Development and validation of a multidimensional machine learning-based nomogram for predicting central lymph node metastasis in papillary thyroid microcarcinoma
Highlight box
Key findings
• Developed a machine learning-based nomogram integrating clinical, ultrasonographic, and serological data to predict central lymph node metastasis (CLNM) in patients with papillary thyroid microcarcinoma (PTMC).
• Achieved an area under the curve of 0.88 in the training cohort, indicating strong predictive performance
What is known and what is new?
• PTMC is generally considered low-risk, but CLNM is associated with higher recurrence and metastasis risks.
• Existing prediction models often rely on isolated clinical or imaging parameters.
• This study introduces a comprehensive nomogram that combines multiple predictors for enhanced preoperative risk stratification in PTMC patients.
What is the implication, and what should change now?
• The nomogram can guide personalized surgical strategies by accurately assessing CLNM risk.
• Clinicians should consider integrating this tool into preoperative evaluations to inform decisions regarding prophylactic central lymph node dissection.
Introduction
Papillary thyroid carcinoma (PTC) represents the most prevalent malignant neoplasm within the spectrum of thyroid cancer. The clinical course of PTC is typically characterized by a relatively indolent behavior, resulting in a favorable 10-year survival rate exceeding 90% following conventional therapeutic interventions (1,2). Enhanced public health awareness has led to more routine check-ups, enabling early detection of papillary thyroid microcarcinoma (PTMC) and reducing diagnoses after symptoms appear. In addition, the application of high-resolution ultrasound technology has made the detection of microscopic tumors easier and improved the clarity of tumor imaging, thus reducing the risk of misdiagnosis and missed diagnosis. As a result, a larger proportion of thyroid cancer patients are receiving early diagnosis thanks to extensive health checkup promotion and the application of cutting-edge medical examination tools (3). Over the past 40 years, the incidence of thyroid cancer has tripled (4). Although PTMC is slow-growing and generally has a favorable prognosis, there is a high prevalence of lymph node metastasis (LNM), especially central LNM (CLNM), which can be as high as 18.3–50%. This is regarded as a potential risk factor for distant metastasis and recurrence (5).
Patients with preoperatively confirmed LNM typically undergo lateral neck dissection and total thyroidectomy. For patients without detectable nodal involvement (cN0), the use of prophylactic central lymph node dissection (PCLND) remains controversial. European and US guidelines restrict PCLND to patients with advanced stages (T3/T4) or lateral cervical metastasis (cN1b), excluding PTMC (6,7). The 2022 National Comprehensive Cancer Network (NCCN) guidelines endorse active surveillance or thyroid lobectomy alone for cN0 PTMC (8). Moreover, the 2023 Chinese guidelines recommend that surgical management for PTMC should be tailored to each patient based on a thorough risk-benefit evaluation rather than routinely performing PCLND, aiming to reduce recurrence and the need for reoperation given the high incidence of CLNM (9).
Multiple risk factors associated with CLNM in patients with PTMC have been identified in several previous studies (10-13). Clinical features such as younger age, larger tumors, multifocality, and serological markers such as thyroid-stimulating hormone (TSH) levels have been identified as significant predictors. In addition, inflammatory markers [e.g., neutrophil-to-lymphocyte ratio (NLR), platelet-to-lymphocyte ratio (PLR)] have also been explored as potential predictors (14). Based on these factors, several predictive models have been developed for the preoperative assessment of CLNM risk. However, many models rely on dichotomous variables or a limited number of predictors, limiting their ability to fully capture the complexity of tumor behavior. In light of these limitations and considering the ongoing controversy regarding the extent of PCLND in patients with PTMC, the present study used a multidimensional risk assessment methodology integrating clinicopathological, ultrasonographic, and serological data to develop a comprehensive and individualized risk model, which is clinically important for improving prediction accuracy and ultimately assisting in individualized surgical decision-making. We present this article in accordance with the TRIPOD reporting checklist (available at https://gs.amegroups.com/article/view/10.21037/gs-2024-508/rc).
Methods
Study population
There were 595 patients diagnosed with PTMC who underwent thyroid surgery at Liaoyang Central Hospital from January 2020 to December 2023 enrolled in this study. All information was obtained from the electronic medical record system while maintaining patient confidentiality, and nothing that could potentially be employed to identify an individual was revealed. In every case, PTMC and central lymphatic nature were definitively diagnosed by postoperative histopathology.
Inclusion criteria
(I) Tumor diameter ≤1.0 cm; (II) pre-operative imaging and physical examination confirmed no cervical lymph node transplantation; (III) initial operation accompanied by central lymph node dissection; (IV) postoperative pathology clearly shows PTC; (V) have complete clinical and pathological data.
Exclusion criteria
(I) The neck has been treated with radiation; (II) patients with postoperative recurrence or secondary surgery; (III) patients with malignant tumors in other parts of the body.
After excluding 92 patients who did not meet the criteria, a total of 503 patients diagnosed with PTMC were included and randomized into a training set (352 patients) and a validation set (151 patients) at a ratio of 7:3. Figure 1 shows the flowchart for the study design.
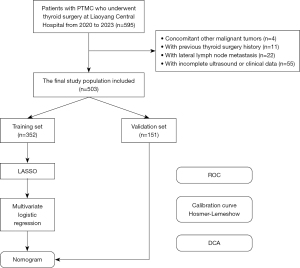
The study was conducted in accordance with the Declaration of Helsinki (as revised in 2013) and approved by the Ethics Committee of Liaoyang Central Hospital (No. 2024080106). Due to the retrospective nature of the study, participant informed consent was waived.
Data collection
Ultrasound features recorded included tumor location, border conditions, microcalcifications, anteroposterior and transverse diameter ratio (A/T), tumor blood supply, echo grade, whether the echoes were homogeneous, cystic solidity, peripheral invasion, and whether the morphology was regular.
Serologic markers include TSH, thyroid peroxidase antibody (TPOAB), NLR, and PLR.
Pathological characterization features included tumor pathology type, maximum tumor diameter, multifocality (tumor foci greater than or equal to two), bilaterality, extrathyroidal extension (ETE), nodular goiter, and CLNM. ETE is characterized as the primary tumor’s invasion of surrounding structures or its expansion through the perithyroid membrane into the soft tissues of the perithyroid, such as the perithyroid fat (15). If at least one of the criteria listed below is satisfied, Hashimoto’s thyroiditis (HT) can be diagnosed: (I) elevated levels of TPOAB; (II) ultrasound features showing diffuse heterogeneity; (III) pathological manifestations characteristic of diffuse lymphocytic thyroiditis (16).
Surgical strategies
If patients had any of the following factors (tumor in the thyroid isthmus, bilateral multifocal), they underwent total thyroidectomy and bilateral central lymph node dissection. Otherwise, patients underwent lobectomy and central lymph node dissection (CLND). All specimens were sent to the Department of Pathology for paraffin fixation and histological analysis.
Statistical analysis
Data were analyzed using SPSS version 26 and R software (version 4.2.2). Normally distributed continuous variables were presented as mean ± standard deviation (SD), the median and interquartile range (IQR) were used to show variables that were not regularly distributed. Frequencies and percentages were employed to express categorical variables. For continuous variables that were normally distributed, the t-test was employed, and for variables that were not normally distributed, the Mann-Whitney U test. Proportional data were analyzed using either the Chi-squared test (χ²) or the Fisher’s exact test, depending on the size and distribution of the sample. P values less than 0.05 were considered statistically significant. Our dataset was randomly split into two cohorts: 70% for the training group and 30% for the validation group. To mitigate confounding and prevent model overfitting, least absolute shrinkage and selection operator (LASSO) regression identified optimal variables, which were subsequently used in multiple logistic regression to establish a risk model for CLNM (17). We evaluated the predictive performance of our model using receiver operating characteristic (ROC) curves, calibration plots, and the area under the ROC curve (AUC), also known as the c-index, to assess model consistency. The c-index quantified discriminatory ability. Decision curve analysis (DCA) was used to assess the clinical usefulness of the model within the intervention.
Results
Dividing the training and validation cohort
In this study, 503 patients were included for analysis, with random division into a training set (n=352) and a test set (n=151) in a 7:3 ratio based on clinical and pathological findings (Table 1). Comparisons of clinicopathologic, ultrasonographic features, and serologic indices between the training and validation sets showed no significant differences (P>0.05), affirming their suitability for training and validation purposes.
Table 1
Variables | Total (n=503) | Train (n=352) | Test (n=151) | Statistic | P |
---|---|---|---|---|---|
CLNM | χ2=0.01 | 0.91 | |||
No | 358 (71.17) | 250 (71.02) | 108 (71.52) | ||
Yes | 145 (28.83) | 102 (28.98) | 43 (28.48) | ||
Gender | χ2=0.20 | 0.65 | |||
Male | 120 (23.86) | 82 (23.30) | 38 (25.17) | ||
Female | 383 (76.14) | 270 (76.70) | 113 (74.83) | ||
Age (years) | 48.50±10.07 | 48.39±9.82 | 48.78±10.66 | t=0.40 | 0.69 |
Tumor diameter (cm) | 0.60 (0.50, 0.80) | 0.60 (0.50, 0.80) | 0.70 (0.40, 0.80) | Z=−0.23 | 0.82 |
Location | χ2=2.15 | 0.54 | |||
Upper | 108 (21.47) | 73 (20.74) | 35 (23.18) | ||
Middle | 197 (39.17) | 139 (39.49) | 58 (38.41) | ||
Lower | 133 (26.44) | 90 (25.57) | 43 (28.48) | ||
Isthmus | 65 (12.92) | 50 (14.20) | 15 (9.93) | ||
Multifocality | χ2=0.75 | 0.39 | |||
No | 376 (74.75) | 267 (75.85) | 109 (72.19) | ||
Yes | 127 (25.25) | 85 (24.15) | 42 (27.81) | ||
Bilaterality | χ2=1.47 | 0.23 | |||
No | 389 (77.34) | 267 (75.85) | 122 (80.79) | ||
Yes | 114 (22.66) | 85 (24.15) | 29 (19.21) | ||
A/T ≥1 | χ2=1.12 | 0.29 | |||
Yes | 367 (72.96) | 252 (71.59) | 115 (76.16) | ||
No | 136 (27.04) | 100 (28.41) | 36 (23.84) | ||
Irregular shape | χ2=0.89 | 0.35 | |||
Yes | 168 (33.40) | 113 (32.10) | 55 (36.42) | ||
No | 335 (66.60) | 239 (67.90) | 96 (63.58) | ||
Echotexture | χ2=2.49 | 0.12 | |||
Homogeneous | 283 (56.26) | 190 (53.98) | 93 (61.59) | ||
Heterogeneous | 220 (43.74) | 162 (46.02) | 58 (38.41) | ||
Echoic | χ2=5.22 | 0.07 | |||
Hypo | 452 (89.86) | 321 (91.19) | 131 (86.75) | ||
Iso | 25 (4.97) | 18 (5.11) | 7 (4.64) | ||
Hyper | 26 (5.17) | 13 (3.69) | 13 (8.61) | ||
Microcalcification | χ2=0.08 | 0.78 | |||
No | 433 (86.08) | 304 (86.36) | 129 (85.43) | ||
Yes | 70 (13.92) | 48 (13.64) | 22 (14.57) | ||
Margin | χ2=0.00 | 0.95 | |||
Clear | 191 (37.97) | 134 (38.07) | 57 (37.75) | ||
Unclear | 312 (62.03) | 218 (61.93) | 94 (62.25) | ||
Capsular invasion | χ2=0.72 | 0.40 | |||
No | 391 (77.73) | 270 (76.70) | 121 (80.13) | ||
Yes | 112 (22.27) | 82 (23.30) | 30 (19.87) | ||
CDFI blood flow | χ2=0.04 | 0.85 | |||
No | 481 (95.63) | 337 (95.74) | 144 (95.36) | ||
Yes | 22 (4.37) | 15 (4.26) | 7 (4.64) | ||
Composition | – | 0.84 | |||
Cyst | 10 (1.99) | 7 (1.99) | 3 (1.99) | ||
Cyst-solid | 486 (96.62) | 339 (96.31) | 147 (97.35) | ||
Solid | 7 (1.39) | 6 (1.70) | 1 (0.66) | ||
Nodular goiter | χ2=2.29 | 0.13 | |||
No | 229 (45.53) | 168 (47.73) | 61 (40.40) | ||
Yes | 274 (54.47) | 184 (52.27) | 90 (59.60) | ||
ETE | χ2=1.25 | 0.26 | |||
No | 401 (79.72) | 276 (78.41) | 125 (82.78) | ||
Yes | 102 (20.28) | 76 (21.59) | 26 (17.22) | ||
HT | χ2=0.01 | 0.94 | |||
No | 362 (71.97) | 253 (71.88) | 109 (72.19) | ||
Yes | 141 (28.03) | 99 (28.12) | 42 (27.81) | ||
TSH (μIU/mL) | 1.54 (0.97, 2.17) | 1.55 (0.97, 2.17) | 1.50 (0.89, 2.19) | Z=−0.26 | 0.80 |
NLR | 2.06 (1.57, 2.66) | 2.07 (1.60, 2.69) | 1.99 (1.55, 2.65) | Z=−0.60 | 0.55 |
PLR | 135.23 (107.29, 170.42) | 137.07 (107.64, 173.28) | 133.94 (105.11, 167.17) | Z=−0.87 | 0.39 |
Continuous variables that follow a normal distribution are typically presented as mean ± standard deviation. For continuous variables that do not follow a normal distribution, the median and interquartile range are used. Categorical variables are expressed as n (%). t, t-test; Z, Mann-Whitney test; χ2, Chi-square test; –, Fisher exact. CLNM, central lymph node metastasis; A/T, anteroposterior and transverse diameter ratio; CDFI, color Doppler flow imaging; ETE, extrathyroidal extension; HT, Hashimoto’s thyroiditis; TSH, thyroid-stimulating hormone; NLR, neutrophil-to-lymphocyte ratio; PLR, platelet-to-lymphocyte ratio.
Fundamental characteristic
Table 2 shows a comparison of the basic characteristics of the lymph node positive and negative groups in the training group. The study included 82 males (23.3%) and 270 females (76.7%), with a mean age of 48.39±9.82 years. Patients in the CLNM group were significantly younger compared to those in the non-CLNM group (P<0.001), and there was a higher proportion of male patients in the CLNM group (P<0.001).
Table 2
Variables | Total (n=352) | CLNM (−) (n=250) | CLNM (+) (n=102) | Statistic | P |
---|---|---|---|---|---|
Gender | χ2=28.59 | <0.001 | |||
Male | 82 (23.30) | 39 (15.60) | 43 (42.16) | ||
Female | 270 (76.70) | 211 (84.40) | 59 (57.84) | ||
Age (years) | 48.39±9.82 | 49.99±9.40 | 44.45±9.76 | t=4.96 | <0.001 |
Tumor diameter (cm) | 0.60 (0.50, 0.80) | 0.60 (0.40, 0.80) | 0.70 (0.60, 1.00) | Z=−4.85 | <0.001 |
Location | χ2=34.33 | <0.001 | |||
Upper | 73 (20.74) | 55 (22.00) | 18 (17.65) | ||
Middle | 139 (39.49) | 113 (45.20) | 26 (25.49) | ||
Lower | 90 (25.57) | 63 (25.20) | 27 (26.47) | ||
Isthmus | 50 (14.20) | 19 (7.60) | 31 (30.39) | ||
Multifocality | χ2=6.62 | 0.01 | |||
No | 267 (75.85) | 199 (79.60) | 68 (66.67) | ||
Yes | 85 (24.15) | 51 (20.40) | 34 (33.33) | ||
Bilaterality | χ2=5.28 | 0.02 | |||
No | 267 (75.85) | 198 (79.20) | 69 (67.65) | ||
Yes | 85 (24.15) | 52 (20.80) | 33 (32.35) | ||
A/T ≥1 | χ2=1.10 | 0.30 | |||
Yes | 252 (71.59) | 183 (73.20) | 69 (67.65) | ||
No | 100 (28.41) | 67 (26.80) | 33 (32.35) | ||
Irregular shape | χ2=2.88 | 0.09 | |||
Yes | 113 (32.10) | 87 (34.80) | 26 (25.49) | ||
No | 239 (67.90) | 163 (65.20) | 76 (74.51) | ||
Echotexture | χ2=0.06 | 0.80 | |||
Homogeneous | 190 (53.98) | 136 (54.40) | 54 (52.94) | ||
Heterogeneous | 162 (46.02) | 114 (45.60) | 48 (47.06) | ||
Echoic | χ2=0.03 | 0.98 | |||
Hypo | 321 (91.19) | 228 (91.20) | 93 (91.18) | ||
Iso | 18 (5.11) | 13 (5.20) | 5 (4.90) | ||
Hyper | 13 (3.69) | 9 (3.60) | 4 (3.92) | ||
Microcalcification | χ2=0.00 | 0.98 | |||
No | 304 (86.36) | 216 (86.40) | 88 (86.27) | ||
Yes | 48 (13.64) | 34 (13.60) | 14 (13.73) | ||
Margin | χ2=0.28 | 0.60 | |||
Clear | 134 (38.07) | 93 (37.20) | 41 (40.20) | ||
Unclear | 218 (61.93) | 157 (62.80) | 61 (59.80) | ||
Capsular invasion | χ2=3.53 | 0.06 | |||
No | 270 (76.70) | 185 (74.00) | 85 (83.33) | ||
Yes | 82 (23.30) | 65 (26.00) | 17 (16.67) | ||
CDFI blood flow | χ2=0.45 | 0.50 | |||
No | 337 (95.74) | 241 (96.40) | 96 (94.12) | ||
Yes | 15 (4.26) | 9 (3.60) | 6 (5.88) | ||
Composition | – | 0.39 | |||
Cyst | 7 (1.99) | 5 (2.00) | 2 (1.96) | ||
Cyst-solid | 339 (96.31) | 239 (95.60) | 100 (98.04) | ||
Solid | 6 (1.70) | 6 (2.40) | 0 (0.00) | ||
Nodular goiter | χ2=2.96 | 0.09 | |||
No | 168 (47.73) | 112 (44.80) | 56 (54.90) | ||
Yes | 184 (52.27) | 138 (55.20) | 46 (45.10) | ||
ETE | χ2=88.67 | <0.001 | |||
No | 276 (78.41) | 229 (91.60) | 47 (46.08) | ||
Yes | 76 (21.59) | 21 (8.40) | 55 (53.92) | ||
HT | χ2=0.49 | 0.48 | |||
No | 253 (71.88) | 177 (70.80) | 76 (74.51) | ||
Yes | 99 (28.12) | 73 (29.20) | 26 (25.49) | ||
TSH (μIU/mL) | 1.55 (0.97, 2.17) | 1.55 (0.99, 2.17) | 1.52 (0.97, 2.19) | Z=−0.55 | 0.58 |
NLR | 2.07 (1.60, 2.69) | 2.07 (1.63, 2.64) | 1.99 (1.56, 2.88) | Z=−0.59 | 0.56 |
PLR | 137.07 (107.64, 173.28) | 132.43 (107.29, 169.67) | 148.58 (108.51, 188.16) | Z=−1.57 | 0.12 |
Continuous variables that follow a normal distribution are typically presented as mean ± standard deviation. For continuous variables that do not follow a normal distribution, the median and interquartile range are used. Categorical variables are expressed as n (%). t, t-test; Z, Mann-Whitney test; χ2, Chi-square test; –, Fisher exact. CLNM, central lymph node metastasis; A/T, anteroposterior and transverse diameter ratio; CDFI, color Doppler flow imaging; ETE, extrathyroidal extension; HT, Hashimoto’s thyroiditis; TSH, thyroid-stimulating hormone; NLR, neutrophil-to-lymphocyte ratio; PLR, platelet-to-lymphocyte ratio.
In terms of ultrasound characteristics, there were statistically significant differences in tumor location, multiplicity, and bilaterality, with all P values less than 0.05. Tumor diameter was greater in the CLNM group than in the non-CLNM group (P<0.001). Pathologic results indicated a significantly higher incidence of ETE in the CLNM group compared to the non-CLNM group (P<0.001). Preoperative serologic results, including TSH levels and inflammatory markers such as PLR and NLR, did not differ significantly between the two groups.
Feature screening
Clinical characteristics were selected using the LASSO binary logistic regression model. LASSO regression was performed on all available variables, including clinicopathological, ultrasound characteristics, and serological indices, and combined with 10-fold cross-validation to determine the optimal regularization parameter (λ). The selection of λ followed a standard error rule that was used to minimize cross-validation error, thus ensuring a balance between model simplicity and predictive accuracy (Figure 2). When the optimal λ value was 0.051, five variables: age, gender, maximum tumor diameter, tumor location, and ETE were found to be significantly associated with CNLM. These five risk factors entered multifactorial logistic regression analysis (Table 3).
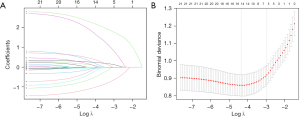
Table 3
Variables | β | SE | P | OR (95% CI) |
---|---|---|---|---|
Age (years) | −0.07 | 0.02 | <0.001 | 0.94 (0.90–0.97) |
Gender | ||||
Male | 1.00 (reference) | |||
Female | −1.22 | 0.34 | <0.001 | 0.30 (0.15–0.57) |
Tumor diameter (cm) | 2.22 | 0.65 | <0.001 | 9.17 (2.54–33.08) |
Location | ||||
Upper | 1.00 (reference) | |||
Middle | −0.41 | 0.42 | 0.33 | 0.66 (0.29–1.51) |
Lower | 0.15 | 0.44 | 0.73 | 1.16 (0.49–2.72) |
Isthmus | 1.23 | 0.5 | 0.01 | 3.41 (1.29–9.04) |
ETE | ||||
No | 1.00 (reference) | |||
Yes | 2.51 | 0.35 | <0.001 | 12.25 (6.11–24.56) |
β, coefficient of regression; SE, standard error; OR, odds ratio; CI, confidence interval; ETE, extrathyroidal extension.
Predictive modeling and validation
By integrating the outcomes of multifactor logistic regression analysis, we developed a predictive model and represented the findings in a nomogram, which enhances the intuitive understanding of the model’s predictive capabilities (Figure 3). ROC and calibration curves were subsequently employed to evaluate the predictive performance. Figure 4 illustrates that the training group’s AUC was 0.88, and the validation group’s was 0.78. In the training group, the model’s c-index was 0.88 [95% confidence interval (CI): 0.83–0.92], and in the validation group, it was 0.78 (95% CI: 0.69–0.87). The sensitivity and specificity for the training group were 0.84 and 0.76; in the validation group, they were 0.80 and 0.70. The reliability and utility of the nomogram were assessed using calibration curves (Figure 5), which indicated excellent agreement between predicted and actual probabilities of CLNM in both training and validation sets, with all mean absolute errors less than 0.05. Clinical DCA curves (Figure 6) demonstrated that the nomogram provided optimal net benefit for predicting CLNM for PTMC when the threshold probability ranged from 15% to 88%.
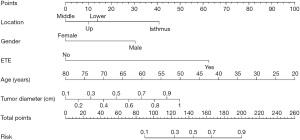
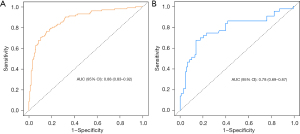
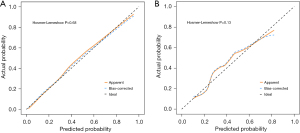
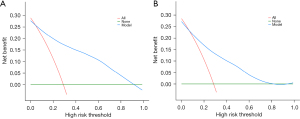
Discussion
Consistent with extensive previous research (18-22), gender, age, ETE, and tumor diameter are associated with an increased risk of LNM in PTMC patients. Although PTMC incidence is higher in females, males are more prone to LNM, possibly due to the dual effects of estrogen or other reproductive-related factors on tumor progression (23,24). ETE is often suggestive of higher aggressiveness, and its presence is associated with extensive LNM, which is an important guide to the clinician’s choice of surgical scope (18,25). Age and tumor size are significant risk factors for LNM in PTMC patients. The model we constructed in this study has a more detailed classification of risk factors such as age, tumor size, and tumor location than many previous studies, which only dichotomized these factors, such as age >55 or <55 years, tumor diameter >0.5 or <0.5 cm, tumor location in the middle-upper pole or middle-lower pole, and did not even consider the specificity of isthmus tumors. This is detrimental to individualized treatment and lacks the precision to guide decision-making, and the model we constructed compensates for the shortcomings of previous studies (19,21,26,27). We observed a 12.92% incidence of lesions in the isthmus, similar to the results of a previous study (10%) (28,29). However, the low incidence showed a higher rate of lymphatic metastasis, a finding that may be due to the anatomical characteristics of the isthmus and its potential invasiveness. This is due to the relatively small size of the thyroid isthmus, which favors the invasion of lesions into adjacent tissues. In addition, lymphatic drainage from the isthmus is more likely to reach the anterior laryngeal and paratracheal lymph nodes before spreading to the paratracheal lymph nodes (30,31).
Inflammation can promote tumor growth, invasion, angiogenesis, and metastasis. Recent research emphasizes the role of inflammatory markers in cancer progression. Numerous studies have shown that increased NLR and PLR are independent prognostic factors in pancreatic and colorectal cancers (32). However, a recent meta-analysis found no significant association between these ratios and prognosis in thyroid cancer patients (33). The role of inflammatory markers in thyroid microcarcinoma remains uncertain, particularly in predicting CLNM in PTMC preoperatively. Our study investigated PLR and NLR as potential predictors but did not identify a statistically significant association with CLNM occurrence, suggesting the need for further extensive prospective studies to confirm these findings. In addition, unlike previous studies, we did not find factors such as TSH, multifocality, and HT to be associated with CLNM, which may be due to differences in small sample sizes in a single center.
In summary, by integrating clinicopathological, ultrasound characteristics, and serological indexes, we investigated risk factors for CLNM in cN0 PTMC patients and developed a predictive model. This model is crucial for guiding preoperative surgical strategies in PTMC patients. The AUC was 0.88 in the training set and 0.78 in the validation set, demonstrating strong predictive performance. According to the calibration curves, there was a mean error of less than 0.05 between the predicted probability of the model and the actual probability of CLNM in patients with thyroid cancer, and the calibration curves, generated using the Bootstrap method (B=1,000 times), indicated good fit and consistency (Hosmer-Lemeshow goodness-of-fit test, P=0.68) between predicted and observed probabilities. We evaluated the clinical utility of the nomogram using clinical decision curves and discovered that, within a specific range of threshold probabilities (training set, 15–88%), it can enhance clinical decision-making and benefit patients. While the nomogram has demonstrated excellent performance, more validation in other clinical situations and demographics is necessary to verify its generalizability and robustness.
Several limitations need to be noted regarding the present study. Firstly, being a retrospective observational study, it could not eliminate confounding factors. Secondly, it was conducted exclusively in a tertiary care hospital, utilizing a model that lacked external validation and potentially carried regional limitations. Thirdly, when information about other nodules was not available, in multifocal nodule cases, just the features of the largest lesion were examined. Fourthly, the observed association between inflammatory factors PLR and NLR with CLNM of PTMC indicates the necessity for further large-sample prospective studies to validate these.
Conclusions
This machine learning-based predictive nomogram provides a reliable tool for assessing CLNM risk in PTMC patients, supporting personalized surgical strategies. Further validation in external cohorts is required to confirm its generalizability.
Acknowledgments
None.
Footnote
Reporting Checklist: The authors have completed the TRIPOD reporting checklist. Available at https://gs.amegroups.com/article/view/10.21037/gs-2024-508/rc
Data Sharing Statement: Available at https://gs.amegroups.com/article/view/10.21037/gs-2024-508/dss
Peer Review File: Available at https://gs.amegroups.com/article/view/10.21037/gs-2024-508/prf
Funding: None.
Conflicts of Interest: All authors have completed the ICMJE uniform disclosure form (available at https://gs.amegroups.com/article/view/10.21037/gs-2024-508/coif). The authors have no conflicts of interest to declare.
Ethical Statement: The authors are accountable for all aspects of the work in ensuring that questions related to the accuracy or integrity of any part of the work are appropriately investigated and resolved. The study was conducted in accordance with the Declaration of Helsinki (as revised in 2013) and approved by the Ethics Committee of Liaoyang Central Hospital (No. 2024080106). Due to the retrospective nature of the study, participant informed consent was waived.
Open Access Statement: This is an Open Access article distributed in accordance with the Creative Commons Attribution-NonCommercial-NoDerivs 4.0 International License (CC BY-NC-ND 4.0), which permits the non-commercial replication and distribution of the article with the strict proviso that no changes or edits are made and the original work is properly cited (including links to both the formal publication through the relevant DOI and the license). See: https://creativecommons.org/licenses/by-nc-nd/4.0/.
References
- Chen DW, Lang BHH, McLeod DSA, et al. Thyroid cancer. Lancet 2023;401:1531-44. [Crossref] [PubMed]
- Bray F, Laversanne M, Sung H, et al. Global cancer statistics 2022: GLOBOCAN estimates of incidence and mortality worldwide for 36 cancers in 185 countries. CA Cancer J Clin 2024;74:229-63. [Crossref] [PubMed]
- Li M, Dal Maso L, Vaccarella S. Global trends in thyroid cancer incidence and the impact of overdiagnosis. Lancet Diabetes Endocrinol 2020;8:468-70. [Crossref] [PubMed]
- Boucai L, Zafereo M, Cabanillas ME. Thyroid Cancer: A Review. JAMA 2024;331:425-35. [Crossref] [PubMed]
- Wen X, Jin Q, Cen X, et al. Clinicopathologic predictors of central lymph node metastases in clinical node-negative papillary thyroid microcarcinoma: a systematic review and meta-analysis. World J Surg Oncol 2022;20:106. [Crossref] [PubMed]
- Haugen BR, Alexander EK, Bible KC, et al. 2015 American Thyroid Association Management Guidelines for Adult Patients with Thyroid Nodules and Differentiated Thyroid Cancer: The American Thyroid Association Guidelines Task Force on Thyroid Nodules and Differentiated Thyroid Cancer. Thyroid 2016;26:1-133. [Crossref] [PubMed]
- Filetti S, Durante C, Hartl D, et al. Thyroid cancer: ESMO Clinical Practice Guidelines for diagnosis, treatment and follow-up†. Ann Oncol 2019;30:1856-83. [Crossref] [PubMed]
- Haddad RI, Bischoff L, Ball D, et al. Thyroid Carcinoma, Version 2.2022, NCCN Clinical Practice Guidelines in Oncology. J Natl Compr Canc Netw 2022;20:925-51. [Crossref] [PubMed]
- Chinese Society of Endocrinology; Thyroid and Metabolism Surgery Group of the Chinese Society of Surgery; China Anti-Cancer Association, Chinese Association of Head and Neck Oncology, et al. Guidelines for the diagnosis and management of thyroid nodules and differentiated thyroid cancer (Second edition). Chinese Journal of Endocrinology and Metabolism 2023;39:181-226.
- Zheng H, Lai V, Lu J, et al. Clinical Factors Predictive of Lymph Node Metastasis in Thyroid Cancer Patients: A Multivariate Analysis. J Am Coll Surg 2022;234:691-700. [Crossref] [PubMed]
- Kang SK, Kim DI, Im DW, et al. A retrospective study of factors affecting contralateral central-neck lymph node metastasis in unilateral papillary thyroid carcinoma. Asian J Surg 2023;46:3485-90. [Crossref] [PubMed]
- Liu W, Zhang D, Jiang H, et al. Prediction model of cervical lymph node metastasis based on clinicopathological characteristics of papillary thyroid carcinoma: a dual-center retrospective study. Front Endocrinol (Lausanne) 2023;14:1233929. [Crossref] [PubMed]
- Gao Y, Tian M, Hou X, et al. Multifocality increases the risk of central compartment lymph node metastasis but is not related to the risk of recurrence and death in papillary thyroid carcinoma. Gland Surg 2024;13:2383-94. [Crossref] [PubMed]
- Cao J, He X, Li X, et al. The potential association of peripheral inflammatory biomarkers in patients with papillary thyroid cancer before radioiodine therapy to clinical outcomes. Front Endocrinol (Lausanne) 2023;14:1253394. [Crossref] [PubMed]
- Tuttle RM, Haugen B, Perrier ND. Updated American Joint Committee on Cancer/Tumor-Node-Metastasis Staging System for Differentiated and Anaplastic Thyroid Cancer (Eighth Edition): What Changed and Why? Thyroid 2017;27:751-6.
- Ragusa F, Fallahi P, Elia G, et al. Hashimotos' thyroiditis: Epidemiology, pathogenesis, clinic and therapy. Best Pract Res Clin Endocrinol Metab 2019;33:101367. [Crossref] [PubMed]
- Tay JK, Narasimhan B, Hastie T. Elastic Net Regularization Paths for All Generalized Linear Models J Stat Softw 2023;106:1. [Crossref] [PubMed]
- Zhan H, Hong Y, Zhang L, et al. Impact of location and size of minimal extrathyroidal extension on lymph node metastasis in papillary thyroid cancer: a retrospective analysis. Gland Surg 2024;13:1619-27. [Crossref] [PubMed]
- Sun J, Jiang Q, Wang X, et al. Nomogram for Preoperative Estimation of Cervical Lymph Node Metastasis Risk in Papillary Thyroid Microcarcinoma. Front Endocrinol (Lausanne) 2021;12:613974. [Crossref] [PubMed]
- Qiu P, Guo Q, Pan K, et al. Development of a nomogram for prediction of central lymph node metastasis of papillary thyroid microcarcinoma. BMC Cancer 2024;24:235. [Crossref] [PubMed]
- Zhang Q, Wang Z, Meng X, et al. Predictors for central lymph node metastases in CN0 papillary thyroid microcarcinoma (mPTC): A retrospective analysis of 1304 cases. Asian J Surg 2019;42:571-6. [Crossref] [PubMed]
- Chen Z, Wang JJ, Du JB, et al. Development and validation of a dynamic nomogram for predicting central lymph node metastasis in papillary thyroid carcinoma patients based on clinical and ultrasound features. Quant Imaging Med Surg 2025;15:1555-70. [Crossref] [PubMed]
- Hu S, Wu X, Jiang H. Trends and projections of the global burden of thyroid cancer from 1990 to 2030. J Glob Health 2024;14:04084. [Crossref] [PubMed]
- Suteau V, Munier M, Briet C, et al. Sex Bias in Differentiated Thyroid Cancer. Int J Mol Sci 2021;22:12992. [Crossref] [PubMed]
- Wang H, Zhao S, Yao J, et al. Factors influencing extrathyroidal extension of papillary thyroid cancer and evaluation of ultrasonography for its diagnosis: a retrospective analysis. Sci Rep 2023;13:18344. [Crossref] [PubMed]
- Wang Y, Guan Q, Xiang J. Nomogram for predicting central lymph node metastasis in papillary thyroid microcarcinoma: A retrospective cohort study of 8668 patients. Int J Surg 2018;55:98-102. [Crossref] [PubMed]
- Feng JW, Ye J, Wu WX, et al. Management of cN0 papillary thyroid microcarcinoma patients according to risk-scoring model for central lymph node metastasis and predictors of recurrence. J Endocrinol Invest 2020;43:1807-17. [Crossref] [PubMed]
- Jasim S, Baranski TJ, Teefey SA, et al. Investigating the Effect of Thyroid Nodule Location on the Risk of Thyroid Cancer. Thyroid 2020;30:401-7. [Crossref] [PubMed]
- Campennì A, Ruggeri RM, Siracusa M, et al. Isthmus topography is a risk factor for persistent disease in patients with differentiated thyroid cancer. Eur J Endocrinol 2021;185:397-404. [Crossref] [PubMed]
- Karatzas T, Charitoudis G, Vasileiadis D, et al. Surgical treatment for dominant malignant nodules of the isthmus of the thyroid gland: A case control study. Int J Surg 2015;18:64-8. [Crossref] [PubMed]
- Lyu YS, Pyo JS, Cho WJ, et al. Clinicopathological Significance of Papillary Thyroid Carcinoma Located in the Isthmus: A Meta-Analysis. World J Surg 2021;45:2759-68. [Crossref] [PubMed]
- Mei Z, Shi L, Wang B, et al. Prognostic role of pretreatment blood neutrophil-to-lymphocyte ratio in advanced cancer survivors: A systematic review and meta-analysis of 66 cohort studies. Cancer Treat Rev 2017;58:1-13. [Crossref] [PubMed]
- Russo E, Guizzardi M, Canali L, et al. Preoperative systemic inflammatory markers as prognostic factors in differentiated thyroid cancer: a systematic review and meta-analysis. Rev Endocr Metab Disord 2023;24:1205-16. [Crossref] [PubMed]