Preliminary study on identification of estrogen receptor-positive breast cancer subtypes based on dynamic contrast-enhanced magnetic resonance imaging (DCE-MRI) texture analysis
Introduction
Worldwide, breast cancer has developed into the leading cause of cancer death in women (1). Currently, breast cancer is divided into four molecular types at the genetic level in medicine, including Luminal A, Luminal B, HER-2 overexpression (HER-2) and basal cell (basal like) (2). Among them, Luminal A and Luminal B breast cancer histopathology are expressed as positive estrogen receptor (ER), and/or positive progesterone receptor (PR), so they collectively referred to as ER positive breast cancer. ER-positive breast cancer is the most common types, accounting for 60% to 80% of all breast cancers (3). Molecular typing of ER-positive breast cancer has significant implications for the choice of treatment regimen and patient prognosis. The prognosis of patients with ER-positive early breast cancer in the first 5 years after surgery is better than that in patients with negative hormone receptors, and the prognosis is relatively poor, with 5–15 years after diagnosis (4).
Immunohistochemistry is commonly used in clinical work to determine molecular typing, which is not only invasive but also time consuming. In many areas of oncology, dynamic contrast-enhanced magnetic resonance imaging (DCE-MRI) has proven to be a clinically useful, non-invasive functional imaging technique to quantify tumor vasculature and tumor perfusion characteristics (5). Texture analysis is an emerging computer-aided diagnosis technology that can quantitatively display subtle changes in image pixels and arrangement, independence of image grayscale and subjective factors of the diagnostics, and its evaluation value for various tumor heterogeneities is obtained. It has generally been confirmed that it has gradually become a research hotspot in recent years (6-9).
However, the differential diagnosis of ER-positive breast cancer subtypes is rare. Therefore, the purpose of this study was to explore the feasibility of using DCE-MRI texture analysis to distinguish Luminal A breast cancer and Luminal B breast cancer.
Methods
Subjects
This study was a retrospective analysis. Patients with ER-positive breast invasive ductal carcinoma confirmed by surgery and pathology from January 20 to October 2018 were included in this study. Inclusion criteria: (I) patients who were confirmed by pathology as ER-positive breast invasive ductal carcinoma; (II) patients with intact mammary gland DCE-MRI data; (III) patients whose image quality and shooting conditions met diagnostic criteria. Exclusion criteria: patients undergoing puncture or neoadjuvant chemotherapy before imaging examination. Finally, 51 patients were enrolled, including 19 patients with Luminal A, with an average age of (50.59±7.73) years, and 32 patients with Luminal B, with an average age of (54.34±12.73) years. All patients with breast cancer underwent tumor resection within 1 week after magnetic resonance (MR) examination and were confirmed by pathological examination. Before performing this study, all patients signed informed consent form, and this study was approved by the Ethics Committee of The Central Hospital of Wuhan, Tongji Medical College, Huazhong University of Science and Technology.
Breast DCE-MRI image acquisition
The PHILIPS Achieva 3.0T TX superconducting MR scanner with an 8-channel double-cavity breast phased array coil was used. Dynamic enhanced scanning was performed by dyn-eTHRIVE sequence volume imaging, with TR/TE of 4.7 ms/2.3 ms and volume of 1 mm in all positions. The mask was scanned before dynamic enhancement, then the high-pressure syringe was used to inject the Magnevis 0.2 mmol/kg through the elbow vein, then bolus injection of 30 mL normal saline in 2 mL/s. Dynamic enhanced scan was immediately performed after the end of the injection. One phase per minute was acquired, and the entire scan sequence collected a total of 8 phases. The MR imaging findings of all patients were independently performed by two senior imaging diagnosticians. Dynamically enhanced scanning of the third phase image were selected (10,11).
Texture analysis
The selected images were imported into the computer aided diagnostic platform Firevoxel [Firevoxel; Center for Advanced Imaging Innovation and Research (CAI2R), New York University School of Medicine, New York, NY, USA] in DICOM format for analysis. Follow the blind law principle, two mammography MR diagnosticians (working years were 12 and 6 years respectively) manually sketched and filled the area of interest (ROI). Tumor ROI was manually defined on each axis of the lesion DCE-MRI sequence, and each layer of ROI included as much tumor tissue as possible, including any cystic, necrotic or hemorrhagic components, for better evaluation of inhomogeneity. However, in order to reduce the partial volume effect, the ROI was slightly smaller than the actual tumor size. The largest lesion was selected in multifocal multicenter cases. Before the film was read, the physician knew that the histopathology was ER-positive breast cancer, but they did not know other clinical data, such as molecular marker information of the tumor. The software automatically extracted the texture parameters (mean, standard deviation, skewness, kurtosis, unevenness and entropy) and the size of the volume. Each parameter was extracted twice and averaged.
Molecular typing standard
The molecular subtypes of ER-positive breast cancer were defined as follows according to the 2017 edition of the Guidelines and Specifications for Breast Cancer Diagnosis and Treatment in China (12) (Table 1).
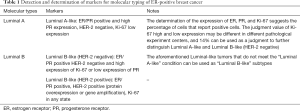
Full table
Statistical analysis
Statistical analysis was performed using SPSS 19.0 software. Measurement data were expressed as means ± standard deviation (
Results
Schematic diagram of single layer target area of Luminal A and Luminal B types
DCE-MRI was performed for 51 patients, the representative diagram of single layer target area for patient with Luminal A and Luminal B types were shown in Figure 1A,B. Histogram and output value interface for texture analysis results after the completion of the entire tumor target area of Luminal A and Luminal B types could be found in Figure 1C,D.
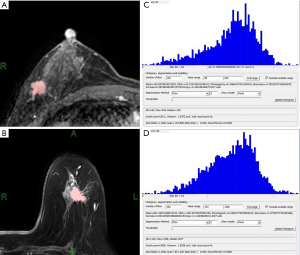
Results of texture analysis
As shown in Table 2, there were no significant differences in mean, standard deviation, skewness, and volume between Luminal A and Luminal B. However, the other three characteristics (peak, inhomogeneity, and entropy) were statistically significant (P<0.01). Among them, the kurtosis in Luminal A type was significantly smaller than that in Luminal B type (P<0.01); while heterogeneity and entropy in Luminal A type were markedly larger than those in Luminal B type (P<0.01, respectively) (Figure 2).
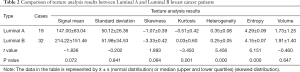
Full table
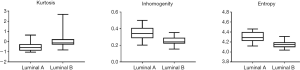
Diagnostic power of kurtosis, heterogeneity and entropy for ER-positive breast cancer subtypes
The diagnostic power of kurtosis, heterogeneity and entropy for ER-positive breast cancer subtypes were shown in Figure 3. The AUC of kurtosis, heterogeneity and entropy were 0.832, 0.859 and 0.891, respectively, and the differential diagnosis of entropy was the highest. The sensitivity of entropy ≤4.22 for Luminal B type was 90.62% and the specificity was 78.95%.
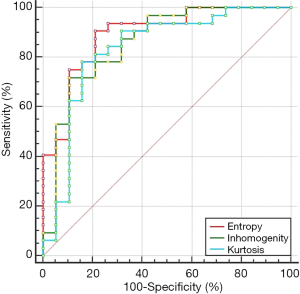
Discussion
At present, the clinical treatment plan for breast cancer is mainly based on molecular typing. For most patients, Luminal A requires only endocrine therapy and has a good prognosis, while Luminal B is usually treated with chemotherapy and endocrine therapy (13,14). Compared with clinical commonly used immunohistochemistry to determine molecular typing, texture analysis does not depend on the subjective factors and clinical experience of physicians, and assists in the diagnosis of diseases by quantitatively analyzing the texture characteristic parameters of images (15). This study preliminarily demonstrated the feasibility of DCE-MRI texture analysis to distinguish Luminal A and Luminal B breast cancer. Features extracted from DCE-MRI can be initially identified as potential biomarkers for identifying Lumina A and B of breast cancer at molecular subtypes.
In clinical work, doctors’ diagnosis of the disease depends to a large extent on the morphology of the lesion. Due to the limitations of the texture analysis software used in this study, only one morphological index of the lesion size was extracted. We believed that this may be related to the time of patient visit and the time of pathological diagnosis, which needed to be further verified by dynamic analysis under the same conditions.
In this study, kurtosis in Luminal A subtype breast cancer was lower than Luminal B subtype, while heterogeneity and entropy in the former has higher than the latter. Kurtosis is a parameter that describes the steepness of the data distribution curve, and is considered to be a biomarker of tumor heterogeneity (16), which is inversely related to treatment effectiveness (17). This was consistent with the clinical features of the Luminal A subtype, which had a lower invasiveness, a better prognosis, and a higher survival rate (18). Patients with grade 3 breast cancer or tumors with high expression of Ki-67 were associated with higher kurtosis and lower diffusivity coefficients (19). Diffusion Kurtosis at 3.0 T can be used as an in vivo imaging marker for breast cancer characterization (20).
Skewness is a parameter that describes the symmetry of the distribution of data curves (21). The skew of the normal distribution is zero, and the skewness of any symmetric data should be close to zero (22). A negative value of the skewness indicates data tilted to the left, and a positive value of the skewness indicates data tilted to the right. The two Luminal subtypes are all skewed to the left, and the Luminal B subtype is more oblique to the left, but the two subtypes have no statistical difference. This may be related to the invasive ductal carcinoma of the breast, the limitation of pathological types, and the small number of cases, so further research is needed. Inhomogeneity and entropy reflect the randomness and average information of the histogram. In the Luminal B subtype histogram, the pixel gray-scale frequency distribution is concentrated and the signal intensity is relatively uniform, while the Luminal A sub-pixel gray-scale frequency distribution is significantly reduced and uneven. This may be due to the fact that the Luminal B subtype has a stronger biological proliferative capacity and can take in more similar voxels, so the voxels seem to be more uniform.
By analyzing the ROC curves of three texture indicators (entropy, inhomogeneity and kurtosis) which were statistically different for identifying Luminal subtype breast cancer, it was concluded that the diagnostic efficiency of all three were high. The diagnostic efficiency of entropy was the highest. AUC reached 0.891, and the sensitivity of Luminal B breast cancer was higher when the entropy was less than 4.22, and the specificity was slightly lower. Holli-Helenius et al. (23) identified the Luminal subtype breast cancer by enhancing the pre-T1 weighted image (T1WI) plain image texture analysis, and the entropy diagnostic efficiency was also higher, with an AUC of 0.828. The mammography was used to analyze the benign and malignant breast nodules, the entropy had the highest discriminative efficiency, and the sensitivity and specificity were 89.0% and 72.0%, respectively (24). It indicated that entropy can be used as a best single texture indicator to identify benign and malignant small nodules. It could be seen that entropy was a commonly used parameter in texture analysis and had high diagnostic efficiency.
There are some limitations in this study: (I) because non-tumor breast cancer lesions were scattered and diffuse, the boundary determination was difficult for physicians to perform manual segmentation, and the non-tumor type breast cancer was not included to reduce the difference between observers due to difficulty in boundary recognition; (II) sample size was not enough, and was a single-center study; (III) independent analysis of several parameters commonly used in texture analysis, no systematic omics study; (IV) this study was only for ER-positive breast cancer subtype prediction, the lymph node metastasis had not been included in the research scope.
In summary, DCR-MRI texture analysis can accurately predict ER-positive breast cancer typing before surgery, and entropy was most likely to become a potential imaging marker, providing clinicians with corresponding reference value. But further study is still needed to verify our results.
Acknowledgments
Funding: None.
Footnote
Conflicts of Interest: All authors have completed the ICMJE uniform disclosure form (available at http://dx.doi.org/10.21037/gs.2020.04.01). The authors have no conflicts of interest to declare.
Ethical Statement: The authors are accountable for all aspects of the work in ensuring that questions related to the accuracy or integrity of any part of the work are appropriately investigated and resolved. Before performing this study, all patients signed informed consent form, and this study was approved by the Ethics Committee of The Central Hospital of Wuhan, Tongji Medical College, Huazhong University of Science and Technology.
Open Access Statement: This is an Open Access article distributed in accordance with the Creative Commons Attribution-NonCommercial-NoDerivs 4.0 International License (CC BY-NC-ND 4.0), which permits the non-commercial replication and distribution of the article with the strict proviso that no changes or edits are made and the original work is properly cited (including links to both the formal publication through the relevant DOI and the license). See: https://creativecommons.org/licenses/by-nc-nd/4.0/.
References
- Bravi F, Decarli A, Russo AG. Risk factors for breast cancer in a cohort of mammographic screening program: a nested case-control study within the FRiCaM study. Cancer Med 2018;7:2145-52. [Crossref] [PubMed]
- Glajcar A, Szpor J, Pacek A, et al. The relationship between breast cancer molecular subtypes and mast cell populations in tumor microenvironment. Virchows Archiv 2017;470:505-15. [Crossref] [PubMed]
- Amanatullah DF, Tamaresis JS, Chu P, et al. Local estrogen axis in the human bone microenvironment regulates estrogen receptor-positive breast cancer cells. Breast Cancer Res 2017;19:121. [Crossref] [PubMed]
- Sennerstam RB, Franzén BSH, Wiksell HOT, et al. Core-needle biopsy of breast cancer is associated with a higher rate of distant metastases 5 to 15 years after diagnosis than FNA biopsy. Cancer Cytopathol 2017;125:748-56. [Crossref] [PubMed]
- Mazaheri Y, Akin O, Hricak H. Dynamic contrast-enhanced magnetic resonance imaging of prostate cancer: A review of current methods and applications. World J Radiol 2017;9:416-25. [Crossref] [PubMed]
- Oppedal K, Engan K, Eftestøl T, et al. Classifying Alzheimer's disease, Lewy body dementia, and normal controls using 3D texture analysis in magnetic resonance images. Biomed Signal Process Control 2017;33:19-29. [Crossref]
- Sollini M, Cozzi L, Chiti A, et al. Texture analysis and machine learning to characterize suspected thyroid nodules and differentiated thyroid cancer: Where do we stand? Eur J Radiol 2018;99:1-8. [Crossref] [PubMed]
- Gilanie G, Bajwa UI, Waraich MM, et al. Computer aided diagnosis of brain abnormalities using texture analysis of MRI images. Int J Imaging Syst Technol 2019;29:260-71. [Crossref]
- Dhahbi S, Barhoumi W, Kurek J, et al. False-positive reduction in computer-aided mass detection using mammographic texture analysis and classification. Comput Methods Programs Biomed 2018;160:75-83. [Crossref] [PubMed]
- Jia ZZ, Shi W, Shi JL, et al. Comparison between perfusion computed tomography and dynamic contrast-enhanced magnetic resonance imaging in assessing glioblastoma microvasculature. Eur J Radiol 2017;87:120-4. [Crossref] [PubMed]
- Li T, Yu T, Li L, et al. Use of diffusion kurtosis imaging and quantitative dynamic contrast-enhanced MRI for the differentiation of breast tumors. J Magn Reson Imaging 2018;48:1358-66. [Crossref] [PubMed]
- Committee CA-CABCP. China Anti-Cancer Association Breast Cancer Treatment Guidelines and Regulations (2017 Edition) Chinese Journal of Cancer 2017;(9). Available online: https://www.cnki.net/kcms/doi/10.19401/j.cnki.1007-3639.2017.09.004.html
- Gao JJ, Swain SM, Luminal A. Breast Cancer and Molecular Assays: A Review. Oncologist 2018;23:556-65. [Crossref] [PubMed]
- Laurberg T, Tramm T, Nielsen T, et al. Intrinsic subtypes and benefit from postmastectomy radiotherapy in node-positive premenopausal breast cancer patients who received adjuvant chemotherapy - results from two independent randomized trials. Acta Oncol 2018;57:38-43. [Crossref] [PubMed]
- Hatt M, Tixier F, Pierce L, et al. Characterization of PET/CT images using texture analysis: the past, the present… any future? Eur J Nucl Med Mol Imaging 2017;44:151-65. [Crossref] [PubMed]
- Hempel JM, Schittenhelm J, Bisdas S, et al. In vivo assessment of tumor heterogeneity in WHO 2016 glioma grades using diffusion kurtosis imaging: Diagnostic performance and improvement of feasibility in routine clinical practice. J Neuroradiol 2018;45:32-40. [Crossref] [PubMed]
- Ribeiro VJ, Zhang ZL, Moon S, et al. Small-time scaling behavior of Internet backbone traffic ☆. Computer Networks 2005;48:315-34. [Crossref]
- Sanga S, Broom BM, Cristini V, et al. Gene expression meta-analysis supports existence of molecular apocrine breast cancer with a role for androgen receptor and implies interactions with ErbB family. BMC Med Genomics 2009;2:59. [Crossref] [PubMed]
- Sun K, Chen X, Chai W, et al. Breast Cancer: Diffusion Kurtosis MR Imaging-Diagnostic Accuracy and Correlation with Clinical-Pathologic Factors. Radiology 2015;277:46-55. [Crossref] [PubMed]
- Huang Y, Lin Y, Hu W, et al. Diffusion Kurtosis at 3.0T as an in vivo Imaging Marker for Breast Cancer Characterization: Correlation With Prognostic Factors. J Magn Reson Imaging 2019;49:845-56. [Crossref] [PubMed]
- Subramanian L, Dixit VU. Tests for the skewness parameter of two-piece double exponential distribution in the presence of nuisance parameters. Statistics 2018;52:115-32. [Crossref]
- Khorasani M, Amigo JM, Sun CC, et al. Near-infrared chemical imaging (NIR-CI) as a process monitoring solution for a production line of roll compaction and tableting. Eur J Pharm Biopharm 2015;93:293-302. [Crossref] [PubMed]
- Holli-Helenius K, Salminen A, Rinta-Kiikka I, et al. MRI texture analysis in differentiating luminal A and luminal B breast cancer molecular subtypes - a feasibility study. BMC Med Imaging 2017;17:69. [Crossref] [PubMed]
- Lei J, Yang P, Zhang L, et al. Reply to Letter to the Editor re: Diagnostic accuracy of digital breast tomosynthesis versus digital mammography for benign and malignant lesions in breasts: a meta-analysis. Eur Radiol 2014;24:928-9. [Crossref] [PubMed]