Diagnostic value of an automated breast volume scanner compared with a hand-held ultrasound: a meta-analysis
Introduction
Breast cancer is reported to be the most common cancer in women, with more than two million new cases and more than 600,000 cancer-related deaths worldwide each year. Annually, in the United States alone, more than 200,000 new cases are identified and more than 40,000 deaths occur (1-3). Early detection and early treatment through screening are the most important means to improve the survival rate of patients with breast cancer.
Mammograms are the most commonly used screening method for breast cancer in Western countries. However, in dense breast tissue, diagnostic sensitivity is reduced by approximately 50% (4,5). In addition, there is a 40–60% increase in the risk of breast cancer in women with dense breasts (4,6). In women aged <40 years and in women of Asian ethnicity, who tend to have small and dense breasts, screening mammography (MG) is not sufficiently effective.
Since ultrasound is non-radioactive and sensitive for differentiating fat and gland tissue echo, as well as it is good at characterizing lesion’s morphology and boundary, it has clear advantages for diagnosing breast cancer and can be used as a beneficial complement to diagnostic mammograms (7-9). However, because of the size limitation of the conventional ultrasonic probe, local omission tends to occur. For conventional ultrasound, namely, hand-held ultrasound (HHUS), whether a lesion is discovered can be dependent on the experience of an operator, and repeatability and standardization both need to be improved. The automated breast volume scanner (ABVS) was introduced in 2009, and is less time-consuming than manual ultrasound as the breast is scanned automatically. ABVS can retain full volume information of the breast and reconstruct any planar image, and can also objectively and reproducibly show information concerning the whole breast (10-12). Therefore, it may be a helpful tool for screening and diagnosis of breast cancer. With the increasing use of ABVS in recent years, available data on this technology have also increased, allowing for an evaluation of ABVS alongside gold standard pathological data, which moves beyond previous studies that have relied on magnetic resonance (MR) or MG as gold standards. With more data available, we conducted a meta-analysis on the diagnostic performance of ABVS and HHUS in relation to pathological gold standard data. Using stringent inclusion criteria to include studies with the highest homogeneity, this meta-analysis aimed to obtain reliable up-to-date results.
Methods
Literature search
On November 14, 2018, we undertook a literature search of articles using PubMed, EMBASE, Cochrane, and SinoMed databases without language restrictions, that included the terms ‘automated breast volume scanner’ and ‘breast cancer’. To avoid missing potentially relevant articles due to a narrow search strategy, we broadened the search scope to include as many relevant papers as possible. Specifically, we included all papers that referred to an automated breast volume scanner (including its synonyms, such as automated breast volume ultrasound*, automated breast volume image*, automated breast volume sonography, automated whole breast volume scan*, automated breast volume ultrasonography, automated breast ultrasound*, automated whole breast ultrasound*, etc.) and breast cancer (including its synonyms, such as breast neoplasm*, breast tumor*, breast carcinoma*, breast lesion*, and breast mass*) in either the title or the abstract. We combined articles detected across different databases and then removed the duplicates.
Inclusion and exclusion criteria
Two researchers independently screened the titles and the abstracts of the relevant literature, and reviewed the full texts to determine which articles were to be included in the analysis. Any disagreements between the two researchers were resolved through consultation.
The inclusion criteria were as follows: (I) ABVS and HHUS examination and Breast Imaging Reporting and Data Systems (BI-RADS) classification were performed for the same population; (II) pathological results were used as the gold standard (a small number of patients with benign ABVS and HHUS imaging findings were followed for at least 1 year, and the lesions without changes were also considered pathologically benign); (III) the data provided in the articles were sufficient to calculate the diagnostic accuracy or detection rate; and (IV) BI-RADS 4–5 were considered as positive.
The exclusion criteria were as follows: (I) review articles, meta-analyses, conference abstracts, and animal experiments; (II) articles in which a pathological examination had not been conducted or pathological data were unavailable; (III) using results (such as MR, MG, or other imaging findings) other than pathological data as the gold standard; (IV) ABVS/HHUS data were combined with those from other methods (such as MR and MG) instead of being reported separately; and (V) BR-AIDS 3–5 or BR-AIDS 4b–5 were considered as positive. (VI) Language is not set as an exclusion criterion, but restricted by our language ability, search was not conducted in other languages websites (such as Japanese, Latin, and so on).
Data extraction and assessment of document quality
Two investigators independently extracted the following data from the literature: first author, publication year, study country, category of the patient included, number of patients, number of lesions, patient age and sex, reference standard, ABVS model, HHUS model, and ABVS and HHUS diagnostic performance data (including true positive, false positive, false negative, true negative, and malignant tumor detection rates).
QUADAS-2, a revised tool for the quality assessment of diagnostic accuracy, was applied to assess the risk of publication bias included in this study, which was undertaken using Review Manager 5.2 software.
Statistical analysis
Diagnostic accuracy and detection rate analyses were conducted in this study. For diagnostic accuracy analysis, measures of interest included sensitivity, specificity, diagnostic odds ratios (DORs), area under the curve (AUC), and Q* index. The closer the AUC is to 1.0, the better the diagnostic method. The Q* index is a statistical value defined as the point on the summary receiver operating characteristic (SROC) curve where sensitivity and specificity are equal. Statistical heterogeneity among studies was evaluated using Cochran’s Q statistic, P values, and I2 statistics. Heterogeneity was considered significant at I2 >50%. A fixed effects model was used to calculate the pooled detection rate, sensitivity, specificity, and the DOR if I2 was <50%, and a random-effect model was used if I2 was >50%. The AUC and the Q* index were subsequently produced from the SROC curve of all the included studies. These analyses were conducted using MetaDisc 1.4 software. A comparison of diagnostic accuracy between ABVS and HHUS was determined using meta-regression analysis, and a corresponding SROC curve displaying both ABVS and HHUS was generated using Review Manager 5.2 software. Publication bias was examined with a Deek’s funnel plot asymmetry test using State 12.0 software.
Concerning detection rate analysis, the detection rate refers to the percentage of malignant lesions being detected. The pooled detection rate and the funnel plot evaluating publication bias were generated using Rx64 3.3.3 for Windows.
Results
We retrieved 256, 229, 19, and 100 articles from the PubMed, EMBASE, Cochrane, and SinoMed databases, respectively, and included 5 articles from other sources. After combining the articles and removing the duplicates, we obtained 404 articles (304 in English, 100 in Chinese). We then screened titles and abstracts to exclude 322 articles that did not meet the inclusion criteria or that met the exclusion criteria. We completely evaluated the remaining 82 articles, among which 17 (12 in English, 5 in Chinese) (13-29) were selected for diagnostic accuracy analysis and 13 (11 in English, 2 in Chinese) (22-34) were selected for detection rate analysis (Figure 1). Because 8 articles were used for both diagnostic accuracy and detection rate, a total of 22 articles (13-34) were included in this study (Table 1).
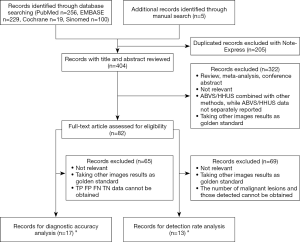
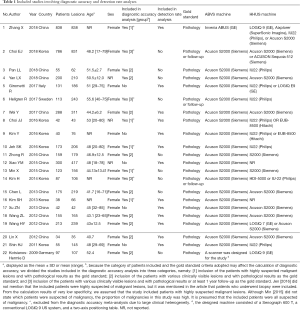
Full table
Diagnostic accuracy analysis
A meta-analysis of the 17 studies selected was performed for assessment of diagnostic accuracy, comprising 3,576 patients and 4,016 lesions. No threshold effect was detected. The pooled sensitivity was 0.91 (95% CI, 0.90–0.93) for ABVS and 0.91 (95% CI, 0.90–0.93) for HHUS, and the specificity was 0.82 (95% CI, 0.81–0.84) for ABVS and 0.78 (95% CI, 0.77–0.80) for HHUS. The heterogeneity was not acceptable: I2 was 48.0% and 76.0% for the pooled sensitivity of ABVS and HHUS, respectively; and I2 was 76.8% and 92.8% for the pooled specificity of ABVS and HHUS, respectively. The pooled DOR was 56.59 (95% CI, 39.20–81.70) for ABVS and 41.21 (95% CI, 28.09–60.47) for HHUS. The heterogeneity was not acceptable: I2 was 56.4% and 60.5% for the pooled DOR of ABVS and HHUS, respectively (Figure 2). The AUC of the SROC was 0.9426 for ABVS and 0.9269 for HHUS, and the Q* index was 0.8808 for ABVS and 0.8614 for HHUS (Supplement I).
Given that the heterogeneity of the above indicators was unacceptable, we attempted to identify the source of this heterogeneity from a clinical perspective. Inclusion of varying patient types is likely to affect the calculation of diagnostic accuracy; for example, including only patients with BI-RADS 4–5 may result in significantly lower specificity within the results. Moreover, the effect of equating long-term follow-up with pathological results on the calculation results cannot be determined. Based on these considerations, we divided the 17 studies included in the diagnostic accuracy analysis into 3 categories as follows: Category 1, inclusion of patients with highly suspected malignant lesions and with pathological results as the gold standard (5 studies); Category 2, inclusion of patients with various clinically visible lesions and with pathological results as the gold standard (9 studies); and Category 3, inclusion of patients with various clinically visible lesions and with pathological results or at least 1 year follow-up as the gold standard (3 studies; Table 1).
Excluding the Category 1 studies that most likely led to heterogeneity and Category 3 studies with an uncertain influence on the calculation results, we conducted a meta-analysis with the 9 studies in Category 2.
The QUADAS-2 tool was used to assess the quality of the included studies, and the results are shown in Figure 2.
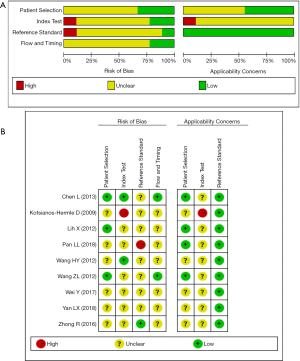
In total, 1,376 patients and 1,527 lesions from 9 studies were included in the final analysis. No threshold effect existed. The pooled sensitivity was 0.93 (95% CI, 0.91–0.95) for ABVS and 0.90 (95% CI, 0.88–0.92) for HHUS, and the pooled specificity was 0.86 (95% CI, 0.83–0.88) for ABVS and 0.82 (95% CI, 0.79–0.84) for HHUS. The heterogeneity was acceptable: I2 was 34.6% and 41.5% for the pooled sensitivity of ABVS and HHUS, respectively; and I2 was 48.9% and 49.0% for the pooled specificity of ABVS and HHUS, respectively (Figure 3). The pooled DOR was 88.66 (95% CI, 51.44–152.78) for ABVS and 41.06 for HHUS (95% CI, 26.58–63.42). The heterogeneity was acceptable: I2 was 44.6% and 38.0% for the pooled DOR of ABVS and HHUS, respectively (Figure 4). The AUC of the SROC was 0.9496 for ABVS and 0.9143 for HHUS, and the Q* index was 0.8899 for ABVS and 0.8469 for HHUS (Figure 5). Meta-regression showed no significant difference between the diagnostic accuracy of ABVS and HHUS (P=0.0771).
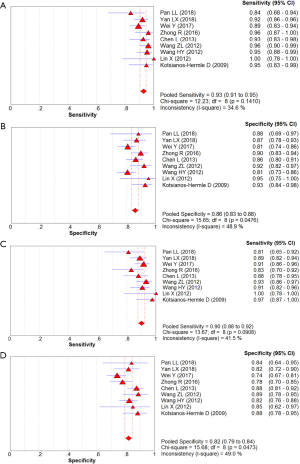
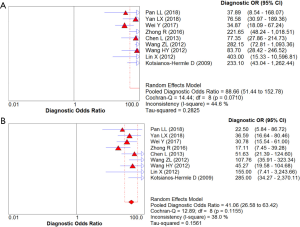
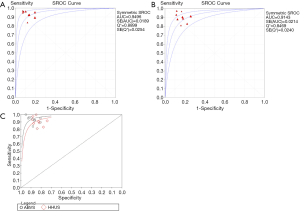
Deek’s funnel plot asymmetry test showed no publication bias for either ABVS or HHUS (Figure 6).
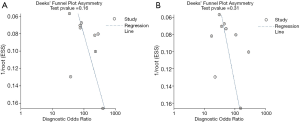
Detection rate analysis
We defined the detection rate as the proportion of pathologically confirmed malignant lesions that had been detected using imaging methods. Regardless of the BI-RADS classification of the imaging findings, as long as the lesion was found, it was considered to be detected. A total of 13 studies were included in the analysis, comprising 1,047 pathologically confirmed malignant lesions. The meta-analysis showed that the heterogeneity between the studies was low, so a fixed effects model was used. The pooled detection rate was 1.00 (95% CI, 1.00–1.00) for both ABVS and HHUS (Figure 7). Publication bias is shown using a funnel plot (Figure 8).
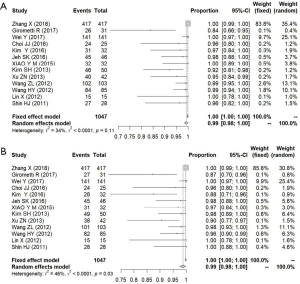
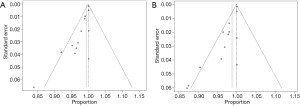
Discussion
Our meta-analysis found that ABVS had the same detection rate (100%) as HHUS in detecting breast cancer, and numerically higher sensitivity (93% vs. 90%) and specificity (86% vs. 82%) compared with HHUS. Meta-regression analysis showed no significant difference between the diagnostic accuracy of ABVS and HHUS (P=0.0771). The DOR was 88.66 for ABVS and 41.06 for HHUS. The AUC of the SROC was 0.9496 for ABVS and 0.9143 for HHUS, and the Q* index was 0.8899 for ABVS and 0.8469 for HHUS. These results indicated that ABVS can be considered an appropriate screening tool for breast cancer.
MG has long been the mainstay for breast cancer screening (35). However, for young or dense-breast women, the expanse of whiteness on the MG can mask a lesion (36). In these cases, ultrasound is a useful supplement (7-9). But the small probe of the HHUS may result in omission and poor repeatability, with high dependence on an examiner’s experience (29). ABVS combines Real-Time Spatial Compounding Imaging Technology and Dynamic Tissue Enhancement Technology to obtain the best image quality after optimization (37,38). In addition, full-volume data and three-dimensional reconstruction may provide whole breast image and make ABVS work better than HHUS in big-breast women. What’s more, ABVS is less time consuming, and the information is comprehensive and repeatable, which has contributed to its rise in popularity (39,40). A malignant lesion in ABVS is mainly identified through a unique convergence sign due owing to infiltration, traction, and deformation of surrounding tissues in a coronal section scan (Figure S1), which is not obtainable using conventional HHUS (25,29,38). Shortcomings of ABVS include no blood flow signal and no real-time probe direction adjustment for suspicious lesions, which need to be further improved in the future. However, for young or dense-breast women, screening using ABVS, combined with HHUS and further examination, may be an effective overall method of assessment at present.
We analyzed all the data since the introduction of ABVS in our meta-analysis and used strict inclusion criteria to eliminate unsuitable data, reduce bias, and enhance the reliability of the conclusions. We targeted diagnostic studies and required that an included study reported pathological results as gold standards; as a result, many studies could not be included because they failed to satisfy these conditions. There were also some studies that included patient data only when a lesion could be observed via HHUS, resulting in a bias toward the benefits of HHUS; therefore, those studies were not included. One meta-analysis on the diagnostic accuracy of ABVS was conducted 5 years previously (41), but it had two limitations. First, the admission criteria were not strictly defined, resulting in increased heterogeneity and reduced credibility concerning the conclusions. The I2 value was as high as 60–70%. For example, in Shin’s study, not all diagnoses relied on pathological results. Rather, some studies were based on HHUS and MG to differentiate benign from malignant tissue, which have been reported to be subject to false negative and false positive results (34). In Kim’s study [2014], some of the lesions were subsequently assessed as being benign after a short-term follow-up, which increased the false negative rate (19). Most of the patients included in a 2019 study by Kim were breast cancer patients, which led to an increase in the specificity and false positive rate (12). We excluded these articles from our analysis. After strict control, the I2 values were within an acceptable range of 30–40%. Second, the published data initially available for evaluating the diagnostic value of ABVS alone were limited. Some of the studies included in Kim’s 2019 study considered ABVS as the only examination method rather than ABVS and HHUS concurrently. ABVS and HHUS were only compared in subgroup analyses that had small numbers of patients in appropriate groups and did not meet acceptable randomized controlled trial criteria. After strict control, we included 1,376 patients and 1,527 lesions, which could address this issue more effectively. Our results showed that the sensitivity and specificity of ABVS were better than HHUS (sensitivity, 0.93 vs. 0.90; specificity, 0.86 vs. 0.82). Although no statistically significant differences were observed in our comparison; nonetheless, we identified a differing trend from that observed in a 2015 study (41) (sensitivity, 0.931 vs. 0.931; specificity, 0.856 vs. 0.866). One study, published in 2018, compared the diagnostic value of 19 imaging methods for the diagnosis of breast cancer with a network meta-analysis, involving both ABVS and HHUS. However, it is difficult to assess the advantages or disadvantages of ABVS and HHUS based on the results of that study, due to two primary limitations. First, there was no direct comparison between ABVS and HHUS; instead both ABVS and HHUS were compared with MG. Second, only one study was included when comparing ABVS and MG and when comparing HHUS and MG, which cannot be considered a meta-analysis, and results in bias (42).
Considering that the purpose of ultrasonography is to detect breast cancer early, we defined the detection rate as the proportion of pathologically confirmed malignant lesions detected using imaging methods, regardless of the BI-RADS classification of the findings. Among 1,047 malignant lesions, 1,030 were detected using ABVS and 1,026 using HHUS. There was no statistically significant difference in the detection rate between ABVS and HHUS. However, the ABVS detected 4 more cancerous lesions than HHUS. This result was consistent with the operational parameters of the two methods, as ABVS is a full-volume automatic scanning system with a large probe covering the entire breast, which may prevent lesion detection failure that could occur with HHUS due to manual inspection using a small probe in some circumstances. Furthermore, ABVS is likely to become more effective as ultrasonographers develop the skills to interpret the ABVS image more accurately in future.
The main role of ABVS is to ensure effective screening. For patients identified with BI-RADS grade 4a disease classification following ultrasound examination, the possibility of grade 4a malignancy is relatively low, between 2% and 10% (43,44). In the selected articles, grade 4a lesion images were interpreted as malignant, which increased sensitivity of the imaging examination but also led to a decrease in specificity and an increase in the false-positive rate. However, given the critical diagnostic role of imaging screening, it is best not to miss a diagnosis. We considered it acceptable to determine grade 4a images as malignant, and also to be in line with the original intentions of the BI-RADS classification and screening system. In this respect, the sensitivity of ABVS to grade 4a is well recognized. ABVS was found to have a sensitivity of 93% and a specificity of 86%, which were higher than the sensitivity (90%) and specificity (82%) found for HHUS; therefore, it can be considered a suitable ultrasound technology for application.
This meta-analysis had some limitations. There was publication bias in terms of the detection rate analysis, but not in terms of the diagnostic accuracy analysis. Positive results are more likely to be published than negative results and some data, such as summaries of meetings, were not included. We only evaluated articles written in English and Chinese, which may have led to publication bias.
In summary, we reviewed all English and Chinese literature published since the emergence of ABVS 10 years previously for our meta-analysis. Our results showed that the malignant mass detection rate, and the sensitivity and specificity of ABVS were better than those of HHUS, but these differences were not statistically significant. Therefore, we consider that ABVS can be used as an appropriate screening tool for breast cancer as well as HHUS in diagnostic accuracy and detection rate. Considering other advantages of ABVS including non-radioactivity, sensitivity to dense breast, three-dimensional reconstruction, time-saving and repeatability, it might be a promising screening tool for young or dense-breast women in the future.
Supplementary
Supplement I Meta-analysis for the 17 studies included in the diagnostic accuracy analysis
Altogether, 3,576 patients and 4,016 lesions from 17 studies were included. No threshold effect existed. The pooled sensitivity was 0.91 (95% CI, 0.90–0.93) for ABVS and 0.91 (95% CI, 0.90–0.93) for HHUS, and the specificity was 0.82 (95% CI, 0.81–0.84) for ABVS and 0.78 (95% CI, 0.77–0.80) for HHUS. The heterogeneity was not acceptable: I2 was 48.0% and 76.0% for the pooled sensitivity of ABVS and HHUS, respectively; and I2 was 76.8% and 92.8% for the pooled specificity of ABVS and HHUS, respectively (Figure S1). The pooled DOR was 56.59 (95% CI, 39.20–81.70) for ABVS and 41.21 (95% CI, 28.09–60.47). The heterogeneity was not acceptable: I2 was 56.4% and 60.5% for the pooled DOR of ABVS and HHUS, respectively (Figure S2). The AUC of the SROC was 0.9426 for ABVS and 0.9269 for HHUS, and the Q* index and 0.8808 for ABVS and 0.8614 for HHUS (Figure S3). Mete-regression showed no significant difference between the diagnostic accuracy of ABVS and that of HHUS (P=0.0771).
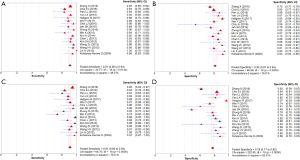
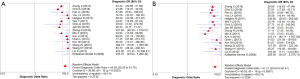
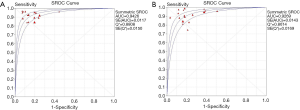
Acknowledgments
Funding: The research was supported by Chinese Academy of Medical Science Initiative for Innovative Medicine (2017-I2M-2-003) and National Key Research and Development Program (2016YFC0104805).
Footnote
Conflicts of Interest: The authors have no conflicts of interest to declare.
Ethical Statement: The authors are accountable for all aspects of the work in ensuring that questions related to the accuracy or integrity of any part of the work are appropriately investigated and resolved.
References
- Samadi P, Saki S, Dermani FK, et al. Emerging ways to treat breast cancer: will promises be met? Cell Oncol (Dordr) 2018;41:605-21. [Crossref] [PubMed]
- Bray F, Ferlay J, Soerjomataram I, et al. Global cancer statistics 2018: GLOBOCAN estimates of incidence and mortality worldwide for 36 cancers in 185 countries. CA Cancer J Clin 2018;68:394-424. [Crossref] [PubMed]
- Siegel RL, Miller KD, Jemal A. Cancer statistics, 2018. CA Cancer J Clin 2018;68:7-30. [Crossref] [PubMed]
- Boyd NF, Guo H, Martin LJ, et al. Mammographic density and the risk and detection of breast cancer. N Engl J Med 2007;356:227-36. [Crossref] [PubMed]
- Kolb TM, Lichy J, Newhouse JH. Comparison of the performance of screening mammography, physical examination, and breast US and evaluation of factors that influence them: an analysis of 27,825 patient evaluations. Radiology 2002;225:165-75. [Crossref] [PubMed]
- McCormack VA, dos Santos Silva I. Breast density and parenchymal patterns as markers of breast cancer risk: a meta-analysis. Cancer Epidemiol Biomarkers Prev 2006;15:1159-69. [Crossref] [PubMed]
- Brem RF, Lenihan MJ, Lieberman J, et al. Screening breast ultrasound: past, present, and future. AJR Am J Roentgenol 2015;204:234-40. [Crossref] [PubMed]
- Burkett BJ, Hanemann CW. A review of supplemental screening ultrasound for breast cancer: certain populations of women with dense breast tissue may benefit. Acad Radiol 2016;23:1604-9. [Crossref] [PubMed]
- Rebolj M, Assi V, Brentnall A, et al. Addition of ultrasound to mammography in the case of dense breast tissue: systematic review and meta-analysis. Br J Cancer 2018;118:1559-70. [Crossref] [PubMed]
- Xiao Y, Wang Z, Chen Z. Automated breast volume scanner (ABVS) in breast cancer - a review. Eur J Gynaecol Oncol 2017;38:184-6. [PubMed]
- Girometti R, Zanotel M, Londero V, et al. Automated breast volume scanner (ABVS) in assessing breast cancer size: a comparison with conventional ultrasound and magnetic resonance imaging. Eur Radiol 2018;28:1000-8. [Crossref] [PubMed]
- Kim SH. Image quality and artifacts in automated breast ultrasonography. Ultrasonography 2019;38:83-91. [Crossref] [PubMed]
- Pan LL, Zhang XH, Li XK. The value of multimodal ultrasound in differentiating benign and malignant breast tumors. Chin J Gerontol 2018;38:64-6.
- Yan LX, Huang BJ, Liu LM, et al. Comparative study of handheld ultrasound, automated breast volume scanner and breast specific gamma imaging in the diagnosis of breast cancer. Chin J Ultrasonogr 2018;27:323.
- Choi EJ, Choi H, Park EH, et al. Evaluation of an automated breast volume scanner according to the fifth edition of BI-RADS for breast ultrasound compared with hand-held ultrasound. Eur J Radiol 2018;99:138-45.
- Min X, Cheng SJ, Liu HZ, et al. Clinical value of conventional handheld ultrasonography combined with automated breast volume scanning in the diagnosis of benign and malignant breast lesion. Chin J Wom & Chi Heal Res 2015;26:1280-3.
- Zhong R, Lv GR, Shen HL, et al. The comparison of automatic breast volume scanner and 2DUS in BI-RADS classification. Chin J Ultraso Med 2016;32:121-3.
- Hellgren R, Dickman P, Leifland K, et al. Comparison of handheld ultrasound and automated breast ultrasound in women recalled after mammography screening. Acta Radiologica 2017;58:515-20. [Crossref] [PubMed]
- Kim H, Cha JH, Oh HY, et al. Comparison of conventional and automated breast volume ultrasound in the description and characterization of solid breast masses based on BI-RADS features. Breast Cancer 2014;21:423-8. [Crossref] [PubMed]
- Chen L, Chen Y, Diao XH, et al. Comparative study of automated breast 3-D ultrasound and handheld B-mode ultrasound for differentiation of benign and malignant breast masses. Ultrasound Med Biol 2013;39:1735-42. [Crossref] [PubMed]
- Kotsianos-Hermle D, Wirth S, Fischer T, et al. Analysis of 107 breast lesions with automated 3D ultrasound and comparison with mammography and manual ultrasound. Eur J Radiol 2009;71:109-15. [Crossref] [PubMed]
- Zhang X, Lin X, Tan Y, et al. A multicenter hospital-based diagnosis study of automated breast ultrasound system in detecting breast cancer among Chinese women. Chin J Cancer Res 2018;30:231-9. [Crossref] [PubMed]
- Wei Y, Jiang YX, Zhang J, et al. Diagnostic value of automated breast volume scanner in breast lesion. Chin J Health Manage 2017;11:514.
- Choi JJ, Kim SH, Kang BJ, et al. Detectability and usefulness of automated whole breast ultrasound in patients with suspicious microcalcifications on mammography: comparison with handheld breast ultrasound. J Breast Cancer 2016;19:429-37. [Crossref] [PubMed]
- Jeh SK, Kim SH, Choi JJ, et al. Comparison of automated breast ultrasonography to handheld ultrasonography in detecting and diagnosing breast lesions. Acta Radiologica 2016;57:162. [Crossref] [PubMed]
- Kim SH, Kang BJ, Choi BG, et al. Radiologists' performance for detecting lesions and the interobserver variability of automated whole breast ultrasound. Korean J Radiol 2013;14:154-63. [Crossref] [PubMed]
- Wang ZL, Xu JH, Li JL, et al. Comparison of automated breast volume scanning to hand-held ultrasound and mammography. Radiol Med. 2012;117:1287-93. [Crossref] [PubMed]
- Wang HY, Jiang YX, Zhu QL, et al. Differentiation of benign and malignant breast lesions: a comparison between automatically generated breast volume scans and handheld ultrasound examinations. Eur J Radiol 2012;81:3190-200. [Crossref] [PubMed]
- Lin X, Wang J, Han F, et al. Analysis of eighty-one cases with breast lesions using automated breast volume scanner and comparison with handheld ultrasound. Eur J Radiol 2012;81:873-8. [Crossref] [PubMed]
- Girometti R, Zanotel M, Londero V, et al. Comparison between automated breast volume scanner (ABVS) versus hand-held ultrasound as a second look procedure after magnetic resonance imaging. Eur Radiol 2017;27:3767-75. [Crossref] [PubMed]
- Xu ZN, Tu ML, Wang YL, et al. Comparison between automated breast volume scanning and conventional two-dimensional ultrasound in the diagnosis of non-invasive ductal carcinoma. Diagn Interv Imaging 2013;22:331-5.
- Kim Y, Kang BJ, Kim SH, et al. Prospective study comparing two second-look ultrasound techniques: handheld ultrasound and an automated breast volume scanner. J Ultrasound Med 2016;35:2103-12. [Crossref] [PubMed]
- Xiao YM, Chen ZH, Zhou QC, et al. The efficacy of automated breast volume scanning over conventional ultrasonography among patients with breast lesions. Int J Gynaecol Obstet 2015;131:293-6. [Crossref] [PubMed]
- Shin HJ, Kim HH, Cha JH, et al. Automated ultrasound of the breast for diagnosis: interobserver agreement on lesion detection and characterization. AJR Am J Roentgenol 2011;197:747-54. [Crossref] [PubMed]
- Tabár L, Vitak B, Chen TH, et al. Swedish two-county trial: impact of mammographic screening on breast cancer mortality during 3 decades. Radiology 2011;260:658-63. [Crossref] [PubMed]
- Assi V, Warwick J, Cuzick J, et al. Clinical and epidemiological issues in mammographic density. Nat Rev Clin Oncol 2011;9:33-40. [Crossref] [PubMed]
- Wojcinski S, Farrokh A, Hille U, et al. The automated breast volume scanner (ABVS): initial experiences in lesion detection compared with conventional handheld B-mode ultrasound: a pilot study of 50 cases. Int J Womens Health 2011;3:337-46. [Crossref] [PubMed]
- Kaplan SS. Automated whole breast ultrasound. Radiol Clin North Am 2014;52:539-46. [Crossref] [PubMed]
- Brem RF, Tabár L, Duffy SW, et al. Assessing improvement in detection of breast cancer with three-dimensional automated breast US in women with dense breast tissue: the SomoInsight Study. Radiology 2015;274:663-73. [Crossref] [PubMed]
- Huang CS, Yang YW, Chen RT, et al. Whole-breast ultrasound for breast screening and archiving. Ultrasound Med Biol 2017;43:926-33. [Crossref] [PubMed]
- Meng Z, Chen C, Zhu Y, et al. Diagnostic performance of the automated breast volume scanner: a systematic review of inter-rater reliability/agreement and meta-analysis of diagnostic accuracy for differentiating benign and malignant breast lesions. Eur Radiol 2015;25:3638-47. [Crossref] [PubMed]
- Zhang XH, Xiao C. Diagnostic value of nineteen different imaging methods for patients with breast cancer: a network meta-analysis. Cell Physiol Biochem 2018;46:2041-55. [Crossref] [PubMed]
- Lee J. Practical and illustrated summary of updated BI-RADS for ultrasonography. Ultrasonography 2017;36:71-81. [Crossref] [PubMed]
- Rao AA, Feneis J, Lalonde C, et al. A pictorial review of changes in the BI-RADS Fifth Edition. Radiographics 2016;36:623-39.