Development and validation of an intratumoral-peritumoral deep transfer learning fusion model for differentiating BI-RADS 3–4 breast nodules
Highlight box
Key findings
• In this study, ultrasonic images were utilized to assess the diagnostic accuracy of deep transfer learning (DTL) features derived from intra-tumoral and peritumoral regions (PTRs) for distinguishing Breast Imaging Reporting and Data System (BI-RADS) 3–4 breast nodules.
What is known and what is new?
• The American College of Radiology (ACR) BI-RADS assists clinicians in formulating treatment plans for breast nodules. However, certain breast nodules, especially those categorized as BI-RADS 3–4, present a broad spectrum of malignancy probabilities, creating diagnostic dilemmas and potentially resulting in unnecessary surgeries or biopsy procedures.
• Developing an accurate and non-invasive method for determining the benignity or malignancy of breast nodules is of utmost importance.
What is the implication, and what should change now?
• The DTL feature model, which is based on intratumoral and PTRs, can efficiently predict the benignity and malignancy of BI-RADS 3–4 breast nodules. It outperforms ultrasound physicians in diagnostic performance and provides significant support for treatment decision-making in patients with breast nodules.
Introduction
Breast cancer is one of the most prevalent malignant tumors in women (1), and it is increasingly affecting younger age groups (2). It has become the leading cause of cancer-related deaths among women aged 20 to 59 years (3). Currently, ultrasound is one of the primary methods for early screening of breast cancer (4). Early diagnosis and treatment are critical for improving the survival rates and quality of life of breast cancer patients (5). The American College of Radiology (ACR) Breast Imaging Reporting and Data System (BI-RADS) (6) aids clinicians in developing treatment plans for breast nodules. However, certain breast nodules, particularly those classified as BI-RADS 3–4, exhibit a wide range of malignancy probabilities, posing diagnostic challenges and leading to unnecessary surgeries or biopsy procedures (7). Therefore, developing an accurate and non-invasive method for determining the benignity or malignancy of breast nodules is of paramount importance.
Over the years, various non-invasive diagnostic models have been developed to help clinicians differentiate benign from malignant BI-RADS 3–4 breast nodules, typically leveraging clinical risk factors, imaging features, and sometimes molecular biomarkers. Predictors such as patient age, nodule shape, margin characteristics, and vascularization patterns on Doppler ultrasound are often included (8-11). Although these models have improved diagnostic accuracy to some extent, they still face limitations, including inter-observer variability in image interpretation, reliance on limited feature sets, and suboptimal performance in specific subgroups of patients. These constraints underscore the need for more sophisticated approaches that can capture the subtle heterogeneity of breast nodules and minimize human interpretation biases.
Breast tumor tissues typically comprise tumor cells and stromal cells, which can induce significant changes in the peritumoral stroma (12). Research in tumor biology has highlighted the critical role of the tumor microenvironment in the initiation, progression, and metastasis of cancer (13). A comprehensive analysis of both the intratumoral region (ITR) and the peritumoral region (PTR) can enhance the differentiation between benign and malignant tumors (14). Radiomics enables the extraction of subtle features from medical images that are not discernible to the naked eye through high-throughput methods (15). These subtle features may be closely associated with tumor heterogeneity and specific biological behaviors of tumor cells (16).
Meanwhile, deep learning (DL), an advanced branch of artificial intelligence (AI), can mimic the human nervous system through multi-layer neural networks (17), performing hierarchical abstraction and feature extraction of complex image data to achieve efficient classification and prediction (18). In recent years, become a significant trend in medical imaging research.
The primary objective of this study is to evaluate the diagnostic value of ultrasound-based deep transfer learning (DTL) features derived from the ITR and various PTRs in distinguishing between benign and malignant BI-RADS 3–4 breast nodules. By integrating DTL features from both ITR and PTR, we aim to develop a more accurate, stable, and objective diagnostic model that enhances the differential diagnostic performance for BI-RADS 3–4 breast nodules, reduces misdiagnosis rates, and optimizes clinical decision-making. We present this article in accordance with the TRIPOD reporting checklist (available at https://gs.amegroups.com/article/view/10.21037/gs-24-457/rc).
Methods
Ethical approval
The study was conducted in accordance with the Declaration of Helsinki and its subsequent amendments. The two-center observational study was conducted using a retrospective cohort design and was approved by Ethics Committees of Shanghai Sixth People’s Hospital Affiliated to Shanghai Jiao Tong University School of Medicine (No. 2019-027) and Shuguang Hospital Affiliated to Shanghai University of Traditional Chinese Medicine (No. 2024-KY-117K). As a retrospective study, it was exempted from the requirement for patient informed consent.
Patient selection
We retrospectively collected data from female patients with breast nodules classified as BI-RADS 3–4 via ultrasound during routine preoperative examinations between June 2017 and June 2023 at two centers. The inclusion criteria were: (I) availability of final surgical or core needle biopsy pathological diagnoses; (II) breast ultrasound examination performed within 2 weeks prior to biopsy or surgery, with clear and complete two-dimensional grayscale ultrasound images of the target nodule’s maximum diameter, accompanied by detailed descriptive reports and clinical pathological data; (III) classification of the target lesion into BI-RADS 3–4A, 4B, or 4C according to the second edition of the ACR BI-RADS (6). The exclusion criteria included: (I) ambiguous pathological results; (II) tumors too large to be fully visualized in images; (III) patients who received anticancer treatments (such as chemotherapy, radiotherapy, or endocrine therapy) before surgery; (IV) incomplete imaging or clinical data.
Ultimately, Center 1 (Shanghai Sixth People’s Hospital Affiliated to Shanghai Jiao Tong University School of Medicine) included 416 patients (age range, 18 to 86 years) and Center 2 (Shuguang Hospital Affiliated to Shanghai University of Traditional Chinese Medicine) included 139 patients (age range, 19 to 88 years). We collected clinical and pathological data, including age, tumor size, BI-RADS classification, and postoperative or biopsy pathological diagnosis, from enrolled patients for subsequent statistical analysis. For patients with multiple BI-RADS 3–4 breast lesions, only the nodule with the highest BI-RADS category was included to ensure statistical independence of each observation. The nodules from Center 1 were randomly divided into a training set (n=291) and an internal validation set (n=125) at a ratio of 7:3, while nodules from Center 2 were used as an external validation set (n=139). The study workflow is illustrated in Figures 1,2.
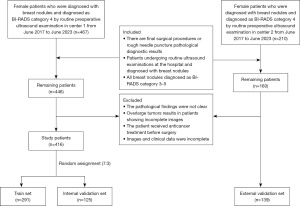
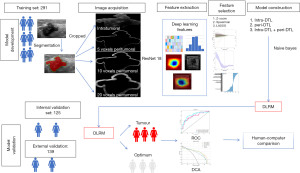
Clinical data collection
We collected clinical characteristics of patients with BI-RADS 3–4 breast nodules, including age, tumor size, ACR BI-RADS classification, and surgical pathological results. All pathological results were obtained from surgical specimens or biopsy samples and served as the gold standard in this study.
Ultrasound image acquisition and preprocessing
Breast ultrasound images were acquired using five different ultrasound systems to ensure data diversity: GE LOGIQ E8, Siemens S2000, Philips EPIQ5, EPIQ7, and IU22. Each ultrasound device was equipped with a linear array probe with a working frequency range of 4 to 12 MHz, capable of generating high-resolution grayscale images. Standard imaging protocols were followed to minimize variability, including consistent probe positioning, compression force, and imaging angles. For each identified breast nodule, the largest unmarked long-axis grayscale ultrasound image was selected for analysis, and the maximum longitudinal diameter of each lesion was recorded to assess size-related features. All ultrasound images were stored in Digital Imaging and Communications in Medicine (DICOM) format to ensure compatibility with subsequent processing and analysis steps. According to the ACR BI-RADS guidelines (6), which provide a standardized scoring system for breast nodules, we classified BI-RADS scores of 3 to 4A as benign and 4B to 4C as malignant. For the controversial nodules, a physician with over 25 years of experience in breast ultrasound diagnosis made the final determination.
Prior to feature extraction, all ultrasound images underwent a series of preprocessing steps to enhance image quality and standardize the input data for deep learning models. Two experienced radiologists independently delineated the regions of interest (ROIs) of the nodules using ITK-SNAP software. In cases of initial annotation discrepancies, a third radiologist was consulted to achieve final consensus on ROI delineation.
The grayscale values of the images were normalized to a range of 0 to 1 to reduce variability introduced by different ultrasound devices and imaging parameters. Subsequently, bilinear interpolation was used to resize the images to 224×224 pixels to meet the input requirements of the DTL models. Additionally, by expanding the boundaries of the intratumoral ROI outward by 5, 10, and 20 voxels, multiple PTRs were defined to capture the contextual information of the surrounding tissue. These peritumoral ROIs were generated automatically to ensure consistency across all samples.
Feature extraction
DTL feature extraction
For deep learning-based feature extraction, we employed a pre-trained ResNet-18 convolutional neural network (CNN) as the primary feature extraction tool. The ResNet-18 model was initialized with weights pre-trained on the ImageNet dataset. To adapt the ResNet-18 model for ultrasound image analysis, the final fully connected layer was removed, retaining only the convolutional layers to extract high-level feature maps.
The preprocessed ultrasound images were input into the modified ResNet-18 model, and global average pooling was applied to obtain fixed-length feature vectors. This process was performed separately for the ITR and each defined PTR (5, 10, and 20 voxels), thereby generating distinct feature sets that capture both the tumor core and its surrounding microenvironment.
Feature selection and model construction
To identify the most discriminative features for differentiating benign from malignant nodules, a two-step feature selection process was implemented. First, analysis of variance (ANOVA) was conducted to evaluate the statistical significance of each feature, retaining those with P values below a predefined threshold (e.g., P<0.05). Subsequently, the least absolute shrinkage and selection operator (LASSO) regression method was applied to further refine the feature set by minimizing overfitting and enhancing the model’s generalizability. The optimal lambda parameter for LASSO was determined through cross-validation. Features selected from both radiomics and DTL approaches were used to construct machine learning models aimed at predicting the malignancy risk of BI-RADS 3–4 breast nodules. Three types of models were developed: intratumoral models, peritumoral models, and combined intratumoral and peritumoral models.
The diagnostic performance of the intratumoral model, peritumoral model, and combined intratumoral-peritumoral model was evaluated by comparing their area under the curve (AUC) values, accuracy, sensitivity, and specificity. The best-performing model was then compared with the diagnostic results of ultrasound physicians.
Statistical analysis
Statistical analyses were performed using Python and SPSS 26.0, with pathological results serving as the gold standard. Continuous variables were tested for normal distribution and homogeneity of variance. Normally distributed data were analyzed using independent two-sample t-tests, while non-normally distributed continuous variables were assessed using the Mann-Whitney U test. Categorical variables were analyzed using the χ2 test. Differences in AUC between different models were compared using the DeLong test. Selected clinical variables were evaluated using SPSS 26.0. Python 3.10 was utilized for calculating intraclass correlation coefficients (ICC), conducting Spearman rank correlation tests, performing Z-score normalization, and executing LASSO regression analyses. All statistical tests were two-sided, with P<0.05 considered statistically significant.
Results
Clinical characteristics analysis
A total of 555 female patients with breast nodules were included in this study, with postoperative pathological results indicating 275 cases as benign lesions and 280 cases as malignant lesions. The age range of the patients was 18 to 88 years, with a mean age of 48.11±14.83 years. The training group consisted of 291 patients (mean age 48.35±15.03 years), and the validation group comprised 264 patients (mean age 47.84±14.63 years). There were no significant differences between the two groups in terms of age (P=0.57), nodule location (P=0.86), and nodule diameter (P=0.38) (Table 1).
Table 1
Feature name | All | Training | Validation | P value |
---|---|---|---|---|
Size (mm) | 19.17±10.20 | 18.88±10.48 | 19.50±9.89 | 0.38 |
Age (years) | 48.11±14.83 | 48.35±15.03 | 47.84±14.63 | 0.57 |
Calcification | 0.60 | |||
No | 400 (72.07) | 213 (73.20) | 187 (70.83) | |
Yes | 155 (27.93) | 78 (26.80) | 77 (29.17) | |
CDFI | 0.18 | |||
No | 339 (61.08) | 186 (63.92) | 153 (57.95) | |
Yes | 216 (38.92) | 105 (36.08) | 111 (42.05) | |
BI-RADS | 0.53 | |||
RADS 3–4A | 387 (69.73) | 199 (68.38) | 188 (71.21) | |
RADS 4B–4C | 168 (30.27) | 92 (31.62) | 76 (28.79) | |
Side | 0.86 | |||
Left | 285 (51.35) | 151 (51.89) | 134 (50.76) | |
Right | 270 (48.65) | 140 (48.11) | 130 (49.24) |
Data are presented as number (percentage), mean ± standard deviation. BI-RADS, Breast Imaging Reporting and Data System; CDFI, color Doppler flow imaging.
Feature extraction model selection
To identify the optimal diagnostic model, we evaluated the performance of models based on different pre-trained networks (ResNet-18, ResNet-50, DenseNet-121, ViT, GoogLeNet, and VGG-11) (Table 2). The results showed that ResNet-18 performed the best, with AUCs of 0.811 [95% confidence interval (CI): 0.761–0.860] in the training set and 0.813 (95% CI: 0.737–0.890) in the internal validation set. In the training set, its accuracy, sensitivity, and specificity were 76.2%, 69.2%, and 83.1%, respectively; in the internal validation set, they were 75.4%, 75.0%, and 75.8%, respectively.
Table 2
Model | Group | AUC (95% CI) | Accuracy | Sensitivity | Specificity |
---|---|---|---|---|---|
ResNet-18 | Training | 0.811 (0.761–0.860) | 0.762 | 0.692 | 0.831 |
Internal validation | 0.813 (0.737–0.890) | 0.754 | 0.750 | 0.758 | |
ResNet-50 | Training | 0.806 (0.756–0.855) | 0.752 | 0.678 | 0.824 |
Internal validation | 0.778 (0.699–0.858) | 0.722 | 0.578 | 0.871 | |
DenseNet-121 | Training | 0.752 (0.697–0.806) | 0.687 | 0.644 | 0.730 |
Internal validation | 0.736 (0.650–0.823) | 0.667 | 0.609 | 0.726 | |
ViT | Training | 0.547 (0.481–0.613) | 0.561 | 0.644 | 0.480 |
Internal validation | 0.559 (0.459–0.661) | 0.532 | 0.437 | 0.629 | |
GoogLeNet | Training | 0.709 (0.650–0.768) | 0.653 | 0.74 | 0.568 |
Internal validation | 0.715 (0.624–0.805) | 0.675 | 0.625 | 0.726 | |
VGG-11 | Training | 0.695 (0.634–0.755) | 0.680 | 0.507 | 0.851 |
Internal validation | 0.708 (0.618–0.798) | 0.651 | 0.594 | 0.710 |
AUC, area under the curve; CI, confidence interval; ViT, vision transformer; VGG, visual geometry group.
From each tumor ROI’s maximum cross-section and various peritumoral cross-sections (5 voxels, 10 voxels, 20 voxels), 512 to 2,048 DTL features were extracted from ultrasound images. Subsequently, feature selection was performed using Spearman rank correlation tests and LASSO regression, and features with non-zero LASSO coefficients were ultimately selected for model construction.
Feature extraction and model diagnostic performance
Intratumoral feature extraction and model diagnostic performance
From the ITR, 512 DTL features were extracted, and after LASSO selection, 26 non-zero radiomics features were obtained. The diagnostic performance of five machine learning models—logistic regression (LR), support vector machine (SVM), K-nearest neighbors (KNN), naive Bayes, and multi-layer perceptron (MLP)—was compared. The results indicated that the naive Bayes model significantly outperformed the other models in the validation cohort, with an AUC of 0.862 (95% CI: 0.796–0.928) in the internal validation set and 0.844 (95% CI: 0.778–0.909) in the external validation set. In the internal validation set, its accuracy, sensitivity, specificity, precision, and F1 score were 0.808, 0.875, 0.738, 0.778, and 0.824, respectively; in the external validation set, they were 0.777, 0.866, 0.694, 0.725, and 0.789, respectively (Table 3, Figure 3).
Table 3
Model | Group | AUC (95% CI) | Accuracy | Sensitivity | Specificity |
---|---|---|---|---|---|
Intra-DTL | Training | 0.878 (0.839–0.917) | 0.797 | 0.826 | 0.768 |
Internal validation | 0.862 (0.796–0.928) | 0.808 | 0.875 | 0.738 | |
External validation | 0.844 (0.778–0.909) | 0.777 | 0.866 | 0.694 | |
Peri (5 voxels)-DTL | Training | 0.873 (0.833–0.914) | 0.801 | 0.671 | 0.937 |
Internal validation | 0.855 (0.787–0.924) | 0.808 | 0.828 | 0.787 | |
External validation | 0.777 (0.700–0.855) | 0.734 | 0.612 | 0.847 | |
Peri (10 voxels)-DTL | Training | 0.903 (0.870–0.936) | 0.821 | 0.765 | 0.880 |
Internal validation | 0.907 (0.853–0.961) | 0.848 | 0.922 | 0.770 | |
External validation | 0.891 (0.836–0.946) | 0.856 | 0.791 | 0.917 | |
Peri (20 voxels)-DTL | Training | 0.902 (0.867–0.936) | 0.838 | 0.819 | 0.859 |
Internal validation | 0.871 (0.812–0.932) | 0.784 | 0.797 | 0.770 | |
External validation | 0.838 (0.772–0.904) | 0.763 | 0.776 | 0.750 | |
Intra-DTL+ peri (10 voxels)-DTL | Training | 0.911 (0.877–0.944) | 0.852 | 0.852 | 0.852 |
Internal validation | 0.909 (0.860–0.958) | 0.824 | 0.734 | 0.918 | |
External validation | 0.910 (0.862–0.959) | 0.863 | 0.881 | 0.847 |
AUC, area under the curve; CI, confidence interval; DTL, deep transfer learning; intra, intratumoral; peri, peritumoral.
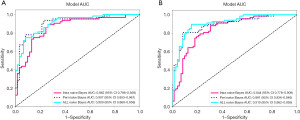
Different PTR feature extraction and model diagnostic performance
From the PTRs at 5 voxels, 10 voxels, and 20 voxels, 512 DTL features were extracted, and after LASSO selection, 42 non-zero radiomics features were obtained. Among the five machine learning models, the naive Bayes model based on features from the 10-voxel PTR performed best, with AUCs of 0.907 (95% CI: 0.853–0.961) the internal validation set and 0.891 (95% CI: 0.836–0.946) in the external validation set. Its accuracy, sensitivity, specificity, precision, and F1 score in the internal validation set were 0.848, 0.922, 0.770, 0.808, and 0.861, respectively; in the external validation set, they were 0.856, 0.791, 0.917, 0.898, and 0.841, respectively (Table 3, Figure 3).
Combined intratumoral and peritumoral model diagnostic performance
From the intratumoral and 10-voxel PTRs, 1,024 DTL features were extracted, and after LASSO selection, 39 non-zero radiomics features were obtained. A naive Bayes model was constructed for diagnosis, achieving an AUC of 0.909 (95% CI: 0.860–0.958, Figure 4A) in the internal validation set and 0.910 (95% CI: 0.862–0.959, Figure 4B) in the external validation set. Its accuracy, sensitivity, specificity, precision, and F1 score in the internal validation set were 0.824, 0.734, 0.918, 0.904, and 0.810, respectively; in the external validation set, they were 0.863, 0.881, 0.847, 0.843, and 0.861, respectively. These results indicate that the combined intratumoral and peritumoral feature model outperformed models using only intratumoral or peritumoral features in both the training and validation sets (Table 3, Figure 3).
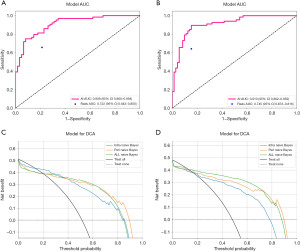
Ultrasound physician diagnostic performance
In differentiating between benign and malignant breast nodules, the diagnostic performance of clinical ultrasound physicians was as follows: an AUC of 0.722 (95% CI: 0.643–0.800) in the internal validation set and 0.745 (95% CI: 0.673–0.816) in the external validation set. In the internal validation set, their accuracy, sensitivity, specificity, precision, and F1 score were 0.720, 0.656, 0.787, 0.764, and 0.706, respectively; in the external validation set, they were 0.748, 0.642, 0.847, 0.796, and 0.711, respectively (Table 4).
Table 4
Cohort | Accuracy | AUC (95% CI) | Sensitivity | Specificity | PPV | NPV | Precision | Recall | F1 | Threshold |
---|---|---|---|---|---|---|---|---|---|---|
Internal validation | ||||||||||
AI | 0.824 | 0.909 (0.860–0.958) | 0.797 | 0.852 | 0.850 | 0.800 | 0.850 | 0.797 | 0.823 | 0.971 |
Physician | 0.720 | 0.722 (0.643–0.800) | 0.656 | 0.787 | 0.764 | 0.686 | 0.764 | 0.656 | 0.706 | 1.000 |
External validation | ||||||||||
AI | 0.849 | 0.910 (0.862–0.959) | 0.836 | 0.861 | 0.848 | 0.849 | 0.848 | 0.836 | 0.842 | 0.091 |
Physician | 0.748 | 0.745 (0.673–0.816) | 0.642 | 0.847 | 0.796 | 0.718 | 0.796 | 0.642 | 0.711 | 1.000 |
AI, artificial intelligence; AUC, area under the curve; CI, confidence interval; NPV, negative predictive value; PPV, positive predictive value.
Overall, the combined intratumoral and peritumoral feature model outperformed models using only intratumoral or peritumoral features in terms of AUC, accuracy, sensitivity, and specificity. Compared to the diagnostic results of ultrasound physicians, the deep learning models demonstrated superior diagnostic efficiency (Figure 4).
Decision curve analysis (DCA)
DCA results indicated that the deep learning models had greater decision-making benefits than clinical physician diagnoses (Figure 4). Across different threshold probabilities, whether using intratumoral features, peritumoral features, or combined features, the naive Bayes models consistently yielded higher net benefits than the “all-treat” or “no-treat” strategies. Particularly within lower threshold ranges, all models exhibited significant net benefit improvements, suggesting that in actual clinical applications, these models can more effectively assist physicians in making rational treatment decisions, thereby reducing the risks of overdiagnosis and missed diagnoses.
Discussion
With the gradual increase in public awareness of health management and the widespread application of high-resolution ultrasound, the detection rate of breast nodules has risen annually (19). Consequently, the number of BI-RADS 3–4 category breast nodules has also increased, and there has been ongoing debate regarding their diagnostic and treatment strategies (20). Therefore, there is an urgent need for methods that can enhance the performance of differentiating benign and malignant BI-RADS 3–4 breast nodules. The study by Raza et al. (21) demonstrated that age is a significant clinical factor in predicting malignant breast tumors. Previous research has suggested that the ACR BI-RADS classification is an important factor in assessing the malignancy risk of breast nodules (22). However, its overall accuracy and benign detection rate are relatively low, potentially leading to overtreatment (23). Additionally, the diagnostic performance of ultrasound physicians largely depends on personal experience, highlighting the necessity for more stable and objective diagnostic methods to accurately differentiate the benignity and malignancy of ACR BI-RADS 4 category nodules.
Radiomics, as an emerging non-invasive technology, has shown tremendous potential in predicting tumor biomarkers. Unlike the previous study (24) that primarily focused on intratumoral features, our research simultaneously considers key biological features in the PTRs, which may enhance the predictive accuracy of radiomics models. The interactions between intratumoral and PTRs, such as the release of cytokines and the formation of an immunosuppressive microenvironment, influence tumor evolution and progression (25). This suggests that integrating intratumoral and peritumoral features within deep learning models could enhance their predictive capabilities.
In recent years, deep convolutional neural networks (CNNs) have achieved significant breakthroughs in the field of medical imaging (26). However, their implementation typically requires large amounts of training data, which can lead to overfitting (27). Transfer learning (TL) based on pre-trained CNNs can be effectively trained on smaller image datasets while mitigating the risk of overfitting (28), making it widely applicable in various medical image analyses. Our study results indicate that models based on intratumoral DTL features achieved AUCs of 0.862 (95% CI: 0.796–0.928) in the internal validation set and 0.844 (95% CI: 0.778–0.909) in the external validation set. Models based on peritumoral 10 voxels region DTL features achieved AUCs of 0.907 (95% CI: 0.853–0.961) in the training set and 0.891 (95% CI: 0.836–0.946) in the internal validation set. The combined intratumoral and peritumoral ultrasound DTL feature-based predictive model achieved AUCs of 0.909 (95% CI: 0.860–0.958) in the internal validation set and 0.910 (95% CI: 0.862–0.959) in the external validation set, demonstrating effective capability in predicting the benignity and malignancy of BI-RADS 3–4 breast nodules. Furthermore, its performance was superior to models based solely on intratumoral features, although the differences did not reach statistical significance (P>0.05). Additionally, the deep learning models outperformed ultrasound physicians outperformed physicians in terms of accuracy (e.g., 92.1% vs. 78.3%), sensitivity (e.g., 88.6% vs. 72.4%), and specificity, indicating their potential in clinical applications. Ji et al. demonstrated that a multicenter-validated Transformer-based Computer Aided Design (CAD) model significantly improved radiologists’ consistency in BI-RADS 3–5 nodule classification (Cohen’s κ =0.85) with 92.1% sensitivity and 88.6% specificity, offering a standardized AI solution to reduce diagnostic variability and unnecessary biopsies (29).
There are several limitations in this study. First, the number of images used for training and testing was relatively small, which may affect the stability of the models. Future research should collect more data to validate the generalizability of the models. Second, as a retrospective study, it requires larger-scale prospective trials to further validate the effectiveness of the models. Finally, this study delineated the ROIs only in two dimensions, neglecting the three-dimensional features of tumors, which may limit the models’ ability to capture the complex structures of tumors.
Conclusions
In summary, this study integrated DTL features from both intratumoral and PTRs to construct an efficient model for differentiating benign and malignant BI-RADS 3–4 breast nodules. The model demonstrated potential superiority over traditional ultrasound diagnosis and single radiomics models. Future studies should optimize the models further and validate them using larger, multicenter datasets.
Acknowledgments
None.
Footnote
Reporting Checklist: The authors have completed the TRIPOD reporting checklist. Available at https://gs.amegroups.com/article/view/10.21037/gs-24-457/rc
Data Sharing Statement: Available at https://gs.amegroups.com/article/view/10.21037/gs-24-457/dss
Peer Review File: Available at https://gs.amegroups.com/article/view/10.21037/gs-24-457/prf
Funding: The study was funded by
Conflicts of Interest: All authors have completed the ICMJE uniform disclosure form (available at https://gs.amegroups.com/article/view/10.21037/gs-24-457/coif). The authors have no conflicts of interest to declare.
Ethical Statement: The authors are accountable for all aspects of the work in ensuring that questions related to the accuracy or integrity of any part of the work are appropriately investigated and resolved. The study was conducted in accordance with the Declaration of Helsinki and its subsequent amendments. The two-center observational study was approved by Ethics Committees of Shanghai Sixth People’s Hospital Affiliated to Shanghai Jiao Tong University School of Medicine (No. 2019-027) and Shuguang Hospital Affiliated to Shanghai University of Traditional Chinese Medicine (No. 2024-KY-117K). As a retrospective study, it was exempted from the requirement for patient informed consent.
Open Access Statement: This is an Open Access article distributed in accordance with the Creative Commons Attribution-NonCommercial-NoDerivs 4.0 International License (CC BY-NC-ND 4.0), which permits the non-commercial replication and distribution of the article with the strict proviso that no changes or edits are made and the original work is properly cited (including links to both the formal publication through the relevant DOI and the license). See: https://creativecommons.org/licenses/by-nc-nd/4.0/.
References
- Lu ZN, Song J, Sun TH, et al. UBE2C affects breast cancer proliferation through the AKT/mTOR signaling pathway. Chin Med J (Engl) 2021;134:2465-74. [Crossref] [PubMed]
- Zhao F, Cai C, Liu M, et al. Identification of the lymph node metastasis-related automated breast volume scanning features for predicting axillary lymph node tumor burden of invasive breast cancer via a clinical prediction model. Front Endocrinol (Lausanne) 2022;13:881761. [Crossref] [PubMed]
- Pal R, Srivastava N, Chopra R, et al. Investigation of DNA damage response and apoptotic gene methylation pattern in sporadic breast tumors using high throughput quantitative DNA methylation analysis technology. Mol Cancer 2010;9:303. [Crossref] [PubMed]
- Zhou Y, Wei J, Wu D, et al. Generating Full-Field Digital Mammogram From Digitized Screen-Film Mammogram for Breast Cancer Screening With High-Resolution Generative Adversarial Network. Front Oncol 2022;12:868257. [Crossref] [PubMed]
- Wu X, Liu H, Han D, et al. Elucidation and Structural Modeling of CD71 as a Molecular Target for Cell-Specific Aptamer Binding. J Am Chem Soc 2019;141:10760-9. [Crossref] [PubMed]
- Maani N, Westergard S, Yang J, et al. NF1 Patients Receiving Breast Cancer Screening: Insights from The Ontario High Risk Breast Screening Program. Cancers (Basel) 2019;11:707. [Crossref] [PubMed]
- Park B, Lim SE, Ahn H, et al. Heterogenous Effect of Risk Factors on Breast Cancer across the Breast Density Categories in a Korean Screening Population. Cancers (Basel) 2020;12:1391. [Crossref] [PubMed]
- Yang Y, Long H, Feng Y, et al. A multi-omics method for breast cancer diagnosis based on metabolites in exhaled breath, ultrasound imaging, and basic clinical information. Heliyon 2024;10:e32115. [Crossref] [PubMed]
- Zhong LC, Yang T, Gu LP, et al. The diagnostic performance of shear wave velocity ratio for the differential diagnosis of benign and malignant breast lesions: Compared with VTQ, and mammography. Clin Hemorheol Microcirc 2021;77:123-31. [Crossref] [PubMed]
- Huang H, Wan J, Ao X, et al. ECM1 and ANXA1 in urinary extracellular vesicles serve as biomarkers for breast cancer. Front Oncol 2024;14:1408492. [Crossref] [PubMed]
- Zhou Y, Li Y, Liu Y, et al. The value of contrast-enhanced energy-spectrum mammography combined with clinical indicators in detecting breast cancer in Breast Imaging Reporting and Data System (BI-RADS) 4 lesions. Quant Imaging Med Surg 2024;14:8272-80. [Crossref] [PubMed]
- Yan Z, Sheng Z, Zheng Y, et al. Cancer-associated fibroblast-derived exosomal miR-18b promotes breast cancer invasion and metastasis by regulating TCEAL7. Cell Death Dis 2021;12:1120. [Crossref] [PubMed]
- Khan K, Long B, Deshpande GM, et al. Bidirectional Tumor-Promoting Activities of Macrophage Ezrin. Int J Mol Sci 2020;21:7716. [Crossref] [PubMed]
- Wu Q, Wang S, Zhang S, et al. Development of a Deep Learning Model to Identify Lymph Node Metastasis on Magnetic Resonance Imaging in Patients With Cervical Cancer. JAMA Netw Open 2020;3:e2011625. [Crossref] [PubMed]
- Jin J, Yao Z, Zhang T, et al. Deep learning radiomics model accurately predicts hepatocellular carcinoma occurrence in chronic hepatitis B patients: a five-year follow-up. Am J Cancer Res 2021;11:576-89. [PubMed]
- Niu S, Wang X, Zhao N, et al. Radiomic Evaluations of the Diagnostic Performance of DM, DBT, DCE MRI, DWI, and Their Combination for the Diagnosisof Breast Cancer. Front Oncol 2021;11:725922. [Crossref] [PubMed]
- Joshi T, Joshi T, Pundir H, et al. Predictive modeling by deep learning, virtual screening and molecular dynamics study of natural compounds against SARS-CoV-2 main protease. J Biomol Struct Dyn 2021;39:6728-46. [Crossref] [PubMed]
- Gu Y, Xu W, Lin B, et al. Deep learning based on ultrasound images assists breast lesion diagnosis in China: a multicenter diagnostic study. Insights Imaging 2022;13:124. [Crossref] [PubMed]
- Zhang J, Zhang Y, Li L, et al. Pregnancy-associated plasma protein-A (PAPPA) promotes breast cancer progression. Bioengineered 2022;13:291-307. [Crossref] [PubMed]
- Castro SM, Tseytlin E, Medvedeva O, et al. Automated annotation and classification of BI-RADS assessment from radiology reports. J Biomed Inform 2017;69:177-87. [Crossref] [PubMed]
- Raza S, Goldkamp AL, Chikarmane SA, et al. US of breast masses categorized as BI-RADS 3, 4, and 5: pictorial review of factors influencing clinical management. Radiographics 2010;30:1199-213. [Crossref] [PubMed]
- Pfob A, Sidey-Gibbons C, Barr RG, et al. The importance of multi-modal imaging and clinical information for humans and AI-based algorithms to classify breast masses (INSPiRED 003): an international, multicenter analysis. Eur Radiol 2022;32:4101-15. [Crossref] [PubMed]
- Strigel RM, Burnside ES, Elezaby M, et al. Utility of BI-RADS Assessment Category 4 Subdivisions for Screening Breast MRI. AJR Am J Roentgenol 2017;208:1392-9. [Crossref] [PubMed]
- Lu H, Yin J. Texture Analysis of Breast DCE-MRI Based on Intratumoral Subregions for Predicting HER2 2+ Status. Front Oncol 2020;10:543. [Crossref] [PubMed]
- Do M, Kim H, Shin D, et al. Marker Identification of the Grade of Dysplasia of Intraductal Papillary Mucinous Neoplasm in Pancreatic Cyst Fluid by Quantitative Proteomic Profiling. Cancers (Basel) 2020;12:2383. [Crossref] [PubMed]
- Koklu M, Cinar I, Taspinar YS. CNN-based bi-directional and directional long-short term memory network for determination of face mask. Biomed Signal Process Control 2022;71:103216. [Crossref] [PubMed]
- Wachinger C, Reuter M, et al. Domain adaptation for Alzheimer's disease diagnostics. Neuroimage 2016;139:470-9. [Crossref] [PubMed]
- Mohan BP, Facciorusso A, Khan SR, et al. Real-time computer aided colonoscopy versus standard colonoscopy for improving adenoma detection rate: A meta-analysis of randomized-controlled trials. EClinicalMedicine 2020;29-30:100622. [Crossref] [PubMed]
- Ji H, Zhu Q, Ma T, et al. Development and validation of a transformer-based CAD model for improving the consistency of BI-RADS category 3-5 nodule classification among radiologists: a multiple center study. Quant Imaging Med Surg 2023;13:3671-87. [Crossref] [PubMed]