groups C vs. G) (Figure 3, left panels). From the results of OPLS-DA permutation test, an obvious metabolic disorder was induced by pancreatic cancer, and the concentrations of the disturbed metabolites have not recovered to the control levels even after the treatment of CUS or gemcitabine (Figure 3, middle panels). Such conclusion could also be confirmed by the pair-comparisons of the PANC group and the different treatment groups (Figure S3 and Table S2). The corresponding correlation coefficient (r) and VIP values could be obtained from these models. The difference multiple of each metabolite content (fold-change) between the pair-wise groups and the transformed P value after t-test could be calculated and analyzed. A four-dimensional volcanic map could be constructed (Figure 3, right panels) based on the correlation coefficients and VIP values from multivariate analysis and the fold change and P values from univariate analysis. Accordingly, the characteristic metabolites with significant differences between the pair-wise groups can be screened out to characterize the pancreatic cancer and its managements. As a result, compared with the C group, 18 of the metabolites in serum showed significant changes in pancreatic cancer, including the higher levels of acetone, dimethylamine, methanol, sarcosine, trimethylamine and very low-density lipoprotein (VLDL) and the lower levels of 3-hydroxybutyrate, allantoin, ascorbate, creatine, high-density lipoprotein (HDL), isobutyrate, lactate, methionine, myo-inositol, pyruvate, succinate, and β-glucose in the PANC group than the C group (Figure 3A and Table S3), which were basically consistent with previous studies (31,32). We also compared the changes of relative concentration of differential metabolites between the different groups. It was found that the contents of 5 differential metabolites, including glutamate, glycine, lipid, methionine, and trimethylamine N-oxide, demonstrated a recovered trend in the CUS-H group compared with the PANC group, 4 kinds of recoverable differential metabolites, including allantoin, low-density lipoprotein (LDL), succinate, and VLDL, were found in the G group, while 7 differential metabolites demonstrated a recoverable metabolic trend in the CUS-L group, including acetone, allantoin, creatine, ethanol, glycine, lipid, methionine (Table 1). The results indicated that both CUS and gemcitabine could regulate the serum metabolic profile of pancreatic cancer mice, and CUS demonstrated a dose-dependent chemotherapeutic efficacy to pancreatic cancer.
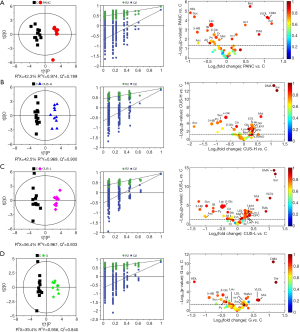
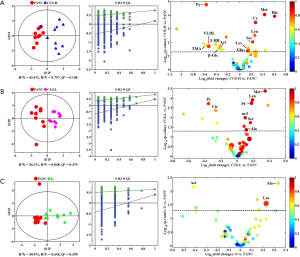
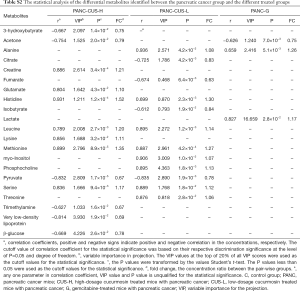
Full table
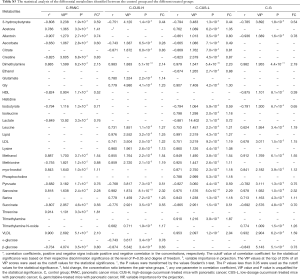
Full table
The affected metabolic pathways following occurrence and management of pancreatic cancer
In order to understand the altered metabolic pathways induced by pancreatic cancer and the different treatments, pathways enrichment analysis was performed on the differential metabolites from the pair-wise groups including groups C vs. PANC, groups C vs. CUS-H, groups C vs. CUS-L, and groups C vs. G. The results were shown in the form of bubble map (Figure 4), where the bubble area was proportional to the influence of the pathway and the bubble color corresponds to match status, i.e., the proportion of the differential metabolites in the pathways (from red for the highest proportion to white for the lowest proportion) (33,34). The results showed that the occurrence of pancreatic mainly induced the disturbance in pyruvate metabolism, glycine, serine and threonine metabolism, TCA cycle, glycolysis or gluconeogenesis, cysteine and methionine metabolism (Figure 4A,E). After the treatment by the different chemotherapy regimens, some disturbed pathways were positively regulated to the normal level, however, some pathways were additionally altered. High-dose CUS and gemcitabine demonstrated a similar chemotherapeutic efficacy, in which they all positively regulated pyruvate metabolism, glycolysis or gluconeogenesis, and cysteine and methionine metabolism, while they simultaneously induced the metabolic alteration in methane metabolism and cysteine and methionine metabolism, and high-dose CUS also induced the additional metabolic variations in aminoacyl-tRNA biosynthesis, TCA cycle, and alanine, aspartate and glutamate metabolism (Figure 4B,C,D,E). Low-dose CUS took few effects on pancreatic cancer, in which it only regulated the pyruvate metabolism but induced a similar additional variation with those of CUS-H including aminoacyl-tRNA biosynthesis, methane metabolism, glycine, serine and threonine metabolism, and valine, leucine and isoleucine biosynthesis. A metabolic pathway network by the pancreatic cancer and the different chemotherapy regimens could be derived from the differential metabolites in the different group to obtain an integral view on the overall metabolic disturbance (Figure 5).
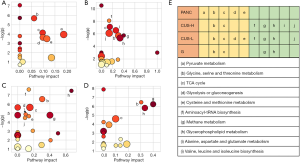
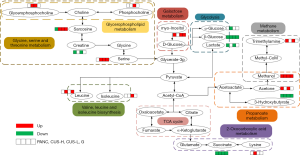
Discussion
In this study, 1H NMR-based serum metabolomic method was used to appraise the chemotherapeutic efficacy of CUS for pancreatic cancer as an alternative of gemcitabine and to study the biological mechanism of CUS in a mouse model with pancreatic cancer.
Metabolic characteristics of pancreatic cancer are associated with metabolic reprogramming of tumor cells
Consistent with previous studies (31,32), in our study the changes of 18 serum metabolites could be identified as the biomarkers of pancreatic cancer. Occurrence of pancreatic cancer was involved in the disturbance in the metabolic pathways especially related to energy metabolism. Studies have shown that pyruvate metabolism is the key to cellular energy metabolism (35). As the final product of glycolysis, pyruvic acid is a key link in the cell for oxidative metabolism, gluconeogenesis, lipid synthesis, cholesterol synthesis and maintenance of the carbon flux of the tricarboxylic acid cycle. Tumor cells still choose pyruvate to be converted into lactate under aerobic conditions instead of entering the oxidative metabolism of mitochondria. The abnormality of mitochondrial pyruvate metabolism and its regulatory enzymes are closely related to tumor occurrence, development and metastasis (36,37). It is well known that the tumor metabolic reprogramming is the key for malignant transformation, and in this process, it is the aerobic glycolysis (Warburg effect) rather than TCA cycle become the special biochemical phenotype (38,39). Through the active aerobic glycolysis, the malignant tumor cells derive the predominant energy and generate extensive lactate to result in microenvironmental acidosis and thus promote the spreading of the tumor cells. Glycolysis enzyme has the effect of antagonizing apoptosis, which can lead to the tolerance of malignant tumors to pro-apoptotic effects such as chemoradiation (40). Cysteine plays an important role in a carbon metabolism pathway, and related studies have shown that one carbon unit is essential for DNA methylation (41), which is associated with the development of many cancers (42). In the development of tumor, amino acid metabolism is also closely related to tumor production through TCA cycle (43,44).
CUS dose-dependently regulates pancreatic tumors by inducing apoptosis of tumor cells
CUS demonstrated a dose-dependent inhibition to the pancreatic tumors. Considering the unknown in vivo adverse effects, the different dosages of CUS (1.0 and 0.5 mg/kg) and gemcitabine (50 mg/kg) was used to treat the mice. Even then, CUS offers a favorable inhibiting ability to the subcutaneous xenograft tumors of pancreatic cancer. High dosage of CUS results in an inhibition rate over half that of gemcitabine, indicating the promising therapeutic drug of CUS development targeted at pancreatic cancer.
In the disturbed biochemical pathways by pancreatic cancer, pyruvate metabolism was positively regulated by all of the treatment regimens, and glycolysis or gluconeogenesis and cysteine and methionine metabolism were further positively regulated by the intervention of high-dose CUS or gemcitabine. We conclude that CUS can functionally regulate pyruvate metabolism, glycolysis or gluconeogenesis and cysteine and methionine metabolism pathways to treat pancreatic cancer. It should be believed that suppressing the active metabolisms in pancreatic tumor is closely related to inhabiting the proliferation of pancreatic cancer cells. RIPs including CUS have been reported to be able to induce apoptosis of tumor cells by bonding to the specific adenosine residue of RNA, and thus leading to removal of the adenine and blockage of protein synthesis (19-21). The affected RNA biosynthesis both in the CUS-H and CUS-L groups also supported this viewpoint (Figure 4E).
Growth factor receptor (GFR) plays an important role in the development of pancreatic cancer. It is a glycoprotein that is expressed in normal cells but overexpressed in tumor cells. Overexpression is mainly related to tumor cell proliferation, invasion, metastasis, infiltration and poor prognosis (19-21), and some studies have indicated that CUS downregulated the expression of GFR at the protein level (19-21), thus it was believed that inactivating GFR signaling pathway and further inducing apoptosis is an important mechanism of anti-tumor function of CUS.
Nevertheless, several additional metabolic pathways appeared in the drug-administered groups compared with the PANC group. Especially, methane metabolism and glycerophospholipid metabolism were shared by CUS-L, CUS-H and G groups. Due to the disturbance of TCA cycle, glutaminolysis is enhanced in pancreatic cancer cells to generate TCA intermediates through anaplerosis reaction, and subsequently served as building blocks for synthesis and metabolism of amino acids and lipids (45), thus causing the variations of glycerophospholipid metabolism in all of the three treated groups and the metabolism and synthesis of amino acids in the CUS-treated groups.
Furthermore, in our study, the metabolites that methane metabolism matches are mainly methanol and trimethylamine. These metabolic abnormalities are not too much associated with the tumor development and inhibition, and they may be related to the microbial activities induced by the intervention of the drugs.
Conclusions
In this study, 1H NMR-based serum metabolomic analysis confirmed that CUS has a significant improvement effect on pancreatic cancer, and the mechanism of action involves in pyruvate metabolism, glycolysis or gluconeogenesis, and cysteine and methionine metabolism by inducing apoptosis of tumor cells. In our opinion, CUS could be an alternative chemotherapeutic drug of gemcitabine for pancreatic cancer if not a better choice. However, it should be mentioned that the sample size is relatively small in this study, and the dose selection and surface modification should be optimized according to the stage of pancreatic cancer. Subsequent studies should be performed in an expanded range both in laboratory and clinical regimes and also combined with other omics techniques.
Acknowledgments
Funding: This work is financially supported by the National Natural Science Foundation of China (No. 31671920 and 81272581) and the United Fujian Provincial Health and Education Project for Tackling the Key Research (No. 2019-WJ-07).
Footnote
Reporting Checklist: The authors have completed the ARRIVE reporting checklist. Available at http://dx.doi.org/10.21037/gs-20-202
Data Sharing Statement: Available at http://dx.doi.org/10.21037/gs-20-202
Peer Review File: Available at http://dx.doi.org/10.21037/gs-20-202
Conflicts of Interest: All authors have completed the ICMJE uniform disclosure form (available at http://dx.doi.org/10.21037/gs-20-202). Drs. JF and HH report grants from the National Natural Science Foundation of China and the United Fujian Provincial Health and Education Project for Tackling the Key Research during the conduct of the study. The other authors have no conflicts of interest to declare.
Ethical Statement: The authors are accountable for all aspects of the work in ensuring that questions related to the accuracy or integrity of any part of the work are appropriately investigated and resolved. Experiments were performed under a project license [NO.: SYXK(Fujian) 2016-0006] granted by the Ethics Committee of the Fujian Medical University Union Hospital, in compliance with the principles of the National Institutes of Health guide for the care and use of Laboratory animals.
Open Access Statement: This is an Open Access article distributed in accordance with the Creative Commons Attribution-NonCommercial-NoDerivs 4.0 International License (CC BY-NC-ND 4.0), which permits the non-commercial replication and distribution of the article with the strict proviso that no changes or edits are made and the original work is properly cited (including links to both the formal publication through the relevant DOI and the license). See: https://creativecommons.org/licenses/by-nc-nd/4.0/.
References
- Tanaka H, Kamata K, Takenaka M, et al. Contrast-enhanced harmonic endoscopic ultrasonography for evaluating the response to chemotherapy in pancreatic cancer. Dig Liver Dis 2019;51:1130-4. [Crossref] [PubMed]
- Abi Jaoude J, Kouzy R, Nguyen ND, et al. Radiation therapy for patients with locally advanced pancreatic cancer: Evolving techniques and treatment strategies. Curr Probl Cancer. 2020. Epub ahead of print. [Crossref] [PubMed]
- Siegel RL, Miller KD, Jemal A. Cancer statistics, 2018. CA Cancer J Clin 2018;68:7-30. [Crossref] [PubMed]
- Usón Junior PL, Franca MS, Rodrigues HV, et al. Higher overall survival in metastatic pancreatic cancer: the impact of where and how treatment is delivered. Einstein (Sao Paulo) 2015;13:347-51. [Crossref] [PubMed]
- Ferlay J, Soerjomataram I, Dikshit R, et al. Cancer incidence and mortality worldwide: Sources, methods and major patterns in GLOBOCAN 2012. Int J Cancer 2015;136:E359-86. [Crossref] [PubMed]
- Rahib L, Smith BD, Aizenberg R, et al. Projecting Cancer Incidence and Deaths to 2030: The Unexpected Burden of Thyroid, Liver, and Pancreas Cancers in the United States. Cancer Res 2014;74:2913-21. [Crossref] [PubMed]
- Saung MT, Zheng L. Current Standards of Chemotherapy for Pancreatic Cancer. Clin Ther 2017;39:2125-34. [Crossref] [PubMed]
- Gillen S, Schuster T, Meyer Zum Büschenfelde C, et al. Preoperative/neoadjuvant therapy in pancreatic cancer: a systematic review and meta-analysis of response and resection percentages. PLoS Med 2010;7:e1000267. [Crossref] [PubMed]
- Aprile G, Negri FV, Giuliani F, et al. Second-line chemotherapy for advanced pancreatic cancer: Which is the best option? Crit Rev Oncol Hematol 2017;115:1-12. [Crossref] [PubMed]
- Jin SF, Fan ZK, Pan L, et al. Gemcitabine-based combination therapy compared with gemcitabine alone for advanced pancreatic cancer: a meta-analysis of nine randomized controlled trials. Hepatobiliary Pancreat Dis Int 2017;16:236-44. [Crossref] [PubMed]
- Garrido-Laguna I, Hidalgo M. Pancreatic cancer: from state-of-the-art treatments to promising novel therapies. Nat Rev Clin Oncol 2015;12:319-34. [Crossref] [PubMed]
- Petrelli F, Coinu A, Borgonovo K, et al. Polychemotherapy or gemcitabine in advanced pancreatic cancer: A meta-analysis. Dig Liver Dis 2014;46:452-9. [Crossref] [PubMed]
- Vogel A, Ciardiello F, Hubner RA, et al. Post-gemcitabine therapy for patients with advanced pancreatic cancer - A comparative review of randomized trials evaluating oxaliplatin-and/or irinotecan-containing regimens. Cancer Treat Rev 2016;50:142-7. [Crossref] [PubMed]
- Petrioli R, Roviello G, Fiaschi AI, et al. Gemcitabine, oxaliplatin, and capecitabine (GEMOXEL) compared with gemcitabine alone in metastatic pancreatic cancer: a randomized phase II study. Cancer Chemother Pharmacol 2015;75:683-90. [Crossref] [PubMed]
- Colucci G, Labianca R, Di Costanzo F, et al. Randomized Phase III Trial of Gemcitabine Plus Cisplatin Compared With Single-Agent Gemcitabine As First-Line Treatment of Patients With Advanced Pancreatic Cancer: The GIP-1 Study. J Clin Oncol 2010;28:1645-51. [Crossref] [PubMed]
- Akkouh O, Ng TB, Cheung RCF, et al. Biological activities of ribosome-inactivating proteins and their possible applications as antimicrobial, anticancer, and anti-pest agents and in neuroscience research. Appl Microbiol Biotechnol 2015;99:9847-63. [Crossref] [PubMed]
- Wang YM, Lu TL, Hsu PN, et al. Ribosome inactivating protein B-chain induces osteoclast differentiation from monocyte/macrophage lineage precursor cells. Bone 2011;48:1336-45. [Crossref] [PubMed]
- Muñoz R, Arias Y, Ferreras JM, et al. Targeting a marker of the tumour neovasculature using a novel anti-human CD105-immunotoxin containing the non-toxic type 2 ribosome-inactivating protein nigrin b. Cancer Lett 2007;256:73-80. [Crossref] [PubMed]
- Barbieri L, Battelli MG, Stirpe F. Ribosome-Inactivating Proteins from Plants. Biochim Biophys Acta 1993;1154:237-82. [Crossref] [PubMed]
- Girbés T, Ferreras JM, Arias FJ, et al. Description, distribution, activity and phylogenetic relationship of ribosome-inactivating proteins in plants, fungi and bacteria. Mini Rev Med Chem 2004;4:461-76. [Crossref] [PubMed]
- Parikh BA, Tumer NE. Antiviral activity of ribosome inactivating proteins in medicine. Mini Rev Med Chem 2004;4:523-43. [Crossref] [PubMed]
- Xie J, Wang C, Yang A, et al. Cucurmosin kills human pancreatic cancer SW-1990 cells in vitro and in vivo. Anticancer Agents Med Chem 2013;13:952-6. [Crossref] [PubMed]
- Zhang B, Huang H, Xie J, et al. Cucurmosin induces apoptosis of BxPC-3 human pancreatic cancer cells via inactivation of the EGFR signaling pathway. Oncol Rep 2012;27:891-7. [PubMed]
- Wen S, Zhan BH, Feng JH, et al. Non-invasively predicting differentiation of pancreatic cancer through comparative serum metabonomic profiling. BMC Cancer 2017;17:708. [Crossref] [PubMed]
- Kanehisa M, Goto S, Sato Y, et al. information, knowledge and principle: back to metabolism in KEGG. Nucleic Acids Res 2014;42:D199-205. [Crossref] [PubMed]
- Wishart DS, Knox C, Guo AC, et al. HMDB: a knowledgebase for the human metabolome. Nucleic Acids Res 2009;37:D603-10. [Crossref] [PubMed]
- Chong J, Soufan O, Li C, et al. MetaboAnalyst 4.0: towards more transparent and integrative metabolomics analysis. Nucleic Acids Res 2018;46:W486-94. [Crossref] [PubMed]
- Li J, Zhao Z, Feng J, et al. Understanding the metabolic fate and assessing the biosafety of MnO nanoparticles by metabonomic analysis. Nanotechnology 2013;24:455102. [Crossref] [PubMed]
- Dieterle F, Ross A, Schlotterbeck G, et al. Metabolite projection analysis for fast identification of metabolites in metabonomics. Application in an amiodarone study. Anal Chem 2006;78:3551-61. [Crossref] [PubMed]
- Demsar U, Harris P, Brunsdon C, et al. Principal Component Analysis on Spatial Data: An Overview. Ann Assoc Am Geogr 2013;103:106-28. [Crossref]
- Kobayashi T, Nishiumi S, Ikeda A, et al. A Novel Serum Metabolomics-Based Diagnostic Approach to Pancreatic Cancer. Cancer Epidemiol Biomarkers Prev 2013;22:571-9. [Crossref] [PubMed]
- Michálková L, Hornik S, Sykora J, et al. Diagnosis of pancreatic cancer via(1)H NMR metabolomics of human plasma. Analyst 2018;143:5974-8. [Crossref] [PubMed]
- Wei DD, Wang JS, Wang PR, et al. Toxic effects of chronic low-dose exposure of thioacetamide on rats based on NMR metabolic profiling. J Pharm Biomed Anal 2014;98:334-8. [Crossref] [PubMed]
- Ruan LY, Fan JT, Hong W, et al. Isoniazid-induced hepatotoxicity and neurotoxicity in rats investigated by H-1 NMR based metabolomics approach. Toxicol Lett 2018;295:256-69. [Crossref] [PubMed]
- Danhier P, Bański P, Payen VL, et al. Cancer metabolism in space and time: Beyond the Warburg effect. Biochim Biophys Acta Bioenerg 2017;1858:556-72. [Crossref] [PubMed]
- Du J, Cleghorn WM, Contreras L, et al. Inhibition of Mitochondrial Pyruvate Transport by Zaprinast Causes Massive Accumulation of Aspartate at the Expense of Glutamate in the Retina. J Biol Chem 2013;288:36129-40. [Crossref] [PubMed]
- Bender T, Martinou JC. The mitochondrial pyruvate carrier in health and disease: To carry or not to carry? Biochim Biophys Acta 2016;1863:2436-42. [Crossref] [PubMed]
- Vander Heiden MG, Cantley LC, Thompson CB. Understanding the Warburg Effect: The Metabolic Requirements of Cell Proliferation. Science 2009;324:1029-33. [Crossref] [PubMed]
- Warburg O. On the origin of cancer cells. Science 1956;123:309-14. [Crossref] [PubMed]
- Hsu PP, Sabatini DM. Cancer cell metabolism: Warburg and beyond. Cell 2008;134:703-7. [Crossref] [PubMed]
- Carter RN, Morton NM. Cysteine and hydrogen sulphide in the regulation of metabolism: insights from genetics and pharmacology. J Pathol 2016;238:321-32. [Crossref] [PubMed]
- Akhavan-Niaki H, Samadani AA. DNA Methylation and Cancer Development: Molecular Mechanism. Cell Biochem Biophys 2013;67:501-13. [Crossref] [PubMed]
- He W, Miao FJP, Lin DCH, et al. Citric acid cycle intermediates as ligands for orphan G-protein-coupled receptors. Nature 2004;429:188-93. [Crossref] [PubMed]
- Francklyn C. tRNA synthetase paralogs: Evolutionary links in the transition from tRNA-dependent amino acid biosynthesis to de novo biosynthesis. Proc Natl Acad Sci U S A 2003;100:9650-2. [Crossref] [PubMed]
- Sheppard K, Yuan J, Hohn MJ, et al. From one amino acid to another: tRNA-dependent amino acid biosynthesis. Nucleic Acids Res 2008;36:1813-25. [Crossref] [PubMed]