Laboratory parameters-based logistic regression models for rapid screening of thyroid nodules
Highlight box
Key findings
• The expression of some laboratory parameters of patients with thyroid nodules (TNs) is different from that in healthy individuals.
• The new models based on laboratory parameters have diagnostic value for rapid screening of different types of TNs.
What is known and what is new?
• In previous studies, the diagnostic models of TNs have often been established by ultrasonography multi-parameter indicators.
• Prediction models of TNs using only laboratory indexes obtained high efficacy.
What is the implication, and what should change now?
• The laboratory parameters-based logistic regression models with easily obtained parameters are convenient and low cost, which is feasible for large-scale screenings to differentiate different types of TNs.
Introduction
Thyroid nodules (TNs) are a common clinical problem (1). There has been an increase of up to 60% in the diagnosis of TNs with the improvement of ultrasonographic techniques and the use of fine-needle aspiration (FNA) (2). Most of the TNs detected are benign TNs (BTNs) and only about 5% are malignant TNs (MTNs). TNs usually cause no clinical symptoms, but some people with large TNs may have symptoms such as neck pain, voice changes, and trouble in breathing. Risk factors of MTNs include being exposed to radiation, family history, and so on (3). The gold standard for diagnosing patients with TNs is FNA biopsy (FNAB) (4). Before FNA, a few nods to ultrasound would be appropriate. The increasing incidence of TNs is placing an increasing burden to radiologists to diagnose MTNs via ultrasonography (US) (2). However, the majority of TNs detected through US are reported to be benign, and only 5–15% of TNs are malignant (5).
Currently, some logistic regression models are used to establish the combined diagnosis with US multi-parameter indicators (6,7). In fact, it is widely known that serum biomarkers can be used for tumor screening and recurrence monitoring (8-10). Luo et al. established a regression diagnostic model for early screening of breast cancer based on serum tumor markers (11). Hwa et al. used serum biomarkers to develop a regression diagnostic model for prediction of the status of breast cancer and lymph node metastases (12). Herein, the objectives of our study were to select valuable laboratory parameters during the routine physical examination and compare their expression levels. Further, we developed and validated the possibility of quick screening models for different types of TNs via logistic regression based on the important parameters. We present this article in accordance with the TRIPOD reporting checklist (available at https://gs.amegroups.com/article/view/10.21037/gs-24-227/rc).
Methods
Patients
From December 2016 to July 2022, we collected 251 healthy individuals undergoing routine physical examination, as well as 478 patients with TNs undergoing thyroidectomy, including 176 patients with BTNs and 302 patients with MTNs who were recruited from Beijing Chaoyang Hospital. Patients with BTNs or MTNs were confirmed by surgical histopathology for the first time. Biopsies were interpreted by expert pathologists who were blinded to patient clinical characteristics and serum measurements. Patients with any of the following conditions were not eligible to take part in the research: (I) insufficient data or records; and (II) severe comorbidities or organ disorders. We divided the different types of patients into different groups: healthy individuals (Healthy group), patients with benign TNs (BTN group), and patients with malignant TNs (MTN group). The data of every group were split into two cohorts separately for the development data set (selecting three-quarters of all data randomly) and validation data set (the remaining quarter of the data). The study was conducted in accordance with the Declaration of Helsinki (as revised in 2013). This study was approved by the Beijing Chaoyang Hospital’s Ethical Committee (No. 2023-ke-25). Informed consent was waived because of the retrospective design of this study.
Laboratory data
Different aspects of laboratory parameters were obtained from the electronic medical records of Beijing Chaoyang Hospital, including serum thyroid function parameters, blood cell parameters, and serum biochemical parameters. For the purpose of maximizing the statistical power and generalizability of the findings, the study utilized all relevant data stored within the database. As composite parameters, we evaluated total peripheral platelets (PLTs) count × neutrophil-to-lymphocyte ratio (NLR) that was reported as a parameter of the systemic immune-inflammation index (SII), and the following formulae were used to calculate the systemic inflammation response index (SIRI): SIRI = neutrophils × monocytes/lymphocytes (13,14). The results of the laboratory examinations were taken from the patients’ last blood sample before surgery.
Statistical analysis
Statistical analyses were conducted by using SPSS software (IBM Corp., Armonk, NY, USA), MedCalc tools (MedCalc Software Ltd., Ostend, Belgium), and GraphPad Prism (GraphPad Software, San Diego, CA, USA). All continuous data were retained in their original scales for statistical analysis. The validation analysis was a test of the diagnostic efficacy of every model created by the retrospective cohort. We first conducted logistic regression analysis for the BTN group and MTN group to the Healthy group separately: BTN group and Healthy group (Group I); MTN group and Healthy group (Group II), and then further directly performed regression analysis between BTN group and MTN group (Group III).
Univariate analysis
Continuous variables including all 55 parameters of laboratory tests during routine physical examination were entered into the logistic univariate analysis including 29 serum biochemical parameters, 19 complete blood count (CBC) parameters, and 7 serum thyroid function parameters. Indexes with both significant differences (P value <0.10) in statistics and clinical aspect were filtered out.
Multivariate analysis
To create suitable models for diagnosing different types of TNs, the mutual parameters which were filtered out through univariate analysis in Group I, Group II, and all about thyroid function were divided into two groups based on association with thyroid disease (Indexes A) and other common laboratory parameters (Indexes B). Several studies have shown that disturbances in thyroid function are closely related to glucose (Glu) and lipid metabolism disorders (15), so we included these indicators of metabolism of Glu and lipids to Indexes A. The independent influencing factors screened by the multivariate analysis of Indexes A were further combined with Indexes B and reanalyzed (during multivariate analysis, the probabilities for stepwise of entry and removal in forward logistic regression were 0.01 and 0.1, respectively). The parameters filtered out by multivariate analyses of Indexes A and Indexes a + B were denoted as Indexes a and Indexes b, respectively.
Establishment and validation of logistic regression models
To construct logistic regression models in each group, Indexes a and Indexes b that were statistically significant in the multivariate analysis were included. Sensitivity, specificity, and area under the curve (AUC) were assessed for each model. The models were further verified by the validation cohort. The AUCs were compared using the DeLong’s test.
Comparison of the expression levels of valuable parameters
Continuous data of normal distribution were presented as mean ± standard deviation (SD); analysis of variance (ANOVA) was used for multi-group comparison, least significant difference (LSD)-test was used for further pairwise comparison. Continuous data of non-normal distribution were presented as median and interquartile range (IQR), Kruskal-Wallis H test was used for multi-group comparison, Bonferroni-corrected Mann-Whitney U test was used for further pairwise comparison. Gender data were presented as frequencies and proportions and analyzed by Chi-squared test.
Results
Basic information of TNs group and Healthy group
The age of the Healthy group (N=251) was 23–76 years old, with a median and IQR of 46 [38–58] years old. The female-to-male ratio of the Healthy group was 1.82:1. General characteristics of patients with TNs are shown in Table 1.
Table 1
Variables | Thyroid nodules (n=478) | BTN groups (n=176) | MTN groups (n=302) | P value |
---|---|---|---|---|
Gender, n (%) | 0.61 | |||
Male | 140 (29.3) | 54 (30.7) | 86 (28.5) | |
Female | 338 (70.7) | 122 (69.3) | 216 (71.5) | |
Age (years), M (P25, P75) | 50.00 (37.00, 59.00) | 58.00 (49.25, 64.00) | 44.50 (35.00, 55.00) | <0.001 |
BMI (kg/m2), M (P25, P75) | 24.97 (22.40, 27.34) | 24.97 (22.57, 27.60) | 24.95 (22.19, 27.34) | 0.83 |
History of diabetes, n (%) | 0.24 | |||
None | 431 (90.2) | 155 (88.1) | 276 (91.4) | |
Yes | 47 (9.8) | 21 (11.9) | 26 (8.6) | |
History of hyperlipidemia, n (%) | 0.63 | |||
None | 459 (96.0) | 168 (95.5) | 291 (96.4) | |
Yes | 19 (4.0) | 8 (4.5) | 11 (3.6) | |
Tumor type (MTN groups), n (%) | ||||
Papillary thyroid carcinoma | – | – | 295 (97.7) | – |
Medullary thyroid carcinoma | – | – | 2 (0.7) | – |
Follicular thyroid carcinoma | – | – | 5 (1.7) | – |
BTN group, patients with benign thyroid nodules; MTN group, patients with malignant thyroid nodules; M (P25, P75), median (25th percentile, 75th percentile); BMI, body mass index.
Univariate and multivariate analyses for each group of parameters
Group I comprised 132 BTNs patients and 190 healthy individuals. The laboratory parameter univariate and multivariate analysis outcomes are displayed in Table 2 and Table S1. For patients with BTNs in the Healthy group, high levels of Glu, total triiodothyronine (TT3) [odds ratio (OR) >1, P<0.001] and low levels of high density lipoprotein cholesterol (HDL-C), free triiodothyronine (FT3), serum thyroid stimulating hormone (sTSH), albumin (ALB), and mean corpuscular hemoglobin (MCH) were significantly associated with the development of BTNs (OR <1, P<0.001).
Table 2
Indexes combination | Laboratory parameters | Univariate analysis | Multivariate analysisc | Multivariate analysisd | |||||||
---|---|---|---|---|---|---|---|---|---|---|---|
OR | P value | OR | Coefficient β value | P value | OR | Coefficient β value | P value | ||||
Indexes A | HDL-C (mmol/L) | 0.114 | <0.001 | 0.08 | −2.525 | <0.001a | 0.102 | −2.343 | <0.001b | ||
TG (mmol/L) | 3.045 | <0.001 | 4.868 | – | 0.03 | – | – | – | |||
Lp(a) (nmol/L) | 1.026 | 0.001 | 3.066 | – | 0.08 | – | – | – | |||
Glu (mmol/L) | 5.861 | <0.001 | 4.55 | 1.515 | <0.001a | 6.499 | 1.85 | <0.001b | |||
Anti-TPO (U/mL) | 1.003 | 0.12 | 1.956 | – | 0.16 | – | – | – | |||
Anti-Tg (U/mL) | 1.1 | <0.001 | 1.748 | – | 0.19 | – | – | – | |||
TT3 (ng/mL) | 0.766 | 0.70 | 1.003 | 0.003 | 0.003a | 1.004 | 0.004 | 0.001b | |||
TT4 (μg/dL) | 0.973 | 0.73 | 1.551 | – | 0.21 | – | – | – | |||
FT3 (μg/dL) | 0.062 | <0.001 | 0.01 | −4.607 | <0.001a | 0.009 | −4.663 | <0.001b | |||
FT4 (ng/dL) | 1.017 | 0.98 | 1.686 | – | 0.19 | – | – | – | |||
sTSH (ulU/mL) | 0.493 | <0.001 | 0.445 | −0.81 | <0.001a | 0.43 | −0.839 | <0.001b | |||
Indexes B | ALB (g/L) | 0.802 | <0.001 | – | – | – | 0.784 | −0.231 | <0.001b | ||
SII | 1.001 | 0.01 | – | – | – | 2.178 | – | 0.14 | |||
SIRI | 3.484 | <0.001 | – | – | – | 5.303 | – | 0.02 | |||
MCH (pg) | 0.851 | 0.03 | – | – | – | 0.696 | −0.361 | 0.003b | |||
PLT (109/L) | 1.004 | 0.07 | – | – | – | 4.562 | – | 0.03 |
a, Indexes a: data filtered from Indexes A; b, Indexes b: data filtered from Indexes a and B; c, multivariate analysis of Indexes A; d, multivariate analysis of Indexes a and B. BTN group, patients with benign thyroid nodules; Healthy group, healthy individuals; OR, odds ratio; HDL-C, high density lipoprotein cholesterol; TG, triglyceride; Lp(a), lipoprotein; Glu, glucose; Anti-TPO, antithyroperoxidase antibody; Anti-Tg, antithyroglobulin antibody; TT3, total triiodothyronine; TT4, total thyroxine; FT3, free triiodothyronine; FT4, free thyroxine; sTSH, serum thyroid stimulating hormone; ALB, albumin; SII, systemic immune-inflammation index; SIRI, systemic inflammatory response index; MCH, mean corpuscular hemoglobin; PLT, platelet.
Group II comprised 228 MTNs patients and 190 healthy individuals. The laboratory parameter univariate and multivariate analysis outcomes are displayed in Table 3 and Table S1. For patients with MTNs in the Healthy group, high levels of lipoprotein [Lp(a)], Glu, antithyroglobulin antibody (Anti-Tg), white blood cell (WBC) count, and PLT (OR >1, P<0.001), and low levels of HDL-C, FT3, sTSH, ALB, and MCH were significantly associated with the development of MTNs (OR <1, P<0.001). It is worth adding that when multivariate regression analysis included SII and SIRI, the model displayed multicollinearity, so the inflammation-related index was changed to WBC.
Table 3
Indexes combination | Laboratory parameters | Univariate analysis | Multivariate analysisc | Multivariate analysisd | |||||||
---|---|---|---|---|---|---|---|---|---|---|---|
OR | P value | OR | Coefficient β value | P value | OR | Coefficient β value | P value | ||||
Indexes A | HDL-C (mmol/L) | 0.114 | <0.001 | 0.061 | −2.789 | <0.001a | 0.076 | −2.579 | <0.001b | ||
TG (mmol/L) | 2.712 | <0.001 | 4.316 | – | 0.04 | – | – | – | |||
Lp(a) (nmol/L) | 1.029 | <0.001 | 1.036 | 0.035 | <0.001a | 1.033 | 0.032 | 0.002b | |||
Glu (mmol/L) | 3.342 | <0.001 | 3.13 | 1.141 | <0.001a | 5.198 | 1.648 | <0.001b | |||
Anti-TPO (U/mL) | 1.006 | 0.06 | 1.009 | – | 0.32 | – | – | – | |||
Anti-Tg (U/mL) | 1.059 | <0.001 | 1.056 | 0.054 | 0.001a | 1.066 | 0.064 | 0.002b | |||
TT3 (ng/mL) | 0.521 | 0.25 | 3.538 | – | 0.06 | – | – | – | |||
TT4 (μg/dL) | 1.061 | 0.28 | 1.193 | – | 0.28 | – | – | – | |||
FT3 (pg/mL) | 0.174 | <0.001 | 0.145 | −1.931 | <0.001a | 0.176 | −1.737 | <0.001b | |||
FT4 (ng/dL) | 1.032 | 0.62 | 1.293 | – | 0.26 | – | – | – | |||
sTSH (ulU/mL) | 0.921 | 0.30 | 0.711 | −0.341 | 0.005a | 0.666 | −0.406 | 0.004b | |||
Indexes B | ALB (g/L) | 0.784 | <0.001 | – | – | – | 0.695 | −0.363 | <0.001b | ||
WBC (109/L) | 1.633 | <0.001 | – | – | – | 1.606 | 0.474 | <0.001b | |||
MCH (pg) | 0.759 | <0.001 | – | – | – | 0.71 | −0.343 | <0.001b | |||
PLT (109/L) | 1.006 | 0.001 | – | – | – | 1.007 | 0.007 | 0.03b |
a, Indexes a: data filtered from Indexes A; b, Indexes b: data filtered from Indexes a and B; c, multivariate analysis of Indexes A; d, multivariate analysis of Indexes a and B. MTN group, patients with malignant thyroid nodules; Healthy group, healthy individuals; OR, odds ratio; HDL-C, high-density lipoprotein cholesterol; TG, triglyceride; Lp(a), lipoprotein; Glu, glucose; Anti-TPO, antithyroperoxidase antibody; Anti-Tg, antithyroglobulin antibody; TT3, total triiodothyronine; TT4, total thyroxine; FT3, free triiodothyronine; FT4, free thyroxine; sTSH, serum thyroid stimulating hormone; ALB, albumin; WBC, white blood cell; MCH, mean corpuscular hemoglobin; PLT, platelet.
Group III comprised 132 BTNs patients and 228 MTNs patients. The outcomes of univariate and multivariate analyses of laboratory parameters are displayed in Table 4 and Table S1. For distinguishing patients with BTNs and MTNs, high level of sTSH (OR >1, P<0.001) and low level of Glu were significantly associated with the development of MTNs (OR <1, P<0.001).
Table 4
Indexes combination | Laboratory parameters | Univariate analysis | Multivariate analysisc | Multivariate analysisd | |||||||
---|---|---|---|---|---|---|---|---|---|---|---|
OR | P value | OR | Coefficient β value | P value | OR | Coefficient β value | P value | ||||
Indexes A | CA (mmol/L) | 0.17 | 0.09 | 1.34 | – | 0.25 | – | – | – | ||
Glu (mmol/L) | 0.743 | 0.004 | 0.739 | −0.303 | 0.004a | 0.739 | −0.303 | 0.004b | |||
Anti-TPO (U/mL) | 1 | 0.06 | 2.572 | – | 0.11 | – | – | – | |||
Anti-Tg (U/mL) | 1.001 | 0.05 | 2.464 | – | 0.12 | – | – | – | |||
TT3 (ng/mL) | 0.734 | 0.57 | 0.092 | – | 0.76 | – | – | – | |||
TT4 (μg/dL) | 1.069 | 0.26 | 2.468 | – | 0.12 | – | – | – | |||
FT3 (pg/mL) | 1.101 | 0.72 | 0.554 | – | 0.46 | – | – | – | |||
FT4 (ng/dL) | 1.031 | 0.66 | 0.559 | – | 0.46 | – | – | – | |||
sTSH (ulU/mL) | 1.393 | 0.003 | 1.398 | 0.335 | 0.003a | 1.398 | 0.335 | 0.003b | |||
Indexes B | GLB (g/L) | 0.95 | 0.07 | – | – | – | 2.09 | – | 0.15 | ||
MCH (pg) | 0.887 | 0.02 | – | – | – | 3.398 | – | 0.07 |
a, Indexes a: data filtered from Indexes A; b, Indexes b: data filtered from Indexes a and B; c, multivariate analysis of Indexes A; d, multivariate analysis of Indexes a and B. BTN group, patients with benign thyroid nodules; MTN group, patients with malignant thyroid nodules; OR, odds ratio; CA, serum calcium; Glu, glucose; Anti-TPO, antithyroperoxidase antibody; Anti-Tg, antithyroglobulin antibody; TT3, total triiodothyronine; TT4, total thyroxine; FT3, free triiodothyronine; FT4, free thyroxine; sTSH, serum thyroid stimulating hormone; GLB, globulin; MCH, mean corpuscular hemoglobin.
Model establishment
Indexes a and Indexes b, which were selected in each group, were defined as independent variables, and diagnosis with the surgical histopathology as dependent variables. Five regression models were established according to the partial regression coefficient β value. The sensitivity, specificity, and AUC of every model is shown in Table 5. The sensitivity, specificity, and AUC of Group I and Group II were high. Their sensitivity ranged from 78.03% to 88.60%, their specificity ranged from 83.68% to 91.05%, and their AUC ranged from 0.893 to 0.940. However, the model demonstrated an AUC of 0.636 (sensitivity =71.49%, specificity =49.24%) in Group III.
Table 5
Group | Indexes combination | P value (H-L test) | Sensitivity (%) | Specificity (%) | AUC | P valuef |
---|---|---|---|---|---|---|
Group I | Iaa | 0.43 | 78.03 | 88.95 | 0.905 | 0.10 |
Ibb | 0.63 | 81.06 | 91.05 | 0.919 | ||
Group II | IIac | 0.11 | 82.89 | 83.68 | 0.893 | <0.001 |
IIbd | 0.13 | 88.60 | 87.37 | 0.940 | ||
Group III | IIIae | 0.92 | 71.49 | 49.24 | 0.636 | >0.99 |
f, comparison of AUC between regression equations by “Indexes a” and “Indexes b” in the retrospective cohort. Indexes a: the parameters filtered out by multivariate analyses of Indexes A; Indexes b: the parameters filtered out by multivariate analyses of Indexes a and B. Group I, BTN group and Healthy group; Group II, MTN group and Healthy group; Group III, BTN group and MTN group. H-L test, Hosmer-Lemeshow test; AUC, area under the curve.
Validation study for every model established with laboratory parameters
The validation study enrolled 179 participants comprising 44 patients with BTNs, 74 patients with MTNs, and 61 healthy individuals. When the predictive models were tested in the validation cohort, the AUCs of the five models were 0.904, 0.927, 0.902, 0.871, and 0.682, respectively, which were not statistically significant differences from the retrospective study except Group IIb (PIa=0.98, PIb=0.82, PIIa=0.76, PIIb=0.03, PIIIa=0.46) (Figure 1, Table 6). There were no significant differences in AUC between the model based on Indexes a and the model based on Indexes b in every group (PI=0.14, PII=0.09, PIII>0.99) (Table 6). In order to use more streamlined combinations of laboratory parameters to achieve excellent diagnostic performance, we finally chose the equations based on Indexes a [1Logit(P), 3Logit(P) and 5Logit(P)] as the diagnostic models for each group respectively.
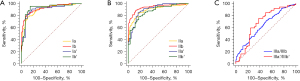
Table 6
Group | Indexes combination | Retrospective cohort | Validation cohort | P valuea | P valueb |
---|---|---|---|---|---|
Group I | Ia' | 0.905 | 0.904 | 0.14 | 0.98 |
Ib' | 0.919 | 0.927 | 0.82 | ||
Group II | IIa' | 0.893 | 0.902 | 0.09 | 0.76 |
IIb' | 0.940 | 0.871 | 0.03 | ||
Group III | IIIa' | 0.636 | 0.682 | >0.99 | 0.46 |
a, comparison of AUC between regression equation by Indexes a and Indexes b in validation cohort (P>0.05); b, comparison of AUC between each regression equation in retrospective cohort and validation cohort. Indexes a: the parameters filtered out by multivariate analyses of Indexes A; Indexes b: the parameters filtered out by multivariate analyses of Indexes a and B. Group I, BTN group and Healthy group; Group II, MTN group and Healthy group; Group III, BTN group and MTN group. AUC, area under the curve.
Comparison of the expression levels of valuable parameters between the BTN group, MTN group, and Healthy group
Figure 2A and Table S2 show that the expression levels of Anti-Tg, Glu, and WBC were all statistically higher in the BTN and MTN groups than in the Healthy group. The Lp(a) and PLT expression levels in the MTN group were higher than in the Healthy group, whereas no significant distinction was identified between the BTN group and Healthy group. In particular, the Glu expression level in the MTN group was lower compared to that in the BTN group. Figure 2B and Table S2 show that the expression levels of FT3, sTSH, HDL-C, and ALB were all statistically lower in BTN and MTN groups than in the Healthy group. The MCH expression level in the MTN group was lower than in the Healthy group, whereas no significant difference was found between the BTN group and the Healthy group. In particular, the sTSH expression level in the MTN group was higher compared to that in the BTN group.
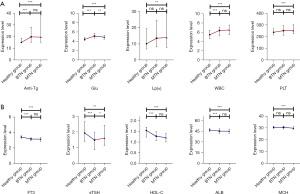
Discussion
In this study, we comprehensively analyzed all relevant laboratory parameters to identify the key parameters for diagnosing different types of TNs. Then, we classified the parameters into two categories based on the clinical characteristics. Parameters associated with thyroid disease of Anti-Tg, FT3, sTSH, HDL-C, Lp(a), and Glu, and other common laboratory parameters of ALB, WBC, PLT, and MCH were considered the important evaluation parameters.
With regard to predictive value of thyroid hormones and thyroid-related antibodies for BTNs and MTNs, Wang et al. pointed out that thyroid stimulating hormone (TSH) is an upstream factor that promotes the production and secretion of thyroid hormones in the thyroid gland. An abnormally high value of TSH indicates, on the one hand, a decrease in the ability of normal thyroid tissue to synthesize thyroid hormones resulting in lower FT3 levels; on the other hand, it suggests that there are new organisms inside the thyroid gland that need to be maintained and grown. The likelihood of MTNs increases with a higher TSH value (16). Zheng et al. found that MTNs patients had markedly higher TSH levels in comparison to BTNs patients (5), which the findings of our study aligned with. Meanwhile, we found that the TSH level in TNs patients was depressed compared to that in healthy individuals. Related research shows that the possible reason is that TNs can maintain the expression level of TSH at a lower normal range through autonomous hyperfunctioning (17). The reasons for these results are also perhaps because the patients had hyperthyroidism or were taking antithyroid medications. Anti-Tg and antithyroperoxidase antibody (Anti-TPO) are critical biomarkers that can predict increased risk of malignancy (18). Anti-Tg can activate Fc receptor-mediated elimination of thyroid follicular cells by natural killer (NK) cells. Anti-TPO causes thyroid follicular cell destruction by the alternative pathway of antibody-dependent cellular cytotoxicity (ADCC) and complement-dependent cytotoxicity (CDC) (19). Our study showed that Anti-Tg and Anti-TPO levels were statistically higher in TNs patients than in healthy individuals. Finally, with good flexibility, independent factors of Anti-Tg, FT3, and sTSH can easily be incorporated into the logistic regression models.
With respect to the predictive value of glycometabolism and lipometabolism for BTNs and MTNs, the abnormal level of blood Glu and lipid may lead to the increased prevalence of TNs (20). Risk factors associated with TNs in an epidemiological study showed that dyslipidemia and hyperglycemia had a significant relationship with the prevalence of TNs (21). This aligns with our study’s findings. Some studies have shown a strong correlation between metabolic syndrome and TNs; hyperglycemia and dyslipidemia are both important components of metabolic syndrome (22-24). Insulin resistance, a central component of metabolic syndrome, may promote the proliferation of thyroid cells, thereby contributing to the formation of TNs, and potentially leading to the development of thyroid cancer (20). However, the relationship between hypercholesterolemia and TNs remains insufficiently understood. The regression equations we have created show that, HDL-C, Lp(a), and Glu, as the auxiliary diagnosis, have great clinical reference value.
As for predictive value of inflammatory parameters for BTNs and MTNs, BTNs and MTNs may have a similar feature with most tumors in that the inflammatory cells found in tumors are inclined to advance tumor evolution, progression, and immunosuppression (25). Tumor cells secrete chemokines that draw neutrophils and other immune cells. These immune cells enter the tumor microenvironment and may potentially acquire a protumoral phenotype under certain circumstances because of their responsiveness to cues in their environment. PLTs are involved in the interplay between inflammation and cancer (26); they have the potential to promote tumor spread by preparing the metastatic microenvironment, increasing neovasculature, and impairing the defense mechanisms (27). Systemic immune-inflammation parameters have been shown to predict central lymph node metastasis in thyroid carcinoma in other studies (27,28). Thus, inflammatory parameters are also of concern in the development of TNs. Although our findings revealed that the expression level of the inflammation parameters of WBC and PLT were not statistically significant when compared patients with BTNs and MTNs, their expression levels in MTNs were significantly higher than they were in healthy individuals. Therefore, we also included WBC and PLT in the models that distinguished patients with MTNs from healthy individuals.
The relationship between ALB and MCH and TNs has been rarely addressed. In our study, we observed that TNs patients had lower levels of ALB compared to healthy individuals. The reasons may be related to the fact that patients with TNs are in a state of inflammation and have increased vascular permeability, resulting in the outward movement of ALB. At the same time, similar to other tumors, the growth of TNs consumes energy, which leads to a decrease in the synthesis of ALB by the liver. The reason for the lower levels of MCH in patients with MTNs compared to healthy individuals could be that the consumption of the body by MTNs leads to anemia, which in turn leads to a decrease in MCH. Moreover, disturbances in the levels of thyroid-related hormones may affect iron metabolism, resulting in iron-deficiency anemia which negatively affects the state of thyroid hormones. Thus, different forms of anemia may occur during thyroid dysfunction (29).
Clinically, the model displays enhanced discrimination when the AUC value falls between 0.7 and 0.9 (30). In our retrospective and validation study, the models of logistic regression equations we established had great diagnostic performance for making the differential diagnosis comparison of BTNs and MTNs with healthy individuals, their AUC in every group was more than 0.85. However, the model had relatively poor performance for making the differential diagnosis between the BTNs and MTNs (AUC =0.682 in the validation study), probably because it is not possible to accurately diagnose TNs as either benign or malignant relying solely on conventional laboratory parameters. Another reason may be that the patients included in our study all underwent thyroidectomy because of the higher risk of malignancy or larger nodules diameter. Although the results of surgical histopathology after surgery were benign, most of the laboratory parameters of these patients were not statistically significant in patients with MTNs. The developed logistic regression equation according to the important laboratory parameters can be used as the additional method of screening TNs following US. These models can further help in the establishment of individualized healthcare programs, which may guide who should be recommended to undergo thyroid US.
Nevertheless, there were limitations in the study, as it was a single-center, retrospective study with a relatively small sample size, which may have been susceptible to selection bias. These problems can be solved by multicenter and prospective studies in the future.
Conclusions
TNs are associated with the expression levels of metabolism of Glu and lipid, thyroid hormone, ALB, MCH, and PLT. Meanwhile, the diagnostic performance of the models we established for diagnosing different types of TNs is great. The laboratory parameters-based logistic regression models with easily obtained parameters are convenient and low-cost, which is feasible for large-scale screenings to differentiate different types of TNs.
Acknowledgments
Funding: This study was supported by
Footnote
Reporting Checklist: The authors have completed the TRIPOD reporting checklist. Available at https://gs.amegroups.com/article/view/10.21037/gs-24-227/rc
Data Sharing Statement: Available at https://gs.amegroups.com/article/view/10.21037/gs-24-227/dss
Peer Review File: Available at https://gs.amegroups.com/article/view/10.21037/gs-24-227/prf
Conflicts of Interest: All authors have completed the ICMJE uniform disclosure form (available at https://gs.amegroups.com/article/view/10.21037/gs-24-227/coif). The authors have no conflicts of interest to declare.
Ethical Statement: The authors are accountable for all aspects of the work in ensuring that questions related to the accuracy or integrity of any part of the work are appropriately investigated and resolved. The study was conducted in accordance with the Declaration of Helsinki (as revised in 2013). This study was approved by the Beijing Chaoyang Hospital’s Ethical Committee (No. 2023-ke-25). Informed consent was waived because of the retrospective design of this study.
Open Access Statement: This is an Open Access article distributed in accordance with the Creative Commons Attribution-NonCommercial-NoDerivs 4.0 International License (CC BY-NC-ND 4.0), which permits the non-commercial replication and distribution of the article with the strict proviso that no changes or edits are made and the original work is properly cited (including links to both the formal publication through the relevant DOI and the license). See: https://creativecommons.org/licenses/by-nc-nd/4.0/.
References
- Alexander EK, Cibas ES. Diagnosis of thyroid nodules. Lancet Diabetes Endocrinol 2022;10:533-9. [Crossref] [PubMed]
- Xia S, Yao J, Zhou W, et al. A computer-aided diagnosing system in the evaluation of thyroid nodules-experience in a specialized thyroid center. World J Surg Oncol 2019;17:210. [Crossref] [PubMed]
- Durante C, Grani G, Lamartina L, et al. The Diagnosis and Management of Thyroid Nodules: A Review. JAMA 2018;319:914-24. Erratum in: JAMA 2018;319:1622. [Crossref] [PubMed]
- Haugen BR, Alexander EK, Bible KC, et al. 2015 American Thyroid Association Management Guidelines for Adult Patients with Thyroid Nodules and Differentiated Thyroid Cancer: The American Thyroid Association Guidelines Task Force on Thyroid Nodules and Differentiated Thyroid Cancer. Thyroid 2016;26:1-133. [Crossref] [PubMed]
- Zheng J, Li C, Lu W, et al. Quantitative assessment of preoperative serum thyrotropin level and thyroid cancer. Oncotarget 2016;7:34918-29. [Crossref] [PubMed]
- Di Z, Li Z, Tian J, et al. The Value of Elasticity Contrast Index in the Differential Diagnosis of Thyroid Solid Nodules. Ultrasound Q 2019;35:259-63. [Crossref] [PubMed]
- Zheng Y, Xu S, Zheng Z, et al. Ultrasonic Classification of Multicategory Thyroid Nodules Based on Logistic Regression. Ultrasound Q 2020;36:146-57. [Crossref] [PubMed]
- Wang YF, Feng FL, Zhao XH, et al. Combined detection tumor markers for diagnosis and prognosis of gallbladder cancer. World J Gastroenterol 2014;20:4085-92. [Crossref] [PubMed]
- Xue W, Zhang X, Niu Z, et al. Neutrophil-Lymphocyte Ratio as a Predictive Factor of Growing Malignant Pulmonary Ground-glass Opacity. Cancer Manag Res 2021;13:5651-5. [Crossref] [PubMed]
- Zhao S, Mei Y, Wang Y, et al. Levels of CEA, CA153, CA199, CA724 and AFP in nipple discharge of breast cancer patients. Int J Clin Exp Med 2015;8:20837-44.
- Luo J, Xiao J, Yang Y, et al. Strategies for five tumour markers in the screening and diagnosis of female breast cancer. Front Oncol 2023;12:1055855. [Crossref] [PubMed]
- Hwa HL, Kuo WH, Chang LY, et al. Prediction of breast cancer and lymph node metastatic status with tumour markers using logistic regression models. J Eval Clin Pract 2008;14:275-80. [Crossref] [PubMed]
- Esenboğa K, Kurtul A, Yamantürk YY, et al. Systemic immune-inflammation index predicts no-reflow phenomenon after primary percutaneous coronary intervention. Acta Cardiol 2022;77:59-65. [Crossref] [PubMed]
- Yamanouchi K, Maeda S. The Efficacy of Inflammatory and Immune Markers for Predicting the Prognosis of Patients with Stage IV Breast Cancer. Acta Med Okayama 2023;77:37-43. [Crossref] [PubMed]
- Liu J, Wang C, Tang X, et al. Correlation analysis of metabolic syndrome and its components with thyroid nodules. Diabetes Metab Syndr Obes 2019;12:1617-23. [Crossref] [PubMed]
- Wang R, Liu SX, Hu ZQ, et al. Study on predictors of TI-RADS Grade 4a thyroid nodule identification. Chongqing Medical Science 2022;51:2379-82.
- Shin J, Kim MH, Yoon KH, et al. Relationship between metabolic syndrome and thyroid nodules in healthy Koreans. Korean J Intern Med 2016;31:98-105. [Crossref] [PubMed]
- Adhami M, Michail P, Rao A, et al. Anti-Thyroid Antibodies and TSH as Potential Markers of Thyroid Carcinoma and Aggressive Behavior in Patients with Indeterminate Fine-Needle Aspiration Cytology. World J Surg 2020;44:363-70. [Crossref] [PubMed]
- Wu J, Long J. Thyroid autoantibodies. Sichuan Journal of Physiological Sciences 2019;41:51-4.
- Ju XL, Pei DM. Correlation between prevalence of thyroid nodule and abnormal metabolism of blood glucose as well as lipid. Journal of Clinical Medicine in Practice 2020;24:53-6.
- Zheng L, Yan W, Kong Y, et al. An epidemiological study of risk factors of thyroid nodule and goiter in Chinese women. Int J Clin Exp Med 2015;8:11379-87.
- Hu L, Li T, Yin XL, et al. An analysis of the correlation between thyroid nodules and metabolic syndrome. Endocr Connect 2020;9:933-8. [Crossref] [PubMed]
- Zhang C, Gao X, Han Y, et al. Correlation Between Thyroid Nodules and Metabolic Syndrome: A Systematic Review and Meta-Analysis. Front Endocrinol (Lausanne) 2021;12:730279. [Crossref] [PubMed]
- Zhang F, Teng D, Tong N, et al. Gender-Specific Associations Between Metabolic Disorders and Thyroid Nodules: A Cross-Sectional Population-Based Study from China. Thyroid 2022;32:571-80. [Crossref] [PubMed]
- Balkwill F, Mantovani A. Inflammation and cancer: back to Virchow? Lancet 2001;357:539-45. [Crossref] [PubMed]
- Franco AT, Corken A, Ware J. Platelets at the interface of thrombosis, inflammation, and cancer. Blood 2015;126:582-8. [Crossref] [PubMed]
- Zhang Z, Xia F, Wang W, et al. The systemic immune-inflammation index-based model is an effective biomarker on predicting central lymph node metastasis in clinically nodal-negative papillary thyroid carcinoma. Gland Surg 2021;10:1368-73. [Crossref] [PubMed]
- Cheong TY, Hong SD, Jung KW, et al. The diagnostic predictive value of neutrophil-to-lymphocyte ratio in thyroid cancer adjusted for tumor size. PLoS One 2021;16:e0251446. [Crossref] [PubMed]
- Szczepanek-Parulska E, Hernik A, Ruchała M. Anemia in thyroid diseases. Pol Arch Intern Med 2017;127:352-60. [Crossref] [PubMed]
- Alba AC, Agoritsas T, Walsh M, et al. Discrimination and Calibration of Clinical Prediction Models: Users' Guides to the Medical Literature. JAMA 2017;318:1377-84. [Crossref] [PubMed]
(English Language Editor: J. Jones)