Construction, validation, and visualization of a web-based nomogram to predict survival in male breast cancer patients with second primary prostate cancer
Highlight box
Key findings
• Our study analyzed a total of 885 male patients with primary breast cancer (BC) and a second primary malignancy (SPM). We found that second primary prostate cancer (spPCa) is a key factor affecting prognosis. Additionally, we developed a web-based nomogram, a visual prediction tool, to estimate the prognosis of male breast cancer (MBC) patients with spPCa.
What is known and what is new?
• Both MBC and prostate cancer (PCa) are hormone-dependent cancers; the risk of developing PCa is increased after BC, and that of BC is increased after PCa.
• MBC patients with spPCa have a better prognosis compared to those with other SPMs. Our study introduces a novel, web-based nomogram that visually predicts MBC prognosis based on the presence of spPCa, providing an innovative tool for clinical use.
What is the implication, and what should change now?
• On the one hand, our prediction model provides an effective tool for evaluating the survival of MBC patients. On the other hand, further research is needed to explore the underlying mechanisms of the interaction between BC and PCa in men, as this remains an area requiring deeper investigation in the future.
Introduction
Male breast cancer (MBC) is an exceptional rarity, comprising a mere 1% of all breast cancers (BCs) and a minuscule 0.003% of malignancies affecting the male population (1). Due to its rarity and limited research data, the study of MBC remains challenging. In recent years, there has been an undeniable increase in the incidence of MBC (2). This phenomenon has sparked widespread attention and in-depth research, aiming to better understand the etiology, diagnostic methods, and treatment strategies for this disease (3). Elevated levels of estrogen and hormonal imbalance are widely recognized as risk factors for the progression of BC in males (4-6). Additionally, genetic elements may be involved, with breast cancer 2 (BRCA2) mutations emerging as the most influential factor for MBC, presenting a nearly 80 times greater risk than that of non-mutations (7). In addition, progress in early detection and therapies has led to a significant percentage of MBC survivors. Among these survivors, the occurrence of a second primary malignancy (SPM) represents one of the most potentially perilous consequences (8).
Prostate cancer (PCa) is the most common male cancer and the third-leading cause of cancer death in men (9). The second primary prostate cancer (spPCa) represents a crucial subclass of SPM (10). A study showed that PCa was increased after BC and BC was increased after PCa (11). However, except for individuals carrying susceptibility alleles of BRCA2 and breast cancer 1 (BRCA1), these hormonal-dependent cancers do not share any known risk factors. MBC risks include obesity, physical inactivity, family history of BC, and other hormone-related conditions such as Klinefelter syndrome, orchitis/epididymitis, and gynecomastia (12). Age, race, family history, and germline mutations are known risk factors for PCa (13). To date, the investigation of spPCa in MBC patients has been inadequate, with limited studies exploring its development, and its impact on prognosis remains uncertain. Since PCa is usually considered “indolent” and patients have high prognostic expectations for these two hormonally-dependent tumors, a straightforward and efficient predictive model is urgently needed to help clinicians make clinical decisions (9).
In recent times, nomograms have gained widespread use in the domain of personalized prognostication for numerous malignant neoplasms, having also demonstrated substantial predictive efficacy in anticipating the prognosis of SPM (14,15). This study aimed to assess the prognosis of spPCa in MBC patients who experienced an SPM. We also developed nomograms that could predict the overall survival (OS) and cancer-specific survival (CSS) of MBC patients with spPCa utilizing the Surveillance, Epidemiology, and End Results (SEER) database. We present this article in accordance with the TRIPOD reporting checklist (available at https://gs.amegroups.com/article/view/10.21037/gs-24-287/rc).
Methods
Data sources
The SEER database is a comprehensive cancer database covering approximately 30% of the US population and includes data from 17 cancer centers. Data for MBC patients who experienced an SPM between 2000 and 2020 were extracted using the SEER database and SEER*Stat software (version 8.4.1.2; http://seer.cancer.gov/). The research approach used in this investigation followed the research guidelines issued by the SEER database. We used site code C50 (including C50.1 to C50.9) for screening BC and site code C61.9 for PCa by the International Classification of Diseases for Oncology third edition (ICD-O-3). The determination of SPM status through the variables “sequence number”, “diagnosis year”, and “total number of in situ/malignant tumors for patients”. Synchronous cancers with MBC diagnosed within two months were excluded from being diagnosed as SPM.
The study was conducted in accordance with the Declaration of Helsinki (as revised in 2013). This article does not contain any studies with human participants or animals so was exempt from the requirement institutional review board approval. The obtainment of informed consent from study participants was not required as this was a retrospective analysis of an existing database.
Data collection
The variables included in this study encompass demographic characteristics (age, race, marital status), tumor characteristics including second primary cancer, laterality, pathological type, grade, American Joint Committee on Cancer (AJCC) stage, tumor size, estrogen receptor (ER), progesterone receptor (PR), human epidermal growth factor receptor 2 (HER2), treatment information (surgery, chemotherapy, radiation therapy), and prognosis information (survival status, cause of death, and survival time). The inclusion criterion was male patients diagnosed with BC histologically between 2000 and 2020. The exclusion criteria were as follows: (I) only with BC or BC as a non-first primary malignancy; (II) three or more primary cancers; (III) cases lacking important variables with incomplete information; (IV) survival duration of less than one month or unknown survival duration; and (V) the laterality is paired site. The flowchart of patient selection and study process is illustrated in Figure 1.
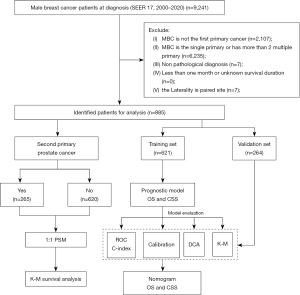
Clinical variables and outcomes
This study examined clinical variables such as age, marital status, race, laterality, pathological type, grade, AJCC stage, tumor size, ER, PR, HER2, spPCa, and treatment methods (surgery, radiation therapy, and chemotherapy). The outcomes included OS and CSS. OS was defined as the time from diagnosis to death from any cause or the end of the follow-up period, whereas CSS was defined as the time from diagnosis to death from the cancer or the end of the follow-up period.
Statistical analysis
The Fisher’s exact test and chi-squared test were used to compare categorical variables. The Kaplan-Meier method and log-rank test were constructed to estimate the OS and CSS variances for MBC survivors with spPCa or other SPMs.
Propensity score matching (PSM) was used to balance the baseline characteristics for patients with PC or other malignancies as SPM. Simultaneously, the entire group was randomly split into a training subset (70%) and a validation subset (30%). Univariate and multivariate Cox regression analyses were initially conducted to discern variables that significantly impacted the OS and CSS of male patients who have undergone primary BC and experienced an SPM, subsequently constructing two nomograms.
Harrell’s concordance index (C-index) and receiver operating characteristic (ROC) curve were used to evaluate the discriminatory ability of the nomogram; calibration curves were used to validate the consistency of the nomogram. Decision curve analysis (DCA) was used to evaluate the clinical utility of the established nomograms and to compare the differences between the nomogram and the traditional AJCC staging model at different probability thresholds by quantifying net benefits. Furthermore, optimal cutoff values of the models were calculated to analyze the difference in survival time among groups at different risks using the Kaplan-Meier method. All statistical analyses were performed using R software (version 4.1.3; R Foundation for Statistical Computing, Vienna, Austria). A two-sided P<0.05 was considered statistically significant.
Results
Clinicopathological characteristics
A total of 885 male patients with first primary BC and concomitant SPM were selected from the SEER database. Among them, there were 265 cases of spPCa, accounting for 29.9% of the total. The optimal cutoff values for age and tumor size were determined using X-tile software (Yale School of Medicine, New Haven, CT, USA) to achieve discretization of age and tumor size. Patients aged ≤77 years accounted for 82.825% of the total, whereas patients with tumor size ≤3 cm accounted for 57.288%. In terms of histological type, invasive ductal carcinoma was the most common (81.017%), and grade and AJCC stage II were the most frequent. Regarding treatment, the majority of patients (96.045%) underwent surgery, a minority received chemotherapy (38.418%), and only 24.294% received radiation therapy. All patients were divided using a 7:3 random stratification method, and the resulting training and validation sets were comparable (P>0.05), as shown in Table 1.
Table 1
Variables | Total (n=885) | Training set (n=621) | Validation set (n=264) | P value |
---|---|---|---|---|
Status | >0.99 | |||
Alive | 407 (45.989) | 286 (46.055) | 121 (45.833) | |
Dead | 478 (54.011) | 335 (53.945) | 143 (54.167) | |
Survival time (months) | 100 [51–150] | 99 [52–154] | 101 [51–140] | 0.32 |
PCa | 0.30 | |||
No | 620 (70.056) | 442 (71.176) | 178 (67.424) | |
Yes | 265 (29.944) | 179 (28.824) | 86 (32.576) | |
Age | 0.54 | |||
≤77 years | 733 (82.825) | 518 (83.414) | 215 (81.439) | |
>77 years | 152 (17.175) | 103 (16.586) | 49 (18.561) | |
Marital status | 0.76 | |||
Unmarried | 89 (10.056) | 60 (9.662) | 29 (10.985) | |
Married | 643 (72.655) | 451 (72.625) | 192 (72.727) | |
SDWU | 153 (17.288) | 110 (17.713) | 43 (16.288) | |
Race | 0.41 | |||
White | 745 (84.181) | 522 (84.058) | 223 (84.47) | |
Black | 103 (11.638) | 76 (12.238) | 27 (10.227) | |
Other | 37 (4.181) | 23 (3.704) | 14 (5.303) | |
Laterality | 0.14 | |||
Left | 481 (54.35) | 348 (56.039) | 133 (50.379) | |
Right | 404 (45.65) | 273 (43.961) | 131 (49.621) | |
Histological | >0.99 | |||
Infiltrating ductal carcinoma | 717 (81.017) | 503 (80.998) | 214 (81.061) | |
Other | 168 (18.983) | 118 (19.002) | 50 (18.939) | |
Grade | 0.33 | |||
I | 117 (13.22) | 86 (13.849) | 31 (11.742) | |
II | 413 (46.667) | 293 (47.182) | 120 (45.455) | |
III/IV | 229 (25.876) | 162 (26.087) | 67 (25.379) | |
Unknown | 126 (14.237) | 80 (12.882) | 46 (17.424) | |
AJCC | 0.48 | |||
I | 324 (36.61) | 218 (35.105) | 106 (40.152) | |
II | 360 (40.678) | 257 (41.385) | 103 (39.015) | |
III/IV | 145 (16.384) | 107 (17.23) | 38 (14.394) | |
Unknown | 56 (6.328) | 39 (6.28) | 17 (6.439) | |
Tumor size | 0.69 | |||
≤3 cm | 507 (57.288) | 350 (56.361) | 157 (59.47) | |
>3 cm | 108 (12.203) | 77 (12.399) | 31 (11.742) | |
Unknown | 270 (30.508) | 194 (31.24) | 76 (28.788) | |
ER | 0.89 | |||
Negative | 21 (2.373) | 15 (2.415) | 6 (2.273) | |
Positive | 757 (85.537) | 533 (85.829) | 224 (84.848) | |
Unknown | 107 (12.09) | 73 (11.755) | 34 (12.879) | |
PR | 0.92 | |||
Negative | 79 (8.927) | 57 (9.179) | 22 (8.333) | |
Positive | 692 (78.192) | 484 (77.939) | 208 (78.788) | |
Unknown | 114 (12.881) | 80 (12.882) | 34 (12.879) | |
HER2 | 0.15 | |||
Negative | 261 (29.492) | 194 (31.24) | 67 (25.379) | |
Positive | 31 (3.503) | 19 (3.06) | 12 (4.545) | |
Unknown | 593 (67.006) | 408 (65.7) | 185 (70.076) | |
Surgery | 0.98 | |||
No | 35 (3.955) | 24 (3.865) | 11 (4.167) | |
Yes | 850 (96.045) | 597 (96.135) | 253 (95.833) | |
Chemotherapy | 0.22 | |||
No/unknown | 545 (61.582) | 391 (62.963) | 154 (58.333) | |
Yes | 340 (38.418) | 230 (37.037) | 110 (41.667) | |
Radiation | 0.33 | |||
No/unknown | 670 (75.706) | 464 (74.718) | 206 (78.03) | |
Yes | 215 (24.294) | 157 (25.282) | 58 (21.97) |
Data are presented as n (%) or median [interquartile range]. PCa, prostate cancer; SDWU, separated, divorced, widowed, or unmarried; AJCC, American Joint Committee on Cancer; ER, estrogen receptor; PR, progesterone receptor; HER2, human epidermal growth factor receptor 2.
Differences in survival between PCa and non-PCa as SPM of MBC
Initially, a comparison of the baseline data was conducted between PCa and non-PCa patients, revealing significant differences in some variables (Table S1). Then PSM (1:1) was performed on the baseline data of PCa and non-PCa groups, resulting in the selection of 257 pairs of comparable patient data, the clinical characteristics of which showed no statistical differences (Table 2).
Table 2
Variables | Total (n=514) | Non-PC (n=257) | PC (n=257) | P value |
---|---|---|---|---|
Status | <0.001 | |||
Alive | 280 (54.475) | 107 (41.634) | 173 (67.315) | |
Dead | 234 (45.525) | 150 (58.366) | 84 (32.685) | |
Survival time (months) | 112.5 [62–158] | 104 [58–148] | 123 [66–167] | 0.008 |
Age | 0.87 | |||
≤77 years | 472 (91.829) | 237 (92.218) | 235 (91.44) | |
>77 years | 42 (8.171) | 20 (7.782) | 22 (8.56) | |
Marital status | 0.83 | |||
Unmarried | 49 (9.533) | 23 (8.949) | 26 (10.117) | |
Married | 374 (72.763) | 190 (73.93) | 184 (71.595) | |
SDWU | 91 (17.704) | 44 (17.121) | 47 (18.288) | |
Race | 0.39 | |||
White | 439 (85.409) | 225 (87.549) | 214 (83.268) | |
Black | 58 (11.284) | 25 (9.728) | 33 (12.84) | |
Other | 17 (3.307) | 7 (2.724) | 10 (3.891) | |
Laterality | 0.93 | |||
Left | 290 (56.42) | 146 (56.809) | 144 (56.031) | |
Right | 224 (43.58) | 111 (43.191) | 113 (43.969) | |
Histological | >0.99 | |||
Infiltrating ductal carcinoma | 415 (80.739) | 208 (80.934) | 207 (80.545) | |
Other | 99 (19.261) | 49 (19.066) | 50 (19.455) | |
Grade | 0.87 | |||
I | 79 (15.37) | 38 (14.786) | 41 (15.953) | |
II | 236 (45.914) | 120 (46.693) | 116 (45.136) | |
III/IV | 140 (27.237) | 72 (28.016) | 68 (26.459) | |
Unknown | 59 (11.479) | 27 (10.506) | 32 (12.451) | |
AJCC | 0.90 | |||
I | 200 (38.911) | 104 (40.467) | 96 (37.354) | |
II | 224 (43.58) | 110 (42.802) | 114 (44.358) | |
III/IV | 61 (11.868) | 29 (11.284) | 32 (12.451) | |
Unknown | 29 (5.642) | 14 (5.447) | 15 (5.837) | |
Tumor size | 0.54 | |||
≤3 cm | 303 (58.949) | 148 (57.588) | 155 (60.311) | |
>3 cm | 58 (11.284) | 27 (10.506) | 31 (12.062) | |
Unknown | 153 (29.767) | 82 (31.907) | 71 (27.626) | |
ER | 0.73 | |||
Negative | 12 (2.335) | 6 (2.335) | 6 (2.335) | |
Positive | 459 (89.3) | 232 (90.272) | 227 (88.327) | |
Unknown | 43 (8.366) | 19 (7.393) | 24 (9.339) | |
PR | 0.53 | |||
Negative | 45 (8.755) | 22 (8.56) | 23 (8.949) | |
Positive | 424 (82.49) | 216 (84.047) | 208 (80.934) | |
Unknown | 45 (8.755) | 19 (7.393) | 26 (10.117) | |
HER2 | 0.50 | |||
Negative | 154 (29.961) | 77 (29.961) | 77 (29.961) | |
Positive | 19 (3.696) | 7 (2.724) | 12 (4.669) | |
Unknown | 341 (66.342) | 173 (67.315) | 168 (65.37) | |
Surgery | >0.99 | |||
No | 13 (2.529) | 7 (2.724) | 6 (2.335) | |
Yes | 501 (97.471) | 250 (97.276) | 251 (97.665) | |
Chemotherapy | >0.99 | |||
No/unknown | 294 (57.198) | 147 (57.198) | 147 (57.198) | |
Yes | 220 (42.802) | 110 (42.802) | 110 (42.802) | |
Radiation | 0.66 | |||
No/unknown | 407 (79.183) | 206 (80.156) | 201 (78.21) | |
Yes | 107 (20.817) | 51 (19.844) | 56 (21.79) |
Data are presented as n (%) or median [interquartile range]. PCa, prostate cancer; PSM, propensity score matching; SDWU, separated, divorced, widowed, or unmarried; AJCC, American Joint Committee on Cancer; ER, estrogen receptor; PR, progesterone receptor; HER2, human epidermal growth factor receptor 2.
Survival analysis was conducted on both pre-PSM and post-PSM between PCa and non-PCa patients. As shown in Figures 2,3, there was a significant difference in OS and CSS before and after PSM. The results revealed that MBC patients who experienced a spPCa had an evidently longer OS and CSS than those with other SPMs.
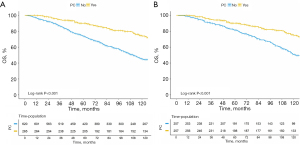
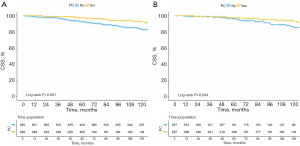
Predictors for OS and CSS among MBC patients with SPM
The univariate Cox regression analysis was employed to screen for relevant prognostic factors, which were then included in the multivariate Cox analysis (Tables 3,4). Independent protective factors for OS of MBC patients with SPM included PCa [hazard ratio (HR): 0.508, 95% confidence interval (CI): 0.386–0.668, P<0.001], age >77 years old (HR: 3.002, 95% CI: 2.257–3.995, P<0.001), other race (HR: 0.400, 95% CI: 0.177–0.907, P=0.03), tumor size >3 cm (HR: 2.267, 95% CI: 1.594–3.225, P<0.001), HER2+ (HR: 0.731, 95% CI: 0.537–0.996, P=0.047), surgery (HR: 0.442, 95% CI: 0.251–0.777, P=0.005), and chemotherapy (HR: 0.720, 95% CI: 0.558–0.931, P=0.01) (Table 3); those for CSS included PC (HR: 0.442, 95% CI: 0.242–0.810, P=0.008), age >77 years old (HR: 1.964, 95% CI: 1.010–3.819, P=0.047), marital status (married vs. unmarried, HR: 0.453, 95% CI: 0.252–0.814, P=0.008), tumor size >3 cm (HR: 3.997, 95% CI: 2.136–7.478, P<0.001), PR unknown (HR: 0.322, 95% CI: 0.110–0.940, P=0.04) and surgery (HR: 0.186, 95% CI: 0.077–0.446, P<0.001) (Table 4).
Table 3
Variables | Univariate | Multivariate | |||||
---|---|---|---|---|---|---|---|
HR | 95% CI | P value | HR | 95% CI | P value | ||
PCa | |||||||
No | 1.000 | 1.000 | |||||
Yes | 0.469 | 0.358–0.615 | <0.001 | 0.508 | 0.386–0.668 | <0.001 | |
Age | |||||||
≤77 years | 1.000 | 1.000 | |||||
>77 years | 3.981 | 3.059–5.181 | <0.001 | 3.002 | 2.257–3.995 | <0.001 | |
Marital status | |||||||
Unmarried | 1.000 | ||||||
Married | 0.904 | 0.618–1.322 | 0.60 | ||||
SDWU | 1.373 | 0.892–2.112 | 0.15 | ||||
Race | |||||||
White | 1.000 | 1.000 | |||||
Black | 1.046 | 0.756–1.447 | 0.79 | 1.075 | 0.771–1.501 | 0.67 | |
Other | 0.418 | 0.186–0.937 | 0.03 | 0.400 | 0.177–0.907 | 0.03 | |
Laterality | |||||||
Left | 1.000 | ||||||
Right | 0.945 | 0.761–1.173 | 0.61 | ||||
Histological | |||||||
Infiltrating duct carcinoma | 1.000 | ||||||
Other | 0.994 | 0.752–1.312 | 0.96 | ||||
Grade | |||||||
I | 1.000 | ||||||
II | 1.015 | 0.731–1.411 | 0.93 | ||||
III/IV | 1.074 | 0.75–1.536 | 0.70 | ||||
Unknown | 1.035 | 0.652–1.643 | 0.89 | ||||
AJCC | |||||||
I | 1.000 | 1.000 | |||||
II | 1.219 | 0.947–1.567 | 0.12 | 1.208 | 0.924–1.58 | 0.17 | |
III/IV | 1.301 | 0.947–1.788 | 0.10 | 1.168 | 0.827–1.649 | 0.38 | |
Unknown | 1.712 | 1.106–2.650 | 0.02 | 1.246 | 0.725–2.14 | 0.43 | |
Tumor size | |||||||
≤3 cm | 1.000 | 1.000 | |||||
>3 cm | 2.329 | 1.680–3.228 | <0.001 | 2.267 | 1.594–3.225 | <0.001 | |
Unknown | 0.951 | 0.744–1.215 | 0.69 | 1.000 | 0.757–1.321 | >0.99 | |
ER | |||||||
Negative | 1.000 | ||||||
Positive | 1.144 | 0.566–2.312 | 0.71 | ||||
Unknown | 1.152 | 0.545–2.435 | 0.71 | ||||
PR | |||||||
Negative | 1.000 | ||||||
Positive | 1.264 | 0.855–1.868 | 0.24 | ||||
Unknown | 1.232 | 0.779–1.948 | 0.37 | ||||
HER2 | |||||||
Negative | 1.000 | 1.000 | |||||
Positive | 1.471 | 0.734–2.947 | 0.28 | 1.311 | 0.642–2.675 | 0.46 | |
Unknown | 0.672 | 0.505–0.893 | 0.006 | 0.731 | 0.537–0.996 | 0.047 | |
Surgery | |||||||
No | 1.000 | 1.000 | |||||
Yes | 0.326 | 0.205–0.520 | <0.001 | 0.442 | 0.251–0.777 | 0.005 | |
Chemotherapy | |||||||
No/unknown | 1.000 | 1.000 | |||||
Yes | 0.650 | 0.516–0.820 | <0.001 | 0.720 | 0.558–0.931 | 0.01 | |
Radiation | |||||||
No/unknown | 1.000 | ||||||
Yes | 0.861 | 0.668–1.110 | 0.25 |
OS, overall survival; MBC, male breast cancer; SPM, second primary malignancy; HR, hazard ratio; CI, confidence interval; PCa, prostate cancer; SDWU, separated, divorced, widowed, or unmarried; AJCC, American Joint Committee on Cancer; ER, estrogen receptor; PR, progesterone receptor; HER2, human epidermal growth factor receptor 2.
Table 4
Variables | Univariate | Multivariate | |||||
---|---|---|---|---|---|---|---|
HR | 95% CI | P value | HR | 95% CI | P value | ||
PC | |||||||
No | 1.000 | 1.000 | |||||
Yes | 0.419 | 0.23–0.763 | 0.004 | 0.442 | 0.242–0.810 | 0.008 | |
Age | |||||||
≤77 years | 1.000 | 1.000 | |||||
>77 years | 2.091 | 1.114–3.928 | 0.02 | 1.964 | 1.010–3.819 | 0.047 | |
Marital status | |||||||
Unmarried | 1.000 | 1.000 | |||||
Married | 0.365 | 0.207–0.642 | <0.001 | 0.453 | 0.252–0.814 | 0.008 | |
SDWU | 0.388 | 0.176–0.855 | 0.02 | 0.429 | 0.188–0.981 | 0.045 | |
Race | |||||||
White | 1.000 | ||||||
Black | 1.159 | 0.594–2.261 | 0.67 | ||||
Other | 0.971 | 0.305–3.095 | 0.96 | ||||
Laterality | |||||||
Left | 1.000 | ||||||
Right | 0.896 | 0.566–1.419 | 0.64 | ||||
Histological | |||||||
Infiltrating duct carcinoma | 1.000 | ||||||
Other | 1.102 | 0.625–1.941 | 0.74 | ||||
Grade | |||||||
I | 1.000 | ||||||
II | 1.193 | 0.578–2.463 | 0.63 | ||||
III/IV | 1.155 | 0.523–2.554 | 0.72 | ||||
Unknown | 1.389 | 0.535–3.603 | 0.50 | ||||
AJCC | |||||||
I | 1.000 | 1.000 | |||||
II | 1.366 | 0.756–2.471 | 0.30 | 1.046 | 0.562–1.949 | 0.89 | |
III/IV | 2.919 | 1.584–5.379 | 0.001 | 1.771 | 0.913–3.432 | 0.09 | |
Unknown | 1.901 | 0.705–5.126 | 0.20 | 1.244 | 0.365–4.238 | 0.73 | |
Tumor size | |||||||
≤3 cm | 1.000 | 1.000 | |||||
>3 cm | 4.693 | 2.622–8.401 | <0.001 | 3.997 | 2.136–7.478 | <0.001 | |
Unknown | 1.137 | 0.661–1.957 | 0.64 | 1.255 | 0.704–2.236 | 0.44 | |
ER | |||||||
Negative | 1.000 | ||||||
Positive | 0.683 | 0.214–2.176 | 0.52 | ||||
Unknown | 0.523 | 0.139–1.973 | 0.34 | ||||
PR | |||||||
Negative | 1.000 | 1.000 | |||||
Positive | 0.561 | 0.306–1.03 | 0.06 | 0.674 | 0.357–1.275 | 0.23 | |
Unknown | 0.409 | 0.169–0.987 | 0.05 | 0.322 | 0.110–0.940 | 0.04 | |
HER2 | |||||||
Negative | 1.000 | ||||||
Positive | 2.105 | 0.613–7.228 | 0.24 | ||||
Unknown | 0.750 | 0.419–1.343 | 0.33 | ||||
Surgery | |||||||
No | 1.000 | 1.000 | |||||
Yes | 0.201 | 0.092–0.44 | <0.001 | 0.186 | 0.077–0.446 | <0.001 | |
Chemotherapy | |||||||
No/unknown | 1.000 | ||||||
Yes | 1.259 | 0.799–1.983 | 0.32 | ||||
Radiation | |||||||
No/unknown | 1.000 | ||||||
Yes | 1.263 | 0.773–2.064 | 0.35 |
CSS, cancer-specific survival; MBC, male breast cancer; SPM, second primary malignancy; HR, hazard ratio; CI, confidence interval; PC, prostate cancer; SDWU, separated, divorced, widowed, or unmarried; AJCC, American Joint Committee on Cancer; ER, estrogen receptor; PR, progesterone receptor; HER2, human epidermal growth factor receptor 2.
Nomograms construction
Two nomograms were constructed based on the aforementioned independent prognostic factors to illustrate the 3-, 5-, 8-, and 10-year OS and CSS of MBC patients who experienced an SPM (Figure 4). Additionally, for clinical convenience, two web-based nomograms were developed in a graphical format to predict the OS and CSS of MBC patients with an SPM (the nomogram for predicting OS: https://mbcpre.shinyapps.io/DynNomapp_OS/; the nomogram for predicting CSS: https://mbcpre.shinyapps.io/DynNomapp_CSS/).
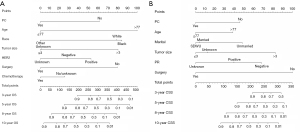
Nomograms validation
Internal cross-validation was used to assess the accuracy and discriminability of the models. C-index values of predicting OS in training and validation sets were 0.707 (95% CI: 0.678–0.736) and 0.694 (95% CI: 0.648–0.740), respectively. C-index values of predicting CSS in training and validation sets were 0.719 (95% CI: 0.654–0.784) and 0.727 (95% CI: 0.639–0.815), respectively. The results indicated that the two nomograms possessed strong identification ability. The calibration curves showed a high degree of consistency between the training and validation sets in predicted and actual values (Figure 5).
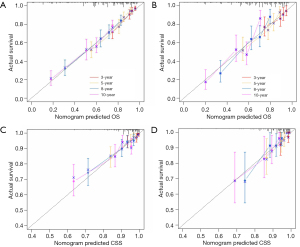
The 3-, 5-, 8-, and 10-year ROC curves and their areas under the curve (AUCs) for the two nomograms can be seen in Figure 6. The AUC values in the training set for predicting OS were 0.790, 0.795, 0.763, and 0.775, respectively. In the validation set, the AUC values were 0.679, 0.740, 0.756, and 0.776, respectively. Meanwhile, in the training set for predicting CSS, the AUC values were 0.816, 0.764, 0.749, and 0.714, respectively. In the validation set, the AUC values were 0.737, 0.794, 0.755, and 0.756, respectively. From the C-index, calibration curve, and AUC value, it can be inferred that the nomograms exhibit ample discriminative capability and are capable of making predictions.
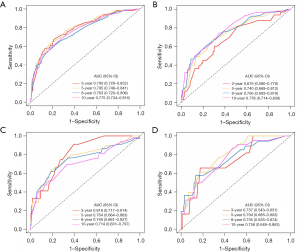
Clinical application of the nomograms
DCA indicated that the nomograms have significant clinical potential value, surpassing the application value of the traditional AJCC stage. Whether in predicting OS or CSS, the model performed well in both the training and validation sets (Figure 7).
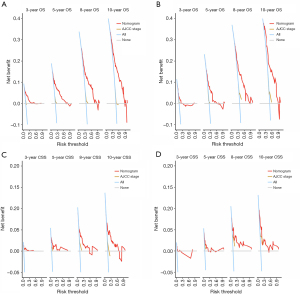
Based on the nomograms, the risk scores and optimal cutoff values were calculated for each patient. Patients were divided into groups at high-risk (total score ≥174), intermediate-risk (137< total score <174), and low-risk (total score ≤137) for predicting OS. For predicting CSS, patients were divided into groups at high-risk (total score ≥147), intermediate-risk (100< total score <147), and low-risk (total score ≤100). According to Kaplan-Meier curves, the high-, intermediate-, and low-risk groups of patients had significantly different OS and CSS (Figure 8).
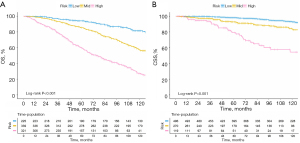
Discussion
In this study, MBC patients who experienced spPCa had significantly longer OS and CSS than those who experienced other SPMs. Multivariate analysis identified independent risk factors for OS (PCa, age, race, tumor size, HER2, surgery, chemotherapy) and CSS (PCa, age, marital, tumor size, PR, surgery). The quantified nomograms presented based on the independent risk factors performed well in prediction ability. Patients with high-risk nomograms showed shorter survival.
MBC is a rare condition with causes that are not fully understood. The treatment of MBC largely follows the guidelines for female BC due to the lack of large-scale randomized prospective studies (16). These patients undergo a series of treatments such as surgery, chemotherapy, radiation therapy, endocrine therapy, targeted therapy, and immunotherapy, which effectively extend their survival period. For these patients, the subsequent concern is the progression of SPM (17). Recently, there have been some reports on the prognosis research of MBC by nomograms. Zhang et al. (18) established a prediction model for predicting patients with advanced MBC based on the SEER database. A model to predict the prognosis of MBC was constructed by Chen et al. (19). Another study developed a nomogram to predict the prognosis of MBC with SPMs via 64 MBC patients who had developed SPMs. In this study, patients with SPMs manifested longer survival (20). These findings suggest that the accumulation rate of SPM is elevated as the duration of survival increases, which implies that the emergence of SPMs may be attributable to an earlier and more readily treatable form of MBC. Our study exclusively included MBC patients who had developed spPCa, allowing for a more focused discussion on subtypes.
There is a discernible correlation between MBC and PCa, potentially attributed to shared environmental, biological, or genetic similarities between these two conditions. Estrogen is a key player in both types of cancer; almost all MBC patients are accompanied by positive ER and PR, and activation of ERs also promotes the progression of PCa (21-23). Recently, a study suggested that selective estrogen receptor modulators (SERMs), which have been used to treat BC, may contribute to PCa treatment by regulating the tumor immune microenvironment (24). Furthermore, androgens in males have been found to play a role in the development of BC and are well known to have essential impact on PCa (25,26). In our study, the survival of spPCa was longer, not only because PCa is more indolent than other cancers but also possibly because the treatment of BC and PCa may potentially synergize and mutually enhance, leading to prolonged patient survival. PCa may be a distinct subtype of MBC patients with SPM, warranting dedicated and targeted investigations. There may be a connection between hormone-sensitive MBC and subsequent primary PC. Abhyankar et al. (27) delineated the characteristics of MBC patients who develop spPCa using the SEER data. However, there is limited research on the prognosis of spPCa of MBC and a lack of reliable prognostic tools.
The spPCa accounts for the highest proportion among SPM in MBC (Figure 1), and it has a longer OS compared to second primary non-PCa (Figure 2, P<0.001). Multivariate analysis revealed that PCa, as opposed to non-PCa, serves as a protective factor for MBC [HR (95% CI) for OS: 0.508 (0.386–0.668); for CSS: 0.442 (0.242–0.810)]. This highlights the significance of considering spPCa as an important factor in predicting the prognosis of MBC patients who develop SPM.
In contrast with current nomograms, age has a strong correlation with the survival of MBC patients (18). This observation could be ascribed to the decrease in organ functionality and resilience to pressure, along with a rise in concurrent health conditions that accompany the process of getting older. Interestingly, tumor size >3 cm significantly affected OS and CSS in our multivariate analysis, but grade and AJCC did not; possibly due to the predominance of I and II, the distinctions in prognosis were not readily apparent. Meanwhile, tumor size has been listed as a prognostic factor for BC in previous reports (28). Larger tumors may indicate a longer duration of carrying the burden, and given that male breasts are inherently smaller compared to females, tumor size may have a greater impact. Additionally, marital status and race were also notably linked to prognosis. This finding aligns with the majority of previous research, potentially due to a multitude of factors, such as financial and ecological factors, along with psychosocial factors (29). Diverse marital statuses and racial backgrounds can give rise to varied lifestyles and income levels, consequently impacting the opportunities for early disease detection and proactive treatment. Variations in gene expression also exist across different ethnicities; Black men are often diagnosed with more aggressive PCa (30).
In terms of treatment options, receiving surgery or not was found to be obviously relevant to both OS and CSS, which aligns with the notion that radical surgical intervention is the preferred therapeutic approach for early-stage MBC. However, whether or not a patient received chemotherapy had little effect on their CSS. Chemotherapy has demonstrated a significant prolongation of the prognosis for BC, whereas the majority of MBC patients are hormone receptor positive, hence hormonal influences may be more significant (31,32). Our results showed that PR is an independent risk factor for CSS, which matches the above perspective. In a previous report, MBC patients who were PR− exhibited more aggressive behavior and had shorter survival compared to PR+ patients (33). Similarly, among females, PR− BC has a better prognosis, benefiting from hormone therapy sensitivity (34). HER2 positivity is typically associated with more aggressive BC and represents a poor prognosis (35). However, current studies are predominantly based on female BC patients. Thus, more research is needed to investigate the prognosis in MBC.
Nomograms, an appropriate scoring mechanism for clinical research, can quantify prognostic factors and display the outcomes in an easily understandable manner. Moreover, the merits and demerits of a predictive model can be assessed through its distinguishing capability, calibration, and clinical utility. A model with excellent differentiation might lack calibration. Hence, discrimination and calibration are indispensable in model evaluation. In this study, the C-index was computed and calibration curves and DCA were conducted for the training and verification sets. The results indicated that our models were very effective in predicting the 3-, 5-,8-, and 10-year OS and CSS for MBC with spPCa.
This study has several advantages over prior research: Firstly, the clinical and pathological information of the nomograms was sourced from the SEER database, which registers from 17 states in the United States. To the best of our knowledge, our study represents the largest dataset to date on MBC patients who have experienced spPCa. Secondly, our study exclusively included MBC survivors with only one type of SPM, thereby minimizing the disparities in baseline among the participants, which ensures that our study findings are more suitable for evaluating the impact of spPCa on MBC patients and predicting the survival of MBC patients who experienced an SPM. Thirdly, the variables used in the nomogram can be easily obtained from patients’ hospitalization records, with the majority being accessible through preoperative imaging examinations. Furthermore, PC, the most prevalent SPM in MBC patients, was selected as an important predictive factor. Our approach becomes more personalized and underscores the influence of PC on the progression of MBC patients with SPM, making it more applicable in clinical settings.
The were some limitations to our study. Firstly, the SEER database lacks some crucial variables that can influence prognosis, such as diet and lifestyle, family history of cancer, BRCA1/2 gene mutation status, cancer gene testing, radiation or chemotherapy regimens, and detailed information on endocrine therapy, targeted therapy (such as olaparib and talazoparib), or immunotherapy. The absence of these genetic backgrounds and treatment strategies might affect the accuracy of our prognostic model. However, the nomogram models we established remain a valuable tool because variables included in the nomogram are readily available and easy to generalize. Additionally, our study was retrospective and might have involved selection bias. Due to the rarity of MBC with spPCa, we were unable to collect data for external validation of the model. Instead, we performed internal validation by dividing the dataset into a 7:3 ratio for the construction and validation cohorts, which showed good predictive performance. Future studies are needed to perform external validation when possible. Larger-scale studies are needed to determine the mechanisms of spPCa in MBC patients.
Conclusions
MBC patients with spPCa demonstrate a better prognosis than those with other SPMs. Our study, for the first time, included spPCa as a crucial factor to develop and validate an OS nomogram and a CSS nomogram for MBC patients with an SPM utilizing commonly accessible clinical variables. The nomograms could predict the OS and CSS of MBC patients with spPCa. The nomogram scores enabled a distinct categorization of patients based on their risk of OS and CSS. Individuals at higher risk might require more intensive postoperative therapy and more frequent monitoring due to their potentially unfavorable prognosis. The nomograms were more personalized for MBC and should be further evaluated in future clinical studies.
Acknowledgments
The authors thank all the medical researchers and staff who participated in maintaining the SEER database.
Funding: The study was supported by
Footnote
Reporting Checklist: The authors have completed the TRIPOD reporting checklist. Available at https://gs.amegroups.com/article/view/10.21037/gs-24-287/rc
Peer Review File: Available at https://gs.amegroups.com/article/view/10.21037/gs-24-287/prf
Conflicts of Interest: All authors have completed the ICMJE uniform disclosure form (available at https://gs.amegroups.com/article/view/10.21037/gs-24-287/coif). The authors have no conflicts of interest to declare.
Ethical Statement: The authors are accountable for all aspects of the work in ensuring that questions related to the accuracy or integrity of any part of the work are appropriately investigated and resolved. The study was conducted in accordance with the Declaration of Helsinki (as revised in 2013).
Open Access Statement: This is an Open Access article distributed in accordance with the Creative Commons Attribution-NonCommercial-NoDerivs 4.0 International License (CC BY-NC-ND 4.0), which permits the non-commercial replication and distribution of the article with the strict proviso that no changes or edits are made and the original work is properly cited (including links to both the formal publication through the relevant DOI and the license). See: https://creativecommons.org/licenses/by-nc-nd/4.0/.
References
- Siegel RL, Miller KD, Fuchs HE, et al. Cancer Statistics, 2021. CA Cancer J Clin 2021;71:7-33. [Crossref] [PubMed]
- Chidambaram A, Prabhakaran R, Sivasamy S, et al. Male Breast Cancer: Current Scenario and Future Perspectives. Technol Cancer Res Treat 2024;23:15330338241261836. [Crossref] [PubMed]
- Fox S, Speirs V, Shaaban AM. Male breast cancer: an update. Virchows Arch 2022;480:85-93. [Crossref] [PubMed]
- Clarke CN, Cortina CS, Fayanju OM, et al. Breast Cancer Risk and Screening in Transgender Persons: A Call for Inclusive Care. Ann Surg Oncol 2022;29:2176-80. [Crossref] [PubMed]
- Anderson WF, Devesa SS. Breast carcinoma in men. Cancer 2005;103:432-3; author reply 433. [Crossref] [PubMed]
- Leone J, Hassett MJ, Freedman RA, et al. Mortality Risks Over 20 Years in Men With Stage I to III Hormone Receptor-Positive Breast Cancer. JAMA Oncol 2024;10:508-15. [Crossref] [PubMed]
- Johansen Taber KA, Morisy LR, Osbahr AJ 3rd, et al. Male breast cancer: risk factors, diagnosis, and management Oncol Rep 2010;24:1115-20. (Review). [Crossref] [PubMed]
- Wood ME, Vogel V, Ng A, et al. Second malignant neoplasms: assessment and strategies for risk reduction. J Clin Oncol 2012;30:3734-45. [Crossref] [PubMed]
- Litwin MS, Tan HJ. The Diagnosis and Treatment of Prostate Cancer: A Review. JAMA 2017;317:2532-42. [Crossref] [PubMed]
- Wang X, Zeng M, Ju X, et al. Correlation between second and first primary cancer: systematic review and meta-analysis of 9 million cancer patients. Br J Surg 2024;111:znad377. [Crossref] [PubMed]
- Hemminki K, Scélo G, Boffetta P, et al. Second primary malignancies in patients with male breast cancer. Br J Cancer 2005;92:1288-92. [Crossref] [PubMed]
- Brinton LA, Richesson DA, Gierach GL, et al. Prospective evaluation of risk factors for male breast cancer. J Natl Cancer Inst 2008;100:1477-81. [Crossref] [PubMed]
- Bergengren O, Pekala KR, Matsoukas K, et al. 2022 Update on Prostate Cancer Epidemiology and Risk Factors-A Systematic Review. Eur Urol 2023;84:191-206. [Crossref] [PubMed]
- Wang M, Wei T, Gong R, et al. The prognostic value of lymph node dissection in patients with parathyroid carcinoma. Gland Surg 2024;13:500-11. [Crossref] [PubMed]
- Zhang H, Tan Q, Xiang C, et al. Increased risk of multiple metastases and worse overall survival of metastatic pancreatic body and tail cancer: a retrospective cohort study. Gland Surg 2024;13:480-9. [Crossref] [PubMed]
- Bhardwaj PV, Gupta S, Elyash A, et al. Male Breast Cancer: a Review on Diagnosis, Treatment, and Survivorship. Curr Oncol Rep 2024;26:34-45. [Crossref] [PubMed]
- O'Malley CD, Prehn AW, Shema SJ, et al. Racial/ethnic differences in survival rates in a population-based series of men with breast carcinoma. Cancer 2002;94:2836-43. [Crossref] [PubMed]
- Zhang LP, Lin H, Wang AJ. Development and validation of a nomogram to predict survival for advanced male breast cancer. Andrologia 2022;54:e14479. [Crossref] [PubMed]
- Chen S, Liu Y, Yang J, et al. Development and Validation of a Nomogram for Predicting Survival in Male Patients With Breast Cancer. Front Oncol 2019;9:361. [Crossref] [PubMed]
- Huang H, Li Z, Huang Z, et al. Development and validation of nomograms to predict the survival probability and occurrence of a second primary malignancy of male breast cancer patients: a population-based analysis. Front Oncol 2023;13:1076997. [Crossref] [PubMed]
- Khan MH, Allerton R, Pettit L. Hormone Therapy for Breast Cancer in Men. Clin Breast Cancer 2015;15:245-50. [Crossref] [PubMed]
- Carruba G. Estrogen and prostate cancer: an eclipsed truth in an androgen-dominated scenario. J Cell Biochem 2007;102:899-911. [Crossref] [PubMed]
- Cheng HH, Shevach JW, Castro E, et al. BRCA1, BRCA2, and Associated Cancer Risks and Management for Male Patients: A Review. JAMA Oncol 2024;10:1272-81. [Crossref] [PubMed]
- Tong D. Selective estrogen receptor modulators contribute to prostate cancer treatment by regulating the tumor immune microenvironment. J Immunother Cancer 2022;10:e002944. [Crossref] [PubMed]
- Di Lauro L, Barba M, Pizzuti L, et al. Androgen receptor and antiandrogen therapy in male breast cancer. Cancer Lett 2015;368:20-5. [Crossref] [PubMed]
- Jenster G. The role of the androgen receptor in the development and progression of prostate cancer. Semin Oncol 1999;26:407-21.
- Abhyankar N, Hoskins KF, Abern MR, et al. Descriptive characteristics of prostate cancer in patients with a history of primary male breast cancer - a SEER analysis. BMC Cancer 2017;17:659. [Crossref] [PubMed]
- He Y, Duan S, Wang W, et al. Integrative radiomics clustering analysis to decipher breast cancer heterogeneity and prognostic indicators through multiparametric MRI. NPJ Breast Cancer 2024;10:72. [Crossref] [PubMed]
- Buja A, Lago L, Lago S, et al. Marital status and stage of cancer at diagnosis: A systematic review. Eur J Cancer Care (Engl) 2018;27: [Crossref] [PubMed]
- Dess RT, Hartman HE, Mahal BA, et al. Association of Black Race With Prostate Cancer-Specific and Other-Cause Mortality. JAMA Oncol 2019;5:975-83. [Crossref] [PubMed]
- Lin AP, Huang TW, Tam KW. Treatment of male breast cancer: meta-analysis of real-world evidence. Br J Surg 2021;108:1034-42. [Crossref] [PubMed]
- Gucalp A, Traina TA, Eisner JR, et al. Male breast cancer: a disease distinct from female breast cancer. Breast Cancer Res Treat 2019;173:37-48. [Crossref] [PubMed]
- Wei JL, Zhang JX, Fu DY. Characterization and prognosis of estrogen receptor-positive/progesterone receptor-negative male breast cancer: a population-based study. World J Surg Oncol 2018;16:236. [Crossref] [PubMed]
- Jia L, Peng J, Sun N, et al. Effect of PR status on the prognosis of advanced ER-high HER2-negative breast cancer patients receiving CDK4/6 inhibitor combined with endocrine as first-line therapy. BMC Cancer 2024;24:850. [Crossref] [PubMed]
- Weng Y, Liang W, Ji Y, et al. Key Genes and Prognostic Analysis in HER2+ Breast Cancer. Technol Cancer Res Treat 2021;20:1533033820983298. [Crossref] [PubMed]
(English Language Editor: J. Jones)