Development and validation of a scoring model for predicting surgical benefit in patients with Masaoka stage III/IV thymic carcinomas
Highlight box
Key findings
• This study developed and applied the surgical decision score (SDS) model, which sought to quantify the factors influencing surgical choices in patients with advanced thymic tumors. Our predictive framework was designed to assess the therapeutic value of surgery in such patients and to identify the patients who are more likely to achieve survival benefits from surgical intervention.
What is known, and what is new?
• Thymoma and thymic carcinoma are aggressive types of cancer that are often diagnosed at advanced stages. While surgery is a common treatment approach, its efficacy in late-stage disease remains uncertain.
• The present study introduces the SDS model, which innovatively quantifies the factors influencing surgical selection. This new model offers a novel method for evaluating the benefits of surgical intervention in patients with advanced thymic tumors, potentially enhancing decision-making processes for treatment.
What is the implication, and what should change now?
• Our research findings have significant implications, as with the help of the SDS model, clinicians can make more informed decisions about surgical interventions for patients with advanced thymic tumors, which in turn could improve patients’ overall survival outcomes.
• This could also enable a shift towards more personalized treatment approaches. Guided by the SDS model, surgical treatment protocols for advanced thymic tumors may need to be reevaluated and adjusted to better serve patient needs and improve prognosis.
Introduction
Thymoma and thymic carcinomas originate from the epithelial cells of the thymus, and are an uncommon group of mediastinal tumors (1-4). Typically diagnosed in advanced stages due to their aggressive infiltrative behavior (1), these neoplasms are staged using the Masaoka clinicopathological system (5,6) and the tumor-node-metastasis (TNM) classification system (7-9) for precise characterization. Notably, the Masaoka staging system is widely employed due to its enhanced prognostic accuracy compared to alternative classification systems (10).
Surgical intervention is the main treatment for thymic tumors (11,12); however, due to the complexity of surgical resection in cases of distant metastases, end-stage patients often require radiotherapy or chemotherapy (13,14). Existing studies have highlighted the critical role of surgical resection in overall survival (OS) (15-17), and retrospective analyses have confirmed improved OS outcomes even for those with stage IVb thymic carcinoma (18,19). Notably, one retrospective investigation showed that the surgical treatment of patients with stage IVa thymic tumors is potentially risky, especially in those with myasthenia gravis (20). Surgical intervention has the potential to extend survival in patients with advanced thymoma by diminishing tumor burden and allowing for more accurate tumor staging, which can subsequently guide targeted pharmacological therapies. However, advanced thymomas are frequently associated with multiple complications and extensive tumor infiltration, factors that can significantly heighten the risks associated with surgical procedures. Therefore, the benefit of surgical treatment for patients with advanced thymic tumors (Masaoka III/IV) remains uncertain. This complexity underscores the necessity for careful patient selection and the development of predictive tools, such as the surgical decision score (SDS), to better evaluate the risks and benefits of surgery in this patient population.
We hypothesized that surgical treatment could provide a therapeutic advantage for patients with advanced thymic tumors and sought to investigate the use of patients’ known clinical information to construct a predictive model for surgical selection. To this end, we designed the SDS model—an innovative predictive scoring framework—to quantify the determinants influencing surgical selection in patients with advanced thymic tumors. The SDS model was designed to validate our hypothesis that surgery has therapeutic value for advanced thymic tumors, and to classify the subset of patients who would derive the greatest survival benefit based on different clinical attributes. We present this article in accordance with the TRIPOD reporting checklist (available at https://gs.amegroups.com/article/view/10.21037/gs-2024-527/rc).
Methods
Data source
The data for this study were obtained from two primary sources: the clinical records of The First Affiliated Hospital of Guangzhou Medical University, and the Surveillance, Epidemiology and End Results (SEER) database (http://www.seer.cancer.gov). The SEER database, established by the National Cancer Institute, is a publicly accessible repository, specifically designed to facilitate cancer research. The relevant clinical attributes, including clinical features, pathological characteristics, and survival outcomes, were accessed using SEER*Stat 8.3.9 software, a tool provided by the National Institutes of Health. The study was conducted in accordance with the Declaration of Helsinki (as revised in 2013). The study was approved by The Institutional Ethics Committee of The First Affiliated Hospital of Guangzhou Medical University (No. 2020-138) and informed consent was taken from all the patients.
Data collection
The thymomas and thymic carcinomas were re-staged using both the Masaoka staging system and the TNM staging system (12). In accordance with the Masaoka staging system, tumors that extended to neighboring organs or mediastinal structures were classified as stage III, while those with lymph node metastasis (LNM) or distant organ metastasis were classified as stage IV. Patients were included in the study if they met the Masaoka stage III/IV criteria and had a histopathologically confirmed diagnosis of thymoma or thymic carcinoma.
Eligible patients were identified in the SEER database. The presence of the primary tumor in the thymus was indicated by the C37.9 code. The histological types align with the International Classification of Disease (ICD) codes. Thymic carcinomas were represented by ICD codes 8020, 8033, 8070, 8082, 8123, 8140, 8260, 8310, 8430, 8480, 8560, and 8586, while thymomas were represented by ICD codes 8580, 8581, 8582, 8583, 8584, and 8585, with the malignant behavior code (/3) appended to all relevant ICD codes. For external validation purposes, patients with thymus tumors treated at the First Affiliated Hospital of Guangzhou Medical University from September 2010 to September 2021 were also identified from the clinical records of the hospital.
To be eligible for inclusion in this study, the patients had to meet the following inclusion criteria: (I) have an age at diagnosis ≥18 years; (II) have only one primary tumor; (III) life expectancy greater than 6 months with no lost to follow-up; and (IV) Masaoka stage III/IV thymic carcinomas confirmed by imaging and histopathologic diagnosis. Patients were excluded from the study if they met any of the following exclusion criteria: (I) did not have Masaoka stage III/IV; (II) had an unknown surgical history; (III) previous malignancy in the past 5 years; (IV) had a survival duration of less than one month; (V) had not had their diagnosis microscopically confirmed by histology or cytology.
Survival analysis
The cohort of eligible patients identified from the SEER database was stratified into two groups based on whether or not they underwent surgical intervention. To address potential differences in the baseline characteristics between groups, a propensity score matching (PSM) analysis was conducted, effectively reducing selection bias and confounding effects to enhance the credibility of the study results. Covariates, such as age, race, gender, histological type, differentiation grade, tumor size, TNM stage, the presence of metastasis at various anatomical sites, including the bone, brain, liver, and lungs, regional LNM, distant LNM, distant organ metastasis status, insurance coverage, and marital status, were included in the propensity model. The caliper distance was set at 0.02, and the nearest propensity score approach was employed to achieve a 1:1 pairing of patients in the surgery and non-surgery groups. The above-mentioned categorical clinical parameters were subjected to χ2 tests to analyze inter-group variations subsequent to PSM.
Within the matched subgroups, survival outcomes were compared using the Kaplan-Meier curves generated by the log-rank test subsequent to PSM. To identify the independent prognostic determinants, a multivariate Cox regression model was used, and the hazard ratios (HRs) were calculated with the 95% confidence intervals (CIs).
Subgroup survival analyses
To gain further insights into the survival patterns, subgroup analyses were conducted based on the extent of tumor spread and the presence of metastasis. Specifically, the patients were categorized into subgroups based on local invasion, positive regional LNM, and distant organ metastasis. In these subgroups, the comparative OS outcomes between the surgical and non-surgical cohorts were assessed by Kaplan-Meier analysis.
Model development
The SDS model was constructed by randomizing the training cohort from the SEER database at a 7:3 ratio. Univariate and multivariate logistic regression analyses were performed to identify the factors in the training cohort that independently determined surgical choice. Models were built by stepwise regression, starting with a simple model that included one clinical factor. The complexity of each model was increased by progressively adding factors, each of which was governed by its Akaike information criterion (AIC) value. The best model fit was determined by the lowest AIC value, which signified superior model performance. In the SDS model, the factor scores are determined by multiplying the natural logarithm of the odds ratios (ORs) extracted from the multivariate binary logistic regression model by 100.
Model validation
The SDS model underwent both internal and external validation, using the SEER database and institutional data, respectively. The discriminatory power and accuracy of the SDS model was assessed by calculating the area under the receiver operating characteristic (ROC) curve through regression analysis. An AUC >0.7 indicates a good predictive model, while an AUC ≥0.8 denotes excellent predictive ability. The validation population was categorized into high- and low-score subgroups based on the mean of the overall population, and the patients in the high- and low-score subgroups were further categorized into surgery and non-surgery groups. Kaplan-Meier analyses were performed between the different subgroups to assess the discriminative validity of the SDS model.
Statistical analysis
All the statistical analyses were performed using IBM SPSS Statistics V25.0 (IBM Corp., Armonk, NY, USA) and R version 4.0.2 software (http://www.r-project.org/) with the survival, survminer, foreign, rms, plyr, and pROC software packages. The significance threshold was set at P<0.05 and all P values are two-sided.
Results
Patient characteristics
Among the cohort of 4,935 patients diagnosed with Masaoka stage III/IV thymoma or thymic carcinoma in the SEER database from 1975 to 2016, a total of 1,106 patients met the pre-defined inclusion criteria (see Figure 1). Of these patients, 684 (61.8%) underwent surgical intervention. Before the application of PSM, significant differences were observed in a range of attributes between the surgery and non-surgery groups. These attributes included age, histological type, differentiation grade, tumor dimensions, TNM stage, and the presence of metastasis at various anatomical sites, including the bone, brain, liver, and lungs, regional LNM, distant LNM, distant organ metastasis status, and insurance and marital status (Table S1). Following the PSM procedure, a final cohort of 474 matched patients was identified. No significant difference in the baseline characteristics was observed between these matched groups (P>0.05) (Table S2).
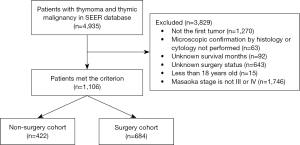
Effect of surgery on survival outcomes
Among the PSM-matched population, the patients in the surgery group had a significantly prolonged median OS time of 76.0 months (95% CI: 59.6–92.4), compared to the patients in the non-surgery group, who had a median follow-up time of only 39.0 months (95% CI: 31.1–46.9). This difference was associated with a significant HR of 0.53 (95% CI: 0.41–0.68, P<0.001), showing the favorable effect of surgical intervention on survival outcomes (see Figure 2A). Further, the stratified analyses revealed compelling survival disparities. Among the patients with local invasion, the median OS time of the surgery group was 93.0 months (95% CI: 62.5–123.5), which surpassed that of the non-surgery group, which had a median OS time of 65.0 months (95% CI: 27.3–102.7). Similarly, in the subset of patients presenting with positive regional lymph nodes, the median follow-up time of 78.0 months (95% CI: 32.9–123.1) of the surgery group exceeded the 49.0 months (95% CI: 31.6–66.4) of the non-surgery group. Importantly, the Kaplan-Meier survival curves, in tandem with the log-rank tests, confirmed the statistically significant difference in the survival rates between the surgery and non-surgery groups in these stratified analyses (see Figure 2B-2D). Cox regression modeling corroborated the independent prognostic value of surgical intervention for advanced-stage thymus tumors, as indicated by a HR of 0.45 (95% CI: 0.34–0.58) (see Table S3).
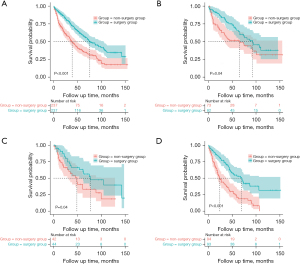
Clinicopathological characteristics of the training and validation cohorts
Details of the clinicopathological characteristics of the patients are provided in Tables S4,S5. The patient cohort from the SEER database was randomly allocated into a training cohort and an internal validation cohort at a ratio of 7:3. Patients were sourced from institutional records to establish the external validation cohort. In the training cohort, the patients had a median age of 59 years (range, 19–93 years). Further, 732 of these patients (96.4%) had received a histologic diagnosis, while 27 patients (3.6%) had received a cytologic diagnosis. A diverse range of surgical interventions were administered, including 383 cases of radical surgery (50.5%), 31 cases of debulking surgery (4.0%), 13 cases of local tumor excision (1.7%), and 34 cases of combined surgical approaches (4.5%). In the internal and external validation cohorts, the patients had median ages of 58 and 59.5 years, respectively. The internal validation cohort comprised 186 males (53.6%) and 161 females (46.3%), and 210 (60.5%) of the patients underwent surgical intervention. While the external validation cohort comprised 55 (54.5%) males and 46 (45.5%) females, and 66 (65.3%) of the patients underwent surgical intervention.
Identification of independent factors for SDS model construction
To reveal the potential selection biases affecting patients with advanced thymic tumors, an initial χ2 test was conducted to evaluate the equilibrium between the two cohorts (i.e., the training versus. internal validation cohorts). Following this assessment, univariate and multivariate binary logistic regression analyses were conducted to identify the factors affecting treatment choices. The balanced baseline characteristics of the two groups are detailed in Table S2. Notably, the multivariate logistic regression analyses revealed that several independent factors were significantly associated with the surgical option, including histological type (P<0.001), differentiation grade (P=0.006), tumor size (P<0.001), T stage (P=0.01), regional LNM status (P=0.001), distant organ metastasis status (P=0.03), and insurance coverage status (P=0.003). Comprehensive details of these findings are provided in Table 1.
Table 1
Variables | Univariate analysis | Multivariate analysis | |||||
---|---|---|---|---|---|---|---|
OR | 95% CI | P value | OR | 95% CI | P value | ||
Histological type | <0.001 | <0.001 | |||||
Type A, thymoma | 0.414 | 0.205–0.837 | 0.01 | 0.536 | 0.218–1.319 | 0.18 | |
Type AB, thymoma | 0.207 | 0.098–0.437 | <0.001 | 0.279 | 0.110–0.708 | 0.007 | |
Type B1, thymoma | 0.345 | 0.186–0.642 | 0.001 | 0.537 | 0.257–1.122 | 0.10 | |
Type B2, thymoma | 0.344 | 0.202–0.587 | <0.001 | 0.388 | 0.198–0.761 | 0.006 | |
Type B3, thymoma | 0.150 | 0.084–0.266 | <0.001 | 0.166 | 0.084–0.328 | <0.001 | |
Thymoma, NOS/thymic carcinoma | Reference | Reference | |||||
Differentiation grade | 0.01 | 0.006 | |||||
Well | 0.499 | 0.232–1.075 | 0.08 | 0.987 | 0.377–2.584 | 0.98 | |
Moderate | 0.161 | 0.037–0.695 | 0.01 | 0.088 | 0.016–0.485 | 0.005 | |
Poor | 1.359 | 0.896–2.060 | 0.15 | 0.855 | 0.492–1.486 | 0.58 | |
Undifferentiated | 0.715 | 0.306–1.672 | 0.44 | 0.238 | 0.086–0.660 | 0.006 | |
Unknown | Reference | Reference | |||||
Age (years) | 0.02 | 0.14 | |||||
<60 | 0.521 | 0.332–0.819 | 0.005 | 0.559 | 0.311–1.006 | 0.052 | |
60–75 | 0.609 | 0.381–0.974 | 0.04 | 0.589 | 0.322–1.076 | 0.09 | |
>75 | Reference | Reference | |||||
Race | 0.33 | ||||||
White | 0.899 | 0.617–1.309 | 0.58 | ||||
Black | 1.221 | 0.748–1.992 | 0.43 | ||||
Other/unknown | Reference | ||||||
Gender | 0.11 | ||||||
Male | 1.271 | 0.945–1.710 | 0.11 | ||||
Female | Reference | ||||||
Tumor size (cm) | <0.001 | <0.001 | |||||
≤6.1 | 0.150 | 0.093–0.242 | <0.001 | 0.207 | 0.113–0.380 | <0.001 | |
>6.1 | 0.248 | 0.166–0.369 | <0.001 | 0.396 | 0.234–0.672 | 0.001 | |
Unknown | Reference | Reference | |||||
T value | <0.001 | 0.01 | |||||
TX–T1 | 8.680 | 4.492–16.773 | <0.001 | 3.267 | 1.478–7.221 | 0.003 | |
T2–T3 | 0.990 | 0.644–1.522 | 0.96 | 1.328 | 0.780–2.261 | 0.30 | |
T4 | Reference | Reference | |||||
N value | <0.001 | 0.30 | |||||
NX–N0 | 0.347 | 0.249–0.483 | <0.001 | 3.149 | 0.362–27.387 | 0.30 | |
N1/N2 | Reference | Reference | |||||
M value | <0.001 | 0.052 | |||||
M0 | 0.167 | 0.121–0.230 | <0.001 | 0.156 | 0.024–1.014 | 0.052 | |
M1/MX | Reference | Reference | |||||
Met at regional lymph nodes | <0.001 | 0.001 | |||||
No | 0.204 | 0.128–0.325 | <0.001 | 0.346 | 0.180–0.665 | 0.001 | |
Yes | 0.895 | 0.539–1.487 | 0.67 | 3.254 | 0.340–31.140 | 0.31 | |
Unknown | Reference | Reference | |||||
Met at distant lymph nodes | <0.001 | 0.78 | |||||
No | 0.616 | 0.259–1.465 | 0.27 | 0.871 | 0.325–2.333 | 0.78 | |
Yes | 0.143 | 0.054–0.376 | <0.001 | 0.871 | 0.325–2.333 | 0.78 | |
Unknown | Reference | Reference | |||||
Met at distant organs | <0.001 | 0.03 | |||||
No | 0.298 | 0.178–0.500 | <0.001 | 6.023 | 0.820–44.228 | 0.08 | |
Yes | 2.176 | 1.267–3.738 | 0.005 | 4.949 | 1.470–16.666 | 0.01 | |
Unknown | Reference | Reference | |||||
Insurance status | <0.001 | 0.003 | |||||
Insured, NOS | 2.064 | 1.419–3.000 | <0.001 | 1.968 | 1.221–3.172 | 0.005 | |
Uninsured | 6.475 | 2.487–16.862 | <0.001 | 5.640 | 1.563–20.347 | 0.008 | |
Unknown | Reference | Reference | |||||
Marital status | 0.45 | ||||||
Married | 1.266 | 0.563–2.848 | 0.57 | ||||
Single | 1.486 | 0.654–3.376 | 0.34 | ||||
Unknown | Reference |
OR, odds ratio; CI, confidence interval; NOS, not otherwise specific; Met, metastasis.
Construction of the SDS model
To establish the SDS model, nested progression using the training cohort as the foundation was employed. Each model in this sequence had a distinct AIC value, indicating its performance (see Table 2). The discernible variability in the AIC values across the stepwise regression represented the diverse performance characteristics of each model. Notably, a lower AIC value indicated enhanced model performance. Among the constructed models, Model 7 showed the optimal performance, characterized by the smallest AIC value. However, it should be noted that Model 7 included insurance status as a contributing factor. While this factor had public relevance for the American cohort, it posed privacy concerns for the Chinese population. Consequently, Model 7 was deemed unsuitable for external validation. As an alternative, Model 6, which included histological type, differentiation grade, tumor size, T stage, regional LNM status, and distant organ metastasis status, was selected as the definitive model. This model was operationalized by attributing a numeric score to each patient based on their clinical attributes; the score was calculated by multiplying the natural logarithm of the OR associated with each variable from the regression model by 100 (see Table 3).
Table 2
Model | Composition | AIC value |
---|---|---|
Model 1 | Histological type | 972.116 |
Model 2 | Histological type and differentiation grade | 966.579 |
Model 3 | Histological type, differentiation grade, and tumor size | 911.084 |
Model 4 | Histological type, differentiation grade, tumor size, and T value | 886.815 |
Model 5 | Histological type, differentiation grade, tumor size, T value, and regional lymph node Met | 840.932 |
Model 6 | Histological type, differentiation grade, tumor size, T value, regional lymph node Met, and distant organ Met | 816.382 |
Model 7 | Histological type, differentiation grade, tumor size, T value, regional lymph node Met, distant organ Met, and insurance status | 804.646 |
The final model was model 6 or 7, which had the smallest AIC value. AIC, Akaike information criterion; Met, metastasis.
Table 3
Variables | P value | OR | 95% CI | Score |
---|---|---|---|---|
Histological type | 0.001 | |||
Type A, thymoma | 0.003 | 3.268 | 1.507–7.085 | 118 |
Type AB, thymoma | 0.06 | 1.890 | 0.971–3.680 | 64 |
Type B1, thymoma | 0.09 | 1.704 | 0.919–3.160 | 53 |
Type B2, thymoma | 0.001 | 2.695 | 1.507–4.818 | 99 |
Type B3, thymoma | 0.05 | 1.613 | 1.005–2.588 | 48 |
Thymoma, NOS/thymic carcinoma | Reference | 0 | ||
Differentiation grade | 0.07 | |||
Well | 0.61 | 1.216 | 0.581–2.545 | 20 |
Moderate | 0.38 | 1.524 | 0.599–3.877 | 42 |
Poor | 0.02 | 0.556 | 0.344–0.899 | −59 |
Undifferentiated | 0.19 | 0.540 | 0.217–1.343 | −62 |
Unknown | Reference | 0 | ||
Tumor size (cm) | <0.001 | |||
≤6.1 | <0.001 | 2.787 | 1.690–4.595 | 103 |
>6 | 0.002 | 2.043 | 1.302–3.205 | 71 |
Unknown | Reference | 0 | ||
T value | 0.75 | |||
TX–T1 | 0.76 | 0.903 | 0.470–1.734 | −10 |
T2–T3 | 0.45 | 0.841 | 0.537–1.318 | −17 |
T4 | Reference | 0 | ||
Regional lymph node Met | <0.001 | |||
No | 0.008 | 2.059 | 1.205–3.518 | 72 |
Yes | 0.88 | 1.045 | 0.588–1.855 | 4 |
Unknown | Reference | 0 | ||
Distant organ Met | 0.01 | |||
No | 0.56 | 1.225 | 0.614–2.444 | 20 |
Yes | 0.33 | 0.699 | 0.343–1.426 | −36 |
Unknown | Reference | 0 |
OR, odds ratio; CI, confidence interval; NOS, not otherwise specific; Met, metastasis.
Validation of the SDS model
A cohort comprising 347 patients was used for the internal validation. While a cohort comprising 101 patients with Masaoka stage III/IV thymus tumors from the First Affiliated Hospital of Guangzhou Medical University was used for the external validation. In accordance with the SDS model, the total score was computed for each patient in both validation cohorts. ROC curves were used to validate the predictive efficacy of the SDS model. The area under the curve (AUC) values for the internal and external validation were 0.795 (95% CI: 0.748–0.842) and 0.732 (95% CI: 0.637–0.828), respectively. These outcomes showed a robust correspondence between the model’s prognostic predictions and actual clinical treatment decisions (see Figure 3).
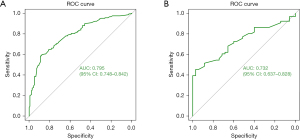
To examine the discriminatory efficacy of the SDS model, Kaplan-Meier analyses were conducted to stratify the patients into high- and low-score groups. The patients were then further subdivided into surgery and non-surgery cohorts. Among the patients from the SEER database in the low-score group, those who underwent surgery had a median OS time of 74.0 months (95% CI: 56.4–91.6), which surpassed the median OS time of 23.0 months (95% CI: 17.2–28.8) recorded for those who did not undergo surgery. Notably, the 3- and 5-year survival rates for the high-score patients who underwent surgery from the SEER database were 67.2% and 46.3%, respectively. Conversely, the median OS time for the high-score patients who did not undergo surgery was 70.0 months (95% CI: 36.8–103.2). Thus, in both the low- and high-score groups from the SEER database, the patients who underwent surgery had a significantly prolonged OS time compared to those who did not undergo surgery (HR: 0.40, 95% CI: 0.32–0.51, P<0.001; HR: 0.37, 95% CI: 0.27–0.51, P<0.001, respectively) (see Figure 4A,4B).
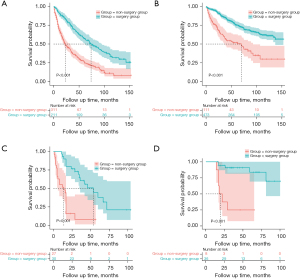
In relation to the external validation cohort, the low-score patients in the surgery and non-surgery groups had median OS times of 14 months (95% CI: 0.4–27.6) and 54 months (95% CI: 32.1–76.0), respectively. While the high-score patients in the non-surgery group had a median OS of 18 months (95% CI: 11.1–24.9). The 3- and 5-year survival rates for the high-score patients in the surgery group were 47.2% and 33.3%, respectively. Notably, consistent with the findings from the SEER cohort, patients from the external validation cohort with both low and high scores benefited significantly from surgical intervention, resulting in significantly prolonged OS times relative to those who did not receive surgical interventions (HR: 0.20, 95% CI: 0.10–0.41, P<0.001; HR: 0.10, 95% CI: 0.03–0.37, P<0.001, respectively) (see Figure 4C,4D).
Clinical implications
The SDS model’s scores were derived from the actual clinical attributes of individual patients, with each variable contributing to an overall score. A higher total score indicated a higher likelihood that a patient would benefit from surgical intervention, leading to improved survival prospects. For example, a 45-year-old male patient diagnosed with undifferentiated type A thymoma at Masaoka stage III and T1N1M0 stage, and a tumor size of 5 cm, had a total score of 173 points. Conversely, a 75-year-old female patient diagnosed with poorly differentiated thymic carcinoma at Masaoka stage IV and T4N1M1 stage, and a tumor size of 7 cm, had a total score of −20 points. Such individualized scores can be used to guide clinical decision making, with higher scores indicating that a patient is more likely to benefit from surgical intervention.
Discussion
Our investigation into advanced-stage thymus tumors emphasized the critical role of surgery in improving OS. This study used insights from previous experiences to provide a comprehensive understanding of the composition of selection bias. More significantly, a groundbreaking quantitative predictive model, the SDS model, was established to aid in identifying which patients with advanced-stage thymus tumors are suitable for surgery. ROC curve and Kaplan-Meier analyses were conducted to examine the robustness and clinical utility of the SDS model, and the results showed its stability and value.
The SDS model includes a range of clinical variables, such as the histological type, differentiation grade, tumor size, T stage, regional LNM status, and the presence of distant organ metastasis, that are instrumental in the decision-making process for surgical intervention. Notably, among these factors, tumor size and regional LNM status emerged as the preeminent factors dictating the choice of surgical intervention. Specifically, patients with smaller primary tumors (<6.1 cm) and no regional LNM were more likely to be recommended for surgical intervention. Notably, the tumor-related attributes, such as the histological type, differentiation grade, tumor size, and T stage, were not found to exert a significant effect on OS in our study.
Other studies have reported similar findings (21-23). For instance, a similar study (21) examining thymic carcinomas and high-risk thymomas highlighted the prognostic relevance of tumor differentiation. Similarly, leveraging an expansive retrospective database from the Chinese Thymomas Alliance, Liu et al. (22) developed a predictive model for Masaoka stage I–IIIa thymic tumors, and found that histological types and elevated T stage exerted a profound effect on tumor recurrence, thereby influencing OS (22). Corroborating these findings, Okumura et al. (23) conducted a retrospective study of thymic tumors, and found that a tumor size >5.0 cm and TNM stage were significant independent predictors of elevated disease-specific survival rates. Together, these findings underscore the significant effect of tumor attributes on patient prognosis.
In our model, the presence of LNM and distant organ metastasis were found to be significant independent factors. Notably, the absence of metastasis conveyed a substantial probability of undergoing surgery, which in turn was correlated with favorable survival outcomes. The intricate interplay between lymph nodes and distant organ involvement underscores their joint significance in the context of advanced-stage thymus tumors. This supports the findings of Song et al. (24) and Lopez et al. (25), who reported an independent association between positive regional LNM and adverse survival outcomes in thymic carcinomas. Moreover, a multicenter prospective observational investigation into thymic malignancies reported that positive LNM had a negative effect on OS (26,27). Research on the relationship between distant organ involvement and OS is limited; however, organ involvement has consistently been found to be an independent predictor of an unfavorable prognosis (28-30). Although these antecedent studies did not explicitly focus on the specific subgroup of advanced-stage thymus tumor patients, their implications resonate significantly with our study. In addition, our study was more convincing than previous researches based on the particular population (advanced-stage thymomas and thymic carcinomas) from the updated SEER database. One strength of our SDS model is that it used variables from both surgery and non-surgery cohorts (the training cohort). Potential institutional and referral bias could be avoided through this approach because there may be selection bias in some medical institutions when choosing treatment for patients.
This study had several limitations. First, we used the Masaoka staging system, as the American Joint Committee on Cancer TNM staging systems could not be used in the SEER database; therefore, we had to approximate Masaoka staging and TNM staging relying on the extension status and metastasis status, deviating from the actual stage of patients. Second, due to the restricted sample size, a detailed analysis of specific surgical procedures as primary outcomes could not be conducted. Third, the lack of granularity in the radiation therapy and chemotherapy data in the SEER database limited our ability to comprehensively account for the effects of these treatments on surgical decision making. This omission introduced a potential bias that might influence surgical selection. Finally, due to the limited sample size, we have had to refrain from making definitive assertions regarding the relationship between specific organ metastasis and surgical decision making.
Conclusions
Our study developed an investigatory methodology to examine the effects of surgery on advanced-stage thymus tumors that included previous surgery selection criteria. In this distinct patient subset, the close relationship between surgery and favorable OS was apparent. Most notably, the SDS model showed exceptional predictive accuracy in determining the propensity for surgery. Notably, patients with moderately differentiated type A thymoma (<6.1 cm) without concurrent regional LNM or distant organ metastasis are likely to benefit from surgical intervention. Pending prospective validation using institutional data, the SDS predictive model has the potential to revolutionize the identification of optimal candidates for surgery.
Acknowledgments
Funding: This work was supported by
Footnote
Reporting Checklist: The authors have completed the TRIPOD reporting checklist. Available at https://gs.amegroups.com/article/view/10.21037/gs-2024-527/rc
Data Sharing Statement: Available at https://gs.amegroups.com/article/view/10.21037/gs-2024-527/dss
Peer Review File: Available at https://gs.amegroups.com/article/view/10.21037/gs-2024-527/prf
Conflicts of Interest: All authors have completed the ICMJE uniform disclosure form (available at https://gs.amegroups.com/article/view/10.21037/gs-2024-527/coif). The authors have no conflicts of interest to declare.
Ethical Statement: The authors are accountable for all aspects of the work in ensuring that questions related to the accuracy or integrity of any part of the work are appropriately investigated and resolved. The study was conducted in accordance with the Declaration of Helsinki (as revised in 2013). The study was approved by The Institutional Ethics Committee of The First Affiliated Hospital of Guangzhou Medical University (No. 2020-138) and informed consent was taken from all the patients.
Open Access Statement: This is an Open Access article distributed in accordance with the Creative Commons Attribution-NonCommercial-NoDerivs 4.0 International License (CC BY-NC-ND 4.0), which permits the non-commercial replication and distribution of the article with the strict proviso that no changes or edits are made and the original work is properly cited (including links to both the formal publication through the relevant DOI and the license). See: https://creativecommons.org/licenses/by-nc-nd/4.0/.
References
- Detterbeck FC, Parsons AM. Thymic tumors. Ann Thorac Surg 2004;77:1860-9. [Crossref] [PubMed]
- Girard N, Mornex F, Van Houtte P, et al. Thymoma: a focus on current therapeutic management. J Thorac Oncol 2009;4:119-26. [Crossref] [PubMed]
- de Jong WK, Blaauwgeers JL, Schaapveld M, et al. Thymic epithelial tumours: a population-based study of the incidence, diagnostic procedures and therapy. Eur J Cancer 2008;44:123-30. [Crossref] [PubMed]
- Gerber TS, Strobl S, Marx A, et al. Epidemiology of thymomas and thymic carcinomas in the United States and Germany, 1999-2019. Front Oncol 2023;13:1308989. [Crossref] [PubMed]
- Bergh NP, Gatzinsky P, Larsson S, et al. Tumors of the thymus and thymic region: I. Clinicopathological studies on thymomas. Ann Thorac Surg 1978;25:91-8. [Crossref] [PubMed]
- Masaoka A, Monden Y, Nakahara K, et al. Follow-up study of thymomas with special reference to their clinical stages. Cancer 1981;48:2485-92. [Crossref] [PubMed]
- Yamakawa Y, Masaoka A, Hashimoto T, et al. A tentative tumor-node-metastasis classification of thymoma. Cancer 1991;68:1984-7. [Crossref] [PubMed]
- Tsuchiya R, Koga K, Matsuno Y, et al. Thymic carcinoma: proposal for pathological TNM and staging. Pathol Int 1994;44:505-12. [Crossref] [PubMed]
- Bedini AV, Andreani SM, Tavecchio L, et al. Proposal of a novel system for the staging of thymic epithelial tumors. Ann Thorac Surg 2005;80:1994-2000. [Crossref] [PubMed]
- Kondo K. Tumor-node metastasis staging system for thymic epithelial tumors. J Thorac Oncol 2010;5:S352-6. [Crossref] [PubMed]
- Rea F, Marulli G, Girardi R, et al. Long-term survival and prognostic factors in thymic epithelial tumours. Eur J Cardiothorac Surg 2004;26:412-8. [Crossref] [PubMed]
- Fu H, Gu ZT, Fang WT, et al. Long-Term Survival After Surgical Treatment of Thymic Carcinoma: A Retrospective Analysis from the Chinese Alliance for Research of Thymoma Database. Ann Surg Oncol 2016;23:619-25. [Crossref] [PubMed]
- NCCN Guidelines for thymomas and thymic carcinomas. Available online: https://www.nccn.org/professionals/physician_gls/pdf/thymic.pdf. Accessed October 5, 2021.
- Huang Q, Zhu L, Liu Y, et al. Thymic epithelial tumor medical treatment: A narrative review. Biochim Biophys Acta Rev Cancer 2024;1879:189167. [Crossref] [PubMed]
- Li D, Minervini F, Planas G, et al. Stage III-IV thymic squamous cell carcinoma in complete pathological remission achieved with thymic cancer resection after immunotherapy combined with chemotherapeutic conversion therapy: a report of two cases from real-world data. Gland Surg 2024;13:117-27. [Crossref] [PubMed]
- Zhao K, Liu Y, Jing M, et al. Long-term survival and prognosis after surgical treatment of patients with thymic carcinoma: a retrospective analysis. J Thorac Dis 2024;16:7582-91. [Crossref] [PubMed]
- Benjamin DJ, Klapheke A, Lara PN, et al. A Population-Based Study of Incidence and Survival of 1588 Thymic Malignancies: Results From the California Cancer Registry. Clin Lung Cancer 2019;20:477-83. [Crossref] [PubMed]
- Okuma Y, Horio H, Hosomi Y, et al. The potency of curative-intent treatment for advanced thymic carcinoma. Lung Cancer 2014;84:175-81. [Crossref] [PubMed]
- Chiappetta M, Sassorossi C, Nachira D, et al. Survival Outcome After Surgery in Patients With Thymoma Distant Recurrence. J Thorac Oncol 2024;19:1086-94. [Crossref] [PubMed]
- Fabre D, Fadel E, Mussot S, et al. Long-term outcome of pleuropneumonectomy for Masaoka stage IVa thymoma. Eur J Cardiothorac Surg 2011;39:e133-8. [Crossref] [PubMed]
- Dai H, Huang Y, Xiao G, et al. Predictive Features of Thymic Carcinoma and High-Risk Thymomas Using Random Forest Analysis. J Comput Assist Tomogr 2020;44:857-64. [Crossref] [PubMed]
- Liu H, Gu Z, Qiu B, et al. A Recurrence Predictive Model for Thymic Tumors and Its Implication for Postoperative Management: a Chinese Alliance for Research in Thymomas Database Study. J Thorac Oncol 2020;15:448-56. [Crossref] [PubMed]
- Okumura M, Yoshino I, Yano M, et al. Tumour size determines both recurrence-free survival and disease-specific survival after surgical treatment for thymoma. Eur J Cardiothorac Surg 2019;56:174-81. [Crossref] [PubMed]
- Song Q, Zhang LL, Qi Y, et al. Effect of clinicopathologic features on survival of patients with thymic carcinomas and thymic neuroendocrine tumors: A population-based analysis. Curr Probl Cancer 2019;43:411-20. [Crossref] [PubMed]
- Lopez H, Botticella A, Belkhir F, et al. Postoperative radiotherapy results in 192 epithelial thymic tumours patients with 10 years of follow-up. Radiother Oncol 2024;195:110272. [Crossref] [PubMed]
- Fang W, Wang Y, Pang L, et al. Lymph node metastasis in thymic malignancies: A Chinese multicenter prospective observational study. J Thorac Cardiovasc Surg 2018;156:824-833.e1. [Crossref] [PubMed]
- Fang W, Girard N, Cilento V, et al. The International Association for the Study of Lung Cancer Thymic Epithelial Tumors Staging Project: Proposals for the N and the M Components for the Forthcoming (Ninth) Edition of the TNM Classification of Malignant Tumors. J Thorac Oncol 2024;19:52-70. [Crossref] [PubMed]
- Wick MR. Prognostic factors for thymic epithelial neoplasms, with emphasis on tumor staging. Hematol Oncol Clin North Am 2008;22:527-42. [Crossref] [PubMed]
- Dai H, Lan B, Li S, et al. Prognostic CT features in patients with untreated thymic epithelial tumors. Sci Rep 2023;13:2910. [Crossref] [PubMed]
- Chiappetta M, Lococo F, Sassorossi C, et al. The Prognostic Role of the Number of Involved Structures in Thymic Epithelial Tumors: Results from the ESTS Database. Ann Surg Oncol 2024;31:4298-307. [Crossref] [PubMed]
(English Language Editor: L. Huleatt)