Identification of key differentially expressed genes between ER-positive/HER2-negative breast cancer and ER-negative/HER2-negative breast cancer using integrated bioinformatics analysis
Introduction
Breast cancer (BC) is a heterogeneous disease with different molecular characteristics. The key molecular characteristics include human epidermal growth factor receptor 2 (HER2, encoded by ERBB2) hormone receptors (HR), including estrogen receptor (ER) and progesterone receptor (PR). The treatment strategies are different for various subtypes of BC with distinct molecular characteristics. Furthermore, in addition to multidisciplinary management, therapeutic approaches for BC focus on individualization of therapy based on the activation of key molecular features (e.g., ER and HER2) (1). Currently, according to histological and molecular characteristics, BC has been classified into four subtypes: luminal A (ER+, PR+, Her2-, Ki67-), luminal B (ER+, PR+, Her2-, Ki67+), HER2-enriched (ER-, PR-, Her2+, Ki67+) and basal type (ER-, PR-, Her2-, Ki67+) (1,2). The features of BC, including proliferative activity, response to chemotherapy, response to targeted therapies, histological grade and prognosis, are different in these subtypes. These features are strongly correlated with ER and HER2 status (1,2). ER-positive/HER2-negative BC accounts for the majority of BC cases. Gene expression profiling analysis has demonstrated that the definition of luminal A and luminal B is mostly based on proliferative activity and the expression levels of ER and ER-related genes (1-3). In addition, ER-negative/HER2-negative BC accounts for approximately 10–17% of all BCs and is remarkably heterogeneous. ER-negative/HER2-negative BC includes multiple subtypes of BC, including triple-negative breast cancer (TNBC). Although ER-negative/HER2-negative BC is not synonymous with TNBC, many studies suggest that most basal-like cancers display an ER-negative/HER2-negative phenotype. Furthermore, most ER-negative/HER2-negative BCs are classified as basal-like subtypes by gene expression profiling analysis (1-3). Since the expression of ER and HER2 is low and there are no definite targets for therapy, there are still clinical challenges for ER-negative/HER2-negative BC (1,3,4). With distinct molecular features (e.g., ER status) among patients, ER-positive/HER2-negative BC and ER-negative/HER2-negative BC display different clinical characteristics (e.g., proliferative activity, histological grade and prognosis) and different responses to endocrine therapy and chemotherapy (1,3,4). Therefore, identification of the key differentially expressed genes (DEGs) between ER-positive/HER2-negative BC and ER-negative/HER2-negative BC and the underlying mechanism are important in the investigation of molecularly targeted therapies.
Currently, gene chips and bioinformatics analysis have been identified as powerful tools for identifying novel key genes and underlying mechanisms of multiple cancers. First, two original microarray datasets, GSE22093 and GSE23988, were chosen from the Gene Expression Omnibus (GEO). Seventy-four ER-positive/HER2-negative BC samples and 85 ER-negative/HER2-negative BC samples were analyzed. Second, the GEO2R online tool and Venn diagram software were used to obtain the common DEGs in the two datasets above. Third, using the Database for Annotation, Visualization and Integrated Discovery (DAVID), these DEGs were analyzed. Fourth, a protein-protein interaction (PPI) network was established, and then Cytoscape MCODE (Molecular Complex Detection) was utilized to analyze those DEGs. Then, 10 core DEGs were identified. In addition, we utilized UALCAN to determine the expression of these 10 core genes in BC. Moreover, Gene Expression Profiling Interactive Analysis (GEPIA) was applied to analyze the correlation between the expression of 10 core genes and ER, PR, and HER2. Then, 10 core genes were imported into the Kaplan-Meier (KM) plotter online database to reveal the significant prognostic information. Finally, CCND1 was found to be a prognostic biomarker for both ER-positive/HER2-negative BC and ER-negative/HER2-negative BC. In addition, PGR (also known as PR) was found to be a prognostic biomarker for ER-positive/HER2-negative BC. Trefoil factor 1 (TFF1), anterior gradient 2 (AGR2) and epidermal growth factor receptor (EGFR) were identified as node metastasis genes for the two subtypes of BC. Taken above, we identified these five genes (CCND1, PGR, TFF1, AGR2 and EGFR) as key DEGs between ER-positive/HER2-negative BC and ER-negative/HER2-negative BC.
Methods
Microarray data information
NCBI-GEO is a free and powerful public database of microarray/gene profiles. By conducting a search of GEO (https://www.ncbi.nlm.nih.gov/geo/), we obtained the gene expression profiles of GSE22093 and GSE23988 in ER-positive/HER2-negative BC and ER-negative/HER2-negative BC tissues. Microarray data of GSE22093 and GSE23988 were all based on GPL96 platforms [(HG-U133A) Affymetrix Human Genome U133A Array], which included 42 ER-positive/HER2-negative BC and 56 ER-negative/HER2-negative BC tissues, and 32 ER-positive/HER2-negative BC and 29 ER-negative/HER2-negative BC tissues, respectively.
Data processing of DEGs
Utilizing the GEO2R online tools (https://www.ncbi.nlm.nih.gov/geo/geo2r/), DEGs between ER-positive/HER2-negative BC specimens and ER-negative/HER2-negative BC specimens were identified with |logFC| >1 and adjusted P value <0.05 (5). The DEGs with logFC >0 were regarded as upregulated genes. In addition, the DEGs with logFC <0 were regarded as downregulated genes. Then, using the Venn software online, the raw data from the two datasets were integrated to detect the common DEGs among the two datasets.
Gene ontology and pathway enrichment analyses
We utilized gene ontology (GO) analysis to identify the characteristic biological attributes of the DEGs (6). In addition, we applied Kyoto Encyclopedia of Gene and Genome (KEGG) pathway enrichment analysis to identify functional attributes for DEGs (7). The Online Database for DAVID and KEGG was used to access GO and pathway enrichment analysis. Additionally, DAVID was applied to visualize the DEG enrichment of biological processes (BPs), molecular functions (MFs), cell components (CCs) and pathways in our study (P<0.05).
PPI network construction and module analysis
We applied STRING (Search Tool for the Retrieval of Interacting Genes) to evaluate and construct the PPI information (8). Then, we used the STRING app in Cytoscape to identify the potential correlation between these DEGs (maximum number of interactors =0 and confidence score ≥0.4) (9). Additionally, we utilized the MCODE app in Cytoscape to study the modules of the PPI network (degree cutoff =2, max depth =100, k-core =2, and node score cutoff =0.2).
Survival analysis and expression of core genes
Using UALCAN, we verified the gene expression levels of 10 central genes in invasive breast carcinoma tissues from The Cancer Genome Atlas (TCGA) database (10). In addition, the GEPIA website was applied to analyze the correlation between ER and 10 central genes, PR and 9 central genes, and HER2 and 10 central genes (11). KM plotter is a commonly applied website tool used to assess the effect of large numbers of genes on survival based on the European Genome-phenome Archive, TCGA and GEO databases (Affymetrix microarrays only) (https://kmplot.com/analysis/index.php?p=service&cancer=pancancer_rnaseq) (12). With 95% confidence intervals, the log rank P values and hazard ratios were analyzed and displayed on the plot.
Results
Identification of DEGs between ER-positive/HER2-negative BC tissues and ER-negative/HER2-negative BC tissues
There were 74 ER-positive/HER2-negative BC tissues and 85 ER-negative/HER2-negative BC tissues in our present study. Using GEO2R online tools, 163 and 635 DEGs were extracted from GSE22093 and GSE23988, respectively. Then, Venn diagram software was applied to identify the common DEGs in the two datasets. We found that a total of 93 common DEGs were detected between ER-negative/HER2-negative BC and ER-positive/HER2-negative BC tissues, including 30 downregulated genes (logFC <0) and 63 upregulated genes (logFC >0) (Table 1, Figure 1).
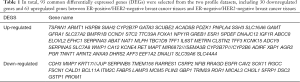
Full table
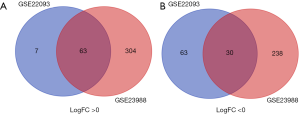
DEG gene ontology and KEGG pathway analysis in breast cancer
All 93 DEGs were analyzed via DAVID software. The results of GO analysis showed that (I) in terms of BPs, upregulated DEGs were particularly enriched in response to iron ion, response to estradiol, phosphatidylinositol 3-kinase signaling, endoplasmic reticulum unfolded protein response, lung goblet cell differentiation, and ureter maturation, and downregulated DEGs were enriched in cellular response to starvation, positive regulation of gene expression, positive regulation of cyclin-dependent protein serine/threonine kinase activity involved in G1/S transition of mitotic cell cycle, positive regulation of superoxide anion generation, eyelid development in camera-type eye, and digestive tract morphogenesis; (II) in terms of CCs, upregulated DEGs were significantly enriched in the extracellular exosome, neuronal cell body, dendrite, axoneme, integral component of plasma membrane, and integral component of endoplasmic reticulum membrane, and downregulated DEGs were enriched in the cell-cell adherens junction, plasma membrane, extracellular exosome, perinuclear region of cytoplasm extracellular space, vesicle; and (III) in terms of MFs, upregulated DEGs were enriched in enzyme binding, transcriptional activator activity, RNA polymerase II core promoter proximal region sequence-specific binding, RNA polymerase II transcription factor activity, and sequence-specific DNA binding while downregulated DEGs were not significantly enriched for any MF (Table 2).
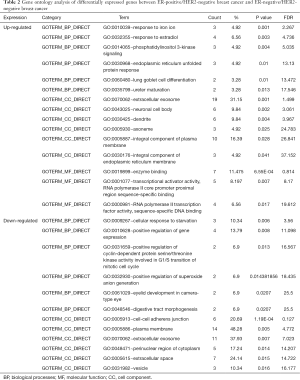
Full table
KEGG analysis results showed that upregulated DEGs were particularly enriched in progesterone-mediated oocyte maturation. However, there were no significant pathways for downregulate DEGs (P<0.05, Table 3).

Full table
Protein-protein interaction network (PPI) and modular analysis
A total of 60 DEGs were imported into the DEG PPI network complex, including 23 downregulated and 37 upregulated genes. There were 60 nodes and 134 edges in the network (Figure 2A). In addition, 33 of the 93 DEGs were not contained in the DEG PPI network (Figure 2A). Then, Cytoscape MCODE was utilized for further analysis, and the results demonstrated that 10 central nodes were identified among the 60 nodes, including 9 upregulated genes and EGFR, a downregulated gene (Figure 2B).
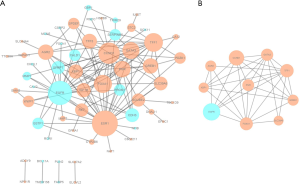
Analysis of core genes by UALCAN
Using UALCAN, we verified the expression of the 10 central genes in invasive breast carcinoma based on the breast cancer subtype. The expression levels of TFF1, SLC39A6, CCND1, XBP1, FOXA1, AGR2, GATA3, PGR and GREB1 in BC tissues of the luminal subclass were higher than those in BC tissues with triple negative subclass. Furthermore, EGFR expression was lower in luminal BC tissues than in triple negative BC tissues (P<0.05, Figure 3).
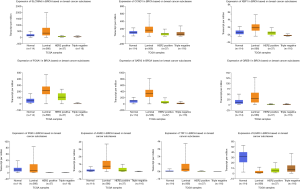
In addition, using the online tool, we verified the expression of the 10 central genes in breast invasive carcinoma based on nodal metastasis status from TCGA database. In tissues with N0 nodal metastasis status, the expression levels of TFF1, SLC39A6, FOXA1, AGR2, GATA3 and GREB1 in BC tissues were higher than those in normal breast tissues. Furthermore, in tissues with N1 nodal metastasis status, their expression in BC tissues was higher than that in BC tissues with N0 nodal metastasis status. However, EGFR expression was lower in BC tissues with N0 nodal metastasis than in normal breast tissues. In tissues with N1 nodal metastasis status, the expression of EGFR in BC tissues was lower than that in BC tissues with N0 nodal metastasis status (P<0.05, Figure 4).
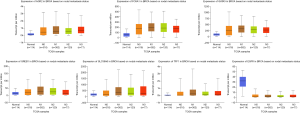
Analysis of core genes by KM plotter and GEPIA
To understand the possible correlation between the 10 central genes and ER, PR and HER2, these genes were analyzed via GEPIA. The results of Pearson correlation analysis showed that the expression levels of TFF1, SLC39A6, CCND1, XBP1, FOXA1, AGR2, GATA3, PGR and GREB1 were positively correlated with the expression of ER. However, the expression of EGFR was negatively correlated with the expression of ER (P<0.05, Figure 5). In addition, there was no significant correlation between the expression of PR and the expression of CCND1 and EGFR (P>0.05, Figure 6). There was no significant correlation between the expression of HER2 and the expression of TFF1, CCND1 XBP1, AGR2 and EGFR (P>0.05, Figure 7).
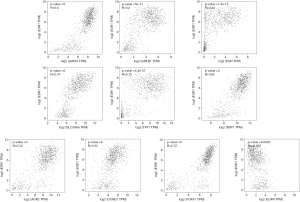
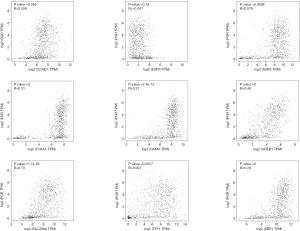
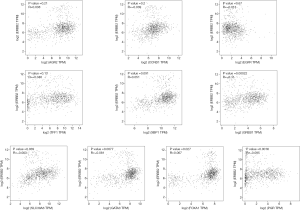
KM plotter (http://kmplot.com/analysis) was used to identify associations between survival data and the expression of the 10 central genes. ER-positive/HER2-negative patients with high expression of PGR had significantly shorter survival than patients with low expression in the KM plotter protein expression analysis (P<0.05, Figure 8A). In addition, ER-negative/HER2-negative BC patients with high expression of CCND1 had significantly shorter survival than patients with low expression in the KM plotter protein analysis (P<0.05, Figure 8B). Both ER-positive patients and ER-negative patients with high expression of CCND1 had significantly shorter survival than patients with low expression in the KM plotter mRNA analysis (P<0.05, Figure 8C,D).
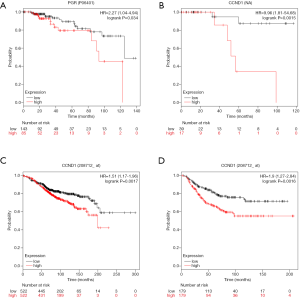
Discussion
Using bioinformatics methods, 63 upregulated DEGs and 30 downregulated DEGs were identified between ER-positive/HER2-negative BC tissues and ER-negative/HER2-negative BC tissues in this study. The DEGs were classified into three groups by GO terms. The GO analysis using DAVID methods showed that (I) in terms of BPs, upregulated DEGs were particularly enriched in response to estradiol and phosphatidylinositol 3-kinase signaling; downregulated DEGs were particularly enriched in positive regulation of gene expression and positive regulation of cyclin-dependent protein serine/threonine kinase activity involved in G1/S transition of mitotic cell cycle; (II) in terms of CCs, downregulated DEGs were enriched in cell-cell adherens junctions; and (III) in terms of MFs, upregulated DEGs were enriched in enzyme binding, transcriptional activator activity, RNA polymerase II core promoter proximal region sequence-specific binding and RNA polymerase II transcription factor activity, and sequence-specific DNA binding. In the pathway analysis, upregulated DEGs were particularly enriched in progesterone-mediated oocyte maturation.
Then, the STRING online database and Cytoscape software were applied to establish the DEG PPI network of 60 nodes and 134 edges. Furthermore, 9 central upregulated genes (TFF1, SLC39A6, CCND1, XBP1, FOXA1, AGR2, GATA3, PGR and GREB1) and 1 central downregulated gene (EGFR) were selected from the PPI network complex via the Cytoscape MCODE analysis app.
Furthermore, through GEPIA analysis, we found that the expression levels of TFF1, SLC39A6, CCND1, XBP1, FOXA1, AGR2, GATA3, PGR and GREB1 were positively correlated with the expression of ER in BC. The expression of EGFR was negatively correlated with the expression of ER (P<0.05, Figure 5). However, there was no significant correlation between the expression of PR and the expression of CCND1 and EGFR in BC (P>0.05, Figure 6). In addition, there was no significant correlation between the expression of HER2 and the expression of TFF1, CCND1, XBP1, AGR2 and EGFR in BC (P>0.05, Figure 7).
Then, the expression of the 10 central genes in breast invasive carcinoma based on the breast cancer subclass was verified by UALCAN. The expression levels of TFF1, SLC39A6, CCND1, XBP1, FOXA1, AGR2, GATA3, PGR and GREB1 in BC tissues of the luminal subclass (ER+, PR+ and HER2-) were higher than those in BC tissues of the triple negative subclass (ER-, PR- and HER2-). Furthermore, EGFR expression was lower in BC tissues of the luminal subclass than in BC tissues of the triple negative subclass (P<0.05, Figure 3).
Taken above, the results of our study suggest that the expression of CCND1 and PGR is positively correlated with ER in ER-positive/HER2-negative BC and ER-negative/HER2-negative BC. The expression of EGFR is negatively correlated with that of ER in ER-positive/HER2-negative BC and ER-negative/HER2-negative BC. The expression of CCND1 and PGR in ER-positive/HER2-negative BC tissues was higher than that in ER-negative/HER2-negative BC tissues. Furthermore, EGFR expression was lower in ER-positive/HER2-negative BC tissues than in ER-negative/HER2-negative BC tissues.
Previous studies found that cyclin-dependent kinase 4 (CDK4) and CDK6 integrated with cyclin D1 (encoded by CCND1), cyclin D2 and cyclin D3 to phosphorylate retinoblastoma protein (RB) and then promoted cell cycle entry and progression from G1 to S phase. The cyclin D1-CDK4/6-RB network plays an oncogenic role in BC, and the network is a target for therapy that may improve outcomes in BC patients. Furthermore, the dysregulation of the cyclin D1-CDK4/6-RB network leads to aberrant activation of the cell cycle, which plays an important role in endocrine resistance in BC. In addition, the amplification of CCND1 (encoding cyclin D1) activates the cyclin D1-CDK4/6-RB pathway. In addition, there are several mechanisms, including the RAS-MAPK and PI3K pathways, that can stimulate cyclin-CDK4/6 complexes and then inhibit RB and promote cell proliferation (1,13). Furthermore, studies found that in addition to its kinase-dependent functions that regulate stem cell self-renewal and promote cellular migration, cyclin D1 is a key inhibitor of differentiation of the breast stem cell population (14). Cyclin D1 functions in a kinase-independent manner to enhance DNA repair and binds DNA in the context of chromatin to regulate the expression of genes governing chromosomal instability. In addition, by enhancing DNA repair, cyclin D1 may protect transformed cells from excessive genomic instability. It can protect BC cells from DNA-damaging therapies (14). Previous studies demonstrated that CCND1 amplification was found in 5−30% of ER-positive BCs, and its amplification has been consistently associated with poor outcome in patients with ER-positive tumors. Studies have suggested that its amplification might be a valuable predictive biomarker of endocrine therapy resistance and that more aggressive treatment should be conducted (1,3,13). CCND1 was found to be a transcriptional target of ER in a previous study. In addition, it might activate ER in a ligand-independent fashion (3). In addition, estrogens have been demonstrated to promote BC cell proliferation by associating with regulatory elements in the genome, thereby enhancing the transcription of cyclin D1 (15). CCND1 is a marker of tamoxifen resistance in BC (16). ER-positive BC with CCND1 amplification is not sensitive to tamoxifen treatment. Furthermore, CCND1 is a target gene of the signal transducer and activator of transcription 3 (STAT3) pathway (16,17). A previous study demonstrated that STAT3 pathway inhibition downregulated the transcription and translation of CCND1 in ER-positive BC and enhanced the sensitivity of breast cancer to tamoxifen (16).
As a member of the nuclear receptor family, ER alpha comprises many functional domains, including two transcriptional activation function domains (AF1 and AF2) and a C-terminal binding domain (13,18). The C-terminal domain is a ligand-dependent receptor. Ligand binding to the C-terminal domain leads to receptor dimerization, nuclear translocation and transactivation of target gene expression. In addition to binding to ligands (e.g., estrogen), membrane-bound ERs can be activated by other signaling pathways, such as EGFR, FGFR and HER2, which contribute greatly to tumor cell proliferation and apoptosis (13,19). Previous studies found that the overexpression of EGFR was associated with more aggressive BC subtypes and poorer outcomes in HR-positive BC patients (20). In addition, the expression of EGFR was negatively associated with the expression of ER-α (20). As ligands bind to the extracellular region, EGFR is activated. Then, the downstream signaling pathways, such as mitogen-activated protein kinase (MAPK) and phosphoinositide 3-kinases (PI3K)/AKT pathways, are activated by EGFR. This aberrant activation promotes cancer cell proliferation, motility, and survival (21). The EGFR gene is mutated or overexpressed in a number of cancers, including BC. Overexpression or mutation can promote tumor progression and drug resistance in many cancers. The activation of EGFR leads to the loss of ER in ER-positive BC. Then, it can enhance tamoxifen resistance. Therefore, EGFR might be a therapeutic target for overcoming tamoxifen resistance in ER-positive BC with high EGFR expression (20). Compared with other subtypes of BC, aberrant expression of EGFR is more common in TNBC, and the overexpression of EGFR is regarded as a factor of poor outcome for TNBC (21,22).
The results of a previous study demonstrated that HR subtype, considering ER and PR status, is a significant independent prognostic factor in female patients with operable invasive BC that is associated with long-term effects. The ER-positive/PR-positive subtype has the best prognosis, followed by the ER-positive/PR-negative subtype, ER-negative/PR-positive subtype, and ER-negative/PR negative subtype (23). This suggests that the expression of ER and PR is associated with the prognosis of BC. PR is induced by estrogen (15). Furthermore, the expression of PR is regarded as a biomarker for a functional ER. In the presence of progesterone, PR interacts with the ER and alters the location at which the ER binds to chromatin (15,24,25). The alteration of binding location leads to the modulation of genes implicated in the proliferation switch to regulate genes associated with cell cycle arrest, differentiation and apoptosis (15). In addition to nuclear transcription factor, PR binds to and activates a great number of cell membrane/cytoplasmic protein kinase signaling pathways, including MAPK pathway, which promotes cell proliferation (26). Both extranuclear actions of PR and transcriptional activity of PR are integrated via the activation of kinases. These kinases modify either PR or other factors associated with PR at specific target gene sites. The association of a PR/Stat3 complex is inducing by the phosphorylation of Stat3 by progestins. Stat3 is a co-activator of PR (26). Furthermore, the phosphorylation of the EGFR by progestins will lead to the activation of p42/p44 MAPK. This phosphorylation is required for the EGFR upregulation and BC cell proliferation (26).
Consistent with the above findings, our study demonstrates that the expression of ER is strongly positively correlated with the expression of PGR (PR) and CCND1 in ER-positive/HER2-negative BC and ER-negative/HER2-negative BC. In addition, the expression of ER is strongly negatively correlated with the expression of EGFR in ER-positive/HER2-negative BC and ER-negative/HER2-negative BC.
A number of studies have found that patients with ER-positive/PR-positive BC are more likely to benefit from endocrine therapy than patients with ER-positive/PR-negative BC (15,27). However, BC patients with ER-positive/PR-negative tumors do not seem to benefit from endocrine therapy. In addition, PR-positive BC patients should benefit from endocrine therapy based on the investigation of the influence of different levels of PR positivity on recurrence risk (28). However, with high expression of PGR, ER-positive/HER2-negative patients had a significantly shorter survival via KM plotter breast protein analysis in our study (P<0.05, Figure 8A). As these are conflicting findings, further clinical studies are required to confirm the predictive value for ER-positive/HER2-negative BC.
Moreover, with high expression of CCND1, ER-negative/HER2-negative BC patients had a significantly shorter survival via KM plotter breast protein analysis in our study (P<0.05, Figure 8B). Additionally, both ER-positive patients and ER-negative patients with high expression of CCND1 had significantly shorter survival than patients with low expression in the KM plotter mRNA analysis (P<0.05, Figure 8C,D).
Taken together, these findings suggest that PGR may be a prognostic biomarker for ER-positive/HER2-negative BC. In addition, with no significant correlation with PR and HER2 (P>0.05, Figures 6,7), CCND1 may be a prognostic biomarker for ER-positive/HER2-negative BC and ER-negative/HER2-negative BC.
In tissues with N0 nodal metastasis status, the expression levels of TFF1, SLC39A6, FOXA1, AGR2, GATA3 and GREB1 in BC tissues were higher than those in normal breast tissues. Furthermore, in tissues with N1 nodal metastasis status, the expression of these genes in BC tissues was higher than that in BC tissues with N0 nodal metastasis status. However, EGFR expression was lower in BC tissues with N0 nodal metastasis than in normal breast tissues. In addition, in tissues with N1 nodal metastasis status, the expression of EGFR in BC tissues was lower than that in BC tissues with N0 nodal metastasis status (P<0.05, Figure 4).
A previous study found that the expression of AGR2 was associated with the expression of ER, especially in ER-positive BC patients. In addition, AGR2 is associated with poor prognosis in ER-positive BC (29). Furthermore, the overexpression of AGR2 enhances resistance to endocrine therapy in ER-positive BC patients (29). A previous study found that AGR2 was induced by ER (30). Others reported that through binding to the AGR2 promoter, ER can activate the transcription of AGR2, regardless of whether it is present in cell lines or cancer samples of BC. ER-positive BC samples expressed higher levels of AGR2 than ER-negative samples. In addition, the expression of AGR2 in ER-positive cells was higher than that in ER-negative cells (31). In addition, several studies have demonstrated that AGR2 is a mediator of a number of tumor signaling pathways, including EGFR and cyclin D1 (29). In addition, AGR2 has been widely regarded as a metastasis-related protein and a prognostic biomarker of poor outcome. Furthermore, as a metastasis-related protein, AGR2 has been intensively studied in multiple tumors, including BC (29,30). TFF1 is regulated by estrogen and has been used as a marker of the estrogen gene. High expression of TFF1 has been found in BC. A previous study found that the expression of TFF1 in the serum of ER-positive BC patients was significantly higher than that in the serum of ER-negative BC patients. In addition, the expression of TFF1 in TNBC was lower than that in non-TNBC. In addition, the expression of TFF1 was significantly associated with nodal status and ER status. Serum TFF1 could be used to predict the prognosis of patients with BC, especially non-TNBC (32). A previous study found that the level of TFF1 mRNA in the blood of metastatic BC patients were significantly higher than non-metastatic BC patients. In addition, the level of TFF1 in blood was significantly associated with the estrogen status of BC patients. The high level of TFF1 mRNA in blood was significantly associated with BC metastasis to bone (33).
Since there is no significant correlation between the expression of TFF1, AGR2 and EGFR and the expression of HER2 (P>0.05, Figure 7), we speculate that the expression of TFF1, AGR2 and EGFR might be closely related to nodal metastasis status in ER-positive/HER2-negative BC and ER-negative/HER2-negative BC. With its significant positive correlation with ER, high expression of TFF1 or AGR2 might suggest nodal metastasis in ER-positive/HER2-negative BC and ER-negative/HER2-negative BC. In addition, with its significant negative correlation with ER, low expression of EGFR might suggest nodal metastasis in ER-positive/HER2-negative BC and ER-negative/HER2-negative BC. This suggests that compared to ER-negative/HER2-negative BC, ER-positive/HER2-negative BC with higher expression of TFF1 or AGR2 is likely to be more prone to nodal metastasis. In addition, compared to ER-positive/HER2-negative BC, ER-negative/HER2-negative BC with lower expression of EGFR is likely to be more prone to nodal metastasis.
Conclusions
Taken together, our study demonstrates that the expression of CCND1, AGR2, PGR, TFF1 and EGFR is strongly associated with the expression of ER in ER-positive/HER2-negative BC and ER-negative/HER2-negative BC. PGR might be a prognostic biomarker for ER-positive/HER2-negative BC. CCND1 could be a poor prognostic biomarker for ER-positive/HER2-negative BC and ER-negative/HER2-negative BC. In addition, TFF1, AGR2 and EGFR might be predictive biomarkers of node metastasis in ER-positive/HER2-negative BC and ER-negative/HER2-negative BC. Overall, CCND1, AGR2, PGR, TFF1 and EGFR are the key DEGs between ER-positive/HER2-negative BC and ER-negative/HER2-negative BC. Furthermore, these predictions should be verified by a series of studies in the future.
Acknowledgments
Funding: This study was funded by Guangdong Medical University Research Fund (grant no. GDMUM 201818, GDMUM 201922 and GDMUM 201936), National Natural Science Foundation of China (grant no. 81702285), Natural Science Foundation of Guangdong Province (grant no. 2016A030313822).
Footnote
Conflicts of Interest: All authors have completed the ICMJE uniform disclosure form (available at http://dx.doi.org/10.21037/gs.2020.03.40). The authors have no conflicts of interest to declare.
Ethical Statement: The authors are accountable for all aspects of the work in ensuring that questions related to the accuracy or integrity of any part of the work are appropriately investigated and resolved.
Open Access Statement: This is an Open Access article distributed in accordance with the Creative Commons Attribution-NonCommercial-NoDerivs 4.0 International License (CC BY-NC-ND 4.0), which permits the non-commercial replication and distribution of the article with the strict proviso that no changes or edits are made and the original work is properly cited (including links to both the formal publication through the relevant DOI and the license). See: https://creativecommons.org/licenses/by-nc-nd/4.0/.
References
- Harbeck N, Penault-Llorca F, Cortes J, et al. Breast cancer. Nat Rev Dis Primers 2019;5:66. [Crossref] [PubMed]
- Majidinia M, Yousefi B. DNA repair and damage pathways in breast cancer development and therapy. DNA Repair 2017;54:22-9. [Crossref] [PubMed]
- Shiu KK, Natrajan R, Geyer FC, et al. DNA amplifications in breast cancer: genotypic–phenotypic correlations. Future Oncol 2010;6:967-84. [Crossref] [PubMed]
- Waks AG, Winer EP. Breast Cancer Treatment: A Review. JAMA 2019;321:288-300. [Crossref] [PubMed]
- Ashburner M, Ball CA, Blake JA, et al. Gene ontology: Tool for the unification of biology. Nat Genet 2000;25:25-9. [Crossref] [PubMed]
- Huang W. Sherman BT, Lempicki RA. Systematic and integrative analysis of large gene lists using DAVID bioinformatics resources. Nat Protoc 2009;4:44-57. [Crossref] [PubMed]
- Huang W. Sherman BT, Lempicki RA. Bioinformatics enrichment tools: paths toward the comprehensive functional analysis of large gene lists. Nucleic Acids Res 2009;37:1-13. [Crossref] [PubMed]
- Szklarczyk D, Gable AL, Lyon D, et al. STRING v11: protein–protein association networks with increased coverage, supporting functional discovery in genome-wide experimental datasets. Nucleic Acids Res 2019;47:D607-13. [Crossref] [PubMed]
- Otasek D, Morris JH, Boucas J, et al. Cytoscape Automation: empowering workflow-based network analysis. Genome Biol 2019;20:185. [Crossref] [PubMed]
- Chandrashekar DS, Bashel B, Balasubramanya SAH, et al. UALCAN: A Portal for Facilitating Tumor Subgroup Gene Expression and Survival Analyses. Neoplasia 2017;19:649-58. [Crossref] [PubMed]
- Tang Z, Li C, Kang B, et al. GEPIA: a web server for cancer and normal gene expression profiling and interactive analyses. Nucleic Acids Res 2017;45:W98-102. [Crossref] [PubMed]
- Menyhárt O, Nagy A. Determining consistent prognostic biomarkers of overall survival and vascular invasion in hepatocellular carcinoma. R Soc Open Sci 2018;5:181006. [Crossref] [PubMed]
- Gul A, Leyland-Jones B, Dey N, et al. A combination of the PI3K pathway inhibitor plus cell cycle pathway inhibitor to combat endocrine resistance in hormone receptor-positive breast cancer: a genomic algorithm-based treatment approach. Am J Cancer Res 2018;8:2359-76. [PubMed]
- Casimiro MC, Crosariol M, Loro E, et al. Cyclins and cell cycle control in cancer and disease. Genes Cancer 2012;3:649-57. [Crossref] [PubMed]
- Nicolini A, Ferrari P, Duffy MJ. Prognostic and Predictive Biomarkers in Breast Cancer: Past, Present and Future. Semin Cancer Biol 2018;52:56-73. [Crossref] [PubMed]
- Xing J, Li J, Fu L, et al. SIRT4 enhances the sensitivity of ER-positive breast cancer to tamoxifen by inhibiting the IL-6/STAT3 signal pathway. Cancer Med 2019;8:7086-97. [Crossref] [PubMed]
- Li Z, Cui J, Yu Q, et al. Evaluation of CCND1 amplification and CyclinD1 expression: diffuse and strong staining of CyclinD1 could have same predictive roles as CCND1 amplification in ER positive breast cancers. Am J Transl Res 2016;8:142-53. [PubMed]
- Anbalagan M, Rowan BG. Estrogen receptor alpha phosphorylation and its functional impact in human breast cancer. Mol Cell Endocrinol 2015;418:264-72. [Crossref] [PubMed]
- Vrtačnik P, Ostanek B, Mencej-Bedrač S, et al. The many faces of estrogen signaling. Biochem Med (Zagreb) 2014;24:329-42. [Crossref] [PubMed]
- Jeong Y, Bae SY, You D, et al. EGFR is a Therapeutic Target in Hormone Receptor-Positive Breast Cancer. Cell Physiol Biochem 2019;53:805-19. [Crossref] [PubMed]
- Nakai K, Hung MC, Yamaguchi H. A perspective on anti-EGFR therapies targeting triple-negative breast cancer. Am J Cancer Res 2016;6:1609-23. [PubMed]
- Marmé F, Schneeweiss A. Targeted Therapies in Triple-Negative Breast Cancer. Breast Care (Basel) 2015;10:159-66. [Crossref] [PubMed]
- Hwang KT, Kim J, Jung J, et al. Long-term prognostic effect of hormone receptor subtype on breast cancer. Breast Cancer Res Treat 2020;179:139-51. [Crossref] [PubMed]
- Mohammed H, Russell IA, Stark R, et al. Progesterone receptor modulates ERα action in breast cancer. Nature 2015;523:313-7. [Crossref] [PubMed]
- Yip CH, Rhodes A. Estrogen and progesterone receptors in breast cancer. Future Oncol 2014;10:2293-301. [Crossref] [PubMed]
- Cenciarini ME, Proietti CJ. Molecular mechanisms underlying progesterone receptor action in breast cancer: Insights into cell proliferation and stem cell regulation. Steroids 2019;152:108503. [Crossref] [PubMed]
- Nordenskjöld A, Fohlin H, Fornander T, et al. Progesterone receptor positivity is a predictor of long-term benefit from adjuvant tamoxifen treatment of estrogen receptor positive breast cancer. Breast Cancer Res Treat 2016;160:313-22. [Crossref] [PubMed]
- Purdie CA, Quinlan P, Jordan LB, et al. Progesterone receptor expression is an independent prognostic variable in early breast cancer: a population-based study. Br J Cancer 2014;110:565-72. [Crossref] [PubMed]
- Salmans ML, Zhao F, Andersen B. The estrogen-regulated anterior gradient 2 (AGR2) protein in breast cancer: a potential drug target and biomarker. Breast Cancer Res 2013;15:204. [Crossref] [PubMed]
- Obacz J, Takacova M, Brychtova V, et al. The role of AGR2 and AGR3 in cancer: similar but not identical. Eur J Cell Biol 2015;94:139-47. [Crossref] [PubMed]
- Guo J, Gong G, Zhang B. Identification and prognostic value of anterior gradient protein 2 expression in breast cancer based on tissue microarray. Tumour Biol 2017;39:1010428317713392. [Crossref] [PubMed]
- Yi J, Ren L, Li D, et al. Trefoil factor 1 (TFF1) is a potential prognostic biomarker with functional significance in breast cancers. Biomed Pharmacother 2020;124:109827. [Crossref] [PubMed]
- Elnagdy MH, Farouk O. TFF1 and TFF3 mRNAs Are Higher in Blood from Breast Cancer Patients with Metastatic Disease than Those without. J Oncol 2018;2018:4793498. [Crossref] [PubMed]