Development and validation of a radiomics-based nomogram for predicting two subtypes of HER2-negative breast cancer
Highlight box
Key findings
• The study developed a nomogram using dynamic magnetic resonance imaging features and clinical indicators to effectively differentiate human epidermal growth factor receptor 2 (HER2)-low from HER2-zero subtypes in HER2-negative breast cancer patients. The model demonstrated high accuracy, with area under curve values of 0.873 and 0.859 in internal and external validation sets, respectively.
What is known and what is new?
• In current clinical practice, HER2 status is primarily classified as positive or negative, with limited research focusing on further subclassifying HER2-negative breast cancer.
• This study provides a novel predictive model that distinguishes between HER2-low and HER2-zero subtypes, offering insights that could guide more precise treatment strategies, especially for HER2-low patients.
What is the implication, and what should change now?
• This nomogram provides a reliable tool for refining treatment decisions in HER2-negative breast cancer. Clinical implementation could improve patient stratification for antibody-drug conjugates therapies, specifically benefiting HER2-low subtype cases, and thereby personalize breast cancer treatment approaches.
Introduction
Among women, breast cancer is the most prevalent malignant tumor, with its occurrence increasing on a yearly basis. Human epidermal growth factor receptor 2 (HER2) is a receptor tyrosine kinase that is produced by the ERBB2 gene. The HER2 status of breast cancer has both independent prognostic and therapeutic predictive relevance (1-4). In 2018, the American Society of Clinical Oncology (ASCO)/American Society of Pathology classified HER2 as either HER2 positive or HER2 negative (5). The molecular evaluation of HER2 involves a binary categorization: immunohistochemistry (IHC) scoring 3+ or 2+ with fluorescence in situ hybridization (FISH) gene amplification indicates the presence of HER2, while IHC 0, 1+, or 2+ without FISH gene amplification indicates the absence of HER2. Current research has primarily been on targeted treatments for HER2-positive breast cancer (6). Several anti-HER2 targeted medications have been found to greatly decrease the likelihood of recurrence and spread of the disease in individuals with HER2-positive breast cancer (7-9). New medicines, such as trastuzumab deruxtecan (SYD-985) and trastuzumab duocarmazine (T-Dxd), which are next-generation HER2 antibody-drug conjugates (ADCs), have emerged and offer potential treatments for patients with “HER2-low” breast cancer (10,11). The notion of “HER2-low” breast cancer originates mostly from the non-randomized experiment DESTINY-Breast04 (DB-04), which showed that novel ADC medicines have some efficacy in patients with advanced HER2-low breast cancer, indicating that HER2-low breast cancer may constitute a distinct subgroup (12).
While the therapeutic approaches for HER2-low breast cancer are similar to those for HER2-negative breast cancer, research has demonstrated that its clinical and molecular features are distinct from HER2-zero breast cancer. Patients with low expression of HER2 had a significantly longer overall survival compared to those with no expression of HER2 in breast cancer (13). Therefore, there is a growing focus on classifying the two subtypes of HER2-negative breast cancer.
HER2 status is mainly determined by IHC and FISH examination of tissues after biopsy or tumor resection. First, biopsy as an invasive procedure may lead to some postoperative complications. Secondly, due to the heterogeneity of HER2 expression in the tumor, the limited sample size obtained from the core needle biopsy may not be sufficient to represent the HER2 status of the entire tumor (14). In addition, the expression of HER2 is highly unstable throughout the progression of breast cancer (15). Therefore, a noninvasive, economical and effective method is needed to predict the status of HER2 in breast cancer. Artificial intelligence has made a substantial impact on the field of medicine by effectively analyzing large amounts of data, thanks to technological advancements. The objective of this study is to combine sophisticated imaging technology with artificial intelligence algorithms in order to promptly detect the two kinds of HER2-negative breast cancer. By employing a multimodal and interdisciplinary approach, our aim is to establish a robust scientific foundation for the identification of possible advantages of ADC medicines and the formulation of more efficient treatment regimens. We present this article in accordance with the TRIPOD reporting checklist (available at https://gs.amegroups.com/article/view/10.21037/gs-24-325/rc).
Methods
Patients
The study was conducted in accordance with the Declaration of Helsinki (as revised in 2013). The study was approved by the ethics board of Affiliated Hospital of Jining Medical University (No. 2021C019) and individual consent for this retrospective analysis was waived. All participating hospitals/institutions were informed and agreed to the study. The criteria for inclusion and exclusion in this multicenter observational study were as follows (Figure 1).
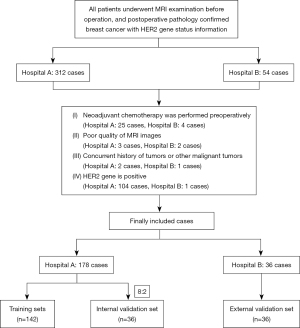
The inclusion criteria were: (I) confirmation of nonspecific invasive breast cancer through postoperative pathological biopsy; (II) patients who underwent magnetic resonance imaging (MRI) dynamic enhancement scanning before surgery; (III) postoperative IHC and/or in situ hybridization (FISH) testing; (IV) availability of complete clinical data. The exclusion criteria included: (I) having received neoadjuvant chemotherapy before surgery; (II) having low-quality MRI images; (III) having concurrent malignancies or a history of other malignant tumors; (IV) testing positive for HER2 expression.
Based on these criteria, 178 patients from Affiliated Hospital of Jining Medical University [2019–2024] were allocated to the training and internal validation sets, while 36 patients from Ningyang No. 1 People’s Hospital [2023–2024] were designated as an external validation set. To maximize generalizability, our sample size was chosen to balance statistical power with data availability from multiple sources. We partitioned the training set into an 8:2 ratio of training to internal validation using a random allocation. A two-sided P value of <0.05 was deemed statistically significant in all analyses. The data for this set were obtained from the Picture Archiving and Communication System (PACS).
HER2 assessment
According to ASCO/College of American Pathologists (CAP) criteria (16), the expression levels of HER2 in postoperative specimens were classified as IHC 0, IHC 1+, IHC 2+, and IHC 3+. Afterwards, FISH analysis was performed on samples that had an IHC status of 2+. Patients who test positive for IHC 1+ or IHC 2+ and negative for FISH were categorized as having HER2-low expression. Patients who test negative for IHC 0 are categorized as having HER2-zero expression. Patients who have an IHC score of 3+ or an IHC score of 2+ along with a positive FISH result are categorized as HER2-positive. Patients who had an indeterminate IHC result of 2+ without undergoing FISH analysis were not included in the analysis.
Imaging examinations
MRI (Table 1): The patient is placed in a face-down posture, with both breasts naturally suspended within the breast coil, starting with the feet.
Table 1
Parameters | GE 750W 3.0T | Prisma 3.0T | |||
---|---|---|---|---|---|
DCE-MRI | DWI | DCE-MRI | DWI | ||
Orientation | Transverse | Transverse | Transverse | Transverse | |
TR (ms) | 7.2 | 3,600 | 4 | 6,200 | |
TE (ms) | 2.4 | 73 | 2.2 | 74 | |
FOV (mm3) | 340×340×340 | 300×350×150 | 290×340×150 | 300×340×144 | |
Matrix | 320×320 | 128×128 | 320×320 | 128×128 | |
Slice thickness | 0.6 | 4 | 0.6 | 4 | |
Gap | 0 | 0 | 0 | 0 | |
Nex | 1 | 2 | 1 | 2 | |
b value (s/mm2) | – | 0, 800 | – | 0, 800 | |
Scanning time | 6 min 15 s | 2 min 38 s | 8 min | 80 s |
MRI, magnetic resonance imaging; DCE-MRI, dynamic contrast enhancement-MRI; DWI, diffusion-weighted imaging; TR, repetition time; TE, echo delay time; FOV, field of view; Nex, number of excitation
Affiliated Hospital of Jining Medical University: initially, a scan was conducted without the use of contrast material. This is immediately followed by an injection of gadopentetate dimeglumine at a flow rate of 2.5 mL/s and a dose of 0.2 mmol/kg through the antecubital vein. Subsequently, a series of eight dynamic contrast-enhanced images were acquired during free-breathing, with each image acquisition lasting 60 seconds. This leads to a cumulative scan time of 8 minutes. The primary scanning sequences and parameters were as outlined below.
Ningyang No. 1 People’s Hospital: initially, a scan without contrast was conducted, immediately followed by an injection of gadopentetate dimeglumine at a flow rate of 3.0 mL/s and a dose of 0.1 mmol/kg into the antecubital vein. Subsequently, six sequential dynamic contrast-enhanced images were acquired during unconstrained respiration, with each image acquisition lasting 60 seconds. This leads to a cumulative scan duration of 6 minutes and 15 seconds.
The DICOM format was used to download the second phase of contrast-enhanced sequence images and diffusion weighted imaging (DWI) images with a b-value of 800 from the PACS system. These images were collected 1–2 minutes after contrast agent injection and showed the most noticeable contrast between the tumor and surrounding tissues (17).
Image preprocessing: in this study, N4 bias field correction was first performed on the magnetic resonance sequence to eliminate the intensity inhomogeneity of the corrected sequence. Then the image was resampled and the voxel size is 1 mm × 1 mm × 1 mm, which was used to reduce the difference between different machines.
Breast lesion delineation and radiomic feature extraction
The MRI image segmentation of the region of interest (ROI) was conducted using ITK-SNAP software (Version 4.0.1). Two radiologists, each with 5 years of experience in breast diagnosis, manually did all segmentations of the MRI images. The radiologists conducting the MRI scan were unaware of the patients’ clinical data and automatically focused on identifying the largest tumor when numerous lesions were present. Firstly, an N4 Bias Field Correction was conducted to rectify the bias field effects induced by inhomogeneity in the MRI apparatus, commonly referred to as brightness or intensity inhomogeneity. Afterwards, radiologists A and B outlined the images from the second phase of the contrast-enhanced sequence and the DWI images. Afterwards, the PyRadiomics software was utilized to extract characteristics from the defined ROIs for each patient’s sequence.
Feature selection and signature construction
At first, radiomic characteristics were assessed to determine the level of agreement between two imaging annotator physicians, using the intraclass correlation coefficient (ICC) as a measure. A total of forty patients were chosen at random, and two physicians with a minimum of 5 years of expertise in imaging diagnostics independently identified the ROIs and extracted the relevant characteristics. Only radiomic characteristics with an interclass correlation coefficient (ICC) of 0.75 or above were kept (18). Afterwards, the radiomic features that were kept were then put through a process of feature selection. In cases where there were features that had a lot of overlap, we utilized the Pearson correlation coefficient to measure how closely related they were to each other. We only kept one of any two features that had a correlation coefficient higher than 0.9. The least absolute shrinkage and selection operator (LASSO) regression model was utilized, employing 10-fold cross-validation to enhance the selection of radiomic characteristics. Ultimately, the radiomic signature was created with the K-Nearest Neighbor (KNN) machine learning method.
Model construction and evaluation
Clinical features include preoperative CA153 and carcinoembryonic antigen (CEA) levels, age, maximum diameter, tumor form, and more. To identify independent predictors, important clinical characteristics from the training set were incorporated in multivariate logistic regression after univariate logistic regression. A clinical model was created employing these independent predictors. To enhance prediction performance, a model was developed by integrating distinct predictors with radiomic features (λ≠0). The receiver operating characteristic (ROC) curve was used to evaluate the predictive performance of three models—clinical, radiomics, and nomogram—for detecting the two HER2-negative subtypes. Model performance measures were sensitivity and specificity. Compare the clinical, radiomics, and nomogram models’ area under curve (AUC) using the DeLong test. Decision curve analysis (DCA) was used to examine the clinical, radiomics, and nomogram models’ net benefits at different threshold probabilities. To compare model forecasts to real findings, calibration curves were used.
Statistical analysis
The objective of this study is to ascertain whether there are any notable statistical disparities in clinical parameters between the group exhibiting low HER2 expression and the group exhibiting zero HER2 expression. Quantitative variables were represented by the mean ± standard deviation (SD). Categorical variables were presented as frequencies and percentages. T-tests were used for quantitative variables that followed a normal distribution, while the Mann-Whitney U test was used for variables that did not follow such a distribution. The Chi-squared test is utilized to analyze categorical variables. Univariate and multivariate logistic regression studies were employed to compute odds ratios (ORs) and 95% confidence intervals (CIs). The selection of radiomics features is performed via LASSO regression. The ROC curve is used to assess the ability of models to discriminate, whereas clinical decision curves are used to evaluate the practical use of the models in a clinical setting. Calibration curves are used to evaluate the accuracy of the model’s predictions by comparing them to real-world outcomes. The statistical analyses are performed using the Stats program (statsmodels 0.11.1) and R software version 4.1.3 (R Foundation for Statistical Computing, Vienna, Austria). The Python package version is scikit-learn 1.1.3. A P value that is less than 0.05 is considered to have statistical significance (two-sided).
Results
Patient clinical information
Between January 2019 and April 2024, a cohort of 214 patients were included in the training set as well as the internal and external validation sets. The internal training set consisted of 142 patients, the internal test set comprised 36 patients, and the external validation set comprised 36 patients. Table 2 displays the correlation between HER2 gene expression and patient clinical information, together with MRI characteristics. The presence of HER2-negative status showed a strong association with the largest size of the tumor (P<0.001), CA153 levels (P<0.001), and CEA levels (P=0.014).
Table 2
Characteristics | Sort | All (n=214) | HER2-low (n=143) | HER2-zero (n=71) | P |
---|---|---|---|---|---|
Number of lesions, n (%) | MCBC | 18 (8.411) | 9 (6.294) | 9 (12.676) | 0.29 |
MFBC | 32 (14.953) | 22 (15.385) | 10 (14.085) | ||
UBC | 164 (76.636) | 112 (78.322) | 52 (73.239) | ||
Background enhancement, n (%) | Marked | 17 (7.944) | 12 (8.392) | 5 (7.042) | 0.78 |
Mild | 174 (81.308) | 117 (81.818) | 57 (80.282) | ||
Moderate | 23 (10.748) | 14 (9.790) | 9 (12.676) | ||
Type of lesion, n (%) | Mass | 157 (73.364) | 101 (70.629) | 56 (78.873) | 0.40 |
Non-mass | 20 (9.346) | 14 (9.790) | 6 (8.451) | ||
Punctate | 37 (17.290) | 28 (19.580) | 9 (12.676) | ||
Lesion morphology, n (%) | Irregular | 140 (65.421) | 97 (67.832) | 43 (60.563) | 0.54 |
Quasi-circular | 51 (23.832) | 31 (21.678) | 20 (28.169) | ||
Lobulated | 23 (10.748) | 15 (10.490) | 8 (11.268) | ||
Edge shape, n (%) | Irregular | 81 (37.850) | 55 (38.462) | 26 (36.620) | 0.20 |
Smooth | 27 (12.617) | 14 (9.790) | 13 (18.310) | ||
Spiculated | 106 (49.533) | 74 (51.748) | 32 (45.070) | ||
Type of TIC curve, n (%) | Type I | 8 (3.738) | 6 (4.196) | 2 (2.817) | 0.82 |
Type II | 42 (19.626) | 29 (20.280) | 13 (18.310) | ||
Type III | 164 (76.636) | 108 (75.524) | 56 (78.873) | ||
Age, mean ± SD, years | 52.752±10.072 | 52.559±10.442 | 53.141±9.268 | 0.69 | |
Maximum diameter, median [IQR], mm | 23.000 [17.000, 29.000] | 20.000 [15.000, 25.000] | 28.000 [24.000, 35.000] | <0.001* | |
ADC value, median [IQR] | 0.890 [0.700, 1.000] | 0.890 [0.700, 1.000] | 0.900 [0.800, 1.000] | 0.84 | |
CA153, median [IQR] | 9.300 [7.400, 14.700] | 8.700 [6.900, 13.500] | 10.800 [8.800, 18.600] | <0.001* | |
CEA, median [IQR] | 1.910 [1.310, 2.710] | 1.780 [1.260, 2.430] | 2.200 [1.560, 3.220] | 0.01* |
*, P<0.05. MCBC, multicentric breast cancer; MFBC, multifocal breast carcinoma; UBC, unifocal breast cancer; TIC curve, time-intensity curve; SD, standard deviation; IQR, interquartile range; ADC, apparent diffusion coefficient; CA153, carbohydrate antigen 153; CEA, carcinoembryonic antigen.
Feature extraction and derivation of rad score
Using the PyRadiomics package, 107 radiomic features were extracted from the 3D images of ROIs. Only radiomic features with an ICC ≥0.75, considered highly stable, were retained for further analysis. Ultimately, 50 features from the second phase of the contrast-enhanced sequence and 65 features from DWI images were preserved, totaling 115 radiomic features. These included First Order Features, Shape Features (2D), Shape Features (3D), GLCM, GLSZM, GLRLM, NGTDM, and GLDM, among others. Pearson correlation coefficients were used to calculate the inter-feature correlation, retaining one of any two features with a correlation coefficient greater than 0.9. Subsequently, the LASSO regression model was used with 10-fold cross-validation to further refine the selection of radiomic features. Ultimately, 6 features were retained. Rad score = 0.3028169014084507 + 0.179385 * original_firstorder_TotalEnergy − 0.016192 * original_ngtdm_Coarseness + 0.039558 * original_glcm_ClusterShade +0.022536 * original_glcm_Correlation + 0.010312 * original_glcm_MCC − 0.008823 * original_shape_SurfaceVolumeRatio.1 (Figure 2).
Uni- and multivariate analyses
In the training set, univariate logistic regression analysis showed that the maximum diameter and CA153 levels were significantly associated with the expression status of HER2-negative (P<0.05). In multivariate logistic regression analysis, the maximum diameter (OR, 1.14; 95% CI: 1.08–1.21; P<0.001) and CA153 levels (OR, 1.07; 95% CI: 1.01–1.14; P=0.03) independently predicted the two subtypes of HER2-negative status (Table 3).
Table 3
Variables | Univariate analysis | Multivariate analysis | |||||||||
---|---|---|---|---|---|---|---|---|---|---|---|
β | S.E | Z | P | OR (95% CI) | β | S.E | Z | P | OR (95% CI) | ||
Age | 0.02 | 0.02 | 0.87 | 0.39 | 1.02 (0.98–1.05) | ||||||
Maximum diameter | 0.14 | 0.03 | 4.85 | <0.001* | 1.15 (1.09–1.21) | 0.13 | 0.03 | 4.53 | <0.001* | 1.14 (1.08–1.21) | |
ADC value | −0.18 | 0.73 | −0.24 | 0.81 | 0.84 (0.20–3.48) | ||||||
CA153 | 0.09 | 0.03 | 3.10 | 0.002* | 1.09 (1.03–1.16) | 0.07 | 0.03 | 2.20 | 0.03* | 1.07 (1.01–1.14) | |
CEA | 0.07 | 0.07 | 1.06 | 0.29 | 1.07 (0.94–1.22) | ||||||
Number of lesions | |||||||||||
MCBC | 1.00 (Reference) | ||||||||||
MFBC | −0.11 | 0.71 | −0.15 | 0.88 | 0.90 (0.23–3.59) | ||||||
UBC | −0.32 | 0.60 | −0.53 | 0.60 | 0.73 (0.23–2.36) | ||||||
Background enhancement | |||||||||||
Marked | 1.00 (Reference) | ||||||||||
Mild | 0.30 | 0.70 | 0.43 | 0.67 | 1.35 (0.34–5.28) | ||||||
Moderate | 0.18 | 0.89 | 0.20 | 0.84 | 1.20 (0.21–6.88) | ||||||
Type of lesion | |||||||||||
Mass | 1.00 (Reference) | ||||||||||
Non-mass | 0.06 | 0.65 | 0.09 | 0.93 | 1.06 (0.30–3.78) | ||||||
Punctate | −0.50 | 0.51 | −0.98 | 0.33 | 0.61 (0.22–1.64) | ||||||
Lesion morphology | |||||||||||
Irregular | 1.00 (Reference) | ||||||||||
Lobulated | 0.31 | 0.56 | 0.55 | 0.58 | 1.37 (0.45–4.11) | ||||||
Quasi-circular | −0.23 | 0.47 | −0.50 | 0.62 | 0.79 (0.32–1.98) | ||||||
Edge shape | |||||||||||
Irregular | 1.00 (Reference) | ||||||||||
Smooth | 0.45 | 0.60 | 0.75 | 0.45 | 1.57 (0.48–5.10) | ||||||
Spiculated | −0.06 | 0.39 | −0.15 | 0.88 | 0.94 (0.44–2.03) | ||||||
Type of TIC curve | |||||||||||
Type I | 1.00 (Reference) | ||||||||||
Type II | −0.05 | 1.23 | −0.04 | 0.97 | 0.95 (0.09–10.71) | ||||||
Type III | 0.35 | 1.17 | 0.30 | 0.77 | 1.42 (0.14–14.13) |
*, P<0.05. OR, odds ratio; CI, confidence interval; ADC, apparent diffusion coefficient; CEA, carcinoembryonic antigen; MCBC, multicentric breast cancer; MFBC, multifocal breast carcinoma; UBC, unifocal breast cancer; TIC curve, time-intensity curve.
Nomogram construction and performance
A nomogram was created using the Rad score and additional independent prognostic indicators to differentiate between the two subtypes of HER2-negative (Figure 3). Table 4 displays the performance metrics of the three models. Figure 3 displays the ROC curves for the models of the training, internal validation, and external validation sets. The nomogram model demonstrated superior diagnostic efficiency compared to the clinical and radiomics models, achieving area under the curve (AUC) values of 0.873 and 0.859, respectively. The test exhibited greater specificity and sensitivity. The radiomics model demonstrated superior performance compared to the clinical model in both the internal and external validation sets, achieving AUC values of 0.773 and 0.837, respectively. On the other hand, the clinical model achieved AUCs of 0.756 and 0.795. The DeLong test revealed no statistically significant disparities in AUC values between the clinical and radiomics models across all three sets (Table 5). DCA demonstrated that the nomogram exhibited superior performance compared to the clinical and radiomics models when considering rational threshold probabilities. This indicates that the nomogram has the ability to forecast the level of HER2 gene expression. The calibration curves demonstrated substantial concurrence between the model’s forecasts and the actual occurrences.
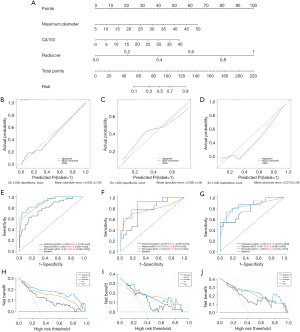
Table 4
Model | AUC | Sensitivity | Specificity | Youden index |
---|---|---|---|---|
Training set | ||||
Clinical model | 0.794 | 0.628 | 0.848 | 0.476 |
Radiomics model | 0.883 | 0.698 | 0.869 | 0.566 |
Nomogram | 0.911 | 0.721 | 0.949 | 0.670 |
Internal validation set | ||||
Clinical model | 0.756 | 0.769 | 0.739 | 0.508 |
Radiomics model | 0.773 | 0.692 | 0.826 | 0.518 |
Nomogram | 0.873 | 0.923 | 0.739 | 0.662 |
External validation set | ||||
Clinical model | 0.795 | 0.533 | 0.952 | 0.486 |
Radiomics model | 0.837 | 0.800 | 0.810 | 0.610 |
Nomogram | 0.859 | 0.733 | 0.905 | 0.638 |
AUC, area under the curve.
Table 5
Model | Clinical score | Rad score | Nomogram |
---|---|---|---|
Training set | |||
Clinical model | 0.04 | <0.001 | |
Radiomics model | 0.04 | 0.10 | |
Nomogram | <0.001 | 0.10 | |
Internal validation set | |||
Clinical model | 0.90 | 0.11 | |
Radiomics model | 0.90 | 0.091 | |
Nomogram | 0.11 | 0.091 | |
External validation set | |||
Clinical model | 0.64 | 0.38 | |
Radiomics model | 0.64 | 0.33 | |
Nomogram | 0.38 | 0.33 |
Discussion
This study involved multiple centers and aimed to create a nomogram that combines radiomic and clinical data to accurately predict the two subtypes of HER2-negative breast cancer. The nomogram demonstrated superior performance compared to individual clinical and radiomics models in accurately predicting states of HER2 low expression and zero expression. The model underwent validation using an external independent dataset, which confirmed its capacity to make accurate predictions and apply to a wide range of situations. Additionally, the validation highlighted the model’s potential for use in clinical settings. Our research indicates that the nomogram has the capability to be a non-intrusive instrument for forecasting the absence of HER2 expression in breast cancer patients, assisting in the process of determining treatment methods.
CA153 is presently a frequently utilized biomarker for breast cancer, with frequently observed excessively high levels in breast cancer serum. It is a highly reliable tool for tracking the spread of cancer, the return of the disease, and the effectiveness of treatment (19,20). CA153 has the ability to promote the growth and viability of cancer cells. Overexpression of this gene can lead to the detachment of tumor cells from surrounding stromal and normal cells, resulting in reduced cell-cell contacts and decreased adhesiveness to the matrix. In addition, there is a favorable correlation between CA153 levels and breast cancer Tumor Node Metastasis (TNM) staging, histological grading, and lymph node metastases (21,22). In addition, there is a significant correlation between the diameter of a breast tumor and its T staging (23). T1 tumors have a diameter of 2 cm or less, T2 tumors have a diameter between 2 and 5 cm, and T3 tumors have a diameter greater than 5 cm. Tumors of greater size are linked to more advanced stages and less favorable prognoses. This study discovered that both the maximal tumor diameter and CA153 are separate risk factors that can be used to predict the classification of the two subtypes of HER2-negative breast cancer. Upon integration into the clinical model, the AUC value reached 0.873, accompanied by a sensitivity of 0.923 and an accuracy of 0.739.
Radiomics is capable of extracting a multitude of quantitative imaging characteristics that provide information on texture, intensity, heterogeneity, and morphology (24-26). These characteristics cannot be easily identified through visual examination, but they can indicate the presence of tumor heterogeneity at the cellular level (27-29). By evaluating microscopic structural changes in the tumor area, radiomics may accurately predict low and zero expression of HER2.
Currently, radiomics analysis employs a range of machine learning algorithms. The KNN technique is a foundational and extensively employed algorithm for classification and regression (30). The fundamental concept behind KNN is straightforward: it entails calculating the distances between various feature points in order to identify the KNN. Subsequently, the category of an unknown point is determined by considering the categories of these neighbors, sometimes through techniques such as voting. The KNN model attained AUC values of 0.883, 0.773, and 0.837 in the training set, internal validation set, and external validation set, respectively, as observed in our study. The findings indicate that the model’s ability to accurately predict low and zero levels of HER2 expression in breast cancer patients is praiseworthy and could aid in the creation of personalized treatment approaches in clinical settings.
In recent investigations, radiomics has been used to predict the positive and negative statuses of HER2 in breast cancer (31-33). However, there are few studies on predicting HER2 low expression and zero expression in breast cancer patients (34). Accurately predicting the occurrence of low and zero levels of HER2 before treatment is crucial for the advancement of effective therapeutic strategies (35,36). We developed a radiomics model by extracting high-throughput data from MRI images in our study. The radiomics model exhibited an AUC of 0.773, accompanied by a sensitivity of 0.692 and a specificity of 0.826. It offers a conservative forecast for categorizing the two subsets of HER2-negative breast cancer. By incorporating clinical parameters such as CA153 and maximum diameter into the development of a nomogram, the AUC increased to 0.873, hence enhancing the predictive capacity of the model. The AUC of the model, when evaluated using an external independent dataset, was 0.859. This value demonstrates the model’s reliability and suitability for practical application in clinical settings. DCA revealed that the nomogram surpasses previous models in accurately forecasting low and zero levels of HER2 expression, as indicated by superior net benefits. This superiority is evident in the training set, internal validation set, and external validation set.
There are specific limitations in this study conducted at multiple centers. Initially, this is a retrospective study. Due to the limited number of samples, our intention was to enhance our data sources by collecting a substantial amount of sample data from various medical institutions. We will then proceed to conduct a prospective study to confirm the widespread applicability and precision of the integrated model. In the future, it will be feasible to gather multi-sequence MRI data and partial gene sequencing data to enhance the characteristics. Regarding picture segmentation, it is important to note that the segmentation of the tumor volume ROI was not done automatically. Instead, it requires a time-consuming semi-automatic segmentation process that relies on the expertise of the physicians performing the delineation. This problem could potentially be resolved in the future with the use of an automated artificial intelligence system designed for segmentation.
To summarize, we have developed a nomogram that utilizes radiomics and clinical data to accurately predict the classification of the two subtypes of HER2-negative breast cancer in patients. This model can assist doctors in properly forecasting the two kinds of HER2-negative breast cancer prior to treatment, showcasing the promise of artificial intelligence in augmenting tailored treatment for breast cancer patients.
Conclusions
This study developed a nomogram combining dynamic MRI radiomic features and clinical indicators to accurately distinguish between HER2-low and HER2-zero subtypes in HER2-negative breast cancer patients. The model demonstrated high predictive accuracy and stability across both internal and external validation sets, indicating its potential as a reliable tool for enhancing clinical decision-making. By effectively identifying HER2-low patients, this nomogram could support personalized treatment approaches, including consideration for next-generation ADCs. The clinical implementation of this tool may improve patient stratification and treatment outcomes in HER2-negative breast cancer.
Acknowledgments
We would like to acknowledge Ningyang No. 1 People’s Hospital for providing the valuable data used in this multicenter study.
Funding: None.
Footnote
Reporting Checklist: The authors have completed the TRIPOD reporting checklist. Available at https://gs.amegroups.com/article/view/10.21037/gs-24-325/rc
Data Sharing Statement: Available at https://gs.amegroups.com/article/view/10.21037/gs-24-325/dss
Peer Review File: Available at https://gs.amegroups.com/article/view/10.21037/gs-24-325/prf
Conflicts of Interest: All authors have completed the ICMJE uniform disclosure form (available at https://gs.amegroups.com/article/view/10.21037/gs-24-325/coif). The authors have no conflicts of interest to declare.
Ethical Statement: The authors are accountable for all aspects of the work in ensuring that questions related to the accuracy or integrity of any part of the work are appropriately investigated and resolved. The study was conducted in accordance with the Declaration of Helsinki (as revised in 2013). The study was approved by the ethics board of Affiliated Hospital of Jining Medical University (No. 2021C019) and individual consent for this retrospective analysis was waived. All participating hospitals/institutions were informed and agreed to the study.
Open Access Statement: This is an Open Access article distributed in accordance with the Creative Commons Attribution-NonCommercial-NoDerivs 4.0 International License (CC BY-NC-ND 4.0), which permits the non-commercial replication and distribution of the article with the strict proviso that no changes or edits are made and the original work is properly cited (including links to both the formal publication through the relevant DOI and the license). See: https://creativecommons.org/licenses/by-nc-nd/4.0/.
References
- Yarden Y. Biology of HER2 and its importance in breast cancer. Oncology 2001;61:1-13. [Crossref] [PubMed]
- Banerji U, van Herpen CML, Saura C, et al. Trastuzumab duocarmazine in locally advanced and metastatic solid tumours and HER2-expressing breast cancer: a phase 1 dose-escalation and dose-expansion study. Lancet Oncol 2019;20:1124-35. [Crossref] [PubMed]
- Cheng X, Jiang J, Liang X, et al. Development of a prognostic nomogram for lymph node positive HR(+)/HER2(-) breast cancer patients: a study of SEER database and a Chinese cohort. Gland Surg 2023;12:1541-53. [Crossref] [PubMed]
- Rala de Paula BH, Crocamo S, Bines J. Clinical considerations for estrogen receptor-negative/progesterone receptor-positive/HER2-negative (ER(-)PR(+)HER2(-)) breast cancer. Transl Breast Cancer Res 2023;4:8. [Crossref] [PubMed]
- Wolff AC, Hammond MEH, Allison KH, et al. Human Epidermal Growth Factor Receptor 2 Testing in Breast Cancer: American Society of Clinical Oncology/College of American Pathologists Clinical Practice Guideline Focused Update. J Clin Oncol 2018;36:2105-22. [Crossref] [PubMed]
- He L, Shen X, Liu Y, et al. The reversal of anti-HER2 resistance in advanced HER2-positive breast cancer using apatinib: two cases reports and literature review. Transl Cancer Res 2022;11:4206-17. [Crossref] [PubMed]
- Ross JS, Fletcher JA, Linette GP, et al. The Her-2/neu gene and protein in breast cancer 2003: biomarker and target of therapy. Oncologist 2003;8:307-25. [Crossref] [PubMed]
- Swain SM, Baselga J, Kim SB, et al. Pertuzumab, trastuzumab, and docetaxel in HER2-positive metastatic breast cancer. N Engl J Med 2015;372:724-34. [Crossref] [PubMed]
- Krop IE, LoRusso P, Miller KD, et al. A phase II study of trastuzumab emtansine in patients with human epidermal growth factor receptor 2-positive metastatic breast cancer who were previously treated with trastuzumab, lapatinib, an anthracycline, a taxane, and capecitabine. J Clin Oncol 2012;30:3234-41. [Crossref] [PubMed]
- van der Lee MM, Groothuis PG, Ubink R, et al. The Preclinical Profile of the Duocarmycin-Based HER2-Targeting ADC SYD985 Predicts for Clinical Benefit in Low HER2-Expressing Breast Cancers. Mol Cancer Ther 2015;14:692-703. [Crossref] [PubMed]
- Modi S, Park H, Murthy RK, et al. Antitumor Activity and Safety of Trastuzumab Deruxtecan in Patients With HER2-Low-Expressing Advanced Breast Cancer: Results From a Phase Ib Study. J Clin Oncol 2020;38:1887-96. [Crossref] [PubMed]
- Tarantino P, Hamilton E, Tolaney SM, et al. HER2-Low Breast Cancer: Pathological and Clinical Landscape. J Clin Oncol 2020;38:1951-62. [Crossref] [PubMed]
- Tang Y, Shen G, Xin Y, et al. The association between HER2-low expression and prognosis of breast cancer: a systematic review and meta-analysis. Ther Adv Med Oncol 2023;15:17588359231156669. [Crossref] [PubMed]
- D'Alfonso T, Liu YF, Monni S, et al. Accurately assessing her-2/neu status in needle core biopsies of breast cancer patients in the era of neoadjuvant therapy: emerging questions and considerations addressed. Am J Surg Pathol 2010;34:575-81. [Crossref] [PubMed]
- Miglietta F, Griguolo G, Bottosso M, et al. HER2-low-positive breast cancer: evolution from primary tumor to residual disease after neoadjuvant treatment. NPJ Breast Cancer 2022;8:66. [Crossref] [PubMed]
- Wolff AC, Somerfield MR, Dowsett M, et al. Human Epidermal Growth Factor Receptor 2 Testing in Breast Cancer: ASCO-College of American Pathologists Guideline Update. J Clin Oncol 2023;41:3867-72. [Crossref] [PubMed]
- Chatterji M, Mercado CL, Moy L. Optimizing 1.5-Tesla and 3-Tesla dynamic contrast-enhanced magnetic resonance imaging of the breasts. Magn Reson Imaging Clin N Am 2010;18:207-24. viii. [Crossref] [PubMed]
- Koo TK, Li MY. A Guideline of Selecting and Reporting Intraclass Correlation Coefficients for Reliability Research. J Chiropr Med 2016;15:155-63. [Crossref] [PubMed]
- Tarighati E, Keivan H, Mahani H. A review of prognostic and predictive biomarkers in breast cancer. Clin Exp Med 2023;23:1-16. [Crossref] [PubMed]
- Fan Y, Chen X, Li H. Clinical value of serum biomarkers CA153, CEA, and white blood cells in predicting sentinel lymph node metastasis of breast cancer. Int J Clin Exp Pathol 2020;13:2889-94.
- Li X, Dai D, Chen B, et al. Clinicopathological and Prognostic Significance of Cancer Antigen 15-3 and Carcinoembryonic Antigen in Breast Cancer: A Meta-Analysis including 12,993 Patients. Dis Markers 2018;2018:9863092. [Crossref] [PubMed]
- Seale KN, Tkaczuk KHR. Circulating Biomarkers in Breast Cancer. Clin Breast Cancer 2022;22:e319-31. [Crossref] [PubMed]
- Giuliano AE, Connolly JL, Edge SB, et al. Breast Cancer-Major changes in the American Joint Committee on Cancer eighth edition cancer staging manual. CA Cancer J Clin 2017;67:290-303.
- Yip SS, Aerts HJ. Applications and limitations of radiomics. Phys Med Biol 2016;61:R150-66. [Crossref] [PubMed]
- Guiot J, Vaidyanathan A, Deprez L, et al. A review in radiomics: Making personalized medicine a reality via routine imaging. Med Res Rev 2022;42:426-40. [Crossref] [PubMed]
- Limkin EJ, Sun R, Dercle L, et al. Promises and challenges for the implementation of computational medical imaging (radiomics) in oncology. Ann Oncol 2017;28:1191-206. [Crossref] [PubMed]
- Kang W, Qiu X, Luo Y, et al. Application of radiomics-based multiomics combinations in the tumor microenvironment and cancer prognosis. J Transl Med 2023;21:598. [Crossref] [PubMed]
- Jiang L, You C, Xiao Y, et al. Radiogenomic analysis reveals tumor heterogeneity of triple-negative breast cancer. Cell Rep Med 2022;3:100694. [Crossref] [PubMed]
- Su GH, Xiao Y, You C, et al. Radiogenomic-based multiomic analysis reveals imaging intratumor heterogeneity phenotypes and therapeutic targets. Sci Adv 2023;9:eadf0837. [Crossref] [PubMed]
- Kataria A, Singh M. A Review of Data Classification Using K-Nearest Neighbour Algorithm. International Journal of Emerging Technology and Advanced Engineering 2013;3:354-60.
- Zhou J, Tan H, Li W, et al. Radiomics Signatures Based on Multiparametric MRI for the Preoperative Prediction of the HER2 Status of Patients with Breast Cancer. Acad Radiol 2021;28:1352-60. [Crossref] [PubMed]
- Xu A, Chu X, Zhang S, et al. Development and validation of a clinicoradiomic nomogram to assess the HER2 status of patients with invasive ductal carcinoma. BMC Cancer 2022;22:872. [Crossref] [PubMed]
- Zhou J, Yu X, Wu Q, et al. Radiomics analysis of intratumoral and different peritumoral regions from multiparametric MRI for evaluating HER2 status of breast cancer: A comparative study. Heliyon 2024;10:e28722. [Crossref] [PubMed]
- Zheng S, Yang Z, Du G, et al. Discrimination between HER2-overexpressing, -low-expressing, and -zero-expressing statuses in breast cancer using multiparametric MRI-based radiomics. Eur Radiol 2024;34:6132-44. [Crossref] [PubMed]
- Denkert C, Seither F, Schneeweiss A, et al. Clinical and molecular characteristics of HER2-low-positive breast cancer: pooled analysis of individual patient data from four prospective, neoadjuvant clinical trials. Lancet Oncol 2021;22:1151-61. [Crossref] [PubMed]
- Schettini F, Chic N, Brasó-Maristany F, et al. Clinical, pathological, and PAM50 gene expression features of HER2-low breast cancer. NPJ Breast Cancer 2021;7:1. [Crossref] [PubMed]