Early death prediction model for breast cancer with synchronous lung metastases: an analysis of the SEER database
Highlight box
Key findings
• We used Surveillance, Epidemiology, and End Results data to develop a predictive model for predicting premature mortality in breast cancer with lung metastases (BCLM) patients.
What is known and what is new?
• With a 5-year survival rate of only 8.5%, patients who develop distant metastases will progress to acquire lung metastases.
• The findings suggest that brain and liver metastases independently predict early death from cancer and other causes in patients with BCLM.
What is the implication, and what should change now?
• We created a bar-line chart with additional prognostic variables that can be readily accessed from medical records and are significant for premature death in BCLM patients.
Introduction
With an incidence of 11.7% in 2020, breast cancer is the most common cancer among women (1-3). Despite improvements in medical care, cells from breast cancer can metastasize through the circulatory and lymphatic systems (4). Fortunately, new therapeutic approaches have allowed breast cancer survival rates to rise to above 90% in recent years (5). Nevertheless, distal non-lymph-node metastases claim the lives of approximately one-third of the patients (6). With a 5-year survival rate of only 8.5%, patients in whom these distant metastases are encountered typically progress to the development of lung metastases (7).
For cancer patients, the term “early death” has no accepted definition. Early death in research and clinical trials is typically characterized as a patient passing away within a short period after diagnosis or treatment (8). We defined premature mortality in individuals with this condition as death occurring within a 3-month period of the initial diagnosis, based on previous research classifications (9). Early death in individuals with breast cancer with lung metastasis (BCLM) is a continuing issue, partly due to unsatisfactory therapeutic options. Checkpoint inhibitor-based immunotherapies, for instance, have demonstrated encouraging clinical advancements in the management of metastatic breast cancer (10). In contrast to individuals with other localized metastases, patients with BCLM have a poorer response to programmed death receptor-1/programmed death protein ligand-1 (PD-1/PD-L1) immunotherapy checkpoint drugs (11). Patients with BCLM, however, usually have worse nutritional and physical conditions than those with nonmetastatic breast cancer. This increases the risk of treatment-related complications, such as chest pain, malignant pleural effusion, pneumonia, dyspnea, and malnourishment (12). This might result in premature mortality, emphasizing the necessity to evaluate clinicopathological characteristics, therapeutic efficacy, safety, and patient health when establishing treatment strategies for BCLM patients (13).
Identifying the multifaceted elements contributing to reduced survival in BCLM patients is essential for developing more effective preventative and therapeutic approaches. Patients with BCLM who pass away too soon clearly have an inferior prognosis. The importance of a thorough examination to find the essential underlying correlations is highlighted by the need for conducting extensive study addressing the risk variables linked with this result.
This study was designed to fill this research void by thoroughly examining the clinicopathological features of BCLM patients. The research relied on data from the Surveillance, Epidemiology, and End Results (SEER) database (14). This rigorous investigation discovered factors that correlate strongly with premature mortality in BCLM patients. These associated factors need to be recognized, and their incidence needs to be forecasted with high precision in the continuous effort to enhance patient outcomes. For this reason, we created and verified a prediction model, especially for predicting early mortality in BCLM patients (15). The goal of this procedure is to increase the accuracy of early death prediction, yielding insightful information that could favor BCLM patient management and treatment plans. We present this article in accordance with the TRIPOD reporting checklist (available at https://gs.amegroups.com/article/view/10.21037/gs-24-240/rc).
Methods
Information source
We performed a retrospective analysis using the SEER database, which contains survival, clinicopathological, and demographic data on cancer patients. The study was conducted in accordance with the Declaration of Helsinki (as revised in 2013). We used data from 17 registries (2000–2019) from the SEER Research Plus, published in November 2022, for our investigation. The SEER*Stat program (version 8.4.2) was utilized to retrieve patient data related to breast cancer from the SEER database. Informed consent was not required for this investigation because the SEER database is accessible to the public, and published data do not require patient consent.
Patient selection
Patients with primary breast cancer (C50.0–C50.9) codes and histopathological confirmation who were diagnosed with breast cancer between 2010 and 2015 were included in this study. These patients were classified using the International Classification of Diseases for Oncology, Third Edition (ICD-O-3). A few patients were not included in this study because of specific requirements. The criteria for excluding patients included the following: (I) identified by death certificate or autopsy; (II) not based on a histological diagnosis; (III) involved several primary malignancies; (IV) had Tis, T0, or an unknown stage of disease; (V) had unknown distant metastases; (VI) had incomplete follow-up; (VII) had anonymous variable information; (VIII) involved male breast cancer. We examined 4,238 patients with BCLM in our investigation. Patients were randomly categorized into two groups, the training group (n=2,966, 70%) and the validation group (n=1,272, 30%), to guarantee the accuracy of the study outcomes. Please refer to Figure 1 for a picture of our patient selection procedure. Several factors were extracted from the SEER database for this study. Age at diagnosis, marital status, race, histology, grade, T stage, N stage, bone metastases, brain metastases, liver metastases, molecular subtypes, tumor size, expression of the estrogen receptor (ER), progesterone receptor (PR), and human epidermal growth factor receptor 2 (HER2), cause of death, and survival time were included. Organ transfer data are gathered annually and stored in the SEER database. The study did not require patients’ informed consent, and the data were readily available to the public.
Statistical analysis
This study investigated the relationships between early mortality and the clinicopathological, survival, and demographic features of individuals with breast cancer. Baseline characteristics of the patients were gathered and classified according to the number and dimensions of the metastatic organs. After selection of a training cohort at random, a nomogram was created and validated. The baseline characteristics of the two groups were compared using the Pearson Chi-squared test. Logistic regression analysis and backward stepwise selection were employed to determine the risk factors for premature death in BCLM patients. Based on these characteristics, we developed nomograms to predict the risk of all-cause and cancer-specific early death for cancers. We employed decision curve analysis (DCA) (16) and receiver operating characteristic (ROC) curves for both the training and validation sets to assess the performance of our model. We used SPSS statistical software (version 26.0) and the R program (version 4.1.3) for analysis. A P value less than 0.05 was considered indicative of statistical significance.
Results
Patient characteristics: clinical and demographic features
Following the use of inclusion and exclusion criteria, the study included 4,238 individuals diagnosed with breast cancer between 2010 and 2015. Over half of the patients (56.0%, n=2,374) were single, while most (71.9%, n=3,049) were white. The most prevalent (73.2%) cancer was invasive ductal carcinoma, followed by lobular carcinoma (3.1%). Grade III cancers made up the most substantial percentage (41.7%). Thirty-one percent of patients had liver metastases, and fifty-six percent of patients had bone metastases. While fewer patients had radiation therapy (26.1%) or surgery (29.6%), of the patients, chemotherapy was administered to more than half (54.1%). There were no discernible differences in demographic or clinicopathological characteristics between the training and validation groups. Table 1 displays the clinicopathological and demographic features of patients with BCLM.
Table 1
Characteristics | Overall (N=4,238) | No early death (N=3,232) | All-cause early death (N=1,006) | Cancer-specific early death (N=891) |
---|---|---|---|---|
Age, years | ||||
<50 | 736 (17.4) | 642 (19.9) | 94 (9.3) | 79 (8.9) |
50–64 | 1,671 (39.4) | 1,330 (41.2) | 341 (33.9) | 310 (34.8) |
65–74 | 953 (22.5) | 717 (22.2) | 236 (23.5) | 214 (24.0) |
≥75 | 878 (20.7) | 543 (16.8) | 335 (33.3) | 288 (32.3) |
Marital status | ||||
Unmarried | 2,374 (56.0) | 1,734 (53.7) | 640 (63.6) | 565 (63.4) |
Married | 1,622 (38.3) | 1,321 (40.9) | 301 (29.9) | 274 (30.8) |
Unknown | 242 (5.7) | 177 (5.5) | 65 (6.5) | 52 (5.8) |
Race | ||||
White | 3,049 (71.9) | 2,329 (72.1) | 720 (71.6) | 651 (73.1) |
Black | 784 (18.5) | 588 (18.2) | 196 (19.5) | 168 (18.9) |
Other | 401 (9.5) | 312 (9.7) | 89 (8.8) | 72 (8.1) |
Unknown | 4 (0.1) | 3 (0.1) | 1 (0.1) | 0 (0.0) |
Histology | ||||
Other | 1,004 (23.7) | 674 (20.9) | 330 (32.8) | 283 (31.8) |
Ductal | 3,101 (73.2) | 2,454 (75.9) | 647 (64.3) | 581 (65.2) |
Lobular | 133 (3.1) | 104 (3.2) | 29 (2.9) | 27 (3.0) |
Grade | ||||
I | 175 (4.1) | 150 (4.6) | 25 (2.5) | 21 (2.4) |
II | 1,189 (28.1) | 956 (29.6) | 233 (23.2) | 206 (23.1) |
III | 1,766 (41.7) | 1,374 (42.5) | 392 (39.0) | 357 (40.1) |
IV | 19 (0.4) | 13 (0.4) | 6 (0.6) | 5 (0.6) |
Unknown | 1,089 (25.7) | 739 (22.9) | 350 (34.8) | 302 (33.9) |
AJCC-T | ||||
T1 | 285 (6.7) | 224 (6.9) | 61 (6.1) | 51 (5.7) |
T2 | 944 (22.3) | 766 (23.7) | 178 (17.7) | 155 (17.4) |
T3 | 630 (14.9) | 498 (15.4) | 132 (13.1) | 116 (13.0) |
T4 | 1,746 (41.2) | 1,339 (41.4) | 407 (40.5) | 367 (41.2) |
TX | 633 (14.9) | 405 (12.5) | 228 (22.7) | 202 (22.7) |
AJCC-N | ||||
N0 | 799 (18.9) | 551 (17.0) | 248 (24.7) | 214 (24.0) |
N1 | 1,941 (45.8) | 1,537 (47.6) | 404 (40.2) | 367 (41.2) |
N2 | 451 (10.6) | 380 (11.8) | 71 (7.1) | 64 (7.2) |
N3 | 566 (13.4) | 469 (14.5) | 97 (9.6) | 87 (9.8) |
NX | 481 (11.3) | 295 (9.1) | 186 (18.5) | 159 (17.8) |
Surgery | ||||
No | 2,980 (70.3) | 2,048 (63.4) | 932 (92.6) | 822 (92.3) |
Yes | 1,254 (29.6) | 1,180 (36.5) | 74 (7.4) | 69 (7.7) |
Unknown | 4 (0.1) | 4 (0.1) | 0 (0.0) | 0 (0.0) |
Radiation | ||||
No/unknown | 3,132 (73.9) | 2,328 (72.0) | 804 (79.9) | 704 (79.0) |
Yes | 1,106 (26.1) | 904 (28.0) | 202 (20.1) | 187 (21.0) |
Chemotherapy | ||||
No/unknown | 1,945 (45.9) | 1,178 (36.4) | 767 (76.2) | 679 (76.2) |
Yes | 2,293 (54.1) | 2,054 (63.6) | 239 (23.8) | 212 (23.8) |
Bone metastases | ||||
Yes | 2,376 (56.0) | 1,779 (55.0) | 597 (59.3) | 546 (61.3) |
No | 1,771 (41.8) | 1,387 (42.9) | 384 (38.2) | 327 (36.7) |
Unknown | 91 (2.2) | 66 (2.0) | 25 (2.5) | 18 (2.0) |
Brain metastases | ||||
No | 3,619 (85.4) | 2,851 (88.2) | 768 (76.3) | 673 (75.5) |
Yes | 448 (10.6) | 261 (8.1) | 187 (18.6) | 173 (19.4) |
Unknown | 171 (4.0) | 120 (3.7) | 51 (5.1) | 45 (5.1) |
Liver metastases | ||||
Yes | 1,299 (30.7) | 852 (26.4) | 447 (44.4) | 409 (45.9) |
No | 2,824 (66.6) | 2,300 (71.2) | 524 (52.1) | 453 (50.8) |
Unknown | 115 (2.7) | 80 (2.5) | 35 (3.5) | 29 (3.3) |
Molecular subtype | ||||
HR−/HER2− | 688 (16.2) | 501 (15.5) | 187 (18.6) | 175 (19.6) |
HR+/HER2+ | 638 (15.1) | 531 (16.4) | 107 (10.6) | 96 (10.8) |
HR−/HER2+ | 397 (9.4) | 303 (9.4) | 94 (9.3) | 84 (9.4) |
HR+/HER2− | 1,942 (45.8) | 1,574 (48.7) | 368 (36.6) | 320 (35.9) |
Unknown | 573 (13.5) | 323 (10.0) | 250 (24.9) | 216 (24.2) |
Tumor size, mm | ||||
≥50 | 2,609 (61.6) | 1,935 (59.9) | 674 (67.0) | 601 (67.5) |
<50 | 1,629 (38.4) | 1,297 (40.1) | 332 (33.0) | 290 (32.5) |
ER | ||||
Negative | 1,219 (28.8) | 894 (27.7) | 325 (32.3) | 301 (33.8) |
Positive | 2,693 (63.5) | 2,169 (67.1) | 524 (52.1) | 460 (51.6) |
Borderline/unknown | 326 (7.7) | 169 (5.2) | 157 (15.6) | 130 (14.6) |
PR | ||||
Negative | 1,713 (40.4) | 1,274 (39.4) | 439 (43.6) | 402 (45.1) |
Positive | 2,159 (50.9) | 1,763 (54.5) | 396 (39.4) | 346 (38.8) |
Borderline/unknown | 366 (8.7) | 195 (6.0) | 171 (17.0) | 143 (16.0) |
HER2 | ||||
Negative | 2,657 (62.7) | 2,086 (64.5) | 571 (56.8) | 509 (57.1) |
Positive | 1,047 (24.7) | 843 (26.1) | 204 (20.3) | 183 (20.5) |
Borderline/unknown | 534 (12.6) | 303 (9.4) | 231 (23.0) | 199 (22.3) |
Data are presented as n (%). BCLM, breast cancer with lung metastases; AJCC, American Joint Committee on Cancer; ER, estrogen receptor; PR, progesterone receptor; HR, hormone receptor; HER2, human epidermal growth factor receptor 2.
Early mortality rates among the study population
Out of a cohort of 4,238 individuals with BCLM, 1,006 (23.7%) died from all causes, including 891 from causes connected to the cancer, and 115 from causes unrelated to the cancer. Aside from the primary location, the most frequently affected organ in all patients who passed away too soon was the bone (59.3%), followed by the liver (44.4%) and brain (18.6%). Individuals older than 50 years exhibited a far greater early mortality rate than individuals younger than 50 years.
Identification of prognostic factors associated with premature death
Following randomization, the training group consisted of 2,966 patients, whereas the validation group consisted of 1,272 individuals. White patients composed the majority of patients in both groups (71.7% and 72.4%). The most common histological type was invasive ductal carcinoma (73.4% and 72.6%, respectively), with stage T4 (41.2% and 41.3%, respectively) and stage N1 (44.9% and 47.9%, respectively) being the most common. Both groups received similar treatment modalities, with radiotherapy (26.2% and 25.9%) and surgery (29.4% and 30.0%) being the minor common sites of metastasis in both groups (56.8% and 54.4%). Except for stage N, there was no discernible difference between the two groups in residual variable distributions (P>0.05). Table 2 displays more specific baseline data for these two cohorts.
Table 2
Characteristics | Training cohort (N=2,966) | Validation cohort (N=1,272) | χ2 | P |
---|---|---|---|---|
Age, years | 1.201 | 0.75 | ||
<50 | 523 (17.6) | 213 (16.7) | ||
50–64 | 1,176 (39.6) | 495 (38.9) | ||
65–74 | 663 (22.4) | 290 (22.8) | ||
≥75 | 604 (20.4) | 274 (21.5) | ||
Marital status | 0.558 | 0.75 | ||
Unmarried | 1,654 (55.8) | 720 (56.6) | ||
Married | 1,138 (38.4) | 484 (38.1) | ||
Unknown | 174 (5.9) | 68 (5.3) | ||
Race | – | 0.96 | ||
Other | 283 (9.5) | 118 (9.3) | ||
White | 2,128 (71.7) | 921 (72.4) | ||
Black | 552 (18.6) | 232 (18.2) | ||
Unknown | 3 (0.1) | 1 (0.1) | ||
Histology | 0.666 | 0.71 | ||
Other | 693 (23.4) | 311 (24.4) | ||
Ductal | 2,178 (73.4) | 923 (72.6) | ||
Lobular | 95 (3.2) | 38 (3.0) | ||
Grade | 0.955 | 0.91 | ||
I | 127 (4.3) | 48 (3.8) | ||
II | 827 (27.9) | 362 (28.5) | ||
III | 1,231 (41.5) | 535 (42.1) | ||
IV | 14 (0.5) | 5 (0.4) | ||
Unknown | 767 (25.9) | 322 (25.3) | ||
AJCC-T | 0.285 | 0.99 | ||
T1 | 200 (6.7) | 85 (6.7) | ||
T2 | 659 (22.2) | 285 (22.4) | ||
T3 | 446 (15.0) | 184 (14.5) | ||
T4 | 1,221 (41.2) | 525 (41.3) | ||
TX | 440 (14.8) | 193 (15.2) | ||
AJCC-N | 11.229 | 0.02 | ||
N0 | 586 (19.8) | 213 (16.7) | ||
N1 | 1,332 (44.9) | 609 (47.9) | ||
N2 | 334 (11.3) | 117 (9.2) | ||
N3 | 388 (13.1) | 178 (14.0) | ||
NX | 326 (11.0) | 155 (12.2) | ||
Surgery | – | 0.87 | ||
No | 2,091 (70.5) | 889 (69.9) | ||
Yes | 872 (29.4) | 382 (30.0) | ||
Unknown | 3 (0.1) | 1 (0.1) | ||
Radiation | 0.051 | 0.82 | ||
No/unknown | 2,189 (73.8) | 943 (74.1) | ||
Yes | 777 (26.2) | 329 (25.9) | ||
Chemotherapy | 0.473 | 0.49 | ||
No/unknown | 1,351 (45.5) | 594 (46.7) | ||
Yes | 1,615 (54.5) | 678 (53.3) | ||
Bone metastases | 2.340 | 0.31 | ||
Yes | 1,684 (56.8) | 692 (54.4) | ||
No | 1,217 (41.0) | 554 (43.6) | ||
Unknown | 65 (2.2) | 26 (2.0) | ||
Brain metastases | 0.793 | 0.67 | ||
No | 2,542 (85.7) | 1,077 (84.7) | ||
Yes | 306 (10.3) | 142 (11.2) | ||
Unknown | 118 (4.0) | 53 (4.2) | ||
Liver metastases | 1.019 | 0.60 | ||
Yes | 923 (31.1) | 376 (29.6) | ||
No | 1,963 (66.2) | 861 (67.7) | ||
Unknown | 80 (2.7) | 35 (2.8) | ||
Molecular subtype | 4.179 | 0.38 | ||
HR−/HER2− | 477 (16.1) | 211 (16.6) | ||
HR+/HER2+ | 465 (15.7) | 173 (13.6) | ||
HR−/HER2+ | 280 (9.4) | 117 (9.2) | ||
HR+/HER2− | 1,356 (45.7) | 586 (46.1) | ||
Unknown | 388 (13.1) | 185 (14.5) | ||
Tumor size, mm | 0.004 | 0.94 | ||
≥50 | 1,825 (61.5) | 784 (61.6) | ||
<50 | 1,141 (38.5) | 488 (38.4) | ||
ER | 1.348 | 0.51 | ||
Negative | 854 (28.8) | 365 (28.7) | ||
Positive | 1,893 (63.8) | 800 (62.9) | ||
Borderline/unknown | 219 (7.4) | 107 (8.4) | ||
PR | 1.494 | 0.47 | ||
Negative | 1,201 (40.5) | 512 (40.3) | ||
Positive | 1,519 (51.2) | 640 (50.3) | ||
Borderline/unknown | 246 (8.3) | 120 (9.4) | ||
HER2 | 4.285 | 0.11 | ||
Negative | 1,852 (62.4) | 805 (63.3) | ||
Positive | 755 (25.5) | 292 (23.0) | ||
Borderline/unknown | 359 (12.1) | 175 (13.8) |
Data are presented as n (%). AJCC, American Joint Committee on Cancer; HR, hormone receptor; ER, estrogen receptor; PR, progesterone receptor; HER2, human epidermal growth factor receptor 2.
Age, marriage status, surgery, histology, chemotherapy, radiation treatment, brain metastases, liver metastases, size of the tumor, and molecular subtypes were shown to be linked with both cancer-specific and all-cause early death in the training cohort according to univariate logistic regression analysis (Table 3). To consider every significant risk factor found in the univariate analysis, we employed multifactor and inverted logic analysis. Cancer-specific and early all-cause death in individuals with breast cancer could be independently predicted by factors such as age, surgery, chemotherapy, radiation, brain metastases, liver metastases, and molecular subtypes. Furthermore, intravenous injection frequently resulted in the death of individuals aged >50 years with hormone receptors (HR)/HER2 subtypes of breast cancer, liver metastases, or brain metastases. Table 4 provides more specific data.
Table 3
Characteristics | All-cause early death | Cancer-specific early death | |||||
---|---|---|---|---|---|---|---|
OR | 95% CI | P | OR | 95% CI | P | ||
Age, years | |||||||
<50 | 1 (ref.) | 1 (ref.) | |||||
50–64 | 0.69 | 0.52–0.92 | 0.011 | 0.64 | 0.47–0.87 | 0.005 | |
65–74 | 0.51 | 0.38–0.69 | <0.001 | 0.47 | 0.34–0.65 | <0.001 | |
≥75 | 0.28 | 0.21–0.38 | <0.001 | 0.28 | 0.21–0.39 | <0.001 | |
Marital status | |||||||
Unmarried | 1 (ref.) | 1 (ref.) | |||||
Married | 0.60 | 0.50–0.72 | <0.001 | 0.63 | 0.52–0.77 | <0.001 | |
Unknown | 1.00 | 0.70–1.42 | 0.990 | 0.89 | 0.61–1.30 | 0.55 | |
Race | |||||||
White | 1 (ref.) | 1 (ref.) | |||||
Black | 1.03 | 0.83–1.28 | 0.763 | 0.98 | 0.78–1.23 | 0.85 | |
Other | 0.90 | 0.67–1.21 | 0.473 | 0.77 | 0.56–1.07 | 0.11 | |
Unknown | 1.57 | 0.14–17.31 | 0.714 | 1.00 | 0.70–1.42 | 0.96 | |
Histology | |||||||
Other | 1 (ref.) | 1 (ref.) | |||||
Ductal | 0.56 | 0.46–0.67 | <0.001 | 0.62 | 0.51–0.76 | <0.001 | |
Lobular | 0.73 | 0.45–1.19 | 0.208 | 0.86 | 0.52–1.41 | 0.54 | |
Grade | |||||||
I | 1 (ref.) | 1 (ref.) | |||||
II | 0.90 | 0.73–1.12 | 0.348 | 0.89 | 0.71–1.12 | 0.33 | |
III | 0.46 | 0.26–0.80 | 0.006 | 0.46 | 0.25–0.82 | 0.009 | |
IV | 1.90 | 0.63–5.70 | 0.255 | 1.60 | 0.50–5.15 | 0.42 | |
Unknown | 1.59 | 1.30–1.95 | <0.001 | 1.47 | 1.19–1.82 | <0.001 | |
AJCC-T | |||||||
T1 | 1 (ref.) | 1 (ref.) | |||||
T2 | 0.82 | 0.65–1.04 | 0.100 | 0.80 | 0.62–1.02 | 0.07 | |
T3 | 1.03 | 0.80–1.33 | 0.801 | 0.99 | 0.75–1.29 | 0.92 | |
T4 | 0.91 | 0.63–1.30 | 0.601 | 0.86 | 0.58–1.26 | 0.43 | |
TX | 1.77 | 1.39–2.24 | <0.001 | 1.69 | 1.32–2.16 | <0.001 | |
AJCC-N | |||||||
N0 | 1 (ref.) | 1 (ref.) | |||||
N1 | 1.62 | 1.30–2.02 | <0.001 | 1.52 | 1.21–1.91 | <0.001 | |
N2 | 0.71 | 0.52–0.98 | 0.038 | 0.70 | 0.50–0.98 | 0.03 | |
N3 | 0.77 | 0.57–1.03 | 0.080 | 0.74 | 0.54–1.00 | 0.052 | |
NX | 2.23 | 1.72–2.88 | <0.001 | 1.88 | 1.43–2.46 | <0.001 | |
Surgery | |||||||
No | 1 (ref.) | 1 (ref.) | |||||
Yes | 0.12 | 0.09–0.17 | <0.001 | 0.14 | 0.10–0.19 | <0.001 | |
Unknown | 1.00 | 0.70–1.42 | 0.967 | 1.00 | 0.70–1.43 | 0.97 | |
Radiation | |||||||
No/unknown | 1 (ref.) | 1 (ref.) | |||||
Yes | 0.64 | 0.52–0.78 | <0.001 | 0.70 | 0.56–0.86 | <0.001 | |
Chemotherapy | |||||||
No/unknown | 1 (ref.) | 1 (ref.) | |||||
Yes | 0.17 | 0.13–0.20 | <0.001 | 0.18 | 0.15–0.22 | <0.001 | |
Bone metastases | |||||||
Yes | 1 (ref.) | 1 (ref.) | |||||
No | 0.78 | 0.65–0.93 | 0.005 | 0.71 | 0.59- 0.86 | <0.001 | |
Unknown | 1.27 | 0.74–2.18 | 0.380 | 0.83 | 0.45–1.53 | 0.54 | |
Brain metastases | |||||||
No | 1 (ref.) | 1 (ref.) | |||||
Yes | 2.85 | 2.23–3.64 | <0.001 | 2.89 | 2.25–3.71 | <0.001 | |
Unknown | 1.60 | 1.07–2.40 | 0.021 | 1.51 | 0.98–2.31 | 0.059 | |
Liver metastases | |||||||
Yes | 1 (ref.) | 1 (ref.) | |||||
No | 0.44 | 0.37–0.52 | <0.001 | 0.42 | 0.35–0.51 | <0.001 | |
Unknown | 0.91 | 0.56–1.48 | 0.695 | 0.74 | 0.44–1.25 | 0.25 | |
Molecular subtype | |||||||
HR−/HER2− | 1 (ref.) | 1 (ref.) | |||||
HR+/HER2+ | 0.57 | 0.41–0.77 | <0.001 | 0.54 | 0.39–0.74 | <0.001 | |
HR−/HER2+ | 0.80 | 0.57–1.12 | 0.198 | 0.70 | 0.49–1.01 | 0.055 | |
HR+/HER2− | 0.65 | 0.51–0.83 | <0.001 | 0.58 | 0.45–0.74 | <0.001 | |
Unknown | 2.06 | 1.55–2.74 | <0.001 | 1.66 | 1.24–2.22 | <0.001 | |
Tumor size, mm | |||||||
≥50 | 1 (ref.) | 1 (ref.) | |||||
<50 | 0.75 | 0.63–0.90 | 0.002 | 0.77 | 0.64–0.93 | 0.006 | |
ER | |||||||
Negative | 1 (ref.) | 1 (ref.) | |||||
Positive | 0.73 | 0.60–0.88 | <0.001 | 0.68 | 0.56–0.83 | <0.001 | |
Borderline/unknown | 2.54 | 1.87–3.45 | <0.001 | 1.91 | 1.39–2.61 | <0.001 | |
PR | |||||||
Negative | 1 (ref.) | 1 (ref.) | |||||
Positive | 0.65 | 0.54–0.78 | <0.001 | 0.62 | 0.51–0.74 | <0.001 | |
Borderline/unknown | 2.33 | 1.76–3.09 | <0.001 | 1.81 | 1.35–2.43 | <0.001 | |
HER2 | |||||||
Negative | 1 (ref.) | 1 (ref.) | |||||
Positive | 0.87 | 0.71–1.08 | 0.201 | 0.87 | 0.70–1.09 | 0.22 | |
Borderline/unknown | 2.70 | 2.14–3.42 | <0.001 | 2.36 | 1.85–3.01 | <0.001 |
OR, odds ratio; CI, confidence interval; ref., reference; AJCC, American Joint Committee on Cancer; ER, estrogen receptor; PR, progesterone receptor; HR, hormone receptor; HER2, human epidermal growth factor receptor 2.
Table 4
Characteristics | All-cause early death | Cancer-specific early death | |||||
---|---|---|---|---|---|---|---|
OR | 95% CI | P | OR | 95% CI | P | ||
Age, years | |||||||
<50 | 1 (ref.) | 1 (ref.) | |||||
50–64 | 0.69 | 0.52–0.93 | 0.013 | 0.65 | 0.48–0.88 | 0.006 | |
65–74 | 0.51 | 0.38–0.69 | <0.001 | 0.47 | 0.34–0.65 | <0.001 | |
≥75 | 0.30 | 0.22–0.41 | <0.001 | 0.29 | 0.21–0.40 | <0.001 | |
Marital status | |||||||
Unmarried | 1 (ref.) | 1 (ref.) | |||||
Married | 0.75 | 0.60–0.93 | 0.009 | 0.81 | 0.65–1.01 | 0.056 | |
Unknown | 0.85 | 0.57–1.29 | 0.454 | 0.73 | 0.48–1.13 | 0.16 | |
Histology | |||||||
Other | 1 (ref.) | ||||||
Ductal | 0.73 | 0.56–0.93 | 0.013 | ||||
Lobular | 0.83 | 0.47–1.47 | 0.530 | ||||
Grade | |||||||
I | 1 (ref.) | 1 (ref.) | |||||
II | 0.73 | 0.56–0.95 | 0.020 | 0.76 | 0.58–0.99 | 0.050 | |
III | 0.38 | 0.20–0.71 | 0.002 | 0.41 | 0.21–0.79 | 0.008 | |
IV | 1.75 | 0.48–7.56 | 0.451 | 1.70 | 0.39–7.45 | 0.48 | |
Unknown | 0.80 | 0.61–1.06 | 0.126 | 0.90 | 0.68–1.18 | 0.43 | |
AJCC-N | |||||||
N0 | 1 (ref.) | 1 (ref.) | |||||
N1 | 1.40 | 1.08–1.82 | 0.010 | 1.34 | 1.03–1.75 | 0.02 | |
N2 | 0.82 | 0.57–1.20 | 0.312 | 0.82 | 0.56–1.21 | 0.32 | |
N3 | 0.89 | 0.63–1.25 | 0.505 | 0.83 | 0.58–1.18 | 0.29 | |
NX | 1.18 | 0.87–1.61 | 0.293 | 1.08 | 0.79–1.48 | 0.63 | |
Surgery | |||||||
No | 1 (ref.) | 1 (ref.) | |||||
Yes | 0.28 | 0.20–0.39 | <0.001 | 0.30 | 0.21–0.43 | <0.001 | |
Unknown | 1.00 | 0.70–1.42 | 0.966 | 1.00 | 0.70–1.42 | 0.96 | |
Radiation | |||||||
No/unknown | 1 (ref.) | 1 (ref.) | |||||
Yes | 0.67 | 0.51–0.87 | 0.003 | 0.74 | 0.57–0.97 | 0.02 | |
Chemotherapy | |||||||
No/unknown | 1 (ref.) | 1 (ref.) | |||||
Yes | 0.19 | 0.15–0.24 | <0.001 | 0.20 | 0.15–0.25 | <0.001 | |
Bone metastases | |||||||
No | 0.73 | 0.58–0.92 | 0.006 | 0.66 | 0.52–0.83 | <0.001 | |
Yes | 1 (ref.) | 1 (ref.) | |||||
Unknown | 0.63 | 0.30–1.31 | 0.216 | 0.42 | 0.19–0.93 | 0.03 | |
Brain metastases | |||||||
No | 1 (ref.) | 1 (ref.) | |||||
Yes | 3.42 | 2.50–4.70 | <0.001 | 3.18 | 2.32–4.36 | <0.001 | |
Unknown | 0.85 | 0.47–1.51 | 0.570 | 0.97 | 0.54–1.75 | 0.93 | |
Liver metastases | |||||||
Yes | 1 (ref.) | 1 (ref.) | |||||
No | 0.43 | 0.34–0.53 | <0.001 | 0.43 | 0.34–0.54 | <0.001 | |
Unknown | 0.70 | 0.35–1.38 | 0.300 | 0.66 | 0.33–1.33 | 0.24 | |
Molecular subtype | |||||||
HR−/HER2− | 1 (ref.) | 1 (ref.) | |||||
HR+/HER2+ | 5.12 | 0.67–39.21 | 0.116 | 0.60 | 0.39–0.91 | 0.01 | |
HR−/HER2+ | 5.93 | 0.78–45.29 | 0.086 | 0.63 | 0.41–0.96 | 0.03 | |
HR+/HER2− | 0.60 | 0.41–0.88 | 0.008 | 0.51 | 0.34–0.75 | <0.001 | |
Unknown | 2.84 | 0.81–9.97 | 0.104 | 1.09 | 0.67–1.79 | 0.72 | |
Tumor size, mm | |||||||
≥50 | 1 (ref.) | 1 (ref.) | |||||
<50 | 0.78 | 0.63–0.96 | 0.019 | 0.79 | 0.63–0.98 | 0.03 | |
PR | |||||||
Negative | 1 (ref.) | 1 (ref.) | |||||
Positive | 0.61 | 0.45–0.81 | <0.001 | 0.59 | 0.44–0.80 | <0.001 | |
Borderline/unknown | 0.68 | 0.41–1.13 | 0.134 | 0.59 | 0.35–0.97 | 0.03 | |
HER2 | |||||||
Negative | 1 (ref.) | ||||||
Positive | 0.13 | 0.02–0.96 | 0.045 | ||||
Borderline/unknown | 0.42 | 0.13–1.36 | 0.148 |
OR, odds ratio; CI, confidence interval; ref., reference; AJCC, American Joint Committee on Cancer; PR, progesterone receptor; HR, hormone receptor; HER2, human epidermal growth factor receptor 2.
Construction of nomograms
To predict the probability of early death from cancer or other causes in patients with breast cancer, we created two nomograms based on independent risk factors identified via multivariate logistic regression analysis. A score was given for each risk factor, and the sum of the scores for all the prognostic factors was used to determine the final score. These scores were used to construct nomograms that supported clinical decision-making and prognostic assessment (Figure 2). A vertical line was drawn, with coefficients ranging from 0.1 to 0.9, below the probability line to indicate the likelihood of dying young. Note that not all of the points have corresponding values. The graph analysis’s findings indicated that the most significant predictive factor for an early mortality was surgery.
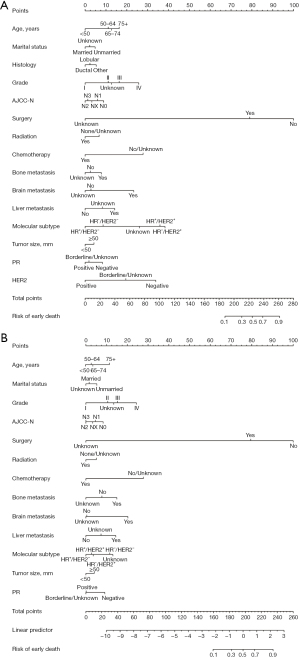
Assessment of nomograms
Cancer-specific death and early all-cause death were both well predicted by the nomogram ROC curve. The training cohort’s areas under the curve (AUCs) were 0.832 and 0.823, as shown in Figure 3A,3B. Figure 3C,3D shows that the validation cohort’s cancer-specific mortality and early all-cause death AUCs were, respectively, 0.815 and 0.810. Figure 4 shows an excellent match between the projected probabilities and the actual observed values in the training and validation sets, as indicated by the calibration curves of both modal plots being near the diagonal. Compared to a single variable, DCA maps showed that the nomograms were more effective at predicting premature death from all causes and cancer-specific causes, with a greater net benefit in both the training and validation groups. Furthermore, our findings indicated the therapeutic relevance of these graphs (Figure 5). These maps therefore have the potential to be very useful in predicting the early mortality of breast cancer patients.
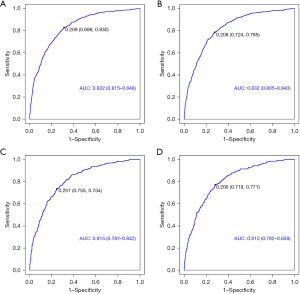
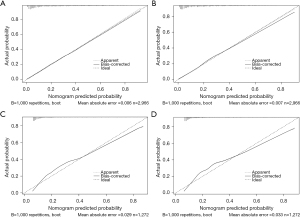
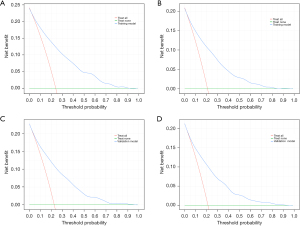
Discussion
Despite advances in breast cancer treatment, including targeted drugs, superior surgical procedures, and hormone therapy, the rate of metastasis is rapidly increasing (10). Patients with breast cancer are at a higher risk of death if they develop lung metastasis, which is the second most prevalent type of metastasis after liver and bone metastasis (17). To identify people at higher risk of dying early, it is essential to discover prognostic indicators linked to early death in BCLM patients. To improve the prognosis and clinical management of these patients, early death should receive increased attention. Numerous studies have examined premature mortality in breast cancer patients; however, no studies have examined early mortality in individuals who have developed lung metastases from breast cancer (17-19). In this study, we attempted to close this gap by investigating predictive markers and creating a histogram to predict premature death in patients with BCLM. This is the first study of its kind that we are aware of. The study’s conclusions will aid in the establishment of efficient treatment plans for breast cancer patients and help decrease likelihood of premature death.
Of the 4,238 participants in the research, 3,232 did not die prematurely. A total of 891 of the 1,006 early fatalities were categorized as early deaths related to cancer. Prompt tumor therapy is crucial, particularly for patients whose breast cancer has spread to the lungs. Furthermore, the substantial increase in the percentage of fatalities related to cancer in the present investigation revealed that individuals diagnosed with BCLM have a greater chance of passing away from their disease. These results emphasize the need to treat each patient uniquely, with very close monitoring. Notably, a prior study revealed that among individuals with advanced breast cancer who passed away within a period of one year, the percentage of mortality attributable to cancer was lower—it was only 80.78% (20). To fully comprehend the causes that lead to premature death in individuals with BCLM, additional research is necessary.
Following the analysis of data from patients who had metastasized lung cancer, we found several characteristics that were independently linked to early mortality from all causes as well as to early death from cancer. According to our research, patients older than 50 years who had lung and brain metastases, as well as the HR−/HER2− subtype, were more likely to pass away prematurely. On the other hand, we also discovered that early mortality was less common in patients who received radiotherapy, chemotherapy, or surgery. These results emphasize how crucial early identification and prompt treatment are for improving outcomes in patients with BCLM.
Several therapies, such as radiotherapy, chemotherapy, and surgery, lower the chance of early patient death during BCLM treatment. Patients with BCLM are treated surgically by pulmonary cuneiform resection, segmentectomy, lobectomy, radiofrequency ablation, and transcatheter arterial chemoembolization (13). It has been demonstrated that partial pneumonectomy significantly improves the prognosis for breast cancer patients with isolated lung metastases. According to Pathy et al., 139 (37.1%) of the 375 BCLM patients underwent surgery; patients without surgery had a 2-year survival rate of 21.2%, whereas those who underwent breast surgery had a 2-year survival rate of 46.3% (21). Surgery for the primary tumor improved the overall and breast cancer-specific survival rates of BCLM patients with single-site metastases, according to Gu et al.’s findings (22). However, there are currently insufficient clinical data to support partial pneumonectomy as the recommended course of treatment for BCLM, particularly in individuals with multiple lung metastases. Between 1972 and 2011, a review examined 795 patients with pulmonary metastasectomy in breast cancer (23). The study concluded that factors such as the number of metastases, original tumor stage at presentation, total resection, and pleural/chest wall involvement did not enhance overall survival. The diagnosis of lung nodules can be made surgically by excisional or imaging-guided biopsy (endoscopic or radiologic). The location and size of the mass, the institution’s experience, and the patient’s preferences all influence the selected treatment method. Thus, prior to surgery, the following considerations should be considered when evaluating patient eligibility: a lengthy disease-free period between a finding of initial breast cancer and BCLM, a low visceral tumor burden, and efficient systemic treatment for the triple-negative and HER2-enriched subtypes. Patients who do not fit these requirements should be managed carefully.
Chemotherapy is an important prognostic factor in BCLM. Patients receiving chemotherapy for borderline cancer of the lung syndrome need to be closely monitored for side effects, physical health, weight fluctuations, laboratory results, and radiological imaging (24). Clinicians should assess and account for all available data to reduce the chance of early death.
Our results showed that patients older than 50 years with BCLM had a greater chance of dying early. This could be caused by the immune system’s aging process as well as co-occurring conditions that include type 2 diabetes, heart, or kidney problems, all may increase the difficulty of treating cancer (25). Furthermore, a lack of social support and self-care among elderly cancer patients might have a detrimental impact on their capacity to handle the psychological and physical strain of cancer therapy (26). As a result, personalized treatment plans suited to each older patient’s unique demands and health status are necessary. This could entail modifying the chemotherapy dosage or schedule, accounting for less harmful options for therapy, or giving medical assistance more weight to manage symptoms, enhancing quality of life and eventually lowering the chance of mortality at a young age (27). Optimizing clinical outcomes requires recognition of the unique issues elderly BCLM patients experience and the provision of thorough, patient-centered care (28).
The prognosis of cancer patients is influenced by a wide range of factors, including age, therapy response, treatment type, stage, and individual health status (29). While weakened immune systems and other health problems may present difficulties for elderly cancer patients, it is crucial to remember that every patient is different and that their prognoses may vary as a result (30). As a result, it is vital to take each patient’s individual characteristics into account while formulating therapy regimens and determining patient prognosis. Clinicians and patients should work closely together to completely understand each patient’s requirements, objectives, and preferences, which will enable the provision of individualized care, enhance the standard of life, and lower the likelihood of premature death (31).
The probability of dying at a young age was positively correlated with the HR−/HER2− subtype. Chemotherapy is still the recommended course of treatment for triple-negative breast cancer (TNBC) because there is no clinical biomarker for endocrine or HER2-targeted treatment in this subtype of breast carcinoma (32). Patients with TNBC in addition to lung metastases, however, have a worse prognosis than do those with other subtypes (33). TNBC is also linked to an increased risk of lung metastases. This could be because the TNBC lung metastatic microenvironment includes increased levels of genes linked to angiogenesis and the extracellular matrix that could put these individuals at risk of dying young (34). It is crucial to remember that each patient’s prognosis is unique and may depend on factors such as the type and stage of their cancer as well as how well they respond to treatment.
Our research demonstrated that individuals with BCLM who have metastases to the brain, liver, or bone are more likely to die young. This result is consistent with another study that found the greatest survival rates were shown in people with bone metastases from breast cancer (34), while the poorest prognosis was seen in those with brain metastases. Compared to liver or lung metastases, bone metastases are usually thought to be less aggressive even though they can nevertheless result in discomfort, a decline in physical condition, and development of adverse events related to the bones (35). Furthermore, the blood-brain barrier’s limited permeability makes treating brain metastases from breast cancer challenging (36,37). Therefore, while choosing a course of treatment for patients with BCLM, the occurrence of lung and brain metastases should be carefully considered.
The nomogram has grown to be an essential tool for giving personalized breast cancer prognostic information (38). A nomogram helps patients and doctors make educated treatment decisions by visually representing predicted outcomes based on quantitative data (39,40). In this work, we created a bar-line graph to forecast BCLM patients’ premature deaths. The SEER database provided an extensive and high-quality sample for our investigation, and additional publicly available predictive indicators were available from medical records. Our study is distinct, even if there have been several recent publications that are comparable. For instance, Rashid et al. (13) reported that at Memorial Sloan-Kettering Cancer Center, patients had to have three lesions or fewer in one lung; otherwise, surgery was thought to provide no benefit.
On the other hand, our study concentrated on premature death in patients with BCLM and produced a more credible predictive model by removing multicollinearity between variables via stepwise regression. It has been discovered that the susceptibility of BCLM patients is influenced by the molecular subtype of the disease (33). Studies have indicated that information regarding molecular subtypes is essential for predicting the likelihood of lung metastases in patients with breast cancer. Gu et al. (22) created a nomogram to predict the survival of BCLM patients using a combination of triple-negative status and surgical removal of oligometastases. Clinicians can determine whether lung metastases are likely to occur in these patients using this nomogram, resulting in more rational treatment decisions (19).
Even though this study offers insightful information, it is crucial to recognize its limitations:
- The absence of data on disease recurrence or secondary sites in the SEER database limited our analysis of individuals with newly diagnosed BCLM.
- Selection bias may have been introduced if specific scenarios were excluded because of insufficient data.
- The primary focus of this study lies in the immediate future outcome of BCLM patients. Additionally, given that our findings have only been internally validated, it is imperative to conduct prospective studies for external validation.
- Regression was performed using backward stepwise regression in the multifactor analysis.
Based on each predictor’s statistical significance, the approach iteratively narrowed the variable table, starting with all of the model’s predictors. However, due to the possible exclusion of important variables from the final model, this method may have lost essential data and predictive ability. These limitations indicate potential biases in the research that could compromise the validity of the analysis.
Conclusions
Devastating BCLM is a disease with a substantial early death rate. Using the SEER information, we aimed to create a predictive model for premature mortality in BCLM patients. According to our research, in addition to bone metastasis, brain and liver metastases independently predict early death from cancer and all causes in BCLM patients. We created a bar-line chart with additional prognostic variables that can be readily accessed from medical records and are significant for premature death in BCLM patients. Stepwise regression is our prediction model’s foundation, helping to remove multicollinearity between variables.
Acknowledgments
We are grateful for the Surveillance, Epidemiology, and End Results database.
Funding: This research was supported by
Footnote
Reporting Checklist: The authors have completed the TRIPOD reporting checklist. Available at https://gs.amegroups.com/article/view/10.21037/gs-24-240/rc
Peer Review File: Available at https://gs.amegroups.com/article/view/10.21037/gs-24-240/prf
Conflicts of Interest: All authors have completed the ICMJE uniform disclosure form (available at https://gs.amegroups.com/article/view/10.21037/gs-24-240/coif). The authors have no conflicts of interest to declare.
Ethical Statement: The authors are accountable for all aspects of the work in ensuring that questions related to the accuracy or integrity of any part of the work are appropriately investigated and resolved. The study was conducted in accordance with the Declaration of Helsinki (as revised in 2013).
Open Access Statement: This is an Open Access article distributed in accordance with the Creative Commons Attribution-NonCommercial-NoDerivs 4.0 International License (CC BY-NC-ND 4.0), which permits the non-commercial replication and distribution of the article with the strict proviso that no changes or edits are made and the original work is properly cited (including links to both the formal publication through the relevant DOI and the license). See: https://creativecommons.org/licenses/by-nc-nd/4.0/.
References
- Giaquinto AN, Sung H, Miller KD, et al. Breast Cancer Statistics, 2022. CA Cancer J Clin 2022;72:524-41. [Crossref] [PubMed]
- Siegel RL, Giaquinto AN, Jemal A. Cancer statistics, 2024. CA Cancer J Clin 2024;74:12-49. [Crossref] [PubMed]
- Bray F, Laversanne M, Sung H, et al. Global cancer statistics 2022: GLOBOCAN estimates of incidence and mortality worldwide for 36 cancers in 185 countries. CA Cancer J Clin 2024;74:229-63. [Crossref] [PubMed]
- Dong Y, Jin Q, Sun M, et al. CLDN6 inhibits breast cancer metastasis through WIP-dependent actin cytoskeleton-mediated autophagy. J Exp Clin Cancer Res 2023;42:68. [Crossref] [PubMed]
- Caswell-Jin JL, Sun LP, Munoz D, et al. Analysis of Breast Cancer Mortality in the US-1975 to 2019. JAMA 2024;331:233-41. [Crossref] [PubMed]
- Ibragimova MK, Tsyganov MM, Kravtsova EA, et al. Organ-Specificity of Breast Cancer Metastasis. Int J Mol Sci 2023;24:15625. [Crossref] [PubMed]
- Nolan E, Kang Y, Malanchi I. Mechanisms of Organ-Specific Metastasis of Breast Cancer. Cold Spring Harb Perspect Med 2023;13:a041326. [Crossref] [PubMed]
- Vaz-Luis I, Lin NU, Keating NL, et al. Factors Associated with Early Mortality Among Patients with De Novo Metastatic Breast Cancer: A Population-Based Study. Oncologist 2017;22:386-93. [Crossref] [PubMed]
- Yang W, Fang Y, Niu Y, et al. A predictive model for early death in elderly patients with gastric cancer: A population-based study. Front Oncol 2022;12:972639. [Crossref] [PubMed]
- Wang J, Wu SG. Breast Cancer: An Overview of Current Therapeutic Strategies, Challenge, and Perspectives. Breast Cancer (Dove Med Press) 2023;15:721-30. [Crossref] [PubMed]
- Elanany MM, Mostafa D, Hamdy NM. Remodeled tumor immune microenvironment (TIME) parade via natural killer cells reprogramming in breast cancer. Life Sci 2023;330:121997. [Crossref] [PubMed]
- Wu M, Liang Y, Zhang X. Changes in Pulmonary Microenvironment Aids Lung Metastasis of Breast Cancer. Front Oncol 2022;12:860932. [Crossref] [PubMed]
- Rashid OM, Takabe K. The evolution of the role of surgery in the management of breast cancer lung metastasis. J Thorac Dis 2012;4:420-4. [Crossref] [PubMed]
- Kwon JH, Kim H, Lee JK, et al. Incidence and Characteristics of Multiple Primary Cancers: A 20-Year Retrospective Study of a Single Cancer Center in Korea. Cancers (Basel) 2024;16:2346. [Crossref] [PubMed]
- Balachandran VP, Gonen M, Smith JJ, et al. Nomograms in oncology: more than meets the eye. Lancet Oncol 2015;16:e173-80. [Crossref] [PubMed]
- Vickers AJ, Holland F. Decision curve analysis to evaluate the clinical benefit of prediction models. Spine J 2021;21:1643-8. [Crossref] [PubMed]
- Xiao W, Zheng S, Liu P, et al. Risk factors and survival outcomes in patients with breast cancer and lung metastasis: a population-based study. Cancer Med 2018;7:922-30. [Crossref] [PubMed]
- Wang J, Zhao H, Ye L, et al. Diagnostic and Prognostic Nomograms for Lung Metastasis in Triple-Negative Breast Cancer. Comput Math Methods Med 2022;2022:1750834. [Crossref] [PubMed]
- Xie Y, Lei C, Ma Y, et al. Prognostic nomograms for breast cancer with lung metastasis: a SEER-based population study. BMC Womens Health 2024;24:16. [Crossref] [PubMed]
- Zhao Y, Xu G, Guo X, et al. Early Death Incidence and Prediction in Stage IV Breast Cancer. Med Sci Monit 2020;26:e924858. [Crossref] [PubMed]
- Pathy NB, Verkooijen HM, Taib NA, et al. Impact of breast surgery on survival in women presenting with metastatic breast cancer. Br J Surg 2011;98:1566-72. [Crossref] [PubMed]
- Gu Y, Wu G, Zou X, et al. Prognostic Value of Site-Specific Metastases and Surgery in De Novo Stage IV Triple-Negative Breast Cancer: A Population-Based Analysis. Med Sci Monit 2020;26:e920432. [Crossref] [PubMed]
- Soh J, Komoike Y, Mitsudomi T. Surgical therapy for pulmonary metastasis of breast cancer. Transl Cancer Res 2020;9:5044-52. [Crossref] [PubMed]
- Kerbel RS. A Decade of Experience in Developing Preclinical Models of Advanced- or Early-Stage Spontaneous Metastasis to Study Antiangiogenic Drugs, Metronomic Chemotherapy, and the Tumor Microenvironment. Cancer J 2015;21:274-83. [Crossref] [PubMed]
- MacDonald T, Krishnan V, Wilson WA. Palliative care access in the elderly metastatic breast cancer population: obstacles and opportunities. Transl Cancer Res 2020;9:S110-5. [Crossref] [PubMed]
- Arthur EK, Nikahd M, Hyer JM, et al. Associations of Demographic and Social Factors on Health-Related Quality-of-Life Changes Among Older Women With Breast or Gynecologic Cancer. Oncol Nurs Forum 2024;51:127-41. [Crossref] [PubMed]
- Chekhun V, Martynyuk O, Lukianova Y, et al. Features of breast cancer in patients of young age: search for diagnosis optimization and personalized treatment. Exp Oncol 2023;45:139-50. [Crossref] [PubMed]
- You YH, Kim MK, Lee JY. Prognosis and Adjusting Factors in Elderly Patients With Triple-Negative Breast Cancer: Comparing With Young and Middle Age Groups. Clin Breast Cancer 2024;24:e258-65. [Crossref] [PubMed]
- Tefferi A, Vannucchi AM, Barbui T. Essential thrombocythemia: 2024 update on diagnosis, risk stratification, and management. Am J Hematol 2024;99:697-718. [Crossref] [PubMed]
- Depboylu B. Treatment and patient related quality of life issues in elderly and very elderly breast cancer patients. Transl Cancer Res 2020;9:S146-53. [Crossref] [PubMed]
- Patel SR, Zayas J, Medina-Inojosa JR, et al. Real-World Experiences With Yoga on Cancer-Related Symptoms in Women With Breast Cancer. Glob Adv Health Med 2021;10:2164956120984140. [Crossref] [PubMed]
- Hatzipanagiotou ME, Pigerl M, Gerken M, et al. Clinical impact of delaying initiation of adjuvant chemotherapy in patients with early triple negative breast cancer. Breast Cancer Res Treat 2024;204:607-15. [Crossref] [PubMed]
- Dieci MV, Tsvetkova V, Orvieto E, et al. Immune characterization of breast cancer metastases: prognostic implications. Breast Cancer Res 2018;20:62. [Crossref] [PubMed]
- Plava J, Trnkova L, Makovicky P, et al. Novel model of triple-negative breast cancer produces viable circulating tumor cells and rapid lung metastasis for functional testing in vivo. Neoplasma 2023;70:514-25. [Crossref] [PubMed]
- He M, Wang D, Li H, et al. Value of CT-based radiomics in evaluating the response of bone metastases to systemic drug therapy in breast cancer patients. Thorac Cancer 2024;15:361-8. [Crossref] [PubMed]
- Rastogi K, Bhaskar S, Gupta S, et al. Palliation of Brain Metastases: Analysis of Prognostic Factors Affecting Overall Survival. Indian J Palliat Care 2018;24:308-12. [Crossref] [PubMed]
- Xiong S, Tan X, Wu X, et al. Molecular landscape and emerging therapeutic strategies in breast cancer brain metastasis. Ther Adv Med Oncol 2023;15:17588359231165976. [Crossref] [PubMed]
- Koopaie M, Kolahdooz S, Fatahzadeh M, et al. Salivary biomarkers in breast cancer diagnosis: A systematic review and diagnostic meta-analysis. Cancer Med 2022;11:2644-61. [Crossref] [PubMed]
- Wang W, Liu J, Chen Y, et al. An effective tool for predicting survival in breast cancer patients with de novo lung metastasis: Nomograms constructed based on SEER. Front Surg 2022;9:939132. [Crossref] [PubMed]
- Wang K, Li Y, Wang D, et al. Web-Based Dynamic Nomograms for Predicting Overall Survival and Cancer-Specific Survival in Breast Cancer Patients with Lung Metastases. J Pers Med 2022;13:43. [Crossref] [PubMed]