A high burden of comorbid conditions leads to decreased survival in breast cancer
Introduction
Breast cancer is the most common cancer in women with 255,180 new diagnoses and 41,070 deaths in the United States in 2017 (1). Together with the large number of patients affected and the growing demographic of patients over 65 years of age, physicians are increasingly being asked to assess and evaluate the best treatment for cancer in the setting of a complex set of chronic conditions (2,3). However, they are limited by our understanding of the specific impact these chronic conditions has on the overall and disease free survival of breast cancer. The aim of this study was to characterize the various significant health comorbidities of our patient population and to determine if the cumulative number of those comorbidities is a significant contributor to the disease free and overall survival of these patients.
As surgeons, understanding the comorbidity profile of the patient is important due to the risk of complications following surgical intervention. Each comorbid condition accounts for a significantly increased risk of a range of complications including wound complications with diabetes, myocardial infarction with bleeding disorders or prior stroke and pneumonia with chronic obstructive pulmonary disease (COPD) (4). Even post-operative urinary tract infections have been shown to be associated with bleeding disorders, hypertension and steroid use (4).
Many risk calculators are in use to estimate the likelihood of post-operative complications. These include Goldman’s Revised Cardiac Risk Index and ACS NSQUIP Risk Calculator (5,6). These are effective tools that have been verified across large cohorts of patients but are limited by the short timeframe in which they can make predications. For non-surgical patients the Charlson Comorbidity Index (CCI) has been verified for longer-term outcomes in patients up to 1 year (7). These timeframes are relatively limited since 30 days to one year is a short timeframe in which to assess outcomes when considering a multitude of chronic conditions over a lifetime.
Additionally, the patient assessment becomes even more complicated by the risks associated with cytotoxic chemotherapy and radiotherapy. Limitations on the medical treatment can often be imposed by the patient’s comorbidities, which then has direct implications for the surgical planning. For example, the CDC reports that almost one third of all American adults have been diagnosed with hypertension (8). If a patient with a long history of hypertension now presents with breast cancer and cannot tolerate her neoadjuvant chemotherapy due to the previous damage her heart has sustained that will have a negative consequence on the treatment plan overall (9,10). Without that critical reduction in tumor burden the surgery may have to be drastically altered and could result in far greater morbidity and decreased survival. Also, adjuvant radiation can have detrimental effects on heart function (10). While the absence of adjuvant radiation following surgical removal may not impact the initial surgery it will likely impact her individual chance of the cancer returning (11). The challenge of the modern patient often lies in their inability to receive the full benefit of our treatments due to their significant comorbidity and fragile medical state.
Furthermore, while heart disease and obesity affect all people from all segments of society it cannot be ignored that they disproportionally affect those of African American descent (3). Especially concerning is the fact that while the incidence rate of breast cancer is similar for white and black females in the US between the years 1999–2013 the mortality among black females continues to be higher than for white females (12). Our society is becoming increasingly diverse (3) and we have a duty to ensure that our treatments are implemented fairly and are equally effective. Our institution is positioned to analyze a significant proportion of African American patients due to our location in Richmond, VA. This provides a unique insight into the disease profile and survival of this group.
Methods
Patient selection
The Virginia Commonwealth University Institutional Review Board approved this study (HM20000345). Patients were requested to be a part of the study at their visit to the Breast Clinic in the Division of Surgical Oncology at VCU Medical Center at which point their consent was attained and their Medical Record Numbers included in the study. Patients were selected without regard to previous disease; age or suspected pathology but all did have a surgical intervention. Additionally, patients were included without regard to the surgeon of record or oncologist directing treatment. Study outcomes did not nor will not impact future management of these patients.
Data collection
A database was created in Microsoft Access to include study participants from the year 2002 to 2012. Significant health co-morbidities including individual behavioral and biological determinants of health were gathered from the electronic medical record. A chart reviewer manually abstracted the data from history and physicals surrounding the time of diagnosis. The medical history sections were reviewed to detect tobacco use, pulmonary disease, obesity, hypertension, hyperlipidemia, diabetes, coronary artery disease, myocardial infarction, endocrine dysfunction, mental illness, chronic infectious processes, drug use, neurologic dysfunction and functional status.
Statistical analysis
The demographic information including the age, average BMI, male to female ratio and ethnicity data was calculated for our sample. Additionally the proportions of pathologic diagnoses including ER, PR, HER2 status were calculated.
Each patient’s comorbidities were categorized and summed to classify patients into groups according to the number of comorbidities. The CCI score was also calculated for each patient concurrently. Disease free survival for each patient was determined by calculating the time elapsed between the date of index surgery and either the patient’s date of relapse or the most recent follow-up without evidence of disease. Overall survival was calculated according to the time elapsed from pathologic diagnosis to death or last known follow-up.
A one-way analysis of variance was then performed to compare the disease free survival and the overall survival to the number of comorbidities each patient carried. Post hoc analysis was calculated using the Dunn’s test. The statistical analysis was carried out in Microsoft Excel with the addition of the XLSTAT software package. P values were set at 0.05 and the standard error was reported when applicable. Of note, for the overall survival analysis 3 data points were missing for which the mean of the entire data set was used. For disease free survival analysis 5 data points were missing for which the mean of the entire data set was used.
Additionally, the difference in disease free survival and overall survival was calculated between the African American population and the Caucasians. Differences were examined with a two-tailed unpaired t-test. Similarly the number of comorbidities and CCI was compared between the African American population and the Caucasians with a two-tailed unpaired t-test. P values were set at 0.05 and the confidence intervals depict standard error of the mean.
Results
There is significant ethnic diversity in the patient sample with half of patients being of African American heritage
A total of 279 patients were included in the analysis. These patients included those with a previous history of breast carcinoma, men, as well as premenopausal and postmenopausal women. This was representative of the demographics of patients treated at Virginia Commonwealth University Medical Center during the years 2002 to 2012. Table 1 depicts the demographic information for our patient sample. Of these 275 (98.6%) were female with only 4 (1.4%) being male. The average age of the patients at diagnosis was 56.1 years with a range of age 25–93. The average BMI was 30.4 with a range of 16.6 to 64. The major ethnicities were African American with 136 (48.7%) and Caucasian, 134 (48.0%). The other ethnicities included Greek, Hawaiian and Puerto Rican. There were three patients of Asian ancestry and two of Hispanic ancestry.
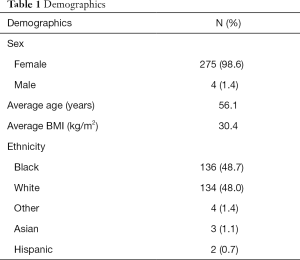
Full table
Pathological characteristics align with previously established population studies
Table 2 outlines the pathologic characterization of patient’s disease. A total of 80.6% of cases were determined to be interductal carcinoma (IDC) according to the pathology report while only 20 cases (7.2%) of cases were interlobular carcinoma (ILC). There were 4 (1.4%) of cases upon which pathologic examination revealed the coexistence of both IDC and ILC. Isolated DCIS was determined to be present in only 10 (3.6%) of final pathologic examination. Papillary, Phyllodes, mucinous, spindle cell, angiosarcoma, granular cell tumor, Paget’s and complex fibroadenomas made up a small minority of cases. The average tumor size upon index operation was 2.8 cm with a range of 0.2 up to 18 cm.
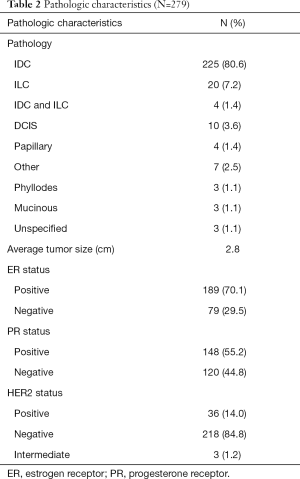
Full table
The receptors expression of these patients was also analyzed. One hundred and eighty-nine patients (70.1%) were characterized as estrogen receptor positive and slightly less 148 (55.2%) were characterized with positive Progesterone receptor expression. The HER2 positive patients only numbered 36 (14.0%). Three (1.2%) patients were characterized as intermediate. It is important to note that while pathologic characterization and receptor status for each patient was sought in the electronic medical record it was not universally available and so there was some missing data in regard to those analyses.
The average patient has two comorbidities and the most common medical comorbidities were hypertension and obesity
Each chronic condition was then assessed for frequency. We found that hypertension with 120 patients (43.0%) and obesity with 117 patients (41.9%) had the highest incidence in our population (Figure 1). This was followed by mental health disorders with 60 patients (21.5%). Depression was the most common and frequently occurred with anxiety, and chronic pain conditions such as fibromyalgia. Ten people also suffered migraines. Two patients were noted to have a history of schizophrenia and one with bipolar. An additional two patients carried the diagnosis of dementia. Tobacco use was noted among 55 patients (20.4%), which is slightly higher than the national average. In this sample any history of tobacco use was counted in our sample, which could contribute to the higher estimation.
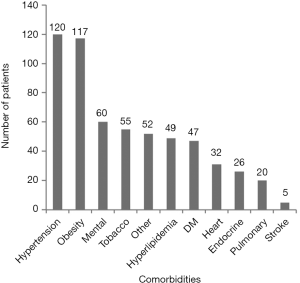
In Figure 2 we plotted the frequency of patients according to the number of comorbidities each patient carried. Patients range between zero to seven comorbidities while the average patient had 2.20 significant diagnoses. The majority (61, 22%) of patients had one comorbidity, which was closely followed by patients with zero comorbidity (57, 20%). The number of patients in each group steadily decreased with each additional comorbidity, so only 4% of patients had greater than six comorbidities.
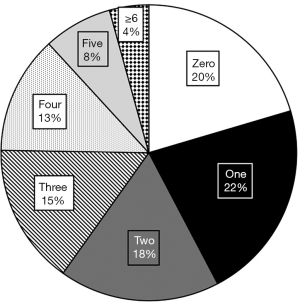
Six comorbidities was the threshold for which decreased overall and disease free survival was to be expected
An unbalanced one-way analysis of variance was calculated to determine if there were statistically significant differences among the means of disease free survival among the comorbidity groups. The average disease free survival was 1,560 days with a range of 0 to 4,424 days. Disease free survival did show a statistically significant difference with 6 degrees of freedom and P=0.010. Upon further analysis with the Dunn test there were significant differences between groups zero and six (P=0.036), one and six (P=0.025) and three and six (P=0.017). Figure 3A demonstrates the means with the error bars showing the standard error. No other significant differences were detected between the groups so we hypothesize that there is a threshold at six comorbidities over which survival decreases.
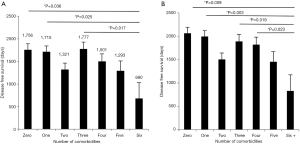
The same analysis was calculated to determine if there were statistically significant differences in overall survival. The average overall survival was 1,792 days with a range of 14 to 4,732 days. Overall survival did show a statistically significant difference with 6 degrees of freedom and a P<0.001. Additionally, when the Dunn Test was run it showed a significant difference between the zero vs. six group (P=0.009), one vs. six group (P=0.003), three vs. six group (P=0.019) and four vs. six (P=0.023). This suggests that there is a threshold at six comorbidities, which would indicate there is a decreased survival. Figure 3B demonstrates the means of each group with error bars depicting the standard error.
Patients with over six comorbidities have a similar profile of disease as all patients
We then wanted to specifically analyze the group that has over six comorbidities to see if they have a higher prevalence of more severe disease. Figure 4 shows that similar to the overall group their comorbidities primarily consisted of hypertension and obesity. Diabetes also significantly contributed to this groups comorbidities as the third most prevalent comorbidity compared to the seventh most prevalent in the overall group. Tobacco use remained the fourth most prevalent tied with hyperlipidemia, which was similar to the overall group. Mental health diseases fell to eighth most prevalent in the high comorbidity group compared with third most prevalent in the entire cohort.
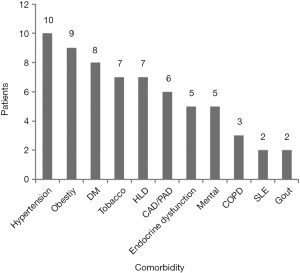
Although the average number of comorbidities trends upward with age there was no difference in the disease free survival or overall survival between age groups
Figure 5A depicts the average number of comorbidities according to age group. There is a gradual trend toward increasing number of comorbid conditions as age increases which is in accordance with clinical experience. Conversely, Figure 5B depicts the average age of patients in each comorbidity number group. A one-way analysis of variance detected a difference (P=0.004, 6 degrees of freedom) however a post hoc analysis (Dunn’s) only detected a significant difference between groups zero and four but no other transitive differences. Therefore, it is possible that though we hypothesized that those with 6 or more comorbidities would be significantly older, in fact, there is no significant difference in the average ages of these groups. This implies that a factor other than age is contributing to their decreased survival. This is further supported by Figure 5C and D which shows that there is not a significant difference in disease free survival and overall survival as age increases.
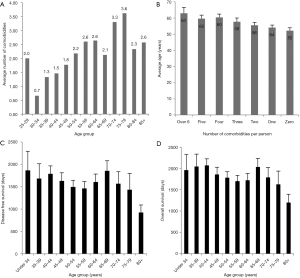
Our sample did not show a difference in overall or disease free survival between Caucasian and African American populations
Ethnicity did not make a significant difference in the disease free survival or the overall survival in our patient population, although there was a trend that African Americans have worse survival (Figure 6A). We did find that African Americans have significantly more comorbid conditions and have a higher CCI score than all others (Figure 6B). The average number of comorbid conditions was 2.61 for African Americans and 1.78 for Caucasians (P<0.005).
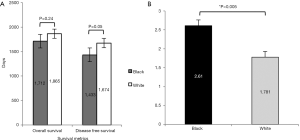
An increasing CCI did not show a decrease in overall or disease free survival
In Figure 7A we plotted the frequency of patients according to their CCI score. The average CCI was 2.51 with a range of scores between zero to seven which was similar to the previous index. However the distribution of scores was vastly different. The majority of patients had a score of two. This is likely explained by the structure of the index, which includes age as a scoring criterion. The average age of our patient population was 56.1, which accounts for two points added to the CCI.
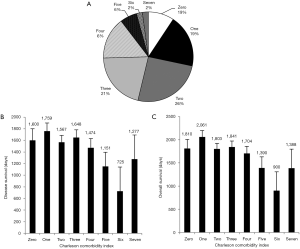
We next wanted to compare our standard of comorbidity accounting to a validated index used by other researchers. We chose the CCI. A one-way ANOVA was calculated for the overall survival of patients according to their CCI score. There was not found to be any significant increase or decrease in survival with an increasing CCI score. (dF =7, F stat =1.828, P=0.082). Similarly the disease free survival was analyzed without any significant finding (dF =7, F stat =1.437, P=0.190). Figure 7B and C depict the average survival metrics according to CCI score. Confidence intervals depict standard error of the mean.
Discussion
The aim of our study was to characterize the chronic conditions and to determine what effect these additional diseases have on their breast cancer prognosis. In our analysis of 279 breast cancer patients over the years 2002 to 2012 in Richmond, Virginia, we found that hypertension, obesity and mental health issues are the primary challenges facing our patients. The mean number of comorbidities patients present with is 2.2. We also found that a threshold of 6 appeared at which the cumulative effect of the comorbid conditions significantly impacts the overall survival and disease free survival of our patients. Because of the negative impact on disease free survival was demonstrated we hypothesize that not only are comorbidities decreasing patients survival they could also be worsening the biological nature of the cancer itself.
Advances in the care of chronic conditions such as diabetes, hypertension and CAD have increased the longevity of peoples’ lives leading to an average life expectancy of 78 years for all Americans (8). With increasing age comes increased likelihood of cancer in addition to a host of other chronic conditions. These chronic diseases require consistent monitoring from primary care providers and are expensive to treat. Treatment for Americans over 65 years old account for 66% of the countries health care budget (3). Furthermore, in our efforts to seek the best treatment for each individual patient it is important to take into consideration the patient’s overall condition when selecting the appropriate treatment regimen.
We first analyzed the dominant comorbidities in our sample. Our patient population had a high incidence of hypertension with 43.0% of our patients diagnosed. This is in line with reports from the CDC, which state that just over half of women between the ages of 55–64 carry that diagnosis (12). This is critically important because hypertension can ultimately lead to heart failure, which is only compounded by the use of anthracyclines, trastuzumab (9) and radiotherapy treatments often used as adjuvants (10). Chemotherapy induced left ventricular dysfunction leading to heart failure will be of increasing importance and consideration as the number of cancer survivors continues to increase in our society as more people successfully complete treatment and enter remission (10).
Obesity closely followed hypertension as the second most common comorbidity and is a well-known contributor to the development of postmenopausal breast cancer. Arnold et al. found that 481,000 (3.6%) of all cancers that developed in 1 year could be attributed to high BMI (13). In postmenopausal women adipose tissue is thought to be the greatest contributor of circulating estrogens, which drives the proliferation of Estrogen Receptor positive breast cancers (14,15). While obesity is harmful, physical activity has been shown to lead to a 34% reduced risk of development of breast cancer compared to women who did not take part in physical activity (16). And long-term weight gain from age 18 was positively associated with postmenopausal breast cancer (17). Furthermore breast cancer survivors have been shown to have significantly higher molecular markers for premature aging and inflammation, which can contribute to a significantly higher burden of disease (18). As a scientific community we know that not only will you be more likely to develop breast cancer but also even following survival, the effects linger.
The third comorbidity that our patients possessed was mental health diagnoses; with an incidence of 28.7%. This primarily included depression, anxiety, and chronic pain conditions but a minority of patients also had dementia, schizophrenia, bipolar, and substance abuse conditions. While our study estimates prevalence of a wide variety of mental health disorders upon diagnosis others have reported on the likelihood that depression will develop subsequent to diagnosis and what this implies for their clinical outcomes. Patients with no history of depression have an increased likelihood of mental health issues following the diagnosis of cancer (19). In a subsequent study published in 2017, Suppli et al. found that women previously treated for depression were more at risk (14%) of receiving treatment outside the guidelines and had significantly worse overall survival (20).
With the aging of our society we wanted to further tease out the impact that age had on survival as well (3). Heart disease and cancer continue to be the leading cause of death among those over 65 years of age (3,8). Are people with more comorbidity older and so die sooner? We saw that there is an overall tread to an increased number of comorbidities with increasing age however this maxes at an average of 3.6, which is far below the threshold of 6 at which we expect to see decreased survival (Figure 5A). Furthermore, when we calculated and compared the average ages of each group of comorbidities we saw that there was no significant difference in the age of those with over six comorbidities and those with zero. This provides further confirmation that the culprit for the decreased survival is the presence of the comorbidities and not simply advanced age (Figure 5B).
We also know that ethnicity can play a role in survival and our institution is perfectly positioned to analyze a significant proportion of African American patients. Many chronic diseases, such as heart disease and diabetes, disproportionally affect those of African American descent (12). This was confirmed by our findings, which showed that African Americans have a higher average number of comorbidities compared to white patients (Figure 6B). Especially concerning is the fact that while the incidence rate of breast cancer is similar for white and black females in the US between the years 1999–2013 the mortality among black females continues to be higher than for white females (8). Our single institution study did not find a difference in overall or disease free survival for our patients; however, this is probably due to small sample size.
It is also important to note that many of the patients included in this study were healthy individuals overall. The majority of patients have between zero and one comorbidity, which leaves them well below the threshold of six at which point decreased overall and long-term survival is seen (Figure 3A,B). This is excellent news as we confirm that most of our patients who walk through the door with will not be doomed to a worse prognosis due to their chronic diseases. While this validates our clinical suspicion for the patient in clinic with a lifetime of multiple complicated comorbid conditions it also provides reassurance that many of our patients should be able to successfully navigate breast cancer treatment even if not in perfect health.
Finally, the strength of our study lies in the long length of follow-up time. With our average follow-up time of 5 years we are able to capture a greater snapshot of our patients lives and thus more reliably estimate the impact of not only the breast cancer but also the chronic conditions they have faced. Other risk indexes only track out to 30 days and 1 year but we have shown that by simply counting the number of comorbidities we can establish a threshold at which survival reliably decreases. It has also been shown that independent of age, stage and type of treatment, increasing comorbidities were strongly associated with an increased risk of death at 3 years (21). Now, in our study we have shown that this increased risk of death extends over a longer timeframe. Also distinctive in our study was our further characterization of the comorbidities that these sick patients carry. We found that the most common comorbidities in those patients were very similar to those with less comorbidity. This provides further confirmation that it is the number or comorbidities and not the type of comorbidity that impacts the overall and disease free survival.
The limitations of our study include the limited sample size, single institution and retrospective nature of the study. The sample size for this database was adequate to analyze our question but was limited by the manual extraction of the data. We will require an increased sample size for further analysis of survival according to stratifications of staging and treatment. The retrospective nature of the study allowed us to study multiple outcomes however without any control over the exposure and the patient environment it falls short of a randomized control study. Furthermore the timeframe over which these patients were included was almost one decade, which could lead to disparities in treatments and thus survival. The final limitation is regarding the abstraction from the medical record, which relied on human labor and thus is subject to error and missing data, which must be accounted for in statistical analysis.
Conclusions
Thankfully the average 5-year survival for women in the United States is almost 90% and mortality has been decreasing steadily over the last decade due to diligent detection efforts by primary care providers and increasingly advanced treatments. Our results show that the population of women who eventually face breast cancer is heterogeneous with a wide variety of comorbidities, which have a significant impact on the disease free and overall survival. By further elucidating the unique health history of each patient whether it ranges from no prior medical history to a host of previous surgeries and diagnosis we can better anticipate their individual treatment needs and risks.
Acknowledgements
The authors would like to express their sincere gratitude and admiration for all the patients’ courage and generosity in participating in this study. We are also very appreciative of the James D. Popp Foundation for the continued support and inspiration that made this study happen.
Funding: This work is supported by National Institute of Health grant (Grant number: R01CA160688) and a Susan G. Komen Foundation Investigator Initiated Research grant (Grant number: IIR12222224) (awarded to Dr. K Takabe).
Footnote
Conflicts of Interest: The authors have no conflicts of interest to declare.
Ethical Statement: The Virginia Commonwealth University Institutional Review Board approved this study (HM20000345). Patients were requested to be a part of the study at their visit to the Breast Clinic in the Division of Surgical Oncology at VCU Medical Center at which point their consent was attained and their Medical Record Numbers included in the study.
References
- Siegel RL, Miller KD, Jemal H. Cancer Statistics, 2017. CA Cancer J Clin 2017;67:7-30. [Crossref] [PubMed]
- U.S. Cancer Statistics Working Group. United States Cancer Statistics: 1999–2013 Incidence and Mortality Web-based Report. Atlanta (GA): Department of Health and Human Services, Centers for Disease Control and Prevention, and National Cancer Institute, 2016.
- Centers for Disease Control and Prevention. The State of Aging and Health in America 2013. Atlanta, GA; Centers for Disease Control and Prevention, US Department of Health and Human Services, 2013.
- Moo TA, Simmons RM. Impact of comorbidities on surgical outcomes following mastectomy in elderly breast cancer patients: An analysis of the NSQIP database. J Clin Oncol 2015;33:abstr 88.
- Goldman L, Caldera DL, Nussbaum SR, et al. Multifactorial index of cardiac risk in non-cardiac surgical procedures. N Engl J Med 1977;297:845-50. [Crossref] [PubMed]
- Bilimoria KY, Liu Y, Paruch JL, et al. Development and Evaluation of the Universal ACS NSQIP Surgical Risk Calculator: A Decision Aid and Informed Consent Tool for Patients and Surgeons. J Am Coll Surg 2013;217:833-42.e1-3.
- Charlson ME, Pompei P, Ales KL, et al. A new method of classifying prognostic comorbidity in longitudinal studies: development and validation. J Chronic Dis 1987;40:373-83. [Crossref] [PubMed]
- Kochanek KD, Murphy SL, Xu J, et al. Deaths: Final Data for 2014. Natl Vital Stat Rep 2016;65:1-122. [PubMed]
- Fadol AP, Banchs J, Hassan S, et al. Recovery of chemotherapy-induced left ventricular dysfunction in cancer survivors. J Clin Oncol 2016;34:abstr 136.
- Saiki H, Petersen IA, Scott CG, et al. Risk of Heart Failure with Preserved Ejection Fraction in Older Women after Completing Radiotherapy for Breast Cancer. Circulation 2017;135:1388-96. [Crossref] [PubMed]
- Clarke M, Collins R, Darby S, et al. Effects of radiotherapy and of differences in the extent of surgery for early breast cancer on local reoccurrence and 15 year survival: an overview of the randomized trials. Lancet 2005;366:2087-106. [Crossref] [PubMed]
- National Center for Health Statistics (US). Health, United States, 2015: With Special Feature on Racial and Ethnic Health Disparities. Hyattsville (MD): National Center for Health Statistics (US); 2016 May. Report No.: 2016-1232.
- Arnold M, Pandaya N, Byrnes G, et al. Global burden of cancer attributable to high body mass index in 2012: a population based study. Lancet Oncol 2015;16:36-46. [Crossref] [PubMed]
- Au CC, Furness JB, Brown KA. Ghrelin and Breast Cancer: Emerging Roles in Obesity, Estrogen Regulation and Cancer. Front Oncol 2017;6:265. [Crossref] [PubMed]
- Guldberg TL, Christensen S, Zachariae R, et al. Prognostic factors in early breast cancer associated with body mass index, physical functioning, physical activity and comorbidity: data from a nationwide Danish cohort. Breast Cancer Res Treat 2017;162:159-67. [Crossref] [PubMed]
- Nunez C, Bauman A, Egger S, et al. Obesity, physical activity and cancer risks: Results from the Cancer, Lifestyle and Evaluation of Risk Study (CLEAR). Cancer Epidemiol 2017;47:56-63. [Crossref] [PubMed]
- Rosner B, Eliassen AH, Toriola AT, et al. Weight and weight changes in early adulthood and later breast cancer. Int J Cancer 2017;140:2003-14. [Crossref] [PubMed]
- Alfano CM, Peng J, Andridge RR, et al. Inflammatory Cytokines and Comorbidity Development in Breast Cancer Survivors Versus Noncancer Controls: Evidence for Accelerated Aging? J Clin Oncol 2017;35:149-56. [Crossref] [PubMed]
- Suppli NP, Johansen C, Christensen J, et al. Increased Risk for Depression After Breast Cancer: A Nationwide Population-Based Cohort Study of Associated Factors in Denmark, 1998-2011. J Clin Oncol 2014;32:3831-9. [Crossref] [PubMed]
- Suppli NP, Johansen C, Kessing LV, et al. Survival After Early-Stage Breast Cancer of Women Previously Treated for Depression: A Nationwide Danish Cohort Study. J Clin Oncol 2017;35:334-42. [Crossref] [PubMed]
- Satariano WA, Ragland DR. The Effect of Comorbidity on 3-Year Survival of Women with Primary Breast Cancer. Ann Intern Med 1994;120:104-10. [Crossref] [PubMed]