Intraoperative parathyroid gland recognition prediction model and key feature analysis based on white light images
Highlight box
Key findings
• This study successfully established and validated a convenient and economical intraoperative parathyroid gland identification model through white light images.
What is known and what is new?
• Timely identification and protection of the parathyroid glands during thyroid surgery are important.
• By extracting and selecting of subtle texture features that cannot be recognized by the naked eye from white light images, a machine learning based model can be constructed to identify the parathyroid gland.
What is the implication, and what should change now?
• Improving the intraoperative identification of the parathyroid gland is of great significance for thyroid surgery. This study offers a brand-new approach for the intraoperative identification of the parathyroid gland. Further research should be conducted to incorporate more data from multiple centers and establish a more practical and robust prediction model.
Introduction
The parathyroid gland is an essential endocrine organ, and its secretion of parathyroid hormone is highly important for maintaining the blood calcium balance (1-3). Protecting the parathyroid glands during thyroid surgery is an extremely crucial step (4). If the parathyroid glands are damaged, it may result in hypocalcemia, potentially causing lifelong hypocalcemia and, in severe cases, symptoms such as tetany (5). These injuries pose a serious threat to patients’ quality of life. Therefore, timely identification and protection of the parathyroid glands during thyroid surgery are highly important (4-6).
During the operation, it is easy to confuse parathyroid glands with some atypical lymph nodes, fat granules, some thymus tissues and atypical thyroid nodules. At present, the following methods are used to assist in the identification of parathyroid glands during surgery (7-12). First, most clinicians identify parathyroid glands based on visual inspection. Clinicians rely on the fact that parathyroid glands are generally brown or tan in color and soft in texture, have a regular shape and smooth surface, and have a complete capsule (7,8). This method is not suitable for physicians with insufficient experience. Suspicious parathyroid tissue is occasionally cut, sent for intraoperative freezing and then transplanted into the muscle after confirmation (9). Second, most intraoperative identification techniques require the input of exogenous substances into the human body. Negative developers such as nanocarbons (10) incur additional costs and are difficult to metabolize in the body. Positive developers such as indocyanine green (ICG) (11) may have the problems of low specificity and limited development time. In addition, regardless of the type of contrast agent, the development effect is easily affected by the local blood supply and scarring.
Research on near-infrared light-based devices for parathyroid gland identification has undergone rapid development in recent years (12). This is a noninvasive and reproducible intraoperative identification technique for parathyroid gland surgery; however, it still requires the purchase of expensive additional equipment and proficiency in its use.
Therefore, the above-mentioned parathyroid gland identification methods remain relatively expensive or time-consuming. For patients with better economic conditions, these devices are affordable. However, those with poorer economic conditions seek a convenient, efficient, and inexpensive method of parathyroid gland identification. This study aims to establish and validate a method for identifying parathyroid glands exclusively using white light photographs. By taking photos of suspected parathyroid tissue during surgery, extracting texture features using radiomic techniques, and further establishing and validating machine learning models, it is possible to identify and infer whether the tissue is parathyroid. We present this article in accordance with the TRIPOD reporting checklist (available at https://gs.amegroups.com/article/view/10.21037/gs-2024-522/rc).
Methods
Study subjects and data collection
This is a prospective, preliminary study. Data from patients who underwent thyroid lobectomy or total thyroidectomy for benign or malignant tumors in Beijing Tongren Hospital affiliated with Capital Medical University from January 1, 2023, to September 1, 2024, were collected. During the operation, ordinary cameras were used to take photos of parathyroid tissue and suspected parathyroid tissue, such as suspicious lymph nodes, fat granules, atypical thyroid nodules, and thymus tissue. The photos of the parathyroid glands taken in this study were all captured in the context of thyroid tissue, specifically by focusing directly within the surgical cavity. The specific information of the camera is as follows: model, Canon EOS 70D; aperture value, f/5; exposure time, 1/160 seconds; ISO, 400; focal length, 5–10 cm, and flash mode, forced.
The inclusion criteria were as follows: (I) adult patients aged over 18 years; (II) patients who underwent conventional thyroid surgery; and (III) no nanocarbon or other contrast agent was injected during the operation. The exclusion criteria were as follows: (I) patients with parathyroid adenoma; and (II) those who refused to sign the informed consent form. For each surgery, one to four photos were obtained from each patient. The confirmation of parathyroid tissue involved intraoperative freezing or joint confirmation by three senior physicians (with more than 10 years of thyroid surgery experience). This study was conducted in accordance with the Declaration of Helsinki (as revised in 2013), and was reviewed and approved by the Ethics Committee of Beijing Tongren Hospital affiliated with Capital Medical University (ethics No. TREC2024-KYS186). All patients signed the informed consent form.
Image data processing
All the images were screened by three senior thyroid surgeons to exclude unclear or blurred photos. Then, the region of interest (ROI) was outlined using ImageJ software. Three physicians simultaneously confirmed and identified ROIs on the same image. When there was a dispute, the three physicians discussed the issue and provided a unanimous final opinion. After confirming the ROI, the photos were saved in “tiff” format. Then, macro code based on ImageJ software was used to convert all the photos into nine color channels: red, blue, green, hue, saturation, Vue, a_star, b_star, and L_star, and a mask file (Figure 1).
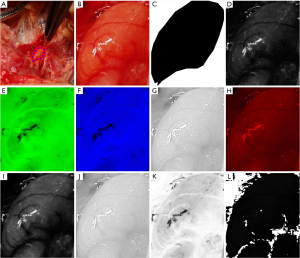
Texture feature extraction
PyRadiomic software, which is based on the Python programming language version 3.9.9, is used for texture feature extraction. This software extracts 3D image-based images as well as texture features from 2D medical images (13). After entering the code for the 9 processed photos and 1 mask, each color channel photo can extract a total of 104 features in 7 categories. For the details of the texture features, please see Appendix 1. The explanations and categories of these features are available on the website https://pyradiomics.readthedocs.io/en/latest/, which details the calculation formulas and principles of all the texture features used in this study. All the extracted features are saved in “csv” file format for further machine learning and feature analysis.
Feature selection
After the above steps, a total of 9*104=936 texture features were extracted. Owing to the excessive number of features, this approach is not conducive to the establishment of the subsequent prediction model and feature analysis. Therefore, we first identify and delete the outliers within the features, subsequently removing any features that contain these outliers. We subsequently conducted a groupwise analysis of the characteristics between the parathyroid gland group and the non-parathyroid gland group, screening out features with clear group differences (P<0.05). The remaining features were further screened using the least absolute shrinkage and selection operator (LASSO) algorithm. The selected features were used for the establishment of the next model.
Establishment of models and analysis of feature importance
All the data were randomly divided into a training set and a test set for testing model performance at a ratio of 7:3. The 12 most commonly used machine learning (ML) algorithms were used to establish models. After each algorithm was repeatedly tuned, the model with the best test results on the test set was selected as the final application model. The SHAP algorithm was subsequently used to analyze the importance of features, confirming which features contributed the most to the model, facilitating interpretability analysis of the model. Model performance was evaluated in terms of accuracy, sensitivity, specificity, and receiver operating characteristic (ROC) curves to calculate the area under the ROC curve (AUC). These parameters are commonly used to evaluate model performance.
Statistical analysis
The statistical analysis involved in this study was completed using SPSS 20.0 (IBM, USA). The differences between groups with different characteristics were analyzed using independent sample t tests, and correlation analysis was performed using Spearman’s algorithm. A P value less than 0.05 was considered statistically significant.
Results
Basic information of the included data
A total of 117 parathyroid glands and 169 suspected but not parathyroid gland tissue photos were included. All 286 photos were successfully delineated based on the ROI and converted to color channels and were randomly divided into a training set and a test set at a ratio of 7:3. The training set included 94 photos of parathyroid tissue and 134 photos of non-parathyroid tissue. The test set and training set data were independent of each other and included 23 cases of parathyroid tissue and 35 cases of non-parathyroid tissue.
Feature selection results
First, a total of 936 features were extracted. After the features with abnormal values were removed, 927 features remained. After conducting an intergroup difference analysis on these features, 293 features were identified as having significant intergroup differences (P<0.05). After LASSO screening, 46 features remained. The screening process of LASSO is shown in Figure 2. Owing to the large number of 46 features, we further used correlation analysis to find and remove features with high correlation coefficients. For example, when the correlation coefficient between two features exceeds 0.8, one of the features is removed to avoid overfitting due to high feature correlation. The six features most relevant to the parathyroid gland were subsequently selected for final model establishment and model interpretability analysis. The correlation heatmap of these six features is shown in Figure 3, which reveals that there is no correlation between these six features exceeding 80%.
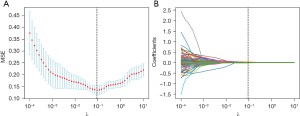
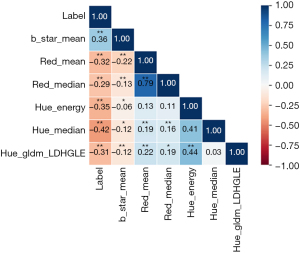
Model establishment results and feature importance analysis
Among all 10 ML algorithms, the RF algorithm achieved the best model test results, with accuracy, sensitivity, specificity, AUC, and kappa values of 89.6%, 85.7%, 91.8%, 88.7%, and 77.5%, respectively (Table 1). The confusion matrix and the ROC curve are shown on the left and right of Figure 4, respectively. The SHAP feature importance analysis results of this model suggest that b_star_mean, Hue_median, Red_median, Hue_gldm_LDHGLE, Red_mean, and Hue_energy are the most important features for predicting parathyroid glands (Figure 5). These six features are derived from three color channels: the b_star color channel, the red color channel, and the hue color channel. These findings indicate that these three-color channels are better for identifying parathyroid glands than the other six-color channels.
Table 1
Algorithms | Accuracy (%) | Sensitivity (%) | Specificity (%) | AUC (%) | Kappa (%) |
---|---|---|---|---|---|
Random forest | 89.6 | 85.7 | 91.8 | 88.7 | 77.5 |
Logistic regression | 84.3 | 63.0 | 98.6 | 80.8 | 65.3 |
K-nearest neighbor | 68.7 | 54.3 | 78.2 | 66.3 | 33.3 |
Decision tree | 80.9 | 78.2 | 82.6 | 80.4 | 60.4 |
AdaBoost | 84.3 | 78.2 | 88.4 | 83.3 | 67.2 |
XGBoost | 84.3 | 83.3 | 84.9 | 84.1 | 66.9 |
Light GBM | 84.3 | 76.1 | 89.9 | 83.0 | 66.9 |
Linear discriminant analysis | 82.6 | 63.0 | 95.7 | 79.3 | 61.8 |
MLP classifier | 74.4 | 39.3 | 96.2 | 67.8 | 39.7 |
SVM | 80.0 | 73.8 | 83.6 | 78.7 | 57.1 |
AdaBoost, Adaptive Boosting; AUC, area under the curve; GBM, gradient boosting machine; ML, machine learning; MLP, multi-layer perceptron; SVM, support vector machine; XGBoost, eXtreme Gradient Boosting.
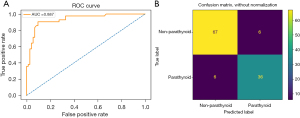
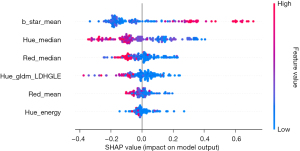
Discussion
This study first explored and searched for key image texture features that can predict parathyroid tissue and then established a preliminary prediction model for parathyroid tissue. The test accuracy is 89.6%, the sensitivity is 85.7%, the specificity is 91.8%, the AUC is 88.7%, and the kappa value is 77.5%. In addition, it was preliminarily demonstrated that the textural features of photos also contribute to the prediction of parathyroid tissue, which has certain practical value.
Currently, medical images of a certain disease, such as those obtained from laryngoscopy or gastroscopy, are primarily analyzed for diagnostic purposes by clinicians on the basis of their experience, using visually recognizable features, such as the morphology of the tissue, the roughness of the surface, and whether the edges are smooth. However, this approach will miss many subtle features, such as the texture features of the image (14,15). The texture feature characterizes the arrangement and pattern of image signals, either in their original spatial layout or in transformed representations such as Fourier or other spectral domains. Texture features have been applied to a certain extent in the medical field. For example, Valjarevic et al. researched the recognition of laryngeal cancer on the basis of the texture features of images (15), and Zhang et al. researched useful information from retinal images (16). Another team led by the first author of this study also developed a predictive model for the risk stratification of vocal cord leukoplakia based on texture features in the early stage (17). This finding indicates that texture features have a very important auxiliary value for the diagnosis of medical image classes.
The identification of parathyroid glands is based mainly on the experience of clinicians, intraoperative freezing, near-infrared light autofluorescence equipment, etc. However, relevant reports on the use of image texture features to predict parathyroid glands are not available. This study not only extracts texture features from a single image but also extracts features separately after disassembling the image’s color channels in advance. Different color channels have different effects on the display of image texture features, which can better assist in image-based diagnosis (18-20).
The important texture features found in this study for predicting parathyroid glands include b_star_mean, Hue_median, Red_median, Hue_gldm_LDHGLE, Red_mean, and Hue_energy. Here, b_star_mean refers to the mean feature from the b_star color channel, which belongs to the CIELab color space and represents the color component yellowness-blueness from blue to yellow. The mean represents the average color intensity of this color channel. The prediction mechanism of this feature may be that parathyroid tissue is slightly yellowish compared with lymph node tissue, which has certain predictive value. However, the specific mechanism requires further research. The red color channel is an independent component that represents the intensity of the red component in an image. This channel has two key predictive features, Red_mean and Red_median. The former represents the average color intensity of the red color channel, whereas the latter represents the median color intensity. In combination with the b_star features mentioned above, these findings further illustrate the possible role of red and yellow colors in distinguishing parathyroid tissue, suspicious lymph nodes, and fat granule tissue.
The remaining three features are derived from the hue color channel. Each hue is one of the hue saturation value (HSV) color channels, which is a color representation method based on three parameters, including hue, saturation, and value. Hue_median represents the median intensity of the hue, and Hue_energy is a measure of the magnitude of the voxel values in the image. These features also play a key role in predicting the parathyroid gland, but the specific mechanism is unknown. This may be because significant hue differences exist between parathyroid gland tissue and other tissues. Hue_gldm_LDHGLE refers to the large dependence high gray level emphasis (LDHGLE) feature in the gray level dependence matrix (GLDM) category of the hue color channel. The latter represents the joint distribution of large dependences with higher gray-level values, which has certain applications in the field of three-dimensional computed tomography (CT) imaging omics (20). However, the mechanism of predicting parathyroid tissue in two-dimensional images requires further exploration.
There are several limitations in this study. First, although additional prospective models were used to validate the dataset, the data were obtained from a single center, and the model performance was only good when used in that center. Thus, the model’s generalizability cannot be guaranteed for data from other centers. Second, the sample size was relatively small. Because few doctors routinely take photos of suspected parathyroid tissue during thyroid surgery to preserve data, it is necessary to increase the sample size and collect more data in the future. Finally, the model is relatively complex to use. Currently, our team has successfully developed software that automatically extracts image texture features. In the future, this software will be publicly available to further facilitate the application of this model.
Conclusions
This study successfully established and validated an efficient, convenient, and inexpensive preliminary prediction model for the parathyroid gland. In the future, further research should be conducted to incorporate more data from multiple centers and establish a more practical and robust prediction model.
Acknowledgments
We wish to thank all the individuals who participated in this study.
Footnote
Reporting Checklist: The authors have completed the TRIPOD reporting checklist. Available at https://gs.amegroups.com/article/view/10.21037/gs-2024-522/rc
Data Sharing Statement: Available at https://gs.amegroups.com/article/view/10.21037/gs-2024-522/dss
Peer Review File: Available at https://gs.amegroups.com/article/view/10.21037/gs-2024-522/prf
Funding: None.
Conflicts of Interest: All authors have completed the ICMJE uniform disclosure form (available at https://gs.amegroups.com/article/view/10.21037/gs-2024-522/coif). The authors have no conflicts of interest to declare.
Ethical Statement: The authors are accountable for all aspects of the work in ensuring that questions related to the accuracy or integrity of any part of the work are appropriately investigated and resolved. This study was conducted in accordance with the Declaration of Helsinki (as revised in 2013), and was reviewed and approved by the Ethics Committee of Beijing Tongren Hospital affiliated with Capital Medical University (No. TREC2024-KYS186). All patients signed the informed consent form.
Open Access Statement: This is an Open Access article distributed in accordance with the Creative Commons Attribution-NonCommercial-NoDerivs 4.0 International License (CC BY-NC-ND 4.0), which permits the non-commercial replication and distribution of the article with the strict proviso that no changes or edits are made and the original work is properly cited (including links to both the formal publication through the relevant DOI and the license). See: https://creativecommons.org/licenses/by-nc-nd/4.0/.
References
- Hannan FM, Elajnaf T, Vandenberg LN, et al. Hormonal regulation of mammary gland development and lactation. Nat Rev Endocrinol 2023;19:46-61. [Crossref] [PubMed]
- Leung EKY. Parathyroid hormone. Adv Clin Chem 2021;101:41-93. [Crossref] [PubMed]
- Li D, Guo B, Liang Q, et al. Tissue-engineered parathyroid gland and its regulatory secretion of parathyroid hormone. J Tissue Eng Regen Med 2020;14:1363-77. [Crossref] [PubMed]
- Lu D, Pan B, Tang E, et al. Intraoperative strategies in identification and functional protection of parathyroid glands for patients with thyroidectomy: a systematic review and network meta-analysis. Int J Surg 2024;110:1723-34. [Crossref] [PubMed]
- Rao SS, Rao H, Moinuddin Z, et al. Preservation of parathyroid glands during thyroid and neck surgery. Front Endocrinol (Lausanne) 2023;14:1173950. [Crossref] [PubMed]
- Stefanou CK, Papathanakos G, Stefanou SK, et al. Surgical tips and techniques to avoid complications of thyroid surgery. Innov Surg Sci 2022;7:115-23. [Crossref] [PubMed]
- Wang X, Si Y, Cai J, et al. Proactive exploration of inferior parathyroid gland using a novel meticulous thyrothymic ligament dissection technique. Eur J Surg Oncol 2022;48:1258-63. [Crossref] [PubMed]
- Yan S, Lin L, Zhao W, et al. An improved method of searching inferior parathyroid gland for the patients with papillary thyroid carcinoma based on a retrospective study. Front Surg 2022;9:955855. [Crossref] [PubMed]
- Mehta S, Dhiwakar M, Swaminathan K. Outcomes of parathyroid gland identification and autotransplantation during total thyroidectomy. Eur Arch Otorhinolaryngol 2020;277:2319-24. [Crossref] [PubMed]
- Chen J, Zhou Q, Feng J, et al. Combined use of a nanocarbon suspension and (99m)Tc-MIBI for the intra-operative localization of the parathyroid glands. Am J Otolaryngol 2018;39:138-41. [Crossref] [PubMed]
- Kim DH, Kim SH, Jung J, et al. Indocyanine green fluorescence for parathyroid gland identification and function prediction: Systematic review and meta-analysis. Head Neck 2022;44:783-91. [Crossref] [PubMed]
- Safia A, Abd Elhadi U, Massoud S, et al. The impact of using near-infrared autofluorescence on parathyroid gland parameters and clinical outcomes during total thyroidectomy: a meta-analytic study of randomized controlled trials. Int J Surg 2024;110:3827-38. [Crossref] [PubMed]
- van Griethuysen JJM, Fedorov A, Parmar C, et al. Computational Radiomics System to Decode the Radiographic Phenotype. Cancer Res 2017;77:e104-7. [Crossref] [PubMed]
- Elazab N, Gab Allah W, Elmogy M. Computer-aided diagnosis system for grading brain tumor using histopathology images based on color and texture features. BMC Med Imaging 2024;24:177. [Crossref] [PubMed]
- Valjarevic S, Jovanovic MB, Miladinovic N, et al. Gray-Level Co-occurrence Matrix Analysis of Nuclear Textural Patterns in Laryngeal Squamous Cell Carcinoma: Focus on Artificial Intelligence Methods. Microsc Microanal 2023;29:1220-7. [Crossref] [PubMed]
- Zhang X, Chen W, Li G, et al. The Use of Texture Features to Extract and Analyze Useful Information from Retinal Images. Comb Chem High Throughput Screen 2020;23:313-8. [Crossref] [PubMed]
- Li Z, Lu J, Zhang B, et al. New Model and Public Online Prediction Platform for Risk Stratification of Vocal Cord Leukoplakia. Laryngoscope 2024;134:4329-37. [Crossref] [PubMed]
- Giuliani D. Metaheuristic Algorithms Applied to Color Image Segmentation on HSV Space. J Imaging 2022;8:6. [Crossref] [PubMed]
- Li S, Xiao K, Li P. Spectra Reconstruction for Human Facial Color from RGB Images via Clusters in 3D Uniform CIELab* and Its Subordinate Color Space. Sensors (Basel) 2023;23:810. [Crossref] [PubMed]
- Shi W, Zhou L, Peng X, et al. HIV-infected patients with opportunistic pulmonary infections misdiagnosed as lung cancers: the clinicoradiologic features and initial application of CT radiomics. J Thorac Dis 2019;11:2274-86. [Crossref] [PubMed]