Diagnostic accuracy of automated breast volume scanning, hand-held ultrasound and molybdenum-target mammography for breast lesions: a systematic review and meta-analysis
Highlight box
Key findings
• Automated breast volume scanner (ABVS) has the highest diagnostic accuracy for differentiating between benign and malignant breast lesions, compared to hand-held ultrasound (HHUS) and molybdenum-target mammography. ABVS shows superior sensitivity, specificity, positive and negative likelihood ratios, and diagnostic odds ratio.
What is known and what is new?
• Molybdenum-target mammography is traditionally used for breast cancer screening but has limitations in sensitivity, especially among younger women. HHUS offers a safe alternative but is operator-dependent and time-consuming.
• This meta-analysis confirms ABVS as a more effective and less operator-dependent method compared to HHUS and molybdenum-target mammography, offering higher reproducibility and accuracy in the diagnosis of breast lesions.
What is the implication, and what should change now?
• The superior diagnostic performance of ABVS suggests it should be considered more prominently in clinical settings for early breast cancer detection. The adoption of ABVS can potentially improve diagnostic workflows and patient outcomes, particularly in settings where quick and reliable diagnosis is critical. Future policies should support broader access to ABVS and further research to solidify its advantages over traditional methods.
Introduction
Breast cancer is one of the most common malignancies and the fifth leading cause of death in women worldwide (1). The incidence of breast cancer is increasing year by year and shows younger trend, which threatens women’s health (2). The 5-year survival rate of breast cancer reaches only 85%, and the rate is ever worse for breast cancer patients with advanced stage (3). Therefore, the advanced diagnostic methods are urgently requited to effectively improve the prognosis for women with breast cancer.
However, the ideal breast cancer diagnostic system is yet to be undetermined. Molybdenum-target mammography is the main screening method for breast cancer with low diagnostic performance, of which the sensitivity is only reported to be 69–90% (4,5). Moreover, the efficacy of molybdenum-target mammography screening in reducing mortality in women younger than 40 years of age has declined because of concerns about radiation and generally thicker breasts (6). The magnetic resonance imaging (MRI) also has some limitations, such as the inadequate particularity and inadmissible accessibility to non-wealthy regions (7). Hand-held ultrasound (HHUS) is another traditional imaging methods for the diagnosis of breast cancer. It is safe and sensitive at distinguishing the echo of gland tissue and fat, and is good at defining the boundary and morphology of lesions (6). However, the duration of the whole-breast examination and operator dependence make it inconvenient in the clinical use. Automated breast volume scanner (ABVS) is a volumetric sonographic technique in which the whole breast volume is acquired with almost isotropic voxels, providing multiple reconstruction of the breast. Compared with HHUS, ABVS has a large number of advantages, such as shorter duration of image acquisition, higher reproducibility, less operator dependence and providing reproducible and high-resolution images as well as extra information on the diagnosis of the coronal plane (8). In 2012, ABVS was approved for use by the U.S. Food and Drug Administration. Previous studies have confirmed that tumor size diagnosed by ABVS is associated with the diagnostic of clinical T1-T2 breast cancer [odds ratio (OR) =1.033; P=0.002] and sclerosing adenosis (sensitivity, specificity, and accuracy: 75%, 86.76%, 73.53%) (9,10). Currently, ABVS is only been recommended as a promising auxiliary diagnostic method for mammography (11). Considering the need for simple, rapid, and cost-effective screening methods, evaluating the accuracy of a single screening tool can provide the necessary data to support clinical decision making. However, the current research results have not reached a unanimous conclusion on this issue. Several studies have shown that ABVS has many advantages over HHUS, such as shorter image acquisition time, higher repeatability, and less dependence on the operator (12,13) While other studies did not provide the evidence which showed the difference between ABVS and other methods in the diagnostic performance for breast cancer (14). Due to the inconsistent results, this research was conducted to systematically compare the efficacy of ABVS, HHUS, and mammography for breast cancer diagnosis. We present this article in accordance with the PRISMA-DTA reporting checklist (available at https://gs.amegroups.com/article/view/10.21037/gs-24-135/rc).
Methods
Search strategy and selection criteria
Based on the predetermined protocol, we searched PubMed, Web of Science, Embase, the Cochrane Database, China National Knowledge Infrastructure (CNKI), VIP, Wanfang and SinoMed from inception to August, 2022, with the terms “breast cancer”, “automated breast volume scanner”, “Hand-held Ultrasound” and “Mammography”. Additionally, the reference lists of reviews were screened for qualifying studies. Two authors independently confirmed the eligibility of studies, collated the data from the qualifying studies and extracted the data. Discrepancies were resolved through discussion. Study quality was assessed as recommended in the Cochrane Handbook. The study language was restricted to English or Chinese.
Selection criteria
The inclusion criteria were listed as follows:
- Population: studies targeted on excisional biopsy confirmed benign or malignant breast lesions patients;
- Intervention and comparison: one of the following diagnostic method should be included, ABVS, HHUS or mammography;
- Outcomes: data on diagnostic accuracy need to be provided, including true positive, false negative, positive predictive value and negative predictive value;
- Study types: diagnostic test.
The exclusion criteria were listed as follows:
- Repeated publications;
- Literature whose data cannot be used;
- Literature with incomplete information;
- Reviews or case reports;
- Non-Chinese or English literature.
Data collection was made regarding the name of the first author, year of publication, the country in which the examination was performed, the objectives, study design, number of participants, screening methods of assessment, patients’ mean age, and the number of lesions.
Quality assessment
Conference papers, original research articles, and review papers became the basis of this study. A thorough check was made on all duplications in maintaining the nature of the review. For the quality assessment, the QUADAS-2 tool was used which involved four domains including ‘index test’, ‘reference standard’, ‘patient selection’, and ‘flow and timing’. Risk of bias in each domain is assessed, and the first 3 domains are also assessed in terms of concerns regarding applicability (15). Signalling questions are included to help judge risk of bias. QUADAS-2 tabular and graphical display can be retrieved from the Web page, http://www.bris.ac.uk/quadas/quadas-2. Dispute between the two reviewers in assessing the quality of the study was settled through discussion.
Statistical analysis
Meta-analysis was performed by Meta-Disc, and outcome measurements are the pooled sensitivity (SEN), specificity (SPE), negatively likelihood ratio (NLR), diagnostic odds ratio (DOR), area under curve (AUC) and their 95% confidence intervals (CIs) (http://www.hrc.es/investigacion/metadisc_en.htm). The heterogeneity between the results of the study was analyzed by the Cochran Q-test, and the I2 index (I2 >50% and Q-test P>0.10 indicated high heterogeneity). If there was no statistical heterogeneity among the results, the fixed effect model was adopted for meta-analysis; If there was a statistical heterogeneity among the research results, the sources of heterogeneity would be needing further analysis, and after excluding the influence of obvious clinical heterogeneity, the random effects model was used for meta-analysis. P values <0.05 were considered statistically significant. We used Stata statistical software (STATA, version 15.1) to obtain a quantitative analysis of all the publication bias according to funnel plots and the Deek’s test. We did subgroup analysis according to the year of publication, study design, sample size and the mean age of participants.
Results
Literature screening and study quality
Literature search yielded a total of 619 publications, including 12 from PubMed, 45 from Embase, 2 from Cochrane, 70 from Web of Science, 45 from CNKI, 83 from VIP, 321 from Wanfang and 41 from SinoMed. After removing duplications, 589 publications remained. After subsequent title and abstract screening, 546 were excluded (533 publications were non-relevant to the topic and 13 publications were reviews). Then the full-text content of the remaining 43 papers was screened. Twelve papers were excluded due to the insufficient calculated data. A total of 31 studies were included in this meta-analysis, comprising 19 retrospective studies and 12 prospective studies (Table 1). A flow diagram of the literature screening selection was outlined in Figure 1. And the characteristics and details concerning the literature type of the included studies were summarized in Table 1. The 31 studies underwent quality assessment using the QUADAS criteria for diagnostic studies. The results of the quality assessment after consensus were displayed graphically in Figure S1 and Table S1.
Table 1
No. | Author | Study design | Age, years | No. of lesion | No. of malignant lesion | No. of benign lesion | Instrument | ||
---|---|---|---|---|---|---|---|---|---|
HHUS | ABVS | Molybdenum-target mammography | |||||||
1 | Zhou, 2010 (16) | Retrospective | 48 | 171 | 78 | 93 | GE logiq | GE logiq | – |
2 | Chen, 2011 (17) | Perspective | 42.3 | 101 | 45 | 56 | GE logiq | GE logiq | – |
3 | Wang, 2012 (14) | Perspective | 43 | 239 | 85 | 154 | GE logiq | Acuson | – |
4 | Wang, 2012 (11) | Retrospective | 43.1 | 165 | 62 | 103 | Acuson | Acuson | Senograph |
5 | Zhu, 2013 (18) | Retrospective | 43.4 | 294 | 91 | 203 | Acuson | Acuson | – |
6 | Chen, 2013 (19) | Retrospective | 41.7 | 219 | 67 | 152 | Acuson | Acuson | – |
7 | Li, 2014 (20) | Perspective | 45.2 | 134 | 68 | 66 | Acuson | Acuson | – |
8 | Cheng, 2014 (21) | Retrospective | 42.3 | 104 | 39 | 65 | Acuson | Acuson | – |
9 | Yang, 2014 (22) | Retrospective | 48.8 | 130 | 74 | 56 | Acuson | Acuson | – |
10 | Shen, 2015 (23) | Retrospective | 46.1 | 470 | 70 | 400 | Acuson | Acuson | – |
11 | Min, 2015 (24) | Retrospective | 44.1 | 156 | 51 | 105 | Acuson | Acuson | – |
12 | Zhong, 2016 (25) | Retrospective | 46.9 | 179 | Not reported | Not reported | Acuson | Acuson | – |
13 | Cheng, 2016 (26) | Perspective | 43.7 | 71 | 31 | 40 | Acuson | Acuson | Siemens |
14 | Kuang, 2016 (27) | Perspective | 46.8 | 136 | 56 | 80 | Philips | Philips | – |
15 | He, 2016 (28) | Retrospective | 50.8 | 170 | 102 | 68 | Acuson | Acuson | – |
16 | Dai, 2016 (29) | Retrospective | 50.5 | 118 | 68 | 50 | Philips | Acuson | – |
17 | Zhu, 2017 (30) | Perspective | 50.6 | 130 | 72 | 58 | Acuson | Acuson | – |
18 | Wei, 2017 (31) | Retrospective | 44.2 | 311 | 141 | 170 | Acuson | Acuson | – |
19 | Huang, 2017 (32) | Perspective | 47.7 | 156 | 83 | 73 | Acuson | Acuson | – |
20 | Yan, 2018 (33) | Retrospective | 53.2 | 168 | 100 | 68 | Acuson | Acuson | – |
21 | Yan, 2018 (34) | Retrospective | 50.5 | 210 | 127 | 83 | Acuson | Acuson | – |
22 | Du, 2018 (35) | Retrospective | 46 | 272 | 120 | 152 | Acuson | Acuson | – |
23 | Zeng, 2018 (36) | Retrospective | 47.6 | 393 | 200 | 193 | Acuson | Acuson | – |
24 | Huang, 2018 (37) | Perspective | 50 | 76 | 18 | 58 | Acuson | Acuson | Philips |
25 | Xu, 2018 (38) | Retrospective | 45.7 | 267 | 18 | 249 | Acuson | Acuson | Senograph |
26 | Zhang, 2018 (39) | Perspective | 45.4 | 1,973 | 417 | 1,556 | Not reported | Not reported | – |
27 | Xu, 2019 (40) | Retrospective | 48.5 | 130 | 56 | 74 | Acuson | Acuson | – |
28 | Pan, 2019 (41) | Retrospective | 49.5 | 120 | 43 | 77 | Not reported | Acuson | – |
29 | Zhang, 2019 (42) | Perspective | 50.1 | 594 | 75 | 519 | Acuson | GE logiq | GE logiq |
30 | Wu, 2020 (43) | Perspective | 47.4 | 200 | 81 | 119 | – | Acuson | Siemens |
31 | Wang, 2022 (44) | Retrospective | 49.2 | 250 | 143 | 107 | Philips | GE logiq | Siemens |
ABVS, automated breast volume scanning; HHUS, hand-held ultrasound.
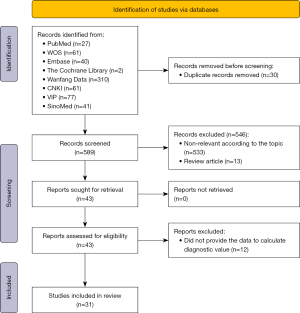
Test of heterogeneity
We first explored heterogeneity through threshold analysis by finding the logarithm of true-positive rates and the logarithm of false-positive rates. The Spearman correlation coefficient for HHUS, ABVS and molybdenum-target mammography, performed as a test for a threshold effect, was 0.091 (P=0.63), −0.052 (P=0.78) and −0.300 (P=0.62), respectively, indicating that the heterogeneity was not caused by a threshold.
Meta-analysis of diagnostic performance for breast cancer
Thirty studies provided data on the diagnostic values of HHUS for the diagnosis of breast cancer. The pooled SEN, SPE, PLR and NLR were 0.86 (0.84, 0.87), 0.80 (0.78, 0.81), 4.20 (3.53, 4.99) and 0.20 (0.16, 0.24), respectively (Table 2). And the DOR and AUC of HHUS were 22.88 (16.84, 31.08) and 0.898, respectively (Figure 2 and Figure S2). The Cochrane’s Q tests and I2 tests revealed significant heterogeneity among studies.
Table 2
Diagnostic performance indicators | HHUS | ABVS | Molybdenum-target mammography |
---|---|---|---|
SEN (95% CI) | 0.86 (0.84, 0.87) | 0.90 (0.89, 0.91) | 0.81 (0.78, 0.84) |
SPE (95% CI) | 0.80 (0.78, 0.81) | 0.87 (0.86, 0.88) | 0.90 (0.88, 0.91) |
PLR (95% CI) | 4.20 (3.53, 4.99) | 7.93 (5.05, 12.45) | 6.94 (4.32, 11.17) |
NLR (95% CI) | 0.20 (0.16, 0.24) | 0.11 (0.09, 0.15) | 0.23 (0.18, 0.29) |
DOR (95% CI) | 22.88 (16.84, 31.08) | 74.63 (45.37, 122.76) | 31.41 (17.01, 57.98) |
AUC | 0.898 | 0.956 | 0.887 |
ABVS, automated breast volume scanning; AUC, area under curve; DOR, diagnostic odds ratio; HHUS, hand-held ultrasound; NLR, negative likely ratio; SEN, sensitivity; SPE, specificity; PLR, positive likely ratio; 95% CI, 95% confidence interval.
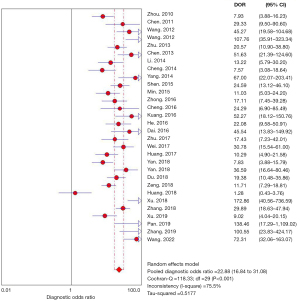
Thirty-one studies provided data on the diagnostic values of ABVS for the diagnosis of breast cancer. The pooled SEN, SPE, PLR and NLR were 0.90 (0.89, 0.91), 0.87 (0.86, 0.88), 7.93 (5.05, 12.45) and 0.11 (0.09, 0.15), respectively (Table 2). And the DOR and AUC of ABVS were 74.63 (45.37, 122.76) and 0.956, respectively (Figure 3 and Figure S3). The Cochrane’s Q tests and I2 tests revealed significant heterogeneity among studies.
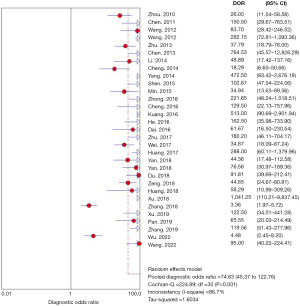
Nine studies provided data on the diagnostic values of molybdenum-target mammography for the diagnosis of breast cancer. The pooled SEN, SPE, PLR and NLR were 0.81 (0.78, 0.84), 0.90 (0.88, 0.91), 6.94 (4.32, 11.17) and 0.23 (0.18, 0.29), respectively (Table 2). And the DOR and AUC of ABVS were 31.41 (17.01, 57.98) and 0.887, respectively (Figure 4 and Figure S4). The Cochrane’s Q tests and I2 tests revealed significant heterogeneity among studies.
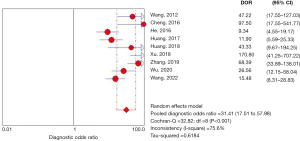
Sub-group analysis
Sub-group analysis was performed to explore the potential source of heterogeneity in DOR based on the covariates including year of publication, study design, sample size and the mean age of participants. Table 3 showed the sub-group analysis results. The DOR value of ABVS was lower in the studies which was publicated after 2016 and in the studies with sample size more than 200 [before 2016 vs. after 2016: 86.74 (52.93, 142.14) vs. 59.21 (26.95, 130.08); sample size less than 200 vs. sample size more than 200: 80.84 (41.63, 156.99) vs. 67.30 (30.34, 149.31)].
Table 3
Sub-group | HHUS | ABVS | Molybdenum-target mammography |
---|---|---|---|
Year of publication | |||
Before 2016 | 24.57 (17.08, 35.34) | 86.74 (52.93, 142.14) | 30.63 (7.44, 126.20) |
After 2016 | 21.18 (12.74, 35.20) | 59.21 (26.95, 130.08) | 32.86 (15.76, 68.49) |
Average age | |||
≤45 years | 26.80 (16.34, 43.97) | 61.72 (34.13, 111.60) | 56.59 (24.02, 133.36) |
>45 years | 21.46 (14.64, 31.47) | 77.43 (40.23, 149.02) | 27.03 (13.53, 54.01) |
Study design | |||
Retrospective | 24.14 (16.87, 34.53) | 71.61 (50.07, 102.42) | 28.44 (10.18, 79.46) |
Perspective | 20.25 (10.84, 37.82) | 65.39 (20.21, 211.58) | 35.03 (15.92, 77.08) |
Sample size | |||
≤200 | 17.03 (10.99, 26.39) | 80.84 (41.63, 156.99) | 23.98 (12.36, 46.51) |
>200 | 32.81 (22.79, 47.24) | 67.30 (30.34, 149.31) | 51.01 (13.40, 194.17) |
ABVS, automated breast volume scanning; DOR, diagnostic odds ratio; HHUS, hand-held ultrasound; 95% CI, 95% confidence interval.
Publication bias
Publication bias of all included studies concerning the diagnostic value of HHUS for breast cancer was determined using Deek’s funnel plot test. The result revealed a good symmetric distribution of the included studies on both sides of the funnel plot, suggesting a small possibility of publication bias (P=0.71, Figure S5).
Discussion
Breast cancer is one of the most common malignancies and has become a serious threat to women’s health worldwide (45). Perceptions towards breast cancer screening and diagnosis have an important role in the early diagnosis of breast cancer, which is crucial in the reduction of breast cancer mortality rate. As a new imaging technology, ABVS can automatically measure the distance between the lesion, skin and nipple, and position the mass clearly within the breast boundaries, providing accurate location information regarding the mass (8). Moreover, ABVS also makes ultrasonography less operator dependent and facilitates easier interpretation of ultrasonographic images (46). Therefore, it is necessary to explore the diagnostic efficacy of ABVS, which is a cost-effective and convenient method for breast cancer.
Some previous studies have compared the ABVS and HHUS with respect to diagnostic performance in the differential diagnosis of benign and malignant breast lesions. Wang et al. included nine studies and found that compared with HHUS, ABVS had higher DOR in the differentiation of benign and malignant breast lesions [61.68 (32.31, 117.76) vs. 52.60 (32.06, 86.35)] (47). However, the areas under the SROC curves in the differentiation of benign and malignant breast lesions were 0.93 and 0.94 for ABVS and HHUS, respectively, which were not significantly different (P=0.853).
In the current meta-analysis, 31 diagnostic studies were included. We found that ABVS had the numerically higher sensitivity, PLR, DOR and AUC compared with HHUS and molybdenum-target mammography. However, the NLR of ABVS was lower than that of HHUS and molybdenum-target mammography. These results indicated that the diagnostic accuracy of ABVS in differentiating benign from malignant breast lesions was high and ABVS had the higher diagnostic performance than the other two methods. Patients who were diagnosed positive by ABVS had a greater likelihood of breast cancer. Patients who were diagnosed negative by HHUS had a greater likelihood of not developing breast cancer. This result was consistent with the previous study. highlighting the importance of using ABVS in the early diagnosis of breast cancer, which could increase the diagnostic performance of breast cancer.
The results of sub-group analysis showed that the year of publication and sample size might be the source of heterogeneity. The results of studies with larger sample size might tend to be more accurate. In addition, the heterogeneity might be associated with the use of blinding, the low quality of literatures, the lesion mass and the experience of doctors. More studies are worthwhile to be explored in future on a larger number of patients, to enable more meaningful sub-group analysis.
However, our study still had some limitations. First, most of included studies were from China. That is due to the fact that breast lesion is tended to be diagnosed by molybdenum-target mammography in western countries. Second, we did not consider the lymph node involvement and distant metastasis in the current meta-analysis. Third, the interval between gold standard and examinations may introduce bias, because the disease status may change during the interval.
Conclusions
In conclusion, ABVS has higher diagnostic performance in the diagnosis of benign and malignant breast lesions. However, more studies are still needed to verify the above conclusion.
Acknowledgments
None.
Footnote
Reporting Checklist: The authors have completed the PRISMA-DTA reporting checklist. Available at https://gs.amegroups.com/article/view/10.21037/gs-24-135/rc
Peer Review File: Available at https://gs.amegroups.com/article/view/10.21037/gs-24-135/prf
Funding: None.
Conflicts of Interest: All authors have completed the ICMJE uniform disclosure form (available at https://gs.amegroups.com/article/view/10.21037/gs-24-135/coif). The authors have no conflicts of interest to declare.
Ethical Statement: The authors are accountable for all aspects of the work in ensuring that questions related to the accuracy or integrity of any part of the work are appropriately investigated and resolved.
Open Access Statement: This is an Open Access article distributed in accordance with the Creative Commons Attribution-NonCommercial-NoDerivs 4.0 International License (CC BY-NC-ND 4.0), which permits the non-commercial replication and distribution of the article with the strict proviso that no changes or edits are made and the original work is properly cited (including links to both the formal publication through the relevant DOI and the license). See: https://creativecommons.org/licenses/by-nc-nd/4.0/.
References
- Shibata MA, Morimoto J, Shibata E, et al. Raloxifene inhibits tumor growth and lymph node metastasis in a xenograft model of metastatic mammary cancer. BMC Cancer 2010;10:566. [Crossref] [PubMed]
- Wang S, Liu X, Huang R, et al. XIAOPI Formula Inhibits Breast Cancer Stem Cells via Suppressing Tumor-Associated Macrophages/C-X-C Motif Chemokine Ligand 1 Pathway. Front Pharmacol 2019;10:1371. [Crossref] [PubMed]
- Mei J, Zhao J, Fu Y. Molecular classification of breast cancer using the mRNA expression profiles of immune-related genes. Sci Rep 2020;10:4800. [Crossref] [PubMed]
- Aberle DR, Chiles C, Gatsonis C, et al. Imaging and cancer: research strategy of the American College of Radiology Imaging Network. Radiology 2005;235:741-51. [Crossref] [PubMed]
- Leung JW. Screening mammography reduces morbidity of breast cancer treatment. AJR Am J Roentgenol 2005;184:1508-9. [Crossref] [PubMed]
- Ibraheem SA, Mahmud R, Mohamad Saini S, et al. Evaluation of Diagnostic Performance of Automatic Breast Volume Scanner Compared to Handheld Ultrasound on Different Breast Lesions: A Systematic Review. Diagnostics (Basel) 2022;12:541. [Crossref] [PubMed]
- Partridge AH, Pagani O, Abulkhair O, et al. First international consensus guidelines for breast cancer in young women (BCY1). Breast 2014;23:209-20. [Crossref] [PubMed]
- Huang A, Zhu L, Tan Y, et al. Evaluation of automated breast volume scanner for breast conservation surgery in ductal carcinoma in situ. Oncol Lett 2016;12:2481-4. [Crossref] [PubMed]
- Li JM, Shao YH, Sun XM, et al. Ultrasonic features of automated breast volume scanner (ABVS) and handheld ultrasound (HHUS) combined with molecular biomarkers in predicting axillary lymph node metastasis of clinical T1-T2 breast cancer. Quant Imaging Med Surg 2024;14:1359-68. [Crossref] [PubMed]
- Li W, Zheng Y, Liu H, et al. Multimodal ultrasound imaging for diagnostic differentiation of sclerosing adenosis from invasive ductal carcinoma. Quant Imaging Med Surg 2024;14:877-87. [Crossref] [PubMed]
- Wang ZL, Xu JH, Li JL, et al. Comparison of automated breast volume scanning to hand-held ultrasound and mammography. Radiol Med 2012;117:1287-93. [Crossref] [PubMed]
- van Zelst JCM, Mann RM. Automated Three-dimensional Breast US for Screening: Technique, Artifacts, and Lesion Characterization. Radiographics 2018;38:663-83. [Crossref] [PubMed]
- Xiao YM, Chen ZH, Zhou QC, et al. The efficacy of automated breast volume scanning over conventional ultrasonography among patients with breast lesions. Int J Gynaecol Obstet 2015;131:293-6. [Crossref] [PubMed]
- Wang HY, Jiang YX, Zhu QL, et al. Differentiation of benign and malignant breast lesions: a comparison between automatically generated breast volume scans and handheld ultrasound examinations. Eur J Radiol 2012;81:3190-200. [Crossref] [PubMed]
- Whiting PF, Rutjes AW, Westwood ME, et al. QUADAS-2: a revised tool for the quality assessment of diagnostic accuracy studies. Ann Intern Med 2011;155:529-36. [Crossref] [PubMed]
- Shichong, Z., et al., The phenomenon and the value of three-dimensional ultrasound in the diagnosis of breast tumours. Shanghai Medical Imaging, 2010. 19(1): p. 28-29,35.
- Chen, M., The value of three-dimensional ultrasound in diagnosis of breast cancer. Guangzhou University of Chinese Medicine, 2011.
- Zhu, L., Automatic breast volume scanning system in the diagnosis of breast carcinoma. Wenzhou Medical University, 2013.
- Chen L, Chen Y, Diao XH, et al. Comparative study of automated breast 3-D ultrasound and handheld B-mode ultrasound for differentiation of benign and malignant breast masses. Ultrasound Med Biol 2013;39:1735-42. [Crossref] [PubMed]
- Li, N., L. Yang, and Z. Li, Differentiation of breast benign and malignant lesions: a comparison between automatically generated breast volume scans and handheld ultrasound examinations. Journal of kunming medical university, 2014. 35(7): p. 106-109.
- Cheng, S., J. Zhu, and G. Wang, Comparison of automated breast volume scanner and handheld ultrasound device in diagnosis of breast lesions. Practical clinical medicine, 2014. 18(8): p. 78-81.
- Yang, L., Primary application of automated breast volume scanner for the diagnosis of breast lesions. Zunyi Medical University, 2014.
- Shen C, Xu C, Zhang X. Automated breast volume scanning and acoustic radiation force impulse technology on the differential diagnosis of breast lesions. Chinese J Ultrasound Med 2015;31:1071-1073.
- Min X, et al. Clinical value of conventional handheld ultrasonography combined with automated breast volume scanning in the diagnosis of benign and malignant breast lesions. Chinese Journal of Woman and Child Health Research 2015;26:1280-1283.
- Zhong R, Lv G, Shen H. The comparion of automatic breast volume scanner and 2DUS in BI-RADS classification. Chinese J Ultrasound Med 2016;32:121-123.
- Cheng, J., Diagnostic value study of ABVS compared with MG and HHUS in breast masses. Anhui Medical University, 2016.
- Kuang X, Li J. Application of three-dimensional ultrasound in differential diagnosis of benign and malignant breast masses. Journal of Clinical Psychosomatic Diseases 2016;137.
- He, Q., A comparative study of breast ultrasound automatic volume imaging technology, common ultrasound and molybdenum target in the diagnosis of breast tumors. Beijing university of chinese medicine, 2016.
- Dai, A., Analysis of Coronal Plane Sonographic Features and Diagnostic Value of Automated breast volume scanner (ABVS) in Diagnosis of Breast Diseases. Hebei Medical University, 2016.
- Zhu, H., The application value of automated breast volume scanning combined with virtual touch tissue imaging quantification technology in diagnosing early breast cancer. Soochow University, 2017.
- Wei Y, et al. Diagnostic value of automated breast volume scanner in breast lesions. Chin J Health Manage 2017;11:514-518.
- Huang, Y., et al., Dignostic value of automated breast volume scanner versus mammography in breast neoplasms. 2017.
- Yan, N., The diagnostic value of ABVS and UE in breast tumor. Qingdao University, 2018.
- Yan L, et al. Comparative study of hand held ultrasound automated breast volume scanner and breast specific gamma imaging in the diagnosis of breast cancer. Chin J Ultrasonogr 2018;27:323-327.
- Du, J., The clinical value of automatic volume imaging combined with general ultrasound in the diagnosis of breast tumors. Beijing university of chinese medicine, 2018.
- Zeng, Z., et al., The comparative analysis of ABVS and conventional ultrasonography in differential diagnosis of benign and malignant breast masses. the Journal of Chinese Medical Sciences, 2018. 8(20): p. 19-22, 39.
- Huang, P., et al., Diagnostic value of automatic full volume breast imaging in dense breast for early breast cancer. the Journal of Zhejiang Clinical Medicine, 2018. 20(6): p. 1118-1119, 1124.
- Xu, Y., Comparative analysis of automatic breast volume imaging, molybdenum target and manual ultrasound in the diagnosis of breast diseases. the Journal of Clinical Research, 2018. 26(1): p. 11-12.
- Zhang, X., A multi-center evaluation of Automated Breast Ultrasound System for breast cancer detection compared with Hand--held ultrasound and Mammography. Peking Union Medical College, 2018.
- Xu X, Wei S, Wei J. The application value of automated breast volume scanner in differential diagnosis of breast lesions. The Journal of New Clinical Medicine in China 2019;12:175-179.
- PAN. Comparison of the diagnostic value of automatic breast full volume scan system and conventional ultrasound in breast tumor. Clinical Journal of Practical Hospitals 2019;16:90-91.
- Zhang L, Bao LY, Tan YJ, et al. Diagnostic Performance Using Automated Breast Ultrasound System for Breast Cancer in Chinese Women Aged 40 Years or Older: A Comparative Study. Ultrasound Med Biol 2019;45:3137-44. [Crossref] [PubMed]
- Wu C, et al. Study on the diagnostic value of ultrasound ABVS in breast tumors. The Journal of Zhejiang Trauma Surgery Department 2020;25:1006-1007.
- Wang C, Yang Z. Automatic breast ultrasound combined with hand-held ultrasound and MRI enhanced scanning in diagnosis of breast diseases. Chinese J Ultrasound Med 2022;38:256-259.
- Li Q, Yang H, Wang P, et al. XGBoost-based and tumor-immune characterized gene signature for the prediction of metastatic status in breast cancer. J Transl Med 2022;20:177. [Crossref] [PubMed]
- Gilja OH, Hatlebakk JG, Odegaard S, et al. Advanced imaging and visualization in gastrointestinal disorders. World J Gastroenterol 2007;13:1408-21. [Crossref] [PubMed]
- Wang L, Qi ZH. Automatic Breast Volume Scanner versus Handheld Ultrasound in Differentiation of Benign and Malignant Breast Lesions: A Systematic Review and Meta-analysis. Ultrasound Med Biol 2019;45:1874-81. [Crossref] [PubMed]