Development and validation of a semi-automatic radiomics ensemble model for preoperative evaluation of breast masses in mammotome-assisted minimally invasive resection
Highlight box
Key findings
• This study utilized data from 773 patients to develop and evaluate a combined model for predicting the nature of breast masses prior to Mammotome minimally invasive excisional biopsy based on semi-automatic segmentation. In the task of predicting tumor versus non-tumor patients, the final combined model achieved an area under the curve (AUC) of 0.890 (training cohort) and 0.780 (testing cohort). In the task of predicting adenosis versus other types of lesions, the final stacking model achieved an AUC of 0.890 (training cohort) and 0.771 (testing cohort).
What is known and what is new?
• Previous radiomics models for breast mass evaluation depend on manual lesion delineation, which is time-consuming and subjective.
• This study introduces two semi-automatic segmentation algorithms [DeepLabv3_ResNet50 and fully convolutional network (FCN)_ResNet50] that achieve high accuracy (Dice coefficients >92%), enabling robust radiomic feature extraction and improved predictive performance.
What is the implication, and what should change now?
• The constructed combined model can reduce reliance on manual delineation, enhance reproducibility, and effectively improve the diagnostic accuracy for the nature of breast masses.
• Further research and validation of the combined model in various clinical settings are necessary to support its integration into routine clinical practice and optimize patient-specific treatment plans.
Introduction
According to the statistics of the International Agency for Research on Cancer, breast cancer is one of the most common malignancies globally, with a continuously high incidence and mortality rate, particularly among women (1,2). The significantly higher incidence of breast cancer compared to other malignancies is an indisputable fact (3). Due to its high incidence and mortality rate, early screening and diagnosis of breast cancer have become of paramount importance (4). Ultrasound (US) has played a significant role in breast cancer screening, enabling doctors to detect and evaluate abnormalities in the breast, including lumps, cystic lesions, and intraluminal proliferations of the mammary ducts (5,6).
In recent years, with the continuous advancement of minimally invasive breast techniques and the increasing aesthetic demands of patients, the US-guided Mammotome system has been widely used for accurate biopsy of suspicious lesions or removal of benign breast lesion (7). The Mammotome minimally invasive excisional biopsy is commonly employed for the treatment and diagnosis of benign breast lesions with a diameter of less than 2 cm, particularly those that cannot be definitively diagnosed by conventional means. Preoperative assessment of the nature of breast lesion is crucial; by improving diagnostic accuracy, physicians can gain a better understanding of the biological characteristics of the lesion, thereby developing personalized treatment plans that enhance efficacy and reduce unnecessary surgical interventions. Moreover, this assessment helps to better determine the suitability of the Mammotome minimally invasive excisional biopsy, ensuring surgical success rates and providing comprehensive information for postoperative follow-up and management. For patients, making informed surgical choices, especially when distinguishing between benign and malignant lesion, significantly impacts their psychological well-being and treatment expectations. Therefore, preoperative assessment is not only a technical consideration but also a vital component of overall patient management (8,9).
Radiomics is a type of computer-assisted technology that can extract a large amount of features from medical images for automated analysis through high-throughput methods, enabling precise quantitative evaluation of lesions (10-12). These features provide deep insights into the microstructure, metabolism, and molecular expression of tumors, enabling more accurate evaluation of tumor malignancy and prognosis, as well as the objective quantification of tumor heterogeneity (13). The accuracy of radiomics models is influenced by lesion segmentation, which is a key factor (14,15). However, most current research still relies on professional radiologists to perform manual segmentation of each slice (16). Manual segmentation remains labor-intensive and prone to human subjectivity, often delaying diagnostic workflows and compromising feature reproducibility (17,18). Semi-automatic methods address these limitations by combining clinician oversight with algorithmic precision, ensuring both efficiency and consistency (19).
DeepLabv3_ResNet50 excels in capturing multi-scale contextual features through its atrous spatial pyramid pooling (ASPP) module, enabling precise boundary delineation of heterogeneous lesions (20,21). However, its computational complexity may limit real-time applications in resource-constrained settings. In contrast, fully convolutional network (FCN)_ResNet50 leverages a fully convolutional architecture to efficiently process entire US images, achieving higher global accuracy but occasionally overlooking subtle texture variations in low-contrast regions (22,23). Both segmentation models have unique strengths and advantages. We evaluate the accuracy of semi-automatic segmentation of US images using both models, as well as their effectiveness in downstream tasks for distinguishing the nature of breast lesions prior to minimally invasive resection. Ultimately, a combined model will be developed to further improve the performance of downstream models. We present this article in accordance with the TRIPOD reporting checklist (available at https://gs.amegroups.com/article/view/10.21037/gs-24-440/rc).
Methods
Study participants
Patients undergoing US-guided Mammotome-assisted minimally invasive resection at the Fifth Affiliated Hospital of Sun Yat-sen University from November 2018 to November 2023 were selected. The inclusion criteria were as follows: (I) completing minimally invasive breast surgery at our hospital; (II) preoperatively underwent US examination within 2 weeks; (III) complete clinical, imaging, and pathological data. The exclusion criteria were as follows: (I) insufficient or lack of US data for US images; (II) malignancy in other parts of the body. Figure 1 shows the binary classification tasks of the study. A total of 773 patients were ultimately included (Figure S1) and divided into a training cohort and a test cohort. Among the 773 patients ultimately enrolled in the study, the non-tumor patients were also stratified into training and validation cohorts in a ratio of 8:2. And the clinical data were reviewed and collected from the electronic medical record system, and Figure 2 displays the study design and pipeline. The sample size estimation for this study was performed using GPower 3.1 software. Based on the research background, we assumed a medium effect size for the relationship between radiomic features and clinical outcomes (Cohen’s d=0.5), with a significance level of 0.05 and statistical power set to 0.8. According to these parameters, GPower estimated that a total sample size of 128 participants would be required. In this retrospective study, 773 patients were included, with 543 tumor patients and 230 non-tumor patients, providing sufficient statistical power for the analysis.
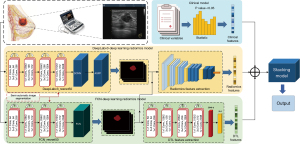
Ethical statement
The study was conducted in accordance with the Declaration of Helsinki (as revised in 2013), and has been approved by the Ethics Review Committee of The Fifth Affiliated Hospital, Sun Yat-sen University (reference number: K271-1). Since this is a retrospective analysis, the requirement for patient’s informed consent was waived.
US acquisition
All patients enrolled underwent preoperative breast US examination using equipment including CHISON Q8 (Nantong, China), TOSHIBA aplio500 (Tokyo, Japan), GE LOGIQ E9 (Boston, USA), Philip EPIQ 7 (Amsterdam, Netherlands) and SIEMENS ACUSON Sequoia (Erlangen, Germany). Typically, patients adopt a supine position and fully expose the breast containing the lesion to capture and store the US images of the breast lesion.
Mammotome-assisted minimally invasive resection guided by US
All minimally invasive surgical procedures were performed using the “EnCor Enspire™ Breast Biopsy System (SenoRX Inc. E4230)”. This system includes a 7G Mammotome biopsy device, control handle, vacuum suction pump, and related software. Patients were placed in a supine or semi-lateral position, with their arm raised. Moderate anesthesia (local anesthesia, 1% lidocaine ≤200 mg) was administered in the planned surgical area, which was the subcutaneous space behind the breast. A 3-mm incision was made in the designated location to insert the 7G Mammotome needle. Through US guidance, the needle was placed in an appropriate position on the deep surface of the breast lesion, ensuring that the lesion was fully within the needle’s groove. Multiple rotational cuts were made to extract lesion tissue until no residual lesion was detected on US images. The surgical area was then subjected to hemostasis. All patients required compression bandages for 72 hours after surgery. Figure 3 displays the surgical procedures.
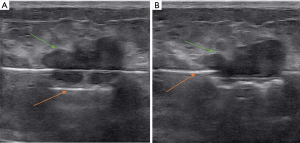
Semi-automatic segmentation of region of interest (ROI)
Two radiologists, with over three years of experience and blinded to the pathology, utilized Picture Archiving and Communication Systems (PACS) to review and select US images of patients for inclusion in the study. We employed a semi-automated image segmentation method, utilizing the labelme assistive software and including DeepLabv3_ResNet50 and FCN_ResNet50 as segmentation models.
Feature extraction and screening
We imported the semi-automatic segmentation results of DeepLabv3_ResNet50 and FCN_ResNet50 into the PyRadiomics platform for radiomics feature extraction. After extracting features, we evaluated the repeatability and stability of radiomics parameters using intraclass correlation coefficients (ICC). We identified highly correlated radiomics features from the t-test with a P value of ≥0.05. Subsequently, we used the Spearman rank correlation test to evaluate the linear correlation between individual features to eliminate redundancy. A previous study reported that features with strong correlation had higher absolute values of their correlation coefficients (24). Additionally, we selected one feature when the Spearman correlation coefficient between each feature was >0.9. Finally, in our previous work, we used least absolute shrinkage and selection operator (LASSO) regression for feature selection, where non-zero coefficients were considered valuable predictors within each feature group.
In this study, we selected ResNet101 as the pre-trained model and performed feature extraction on the dataset (25). The specific network architecture is described in Figure 2. After training the deep learning model, we extracted features from the avgpool layer as deep learning features. As the dimension of the transferred deep features is 2048, we used principal component analysis (PCA) to reduce their dimension and ensure balance between features. To improve the accuracy of the prediction model, we have integrated the radiomics features from the DeepLabv3_ResNet50 pathway and FCN_ResNet50 pathway, as well as 8-dimensional deep transfer learning (DTL) features, to construct a deep learning radiomics model.
Model construction and assessment
Using Python (version 3.12) for model building and evaluation, we selected features for clinical model building based on baseline statistics with P values less than 0.05. We screened and built radiomics features using different machine learning models into DeepLabv3-radiomics and FCN-radiomics models. The signature for building deep learning models were compressed DTL features. Our goal was to create deep learning radiomics labels, build DeepLabv3-deep learning radiomics model and FCN-deep learning radiomics model based on the selected radiomics features and compressed DTL features. Finally, we combined the DeepLabv3-deep learning radiomics model, FCN-deep learning radiomics model, and clinical model in a stacking manner to form the final model.
Statistical analysis
Using the SPSS software package (version 20.0) to evaluate baseline data, continuous variables were described as mean ± standard deviation, and categorical variables were described as frequency and percentage. For continuous variables, comparisons were made using independent sample t-test or Mann-Whitney U test; for categorical variables, comparisons were made using Chi-squared test or Fisher’s exact test. The area under the curve (AUC) was compared using DeLong’s test. A P value <0.05 was considered statistically significant (26). Optimal classification thresholds were determined by maximizing Youden’s index (J = sensitivity + specificity − 1) to ensure clinical relevance.
Results
Characteristics of patients
In this study, we recruited a total of 773 patients, including 543 with breast tumors and 230 with non-breast tumors. The characteristics of all patients are in the Table S1. Significant differences were observed in the clinical features between the two cohorts, including age, maximum diameter, and Breast Imaging Reporting and Data System (BI-RADS) classification. However, there were no significant differences in the tumor location and focus between the training and testing cohorts. After comprehensive multivariate analysis, age, maximum diameter, and BI-RADS classification were ultimately identified as key indicators and were rigorously integrated into the construction of our clinical prediction model. Table S2 presents a summary of characteristics for both the training and testing cohorts among non-breast tumor patients. Following comprehensive multivariate analysis, focus emerged as a crucial factor and was subsequently incorporated into the development of a clinical prediction model.
Results of semi-automatic segmentation
We summarized the training results of the two segmentation models (Figure 4). It was observed that both models achieved high accuracy. Specifically, as shown in Table 1, the DeepLabv3_ResNet50 model attained a global accuracy of 99.4%, an average intersection over union (IoU) of 86.2%, and an average Dice coefficient of 92.0% during the best epoch. Similarly, the FCN_ResNet50 model attained a global accuracy of 99.5%, an average IoU of 88.7%, and an average Dice coefficient of 93.7% during the best epoch. Both segmentation models were trained for 100 epochs.
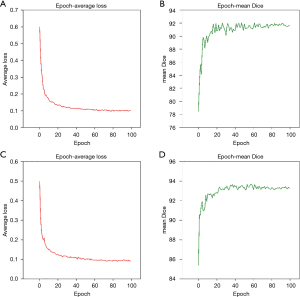
Table 1
Model name | Global_acc, % | MIoU, % | Dice, % | MDice, % | Epoch |
---|---|---|---|---|---|
DeepLabv3 | 99.4 | 86.2 | 84.4–99.7 | 92.0 | 100 |
FCN | 99.5 | 88.7 | 87.6–99.8 | 93.7 | 100 |
MIoU, median intersection over union; FCN, fully convolutional network.
Performance of the radiomics and deep learning models in predicting tumor and non-tumor patients
In this study, we constructed radiomics models and deep learning models using the results of the DeepLabv3 and FCN approaches, respectively (Figure 5). The results showed that the AUC range of the DeepLabv3-radiomics model was 0.716–0.886. The AUC of the DeepLabv3-deep learning model in the training cohorts was 0.839 [95% confidence interval (CI): 0.807–0.872], and in the testing cohorts, it was 0.716 (95% CI: 0.632–0.800). The AUC range of the FCN-radiomics model was 0.729–0.887. The AUC of the FCN-deep learning model in the training cohorts was 0.846 (95% CI: 0.815–0.877), and in the testing cohorts, it was 0.701 (95% CI: 0.609–0.793).
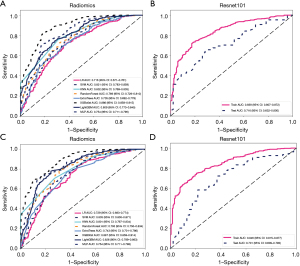
Development and performance of the combined and stacking models in predicting tumor and non-tumor patients
In the subsequent study, we combined deep learning features and radiomics features from different segmentation approaches to construct the DeepLabv3 deep learning radiomics model and FCN deep learning radiomics model. Figure 6 shows the performance of these two models in the testing cohort, with an AUC of 0.659–0.753 for the DeepLabv3 model and an AUC of 0.646–0.733 for the FCN model. The DeepLabv3 model performed slightly better than the FCN model in the testing cohort.
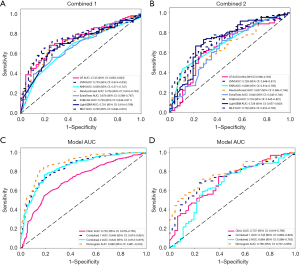
Finally, we utilized stacking to fuse the clinical model, DeepLabv3 deep learning radiomics model, and FCN deep learning radiomics model using logistic regression, resulting in the final stacking model (nomogram). Experimental results in Figure 6 demonstrated that the stacking model, which combined the clinical model, combined 1, and combined 2, significantly improved the ability to differentiate between tumor and non-tumor patients, with an AUC of 0.890 (95% CI: 0.861–0.918). This model demonstrated a sensitivity of 0.844 and a specificity of 0.815. In the testing cohort, the AUC of this stacking model reached 0.780 (95% CI: 0.707–0.853). The sensitivity of the stacking model was 0.713, and the specificity (0.739) was sufficiently high to identify non-tumor patients (Table 2).
Table 2
Model | Threshold | AUC (95% CI) | Sensitivity | Specificity |
---|---|---|---|---|
Stacking model (training) | 0.42 | 0.890 (0.861–0.918) | 0.844 | 0.815 |
Stacking model (testing) | 0.38 | 0.780 (0.707–0.853) | 0.713 | 0.739 |
AUC, area under the curve; CI, confidence interval.
To assess the clinical utility of different prediction models, we evaluated their performance using decision curve analysis (DCA). As shown in the Figure 7, various deep learning radiomics models, including the DeepLabv3 deep learning radiomics model (combined 1), the FCN deep learning radiomics model (combined 2), and the stacking model, were demonstrated in the DCA across the training and testing cohorts. These findings indicate that within the low threshold range (0.2–0.4), the stacking model effectively identifies most patients who require further evaluation or treatment, thereby reducing missed diagnoses. In the intermediate threshold range (0.4–0.6), the model demonstrates a favorable balance between sensitivity and specificity, making it well-suited for clinical decision-making for patients at moderate risk. Conversely, in the high threshold range (>0.6), the stacking model exhibits relatively weak performance for stringent tumor screening, suggesting that additional clinical information may be needed to support decision-making.
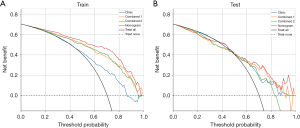
Development and performance of the combined and stacking models in predicting adenosis and other type of lesions
In the prediction task of adenosis and other lesion types, we have developed corresponding DeepLabv3 and FCN deep learning radiomics models. As depicted in Figure 8, the performance of these two models in the testing cohort revealed AUC values ranging from 0.663 to 0.802 for the DeepLabv3 model and 0.677 to 0.737 for the FCN model. The final stacking model effectively distinguished patients with adenosis from those with other lesions, achieving an AUC of 0.813 (95% CI: 0.752–0.873) in the training cohort. The sensitivity and specificity of this model were 0.613 and 0.859, respectively. In the testing cohort, the stacking model exhibited an AUC of 0.771 (95% CI: 0.617–0.924), along with a sensitivity of 0.759 and specificity of 0.765 (Table 3).
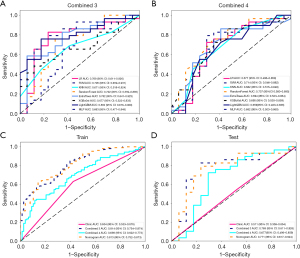
Table 3
Model | Threshold | AUC (95% CI) | Sensitivity | Specificity |
---|---|---|---|---|
Stacking model (training) | 0.35 | 0.813 (0.752–0.873) | 0.613 | 0.859 |
Stacking model (testing) | 0.40 | 0.771 (0.617–0.924) | 0.759 | 0.765 |
AUC, area under the curve; CI, confidence interval.
The DCA curve presented in Figure 9 demonstrated the clinical utility of the DeepLabv3 deep learning radiomics model, the FCN deep learning radiomics model, and the stacking model in both the training and testing cohorts. These findings suggest that in the low threshold range (0.1–0.3), the stacking model serves as an auxiliary tool for the preliminary screening of patients with adenopathy, and it should be used in conjunction with additional clinical information for a comprehensive evaluation. In the intermediate threshold range (0.3–0.6), when establishing a definitive diagnosis, the model effectively balances sensitivity and specificity, thereby reducing both missed and incorrect diagnoses. However, in the high threshold range (>0.6), where a high degree of diagnostic certainty is required, the model’s performance may be insufficient, warranting cautious use.
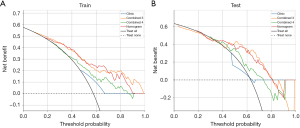
Discussion
With the increase in breast disease screening coverage and public health awareness, the early detection rate of breast lesion has significantly improved (27). Given the presence of breast lesion, more and more patients face psychological pressure, such as cancerophobia, and therefore seek to determine the pathological nature of the lesion and undergo surgical removal (28). Providing appropriate treatment plans is crucial for surgeons. Research has shown that compared to traditional open surgery, Mammotome-assisted minimally invasive resection has significant advantages in terms of skin incision size, intraoperative blood loss, healing time, scar formation, wound infection, and cosmetic outcomes (29,30). Additionally, real-time dynamic monitoring of the lesion through US allows for more precise and complete removal. On the other hand, Mammotome-assisted minimally invasive resection is associated with minimal pain and high patient satisfaction (31). Preoperative assessment before surgery is helpful in achieving precision medicine, providing a basis for physicians to carry out individualized treatment, and significantly alleviating patients’ psychological pressure.
Image segmentation is an important step in radiomics analysis and is crucial for identifying radiomic features. Common image segmentation methods include manual segmentation, semi-automatic segmentation, and automatic segmentation (32). Semi-automatic and automatic segmentation not only simplify the process but also improve repeatability. In recent years, deep learning has emerged as a powerful alternative for supervised segmentation (33-35). DeepLabv3_ResNet50 utilizes a self-attention encoder model structure and combines it with ResNet-50 as a feature extractor (36). It achieves high accuracy and efficiency while maintaining good scalability. FCN is a convolutional neural network used for image segmentation. It performs feature extraction and prediction on images layer by layer in a fully convolutional manner, ultimately obtaining pixel-level segmentation results. The FCN_ResNet50 algorithm also incorporates ResNet-50 as a feature extractor to utilize its excellent feature representation capability. Combined with the fully convolutional approach of FCN, it enables efficient image segmentation (37). The semi-automatic segmentation models used in this study demonstrate high accuracy, with a global accuracy of 99.4% (DeepLabv3_ResNet50) and 99.5% (FCN_ResNet50).
In the task of predicting tumor and non-tumor patients, the stacking model demonstrated impressive discriminatory power in the training cohort, achieving an AUC value of 0.890 (95% CI: 0.861–0.918). When evaluated in the testing cohort, the model maintained a strong performance with an AUC of 0.780 (95% CI: 0.707–0.853). In the task of predicting adenosis and other lesion types, while the clinical model’s predictive performance was suboptimal, the stacking model yielded superior results. Specifically, it achieved an AUC of 0.813 in the training cohort and 0.771 in the testing cohort. Although the clinical model underperformed in predicting adenosis (AUC =0.68), incorporating it into the ensemble improved specificity by 7%. This aligns with a study showing that even weak sub-models can enhance diagnostic robustness through complementary feature spaces (38). Compared with current clinical protocols, the stacking model based on semi-automatic segmentation not only effectively reduces unnecessary biopsies and follow-ups but also significantly decreases the manual annotation time required per case, thereby allowing clinicians to prioritize decision-making for repetitive tasks. Furthermore, the cost savings achieved with this technology enable healthcare institutions to reallocate resources to higher-priority care services, while the effective management of patients’ preoperative anxiety underscores its psychosocial benefits.
Overall, our study demonstrates the efficacy of enhancing model performance through the integration of multiple predictive models. However, further work and research are necessary to confirm the reliability of these findings. The retrospective nature and single-institution data may constrain generalizability. While internal validation demonstrates model robustness under controlled conditions, future prospective, multicenter studies with heterogeneous cohorts are essential to assess real-world adaptability. We must continue to strive for improved research quality to ensure the accuracy and reliability of our findings.
Conclusions
In summary, this study utilized preoperative US images from Mammotome-assisted minimally invasive resections to develop an ensemble learning model employing a semi-automated segmentation approach. This model accurately distinguishes between tumor and non-tumor patients, as well as adenosis and other lesion types, thereby offering valuable insights for individualized treatment planning. The findings underscore the potential of this approach to enhance preoperative diagnostic accuracy and to guide personalized medical interventions. The stacking model demonstrates potential clinical value in accurately differentiating tumor from non-tumor patients and adenosis from other lesions. Future studies should focus on refining this model and expanding its applicability to additional types of breast lesions. Moreover, continuous improvements in research quality will be essential to ensure the clinical utility of these predictive models.
Acknowledgments
We thank the Department of Ultrasound for providing ultrasound images and appreciate the Python Technology providing the OnekeyAI platform, which greatly supports our research.
Footnote
Reporting Checklist: The authors have completed the TRIPOD reporting checklist. Available at https://gs.amegroups.com/article/view/10.21037/gs-24-440/rc
Data Sharing Statement: Available at https://gs.amegroups.com/article/view/10.21037/gs-24-440/dss
Peer Review File: Available at https://gs.amegroups.com/article/view/10.21037/gs-24-440/prf
Funding: None.
Conflicts of Interest: All authors have completed the ICMJE uniform disclosure form (available at https://gs.amegroups.com/article/view/10.21037/gs-24-440/coif). The authors have no conflicts of interest to declare.
Ethical Statement: The authors are accountable for all aspects of the work in ensuring that questions related to the accuracy or integrity of any part of the work are appropriately investigated and resolved. The study was conducted in accordance with the Declaration of Helsinki (as revised in 2013), and has been approved by the Ethics Review Committee of The Fifth Affiliated Hospital, Sun Yat-sen University (reference number: K271-1). Since this is a retrospective analysis, the requirement for patient’s informed consent was waived.
Open Access Statement: This is an Open Access article distributed in accordance with the Creative Commons Attribution-NonCommercial-NoDerivs 4.0 International License (CC BY-NC-ND 4.0), which permits the non-commercial replication and distribution of the article with the strict proviso that no changes or edits are made and the original work is properly cited (including links to both the formal publication through the relevant DOI and the license). See: https://creativecommons.org/licenses/by-nc-nd/4.0/.
References
- Sung H, Ferlay J, Siegel RL, et al. Global Cancer Statistics 2020: GLOBOCAN Estimates of Incidence and Mortality Worldwide for 36 Cancers in 185 Countries. CA Cancer J Clin 2021;71:209-49. [Crossref] [PubMed]
- Harbeck N, Gnant M. Breast cancer. Lancet 2017;389:1134-50. [Crossref] [PubMed]
- Xiong X, Zheng LW, Ding Y, et al. Breast cancer: pathogenesis and treatments. Signal Transduct Target Ther 2025;10:49. [Crossref] [PubMed]
- Ohuchi N, Suzuki A, Sobue T, et al. Sensitivity and specificity of mammography and adjunctive ultrasonography to screen for breast cancer in the Japan Strategic Anti-cancer Randomized Trial (J-START): a randomised controlled trial. Lancet 2016;387:341-8. [Crossref] [PubMed]
- Tadesse GF, Tegaw EM, Abdisa EK. Diagnostic performance of mammography and ultrasound in breast cancer: a systematic review and meta-analysis. J Ultrasound 2023;26:355-67. [Crossref] [PubMed]
- Huang R, Lin Z, Dou H, et al. AW3M: An auto-weighting and recovery framework for breast cancer diagnosis using multi-modal ultrasound. Med Image Anal 2021;72:102137. [Crossref] [PubMed]
- Banys-Paluchowski M, Rubio IT, Karadeniz Cakmak G, et al. Intraoperative Ultrasound-Guided Excision of Non-Palpable and Palpable Breast Cancer: Systematic Review and Meta-Analysis. Ultraschall Med 2022;43:367-79. [Crossref] [PubMed]
- Wang H, Wang Q, Zhang Y, et al. Value of ultrasound BI-RADS classification in preoperative evaluation of the ultrasound-guided Mammotome-assisted minimally invasive resection of breast masses: A retrospective analysis. Exp Ther Med 2023;25:143. [Crossref] [PubMed]
- Trayes KP, Cokenakes SEH. Breast Cancer Treatment. Am Fam Physician 2021;104:171-8.
- Ab Mumin N, Ramli Hamid MT, Wong JHD, et al. Magnetic Resonance Imaging Phenotypes of Breast Cancer Molecular Subtypes: A Systematic Review. Acad Radiol 2022;29:S89-S106. [Crossref] [PubMed]
- Conti A, Duggento A, Indovina I, et al. Radiomics in breast cancer classification and prediction. Semin Cancer Biol 2021;72:238-50. [Crossref] [PubMed]
- Sohn JH, Fields BKK. Radiomics and Deep Learning to Predict Pulmonary Nodule Metastasis at CT. Radiology 2024;311:e233356. [Crossref] [PubMed]
- Tagliafico AS, Piana M, Schenone D, et al. Overview of radiomics in breast cancer diagnosis and prognostication. Breast 2020;49:74-80. [Crossref] [PubMed]
- Yue WY, Zhang HT, Gao S, et al. Predicting Breast Cancer Subtypes Using Magnetic Resonance Imaging Based Radiomics With Automatic Segmentation. J Comput Assist Tomogr 2023;47:729-37. [Crossref] [PubMed]
- Wang X, Xie T, Luo J, et al. Radiomics predicts the prognosis of patients with locally advanced breast cancer by reflecting the heterogeneity of tumor cells and the tumor microenvironment. Breast Cancer Res 2022;24:20. [Crossref] [PubMed]
- Gryska E, Schneiderman J, Björkman-Burtscher I, et al. Automatic brain lesion segmentation on standard magnetic resonance images: a scoping review. BMJ Open 2021;11:e042660. [Crossref] [PubMed]
- Fel JT, Ellis CT, Turk-Browne NB. Automated and manual segmentation of the hippocampus in human infants. Dev Cogn Neurosci 2023;60:101203. [Crossref] [PubMed]
- Li Y, Liu Y, Huang L, et al. Deep weakly-supervised breast tumor segmentation in ultrasound images with explicit anatomical constraints. Med Image Anal 2022;76:102315. [Crossref] [PubMed]
- Wang X, Bao N, Xin X, et al. Automatic evaluation of endometrial receptivity in three-dimensional transvaginal ultrasound images based on 3D U-Net segmentation. Quant Imaging Med Surg 2022;12:4095-108. [Crossref] [PubMed]
- Murugappan M, Bourisly AK, Prakash NB, et al. Automated semantic lung segmentation in chest CT images using deep neural network. Neural Comput Appl 2023;35:15343-64. [Crossref] [PubMed]
- Shia WC, Hsu FR, Dai ST, et al. Semantic Segmentation of the Malignant Breast Imaging Reporting and Data System Lexicon on Breast Ultrasound Images by Using DeepLab v3. Sensors (Basel) 2022;22:5352. [Crossref] [PubMed]
- Gómez-Flores W, Coelho de Albuquerque Pereira W. A comparative study of pre-trained convolutional neural networks for semantic segmentation of breast tumors in ultrasound. Comput Biol Med 2020;126:104036. [Crossref] [PubMed]
- Hsieh YH, Hsu FR, Dai ST, et al. Incorporating the Breast Imaging Reporting and Data System Lexicon with a Fully Convolutional Network for Malignancy Detection on Breast Ultrasound. Diagnostics (Basel) 2021;12:66. [Crossref] [PubMed]
- Akoglu H. User's guide to correlation coefficients. Turk J Emerg Med 2018;18:91-3. [Crossref] [PubMed]
- Toseef M, Olayemi Petinrin O, Wang F, et al. Deep transfer learning for clinical decision-making based on high-throughput data: comprehensive survey with benchmark results. Brief Bioinform 2023;24:bbad254. [Crossref] [PubMed]
- DeLong ER, DeLong DM, Clarke-Pearson DL. Comparing the areas under two or more correlated receiver operating characteristic curves: a nonparametric approach. Biometrics 1988;44:837-45.
- Wang Y, Li Y, Song Y, et al. Comparison of ultrasound and mammography for early diagnosis of breast cancer among Chinese women with suspected breast lesions: A prospective trial. Thorac Cancer 2022;13:3145-51. [Crossref] [PubMed]
- Dinapoli L, Colloca G, Di Capua B, et al. Psychological Aspects to Consider in Breast Cancer Diagnosis and Treatment. Curr Oncol Rep 2021;23:38. [Crossref] [PubMed]
- Johnson AT, Henry-Tillman RS, Smith LF, et al. Percutaneous excisional breast biopsy. Am J Surg 2002;184:550-4; discussion 554. [Crossref] [PubMed]
- Tang X. Mammotome-Assisted Liposuction: A Novel Technique for Accessory Breasts. Aesthetic Plast Surg 2017;41:517-23. [Crossref] [PubMed]
- Chang DH, Shu YL. Clinic efficacy and safety of ultrasound-guided Mammotome-assisted surgery for patients with breast benign tumors. Eur Rev Med Pharmacol Sci 2023;27:5985-92. [Crossref] [PubMed]
- Ilesanmi AE, Chaumrattanakul U, Makhanov SS. Methods for the segmentation and classification of breast ultrasound images: a review. J Ultrasound 2021;24:367-82. [Crossref] [PubMed]
- Wang P, Chen P, Yuan Y, et al. Understanding Convolution for Semantic Segmentation. 2018 IEEE Winter Conference on Applications of Computer Vision (WACV), Lake Tahoe, NV, USA; 2018:1451-60.
- Badrinarayanan V, Kendall A, Cipolla R. SegNet: A Deep Convolutional Encoder-Decoder Architecture for Image Segmentation. IEEE Trans Pattern Anal Mach Intell 2017;39:2481-95. [Crossref] [PubMed]
- Kwak D, Choi J, Lee S. Rethinking Breast Cancer Diagnosis through Deep Learning Based Image Recognition. Sensors (Basel) 2023;23:2307. [Crossref] [PubMed]
- Zhao D, Che NY, Song ZG, et al. Pathological diagnosis of lung cancer based on deep transfer learning. Zhonghua Bing Li Xue Za Zhi 2020;49:1120-5. [Crossref] [PubMed]
- Shelhamer E, Long J, Darrell T. Fully Convolutional Networks for Semantic Segmentation. IEEE Trans Pattern Anal Mach Intell 2017;39:640-51. [Crossref] [PubMed]
- Hägele M, Eschrich J, Ruff L, et al. Leveraging weak complementary labels enhances semantic segmentation of hepatocellular carcinoma and intrahepatic cholangiocarcinoma. Sci Rep 2024;14:24988. [Crossref] [PubMed]