Integrated immune-related gene signature predicts clinical outcome for patients with Luminal B breast cancer
Highlight box
Key findings
• We have developed a novel signature of immune-related gene which can predicts clinical outcomes in Luminal B breast cancer patients.
What is known and what is new?
• Several reclassification methods for breast cancer have been proposed.
• Using machine learning algorithm to construct a prognostic prediction model based on immune-related genes to reclassify patients with Luminal B breast cancer.
What is the implication, and what should change now?
• This signature can be used to predict prognosis of patients with Luminal B breast cancer, aiming to bring a more accurate and individualized treatment plan with fewer-to-no side effects.
Introduction
Breast cancer, one of the most common causes of cancer in women, has drawn great attention around the globe. In recent years, breast cancer incidence and mortality rates are still on the rise, which seriously threatens women’s health (1). At the molecular level, breast cancer can be generally divided into four subtypes: Luminal A, Luminal B, human epidermal growth factor receptor 2 (HER2)-enriched, and basal-like (2,3). Luminal A breast cancer is usually sensitive to endocrine therapy and has a relatively good prognosis. Luminal B subtype includes all types of hormone receptor-positive breast cancer, except for Luminal A, accounting for about one-fourth of the breast cancer cases (4). Compared with Luminal A breast cancer, the survival rate of patients with Luminal B breast cancer is much lower, and their prognosis and treatment sensitivity are inconsistent and varied (5,6). Patients with Luminal B breast cancer are routinely treated with chemotherapy and endocrine therapy as adjuvant treatment, and their prognosis cannot be accurately assessed and remains a matter of controversy. Only a few studies focus on the precise reclassification of Luminal B breast cancer and various appropriate treatment strategies. Therefore, it has become indispensable to find valuable biomarkers to reclassify Luminal B breast cancer more accurately.
Immunotherapy is a newly emerging treatment modality in cancer therapy that has promising potential. However, most patients with breast cancer treated by immunotherapy show negative results, except for several trials in selected patients with triple-negative breast cancer. Some studies attempt to examine the effectiveness of immunotherapy by subtyping triple-negative breast cancer to classify their characteristics and prognosis (7-13), whereas no such positive evidence has been observed in patients with Luminal B breast cancer.
In the previous studies, several reclassification methods for breast cancer have been proposed, and some of them have shown significant clinical benefits (3,14,15). However, a more precise and effective treatment could be found by using machine learning algorithm to construct a prognostic prediction model based on immune-related genes to reclassify patients with Luminal B breast cancer. Therefore, we constructed an immune-related gene signature (IRGS) to predict clinical outcomes of patients with Luminal B breast cancer, aiming to bring a more accurate and individualized treatment plan with fewer-to-no side effects, which may also provide a foothold for the clinical practice of precision medicine. We present this article in accordance with the TRIPOD reporting checklist (available at https://gs.amegroups.com/article/view/10.21037/gs-24-377/rc).
Methods
Patients
We collected the gene expression profile of patients with Luminal B breast cancer from two datasets to identify an IRGS. We included 738 patients with Luminal B breast cancer in this study: 488 from the Metabric dataset (training cohort) and 250 from The Cancer Genome Atlas (TCGA) dataset (validation cohort). The level 3 RNA expression profile data of TCGA cohort were downloaded from the GDC Data Portal (https://portal.gdc.cancer.gov/), and log2-transformed transcripts per million (TPM) were utilised. ‘Combat’ algorithm in R package ‘sva’ was used to remove batch effects. The exclusion criterion was patients without sound survival information. The study was conducted in accordance with the Declaration of Helsinki (as revised in 2013).
Construction and validation of a prognostic signature
From the ImmPort database (www.immport.org), we downloaded the gene list of 2,498 immune-related genes. By overlapping them with the Metabric and TCGA dataset, 1,090 commonly expressed immune-related genes were retained. Then, we screened out top 50% highly variant and highly expressed genes with a median absolute deviation of >0.5. After resampling 1,000 times and performing a univariate Cox regression analysis each time, those genes robustly associated with prognosis were retained. Least absolute shrinkage and selection operator (LASSO)-Cox regression were used to establish the final prognostic prediction model.
The cut-off of the risk score (RS) was determined by the time-dependent receiver operating characteristic (ROC) curve at 5 years; subsequently, patients were divided into immune high- and low-risk groups. Kaplan-Meier survival analysis and log-rank tests were performed to compare disease-free survival (DFS) of the two risk groups. Univariate analysis and multivariate analysis integrating with clinical and pathologic variables were used to test the independent prognostic value of IRGS in patients with Luminal B breast cancer.
Functional annotation and analysis
Enrichment analysis and pathway annotations were used for signature genes and differentially expressed genes (DEGs) in the two risk groups by R package ‘gProfleR’. Gene set enrichment analysis (GSEA) was conducted to further explore the underlying pathways through the Bioconductor package ‘HTSanalyzeR’. We investigated the distribution of immune and stromal composition by the Estimation of Stromal and Immune cells in Malignant Tumor tissues using Expression data (ESTIMATE) algorithm. In addition, we compared the distribution of immune cells in low- and high-risk groups in the Metabric and TCGA datasets by EPIC algorithm (16).
Statistical analysis
For comparison, the independent t-test was used for continuous variables, and the Chi-squared test or Fisher’s exact test was performed for categorical variables. LASSO regression was performed via the ‘glmnet’ R package (version 2.0-16). We performed univariate and multivariate analyses to test the association between IRGS and DFS. Variables with P<0.05 in the univariate analysis were included in the multivariate analysis through the Cox proportional hazards regression model. Statistical significance was set at P<0.05. Statistical analysis was performed in R software (version 3.5.1; http://www.Rproject.org).
Results
Construction and definition of the IRGS
In order to establish an IRGS to predict the prognosis of patients with Luminal B breast cancer, we collected data on the gene expression profiles of those patients from the Metabric and TCGA datasets for preprocessing. Next, we filtered out 488 patients from the Metabric and 250 patients from TCGA datasets. There was no significant difference in the characteristics of these two cohorts (Table 1). We obtained 2,498 immune-related genes from the ImmPort dataset. Then we screened out 396 highly expressed and highly variant immune-related genes as the candidate genes for constructing the prognostic model.
Table 1
Characteristic | Metabric LumB | TCGA BRCA LumB |
---|---|---|
Number of patients | 488 | 250 |
Patients with survival data | 488 | 242 |
Age (mean ± SD), years | 65.24±11.48 | 59.47±13.78 |
Degree of differentiation | ||
Grade 1 | 18 | NA |
Grade 2 | 193 | NA |
Grade 3 | 259 | NA |
NA | 18 | 250 |
TNM stage | ||
Stage I | 109 | 23 |
Stage II | 215 | 146 |
Stage III | 31 | 72 |
Stage IV | 6 | 5 |
NA | 127 | 4 |
Tumor location | ||
Left breast | 246 | 137 |
Right breast | 212 | 113 |
NA | 30 | 0 |
Hormone therapy | ||
Yes | 178 | 165 |
No | 310 | 85 |
NA | 0 | 0 |
Chemotherapy | ||
Yes | 178 | 156 |
No | 310 | 94 |
Radiotherapy | ||
Yes | 178 | NA |
No | 310 | NA |
NA | 0 | 250 |
TCGA, The Cancer Genome Atlas; TNM, tumour, node, metastasis; SD, standard deviation; NA, not available.
Cox regression analysis was performed to select the genes related to recurrence and metastasis in Luminal B breast cancer from the Metabric dataset. After resampling 1,000 times, 14 genes with statistically significant times >900 times (P<0.05) were retained. Through LASSO-Cox regression, 12 immune-related genes were then chosen to construct the IRGS, and the corresponding parameters were obtained. Therefore, a preliminary prognostic model comprising 12 genes for patients with Luminal B breast cancer was established (Figure 1A,1B). The RS in patients with Luminal B breast cancer was calculated using the following formula: RS = (0.090 × THBS1) + (0.213 × S100A1) − (0.047 × LANCL1) + (0.254 × PDGFRB) − (0.289 × ACO1) − (0.287 × SEMA3G) + (0.343 × ACVR1B) − (0.157 × IGF1R) + (0.093 × NR2F1) − (0.156 × PGRMC) − (0.555 × PPARA) − (0.070 × TNFRSF18).
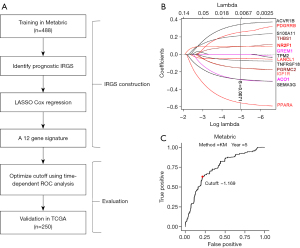
We defined a cut-off score of −1.169 by the time-dependent ROC at 5 years (Figure 1C, https://cdn.amegroups.cn/static/public/10.21037gs-24-377-1.xlsx).
IRGS powerfully predicted DFS of patients with Luminal B breast cancer
We classified the patients into high- (RS ≥−1.169) and low-risk groups (RS <−1.169) by constructing the IRGS. The distribution of the IRGS RS for individual patients in the training and validation cohorts is shown in Figure 2A,2B. Furthermore, Kaplan-Meier analysis showed that the 5-year DFS in the high-risk group was significantly lower as compared to the low-risk group, both in the training cohort [hazard ratio (HR) =4.95, 95% confidence interval (CI): 3.22–7.62, P<0.001, Figure 2C] and the validation cohort (HR =2.47, 95% CI: 1.29–4.75, P=0.005, Figure 2D), using this novel IGRS model.
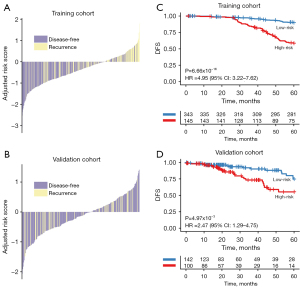
IRGS is an independent risk factor for patients with Luminal B breast cancer
To analyse the factors impacting the prognosis of Luminal B patients, we performed a univariate analysis of multiple prognostic-related indicators of breast tumours, such as RS; age; tumour location; tumour, node, metastasis (TNM) stage; hormone therapy; and chemotherapy. Among them, the RS and TNM stage were independent prognostic factors for Luminal B breast cancer, both in the training and validation cohort (P<0.05). Subsequently, multivariate analysis was used to confirm the effect of these two independent prognostic factors (training cohort RS: HR =4.96, 95% CI: 3.00–8.18, P<0.001; TNM staging: HR =1.69, 95% CI: 1.20–2.39, P=0.003; validation cohort RS: HR =2.56, 95% CI: 1.28–5.09, P=0.007; TNM staging: HR =2.25, 95% CI: 1.43–3.53, P<0.001) (Table 2).
Table 2
Characteristic | Metabric Luminal B | TCGA BRCA Luminal B | |||||||||
---|---|---|---|---|---|---|---|---|---|---|---|
Univariate | Multivariate | Univariate | Multivariate | ||||||||
HR (95% CI) | P value | HR (95% CI) | P value | HR (95% CI) | P value | HR (95% CI) | P value | ||||
Risk score | 4.95 (3.22–7.62) | <0.001 | 4.96 (3.00–8.18) | <0.001 | 2.47 (1.29–4.75) | 0.005 | 2.56 (1.28–5.09) | 0.007 | |||
Age | 1.00 (0.98–1.02) | 0.92 | 1.02 (1.00–1.05) | 0.068 | |||||||
Tumor location | 0.90 (0.58–1.39) | 0.62 | 0.73 (0.38–1.40) | 0.35 | |||||||
TNM stage | 2.04 (1.49–2.81) | <0.001 | 1.69 (1.20–2.39) | 0.003 | 2.26 (1.40–3.64) | <0.001 | 2.25 (1.43–3.53) | <0.001 | |||
Hormone therapy | 1.27 (0.81–1.99) | 0.29 | 1.41 (0.74–2.68) | 0.3 | |||||||
Chemotherapy | 1.27 (0.81–1.99) | 0.29 | 0.41 (0.19–0.90) | 0.021 | 0.26 (0.11–0.63) | 0.003 |
TCGA, The Cancer Genome Atlas; TNM, tumour, node, metastasis; HR, hazard ratio; CI, confidence interval.
Function annotation of IRGS
Furthermore, we carried out gene ontology analysis of the 12 immune-related genes in the predictive model to examine their functional pathways. The results revealed that the 12 immune-related genes were mainly enriched in pathways related to chemotherapy response (Figure 3A). The expression level of each gene showed a distinctly opposite trend between the high- and low-risk groups in both the training and the validation cohorts (Figure 3B). The result of gene ontology analysis of DEGs showed an enrichment trend in stromal related microenvironment pathways in the Metabric cohort (Figure 3C). GSEA showed that epithelial-mesenchymal transit, angiogenesis, interferon-alpha response, and interferon-gamma response were the leading pathways (Figure 3D).
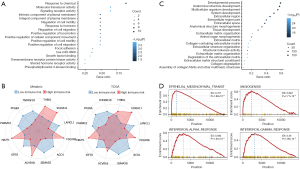
Through the ESTIMATE, immune infiltration of the high-risk group was significantly higher than the low-risk group with a significant difference in the immune score (P=0.006), the stromal score (P<0.001), and the ESTIMATE score (P<0.001) in the Metabric cohort (Figure 4A). Interestingly, we found that the immune high-risk group had a higher percentage of cancer-associated fibroblast (CAF) (P<0.001) in both the Metabric and TCGA cohorts (Figure 4B, Figures S1,S2).
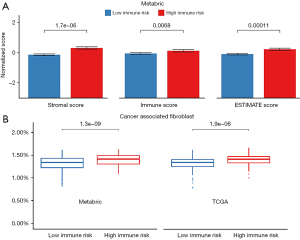
Discussion
Precision medicine has received widespread attention since its discovery, and treatment based on the molecular biology of breast cancer has become a consensus in clinical practice. Previous studies have shown that breast cancer is a heterogeneous malignant tumour (17,18). The tumour of patients with the same clinicopathological characteristics can still exhibit different biological behaviours, and their responses to treatment and their prognosis are not the same (19). This is especially seen in patients with Luminal B breast cancer, where the sensitivity of personalised treatment shows strong heterogeneity (20,21). Patients with Luminal B breast cancer are routinely treated with chemotherapy and endocrine therapy. However, there is still no effective and feasible biomarker that predicts the prognosis and the therapeutic effects of drugs on patients with Luminal B breast cancer.
At present, there are several precision treatment options at the genetic level. In patients with hormone receptor-positive breast cancer, Oncotype DX (21-gene) and Mamaprint (70-gene) can be used to detect patients with a low risk of tumour recurrence, which may be avoided with additional chemotherapy (22-24). Patients with germline BRCA gene mutations in triple-negative breast cancer can be specifically treated with poly ADP-ribose polymerase (PARP) inhibitors which have shown excellent clinical effect. There are also a small group of patients specifically treated with androgen receptor (AR) (25,26). Therefore, we have constructed this 12-gene prognostic prediction model through deep-learning analysis of the database, and this project has been identified to have a good prognostic prediction performance for patients with Luminal B breast cancer. For patients in different risk groups, the recommended treatment plans may differ. The result of gene ontology analysis revealed that the 12 genes are mainly enriched in pathways related to chemotherapy response, which may indicate its clinical potential in predicting the therapeutic response to chemotherapy.
The genes constituting the IRGS (Table S1) have been reported to correlate with tumour progression and immunotherapy. IGF1R is tightly linked with cancer incidence, which might be related to BRCA1 (27). A combined inhibiting effect of IGF1R and EGFR could promote immune activation in pancreatic cancer treatment (28). In breast cancer, lncRNA NR2F1 has been reported to promote angiogenesis by activating the IGF1R pathway (29). THBS1, an angiogenesis inhibitor as well as a potential therapeutic target, plays a vital role in tumour progression and acts as a promotor in certain pathways but as an inhibitor in others (30). THBS1 accelerates liver metastasis via epithelial-mesenchymal transition in patients with colorectal cancer (31), while activating the THBS1 signal axis in breast cancer facilitates focal adhesion and cancer aggressiveness (32). Furthermore, activating the interferon pathway with suppression of the tumour microenvironment and resistance to the immune checkpoint is an effective treatment option for breast cancer (33,34). Those echoed with GSEA analysis. The tumour stroma is closely related to the malignancy of epithelial cells, and studies have reported that the genetic inactivation of PTEN in mouse mammary stromal fibroblasts changed the tumour microenvironment through the large-scale remodelling of the extracellular matrix (ECM), innate immune cell infiltration, and increased angiogenesis, thereby affecting the development and deterioration of breast cancer (35). Moreover, the amount of stromal collagen affects the infiltration of immune-competent cells in gastric cancer, thereby changing the immune characteristics (36). Another report focused on the stromal and immune infiltration genes related to the prognosis of breast cancer, highlighting the underlying genes behind the regulation of the tumour microenvironment and cell infiltration (37). The above findings are consistent with the results of DEGs and ESTIMATE in our model. In the four consensus molecular subtypes (CMSs) of colorectal cancer, CMS1, with stronger immune activity, showed better prognosis while CMS4, with higher stromal invasion, usually had a poorer prognosis (38); comparing this to the results of the DEGs and ESTIMATE in our model, the high-risk group also exhibited the characteristics of higher stromal infiltration. Through single-cell analysis, the coordinated relationship between stromal heterogeneity and cancer immune response has been previously validated (39). In solid cancers, mesenchymal stromal cells have become vital mediators of immune function and immunotherapy response and even serve as novel predictors of drug response and new drug targets. Distinct subclasses of CAF play a critical role in immune regulation (40). A growing number of studies have reported that CAF, which are highly heterogeneous components in the tumour microenvironment, can regulate immune activity and anti-tumour immune response (41). Evidence shows that CAF, which plays a key role in breast cancer progression, is influenced by PDGFRB (42), which blocks invasive growth and migration in triple-negative breast cancer (43). The immune-checkpoint blockade treatment with PDGFRB aptamer in triple-negative breast cancer (44) gives enlightenment in treating Luminal B breast cancer. This indicates that IGRS may have potential immunotherapy significance; however, more studies are needed for further exploration and validation.
Interestingly, in our research, we found that the 12 immune-related genes mainly existed in chemotherapy-related pathways, and the gene expression is inconsistent in high- and low-risk patients. This might suggest that patients in different risk groups have different sensitivity to chemotherapy and immunotherapy. At present, immunotherapy has been selectively used for patients with triple-negative breast cancer in neoadjuvant and palliative treatment (45,46). Based on these results, we suppose that similar therapeutic plans containing immunotherapy seem feasible for high-risk patients with Luminal B breast cancer. A prospective phase II clinical trial of neoadjuvant chemotherapy plus immunotherapy in Luminal B breast cancer provided evidence for our results (47). On the other hand, the better prognosis of patients with low risk showed a possibility of reducing treatment in patients with low immune risk in the future.
Conclusions
We have developed a novel IRGS for predicting clinical outcomes in patients with Luminal B breast cancer. Furthermore, those 12 genes mostly related to response to chemical, and the expression levels were completely opposite in patients of immune low- and high-risk groups. More studies are needed to assess the clinical effectiveness of this system in predicting prognosis and treatment options for Luminal B breast cancer patients, thereby assisting clinicians to make individualised treatment plans.
Acknowledgments
The authors acknowledge all the participants and the helpful discussions with other members of The First Affiliated Hospital and The Sixth Affiliated Hospital of Sun Yat-sen University.
Funding: This study was supported by
Footnote
Reporting Checklist: The authors have completed the TRIPOD reporting checklist. Available at https://gs.amegroups.com/article/view/10.21037/gs-24-377/rc
Peer Review File: Available at https://gs.amegroups.com/article/view/10.21037/gs-24-377/prf
Conflicts of Interest: All authors have completed the ICMJE uniform disclosure form (available at https://gs.amegroups.com/article/view/10.21037/gs-24-377/coif). The authors have no conflicts of interest to declare.
Ethical Statement: The authors are accountable for all aspects of the work in ensuring that questions related to the accuracy or integrity of any part of the work are appropriately investigated and resolved. The study was conducted in accordance with the Declaration of Helsinki (as revised in 2013).
Open Access Statement: This is an Open Access article distributed in accordance with the Creative Commons Attribution-NonCommercial-NoDerivs 4.0 International License (CC BY-NC-ND 4.0), which permits the non-commercial replication and distribution of the article with the strict proviso that no changes or edits are made and the original work is properly cited (including links to both the formal publication through the relevant DOI and the license). See: https://creativecommons.org/licenses/by-nc-nd/4.0/.
References
- Fahad Ullah M. Breast Cancer: Current Perspectives on the Disease Status. Adv Exp Med Biol 2019;1152:51-64. [Crossref] [PubMed]
- Parker JS, Mullins M, Cheang MC, et al. Supervised risk predictor of breast cancer based on intrinsic subtypes. J Clin Oncol 2009;27:1160-7. [Crossref] [PubMed]
- Jiang YZ, Ma D, Suo C, et al. Genomic and Transcriptomic Landscape of Triple-Negative Breast Cancers: Subtypes and Treatment Strategies. Cancer Cell 2019;35:428-440.e5. [Crossref] [PubMed]
- Wang H, Liu XY, Jiang YZ, et al. Challenges and countermeasures in the treatment of luminal breast cancer. Zhonghua Zhong Liu Za Zhi 2020;42:192-6. [Crossref] [PubMed]
- Li ZH, Hu PH, Tu JH, et al. Luminal B breast cancer: patterns of recurrence and clinical outcome. Oncotarget 2016;7:65024-33. [Crossref] [PubMed]
- Gluz O. Luminal Breast Cancer: The Head of Janus in Breast Oncology. Breast Care (Basel) 2020;15:325-6. [Crossref] [PubMed]
- Franzoi MA, de Azambuja E. Atezolizumab in metastatic triple-negative breast cancer: IMpassion130 and 131 trials - how to explain different results? ESMO Open 2020;5:e001112. [Crossref] [PubMed]
- Emens LA, Adams S, Barrios CH, et al. First-line atezolizumab plus nab-paclitaxel for unresectable, locally advanced, or metastatic triple-negative breast cancer: IMpassion130 final overall survival analysis. Ann Oncol 2021;32:983-93. Erratum in: Ann Oncol 2021;32:1308 Erratum in: Ann Oncol 2021;32:1650. [Crossref] [PubMed]
- Schmid P, Rugo HS, Adams S, et al. Atezolizumab plus nab-paclitaxel as first-line treatment for unresectable, locally advanced or metastatic triple-negative breast cancer (IMpassion130): updated efficacy results from a randomised, double-blind, placebo-controlled, phase 3 trial. Lancet Oncol 2020;21:44-59. [Crossref] [PubMed]
- Nanda R, Chow LQ, Dees EC, et al. Pembrolizumab in Patients With Advanced Triple-Negative Breast Cancer: Phase Ib KEYNOTE-012 Study. J Clin Oncol 2016;34:2460-7. [Crossref] [PubMed]
- Schmid P, Salgado R, Park YH, et al. Pembrolizumab plus chemotherapy as neoadjuvant treatment of high-risk, early-stage triple-negative breast cancer: results from the phase 1b open-label, multicohort KEYNOTE-173 study. Ann Oncol 2020;31:569-81. [Crossref] [PubMed]
- Adams S, Loi S, Toppmeyer D, et al. Pembrolizumab monotherapy for previously untreated, PD-L1-positive, metastatic triple-negative breast cancer: cohort B of the phase II KEYNOTE-086 study. Ann Oncol 2019;30:405-11. [Crossref] [PubMed]
- Winer EP, Lipatov O, Im SA, et al. Pembrolizumab versus investigator-choice chemotherapy for metastatic triple-negative breast cancer (KEYNOTE-119): a randomised, open-label, phase 3 trial. Lancet Oncol 2021;22:499-511. [Crossref] [PubMed]
- Gong Y, Ji P, Yang YS, et al. Metabolic-Pathway-Based Subtyping of Triple-Negative Breast Cancer Reveals Potential Therapeutic Targets. Cell Metab 2021;33:51-64.e9. [Crossref] [PubMed]
- Zhao S, Zuo WJ, Shao ZM, et al. Molecular subtypes and precision treatment of triple-negative breast cancer. Ann Transl Med 2020;8:499. [Crossref] [PubMed]
- Racle J, de Jonge K, Baumgaertner P, et al. Simultaneous enumeration of cancer and immune cell types from bulk tumor gene expression data. Elife 2017;6:e26476. [Crossref] [PubMed]
- Loo LWM, Williams M, Hernandez BY. The high and heterogeneous burden of breast cancer in Hawaii: A unique multiethnic U.S. Population. Cancer Epidemiol 2019;58:71-6. [Crossref] [PubMed]
- Corso G, Magnoni F, Provenzano E, et al. Multicentric breast cancer with heterogeneous histopathology: a multidisciplinary review. Future Oncol 2020;16:395-412. [Crossref] [PubMed]
- Leong SP, Shen ZZ, Liu TJ, et al. Is breast cancer the same disease in Asian and Western countries? World J Surg 2010;34:2308-24. [Crossref] [PubMed]
- Puppe J, Seifert T, Eichler C, et al. Genomic Signatures in Luminal Breast Cancer. Breast Care (Basel) 2020;15:355-65. [Crossref] [PubMed]
- Bediaga NG, Beristain E, Calvo B, et al. Luminal B breast cancer subtype displays a dicotomic epigenetic pattern. Springerplus 2016;5:623. [Crossref] [PubMed]
- Baker H. 21-gene assay identifies patients who can avoid chemotherapy. Lancet Oncol 2015;16:e531. [Crossref] [PubMed]
- Sparano JA, Gray RJ, Makower DF, et al. Prospective Validation of a 21-Gene Expression Assay in Breast Cancer. N Engl J Med 2015;373:2005-14. [Crossref] [PubMed]
- van der Hoeven JJ. 70-Gene signature as an aid to treatment decisions in early-stage breast cancer. Ned Tijdschr Geneeskd 2017;161:D1369.
- Diéras V, Han HS, Kaufman B, et al. Veliparib with carboplatin and paclitaxel in BRCA-mutated advanced breast cancer (BROCADE3): a randomised, double-blind, placebo-controlled, phase 3 trial. Lancet Oncol 2020;21:1269-82. [Crossref] [PubMed]
- Pantelidou C, Sonzogni O, De Oliveria Taveira M, et al. PARP Inhibitor Efficacy Depends on CD8(+) T-cell Recruitment via Intratumoral STING Pathway Activation in BRCA-Deficient Models of Triple-Negative Breast Cancer. Cancer Discov 2019;9:722-37. [Crossref] [PubMed]
- Werner H, Sarfstein R. Transcriptional and epigenetic control of IGF1R gene expression: implications in metabolism and cancer. Growth Horm IGF Res 2014;24:112-8. [Crossref] [PubMed]
- Schanzer JM, Wartha K, Moessner E, et al. XGFR*, a novel affinity-matured bispecific antibody targeting IGF-1R and EGFR with combined signaling inhibition and enhanced immune activation for the treatment of pancreatic cancer. MAbs 2016;8:811-27. [Crossref] [PubMed]
- Zhang Q, Li T, Wang Z, et al. lncRNA NR2F1-AS1 promotes breast cancer angiogenesis through activating IGF-1/IGF-1R/ERK pathway. J Cell Mol Med 2020;24:8236-47. [Crossref] [PubMed]
- Wang P, Zeng Z, Lin C, et al. Thrombospondin-1 as a Potential Therapeutic Target: Multiple Roles in Cancers. Curr Pharm Des 2020;26:2116-36. [Crossref] [PubMed]
- Liu X, Xu D, Liu Z, et al. THBS1 facilitates colorectal liver metastasis through enhancing epithelial-mesenchymal transition. Clin Transl Oncol 2020;22:1730-40. [Crossref] [PubMed]
- Shen J, Cao B, Wang Y, et al. Hippo component YAP promotes focal adhesion and tumour aggressiveness via transcriptionally activating THBS1/FAK signalling in breast cancer. J Exp Clin Cancer Res 2018;37:175. [Crossref] [PubMed]
- Yamashita N, Long M, Fushimi A, et al. MUC1-C integrates activation of the IFN-γ pathway with suppression of the tumor immune microenvironment in triple-negative breast cancer. J Immunother Cancer 2021;9:e. Erratum in: J Immunother Cancer 2021;9:e002115corr1. [Crossref] [PubMed]
- De Angelis C, Fu X, Cataldo ML, et al. Correction: Activation of the IFN Signaling Pathway is Associated with Resistance to CDK4/6 Inhibitors and Immune Checkpoint Activation in ER-Positive Breast Cancer. Clin Cancer Res 2021;27:4939. [Crossref] [PubMed]
- Trimboli AJ, Cantemir-Stone CZ, Li F, et al. Pten in stromal fibroblasts suppresses mammary epithelial tumours. Nature 2009;461:1084-91. [Crossref] [PubMed]
- Ohno S, Tachibana M, Fujii T, et al. Role of stromal collagen in immunomodulation and prognosis of advanced gastric carcinoma. Int J Cancer 2002;97:770-4. [Crossref] [PubMed]
- Xu M, Li Y, Li W, et al. Immune and Stroma Related Genes in Breast Cancer: A Comprehensive Analysis of Tumor Microenvironment Based on the Cancer Genome Atlas (TCGA) Database. Front Med (Lausanne) 2020;7:64. [Crossref] [PubMed]
- Guinney J, Dienstmann R, Wang X, et al. The consensus molecular subtypes of colorectal cancer. Nat Med 2015;21:1350-6. [Crossref] [PubMed]
- Wu SZ, Swarbrick A. Single-cell advances in stromal-leukocyte interactions in cancer. Immunol Rev 2021;302:286-98. [Crossref] [PubMed]
- Mhaidly R, Mechta-Grigoriou F. Role of cancer-associated fibroblast subpopulations in immune infiltration, as a new means of treatment in cancer. Immunol Rev 2021;302:259-72. [Crossref] [PubMed]
- Chen PY, Wei WF, Wu HZ, et al. Cancer-Associated Fibroblast Heterogeneity: A Factor That Cannot Be Ignored in Immune Microenvironment Remodeling. Front Immunol 2021;12:671595. [Crossref] [PubMed]
- Primac I, Maquoi E, Blacher S, et al. Stromal integrin α11 regulates PDGFR-β signaling and promotes breast cancer progression. J Clin Invest 2019;129:4609-28. [Crossref] [PubMed]
- Camorani S, Hill BS, Collina F, et al. Targeted imaging and inhibition of triple-negative breast cancer metastases by a PDGFRβ aptamer. Theranostics 2018;8:5178-99. [Crossref] [PubMed]
- Camorani S, Passariello M, Agnello L, et al. Aptamer targeted therapy potentiates immune checkpoint blockade in triple-negative breast cancer. J Exp Clin Cancer Res 2020;39:180. [Crossref] [PubMed]
- Keenan TE, Tolaney SM. Role of Immunotherapy in Triple-Negative Breast Cancer. J Natl Compr Canc Netw 2020;18:479-89. [Crossref] [PubMed]
- Katz H, Alsharedi M. Immunotherapy in triple-negative breast cancer. Med Oncol 2017;35:13. [Crossref] [PubMed]
- Dieci MV, Guarneri V, Tosi A, et al. Neoadjuvant Chemotherapy and Immunotherapy in Luminal B-like Breast Cancer: Results of the Phase II GIADA Trial. Clin Cancer Res 2022;28:308-17. [Crossref] [PubMed]