Computed tomography-based radiomics and body composition analysis for predicting clinically relevant postoperative pancreatic fistula after pancreaticoduodenectomy
Highlight box
Key findings
• We have developed a model that combines radiomics and body composition analysis, which can be used for accurate preoperative prediction of clinically relevant postoperative pancreatic fistula (CR-POPF).
What is known and what is new?
• Previous studies have found that both computed tomography (CT)-based radiomics and body composition analysis can be used for predicting CR-POPF.
• We have combined quantitative body composition analysis with radiomics for the first time to enhance the predictive ability of the model. In addition, we have found that radiomics can reflect the softness and hardness of pancreatic texture.
What is the implication, and what should change now?
• By identifying high-risk CR-POPF patients before surgery, nutritional support can be provided to reduce risks and facilitate preoperative doctor-patient communication. At the same time, surgery for such patients should be performed by experienced physicians.
Introduction
Pancreaticoduodenectomy (PD) is the main surgical approach used in the treatment of pancreatic diseases involving the head of the pancreas. There is a high incidence of postoperative morbidity and mortality with PD due to its extensive resections, intricate vasculature of the anatomical region, and the complexity of reconstructive anastomoses (1). As one of the most common and dangerous postoperative complications of PD, the incidence of postoperative pancreatic fistula (POPF) still fluctuates between 5% and 40% (1,2), which seriously affects the early survival and long-term prognosis of patients, prolongs hospital stay, and increases medical costs (3,4). For the needs of clinical practice and research, the International Study Group of Pancreatic Surgery (ISGPS) proposed the concept of clinically relevant POPF (CR-POPF) in 2016, including grade B pancreatic fistula and grade C pancreatic fistula. CR-POPF emphasizes the changes in clinical interventions or clinical outcomes caused by POPF, not just the increase in amylase in abdominal drainage fluid (5). The incidence of CR-POPF among patients undergoing PD is approximately 10–28% at present (6).
The widely used risk assessment tools for CR-POPF are pancreatic fistula risk score (FRS) (7) and alternative pancreatic fistula risk score (A-FRS) (8), but both are mainly based on intraoperative factors which are not conducive to the preoperative evaluation of CR-POPF. Moreover, the intraoperative evaluation of pancreatic texture is subjective due to deviations in judgment standards between operators (9). The accuracy of pancreatic texture evaluation has reduced further as a result of the popularity of laparoscopic technique, making it more challenging to standardize the evaluation (10). Therefore, it is necessary to develop a preoperative risk assessment method with more objective factors.
Compared with the qualitative or semiquantitative data obtained through imaging technology, radiomics technology enables in-depth analysis and high-throughput analysis of imaging data, which can be used as a biomarker for disease diagnosis, grading, and prognosis evaluation (11-14). The radiomics features extracted from the pancreatic parenchyma include shape-based features and textural features and these features may reflect the textural pattern or tissue distribution, pancreatic texture, degree of pancreatic fibrosis, and pancreatic duct dilatation related to POPF (7,15). To date, several studies have used radiomics to predict POPF and showed good predictive performance (16,17). Therefore, it is reasonable to assume that radiomics has great potential in predicting CR-POPF.
Body composition analysis is another important aspect of POPF risk assessment (18,19). To date, many studies have shown the value of computed tomography (CT)-based body composition analysis in predicting POPF, which can describe the distribution of adipose and skeletal muscle tissue quantitatively (8,20-22). The visceral fat distribution reflects fat infiltration of the pancreas (20) and visceral obesity may indicate greater pancreatic parenchymal fragility (22). The pancreatic leak occurring in a fatty environment is more likely to be harmful because of the lipolytic activity of pancreatic enzymes (23). Meanwhile, the decrease in skeletal muscle is an objective index reflecting the fragility of patients and is associated with delayed healing postoperatively (24). At the same time, skeletal muscle may secrete specific myokines to resist the harmful effects of proinflammatory adipokines (cytokines) expressed in obesity. However, this inhibitory effect is weakened when skeletal muscle is reduced (25).
At present, there is no relevant research on the application of combining radiomics, pancreatic duct diameter, and body composition analysis to the prediction of CR-POPF in patients undergoing PD. Hence, the purpose of this study was to establish a combined model that incorporates the radiomics score (Rad-score), pancreatic duct diameter, and body composition analysis indicators and compare it with a single Rad-score or clinical model that incorporates pancreatic duct diameter and body composition analysis to determine the predictive value of the combined model. We present this article in accordance with the TRIPOD reporting checklist (available at https://gs.amegroups.com/article/view/10.21037/gs-24-167/rc).
Methods
Patient characteristics
The study’s whole procedure is depicted in Figure 1. The study encompassed patients who were treated with PD at Wushan County People’s Hospital between January 2015 and November 2021. The inclusion criteria were as follows: (I) available preoperative contrast-enhanced CT scan performed within 1 month before PD; (II) patients with complete perioperative clinical data, including history of present illness, personal history, past history, and laboratory examination; (III) complete operation-related data and postoperative pathology results and amylase level of abdominal drainage fluid; and (IV) complete postoperative treatment plan and nursing-related data. The exclusion criteria were as follows: (I) patients with limited CT examinations due to technical issues or poor image quality; and (II) the lack of necessary clinical data affecting the judgment of CR-POPF. Consequently, a total of 139 patients were enrolled according to the inclusion and exclusion criteria (Figure S1).
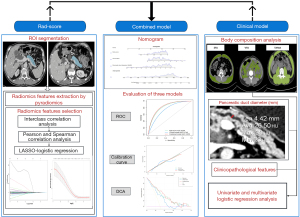
The study was conducted in accordance with the Declaration of Helsinki (as revised in 2013). The study was approved by the Ethics Committee of Wushan County People’s Hospital of Chongqing (No. WSEC2023-06) and the requirement for written informed consent to participate was waived for its retrospective nature.
Surgical procedure
All PD procedures were completed by the same team of surgeons of hepatobiliary surgery at Wushan County People’s Hospital. The operation experience is rich, the team members are fixed, and the operation process is consistent with standard PD. The digestive tract was reconstructed by the Child method, that is, according to the pancreaticojejunal, bilioenteric, and gastrointestinal anastomosis. The pancreaticoduodenal anastomosis adopts duct-to-mucosa anastomosis, end-to-end or end-to-side of the proximal end of the stump and the jejunum. Three intra-abdominal drains and one pancreatic duct stent were routinely placed in all patients. The first one is a silicone groove drainage tube, which passes through the tail of the pancreaticojejunostomy, behind the pancreaticojejunostomy and bilioenterostomy to the hepatorenal space, and is led out through the left abdominal wall. The second intra-abdominal drain also uses a silicone groove tube, which passes in front of the biliary-enteric anastomosis, points to the splenic fossa along the liver, reaches the deepest part of the lesser omental sac, exits through the right abdominal wall, and crosses the first drainage tube on the right side of the biliary-enteric anastomosis. The third intra-abdominal drain is a latex single-lumen tube, placed in front of the pancreaticojejunostomy, pointing to the lesser omental sac, exiting through the right abdominal wall, crossing with drain 1 at the tail of the pancreaticojejunostomy, and crossing with drain 2 at the lesser omental sac.
During the process of pancreatic duct stent drainage, the tube at one end was cut into 3–4 lateral holes and placed into the main pancreatic duct. In the internal drainage group, the other end of the pancreatic duct stent tube was placed 1–2 cm into the jejunum through the pancreaticojejunostomy, allowing pancreatic juice to be discharged into the jejunum. In the external drainage group, the other end of the pancreatic duct stent tube was inserted into the jejunum and moved about 10–15 cm along the free end of the jejunum. Then, the pancreatic duct stent tube was punctured from the jejunum to the abdominal cavity and led out through the abdominal wall to form external drainage. Unified and standardized postoperative management was carried out after the operation (Appendix 1).
CT-based body composition analysis
The enhanced CT process and equipment parameters are shown in Appendix 2. Height and weight were obtained from medical records. Body mass index (BMI) was calculated as weight (kg)/height2 (m2). The visceral fat area (VFA) (cm2) and subcutaneous fat area (SFA) (cm2) were measured on CT images at the level of the umbilicus, and the total abdominal muscle area (TAMA) (cm2) was measured at the level of the third lumbar vertebra (L3). Specific tissue demarcation using predefined Hounsfield unit (HU) thresholds was performed by Syngo Via (version vb20; Siemens, Erlangen, Germany) software (Figure S2). Tissue boundaries were obtained by semi-automated measurement and manual outlining, and corrected manually as needed by a radiologist with rich experience in abdominal image diagnosis who was blinded to the clinical data. VFA and SFA were identified using the following adipose tissue thresholds: −150 to −50 HU and −190 to −30 HU, respectively. TAMA was identified using the following muscle tissue threshold: −29 to +150 HU. The TAMA, including paravertebral muscle and abdominal wall muscle, was normalized to stature by dividing the muscle area by the patient’s height squared, which is termed the TAMA index [TAMAI = TAMA (cm2)/height2 (m2)]. The HU average calculation (HUAC) is measured in HU and consists of the average of the measurements of the densitometric attenuation coefficient by the muscular adipose infiltration of the right and left psoas (26). It is calculated according to the formula HUAC = (right psoas density/right psoas area) + (left psoas density/left psoas area)/right + left psoas area (Figure S3).
Radiomic feature extraction and selection
Contrast-enhanced CT image acquisition parameters are detailed in Appendix 2. The CT images of each patient in the portal vein phase were exported from the image storage and transmission system and uploaded to 3D Slicer (version 4.11.20210226, open-source software, https://www.slicer.org/) in Digital Imaging and Communications in Medicine (DICOM) format. The drawing tool available in the Editor module of 3D Slicer was used to segment the region of interest (ROI), and an ROI was defined in this study as the plane of the body and tail of the pancreas that can expose the maximum diameter of the pancreatic duct in the portal venous phase of abdominal contract-enhanced CT (Figure S4A). The pancreatic duct diameter was measured at the same section with ROI by Syngo Via (version vb20) software (Figure S4B). When the tumor or other focus was present on the section, we chose the normal pancreatic body and tail tissue as the ROI without the tumor or other focus intended for resection. The segmentation of the ROI was independently completed by two doctors who had more than 10 years of experience in interpretation related to abdominal CT imaging and were blinded to the clinical data. The radiomics function module of 3D Slicer was used to extract the radiomics features.
The Z score was used for standardization of radiomics features. The interclass correlation coefficient (ICC) was used to evaluate the consistency between the two observers, and radiomics features with ICC <0.75 were excluded (Figure S5). Spearman correlation analysis and Pearson correlation analysis were used to analyze the linear correlation between each feature. For a set of features with correlation coefficients greater than 0.9, one of them was deleted randomly. Furthermore, the least absolute shrinkage and selection operator (LASSO) logistic regression method by 10-fold cross-validation was used to select the most useful features (Figure S6). The Rad-scores were calculated for each patient by a linear combination of selected features weighted by their respective coefficients.
Models building and validation
The impacts of clinicopathological features, pancreatic duct diameter, and body composition measures on CR-POPF were assessed by univariate and multivariate logistic regression analysis; only statistically significant variables in univariate analysis were entered into multivariate logistic regression analysis. Then, variables with P<0.05 in the multivariate logistic regression analysis were used to build a preoperative clinical model, and these variables were used together with the Rad-score to build a preoperative combined model. The models were internally validated by 1,000 bootstrap resampling and the evaluation indicators of model performance, including the sensitivity, specificity, and the area under the receiver operating characteristic (ROC) curve (AUC), were the averages obtained from 1,000 times bootstrap resampling.
Statistical analysis
The Kolmogorov-Smirnov test was used to test the normality of the data. Continuous data conforming to a normal distribution were expressed as the mean ± standard deviation (SD), and continuous data with a nonnormal distribution were expressed as the median (upper and lower quartiles). Categorical data were expressed as frequencies and percentages. The Chi-squared test was used for the comparison between categorical variables. The Mann-Whitney U test was used for the comparison of nonnormally distributed continuous variables, and an independent sample t-test was used for the comparison of normally distributed continuous variables. The combined model and clinical model were constructed by multivariate logistic regression. ROC curve and AUC were used to evaluate the discrimination of the model. The calibration curve was used to evaluate the calibration of the model; decision curve analysis (DCA) was performed to compare the net benefits at different threshold probabilities. The accuracy, sensitivity, specificity, positive predictive value (PPV), and negative predictive value (NPV) were also calculated at a cut-off value that maximized the value of the Youden index. DeLong’s test was used to compare the performance of different models. All the above analyses were completed using R software (version 3.6.3; www.r-project.org). Statistical significance was indicated by a P value less than 0.05.
Results
Patient characteristics
A total of 139 consecutive patients undergoing PD were included in this study. They were divided into a non-CR-POPF group (n=107) and a CR-POPF group (n=32). The incidence of CR-POPF in this study was 23.02%. The CR-POPF group had a higher BMI (23.72 vs. 22.26 kg/m2, P=0.01), larger SFA (150.09 vs. 122.82 cm2, P=0.03), smaller pancreatic duct diameter (3.85 vs. 6.3 mm, P=0.001), larger VFA/TAMAI (2.80 vs. 1.84, P=0.001), higher proportion of soft pancreas (71.88% vs. 23.36%, P<0.001), and higher preoperative total bilirubin level (94.40 vs. 51.60 µmol/L, P=0.03). The two groups were not significantly different in age, sex, smoking history, drinking history, past history (diabetes, hypertension, preoperative pancreatitis, abdominal operation history), serum albumin, Controlling Nutritional status (CONUT) grade, HUAC, American Society of Anesthesiologists (ASA) grading, operative time, diameter of pancreatic duct stent, intraoperative blood loss, intraoperative blood transfusion, pancreatic fluid drainage method, and pancreaticoenteric anastomosis method (Table 1).
Table 1
Characteristics | Non-CR-POPF (n=107) | CR-POPF (n=32) [grade B POPF (n=27) + grade C POPF (n=5)] | P value |
---|---|---|---|
Age (years) | 61.00 [51.00, 67.00] | 65.00 [57.75, 70.25] | 0.10 |
Sex | 0.49 | ||
Male | 63 (58.88) | 21 (65.63) | |
Female | 44 (41.12) | 11 (34.38) | |
Smoking history | 0.36 | ||
No | 57 (53.27) | 20 (62.50) | |
Yes | 50 (46.73) | 12 (37.50) | |
Drinking history | 0.21 | ||
No | 71 (66.36) | 25 (78.13) | |
Yes | 36 (33.64) | 7 (21.87) | |
History of abdominal surgery | 0.84 | ||
No | 69 (64.49) | 20 (62.50) | |
Yes | 38 (35.51) | 12 (37.50) | |
Preoperative pancreatitis | 0.09 | ||
No | 92 (85.98) | 31 (96.88) | |
Yes | 15 (14.02) | 1 (3.13) | |
Hypertension | 0.91 | ||
No | 86 (80.37) | 26 (81.25) | |
Yes | 21 (19.63) | 6 (18.75) | |
Diabetes | 0.21 | ||
No | 87 (81.31) | 29 (90.63) | |
Yes | 20 (18.69) | 3 (9.38) | |
BMI (kg/m2) | 22.26±2.71 | 23.72±2.72 | 0.01 |
SFA (cm2) | 122.82±58.89 | 150.09±60.04 | 0.03 |
VFA (cm2) | 79.26 [53, 108.38] | 111.51 [70.9, 145.44] | 0.002 |
TAMAI | 43.24±7.04 | 42.01±8 | 0.40 |
VFA/TAMAI | 1.84 [1.16, 2.64] | 2.80 [1.77, 3.35] | 0.001 |
HUAC | 43.96±9.12 | 42.56±10.65 | 0.46 |
ONS | 0.93 | ||
TPN | 75 (70.09) | 23 (71.88) | |
SP | 27 (25.23) | 8 (25.00) | |
TP | 5 (4.67) | 1 (3.13) | |
Pancreatic duct diameter (mm) | 6.3 [3.87, 8.91] | 3.85 [3.1, 5.14] | 0.001 |
Diameter of pancreatic duct stent (Fr) | 0.07 | ||
5 | 7 (6.54) | 4 (12.50) | |
6 | 28 (26.17) | 14 (43.75) | |
7.5 | 16 (14.95) | 7 (21.88) | |
8 | 16 (14.95) | 4 (12.50) | |
9 | 19 (17.76) | 3 (9.38) | |
10.5 | 16 (14.95) | 0 | |
12 | 5 (4.67) | 0 | <0.001 |
Pancreas texture | |||
Hard | 82 (76.64) | 9 (28.13) | |
Soft | 25 (23.36) | 23 (71.88) | |
Albumin (g/L) | 37.54±3.96 | 36.38±3.80 | 0.18 |
Total bilirubin (μmol/L) | 51.60 [10.25, 150.55] | 94.40 [59.23, 174.58] | 0.03 |
Total cholesterol (mg/dL) | 177.5 [150.81, 218.29] | 198.69 [168.94, 265.46] | 0.04 |
Total lymphocyte count (/mm2) | 1,180 [945, 1,540] | 1,085 [937.5, 1,610] | 0.62 |
ASA grade | 0.77 | ||
I | 1 (0.93) | 1 (3.13) | |
II | 56 (52.34) | 17 (53.13) | |
III | 49 (45.79) | 14 (43.75) | |
IV | 1 (0.93) | 0 | |
Operation time (minutes) | 365.00 [315.00, 430.00] | 375.00 [350.00, 441.25] | 0.32 |
Intraoperative blood loss (mL) | 0.74 | ||
<700 | 84 (78.50) | 26 (81.25) | |
≥700 | 23 (21.50) | 6 (18.75) | |
Intraoperative blood transfusion | 0.62 | ||
No | 72 (67.29) | 20 (62.50) | |
Yes | 35 (32.71) | 12 (37.50) | |
Pancreatic fluid drainage method | 0.056 | ||
Internal drainage | 54 (50.47) | 10 (31.25) | |
External drainage | 53 (49.53) | 22 (68.75) | |
Pancreaticoenteric anastomosis method | 0.53 | ||
Duct-to-mucosa | 67 (62.62) | 20 (62.50) | |
End-to-end | 24 (22.43) | 5 (15.63) | |
End-to-side | 16 (14.95) | 7 (21.88) | |
Results of postoperative pathology | 0.91 | ||
Neoplasm | 91 (85.05) | 29 (90.63) | |
Chronic pancreatitis/pancreatic duct stone | 9 (8.41) | 2 (6.25) | |
Others | 7 (6.54) | 1 (3.13) | |
CONUT grade | 0.56 | ||
Normal | 30 (28.04) | 10 (31.25) | |
Mild | 61 (57.01) | 15 (46.88) | |
Moderate | 15 (14.02) | 7 (21.88) | |
Severe | 1 (0.93) | 0 |
Categorical data were expressed as frequencies (percentages). Continuous data conforming to a normal distribution were expressed as the mean ± standard deviation, and continuous data with a non-normal distribution were expressed as the median [upper, lower quartiles]. CR-POPF, clinically relevant postoperative pancreatic fistula; BMI, body mass index; SFA, subcutaneous fat area; VFA, visceral fat area; TAMAI, total abdominal muscle area index; HUAC, Hounsfield unit average calculation; ONS, oral nutritional supplements; TPN, total parenteral nutrition; SP, short peptide formula; TP, total protein formula; Fr, French; ASA, American Society of Anesthesiologists; CONUT, Controlling Nutritional status.
Radiomics signature construction
A total of 1,130 groups of five categories of features were extracted, including shape features (n=14), first-order features (n=18), texture features: gray level cooccurrence matrix features (n=24), gray level dependence matrix features (n=14), gray level size zone matrix features (n=16), gray level run length matrix features (n=16), neighboring gray tone difference matrix features (n=5), wavelet features (n=744), and features based on the Laplacian of Gaussian filter (n=279).
After ICC and Spearman or Pearson correlation analysis, 196 features remained. LASSO-logistic regression yielded 11 radiomics features related to CR-POPF and the corresponding coefficients (Figure S6). The formula for calculating the Rad-score is shown in Table 2. Through this formula, we calculated the Rad-score of each patient. The Rad-score of patients with CR-POPF was significantly higher than that of patients without CR-POPF (Figure 2).
Table 2
Variables | Coefficient |
---|---|
Intercept | −1.2034 |
Log-sigma-3-0-mm-3D_firstorder_90Percentile | −0.5046 |
Log-sigma-3-0-mm-3D_glrlm_RunEntropy | 0.1242 |
Log-sigma-3-0-mm-3D_glrlm_RunVariance | 0.0051 |
Log-sigma-5-0-mm-3D_ngtdm_Coarseness | −0.8381 |
Wavelet-LLH_glrlm_RunLengthNonUniformity | 0.0242 |
Wavelet-LLH_glszm_SmallAreaEmphasis | 0.1364 |
Wavelet-LHL_glszm_LargeAreaHighGrayLevelEmphasis | 0.0280 |
Wavelet-LHL_glszm_SmallAreaEmphasis | 0.6832 |
Wavelet-HLL_glszm_LargeAreaHighGrayLevelEmphasis | 0.0096 |
Wavelet-HLH_ngtdm_Contrast | −0.1069 |
Wavelet-LLL_glszm_LargeAreaHighGrayLevelEmphasis | 0.1628 |
Rad, radiomics; LLH, low-low-high; LHL, low-high-low; HLL, high-low-low; HLH, high-low-high; LLL, low-low-low.
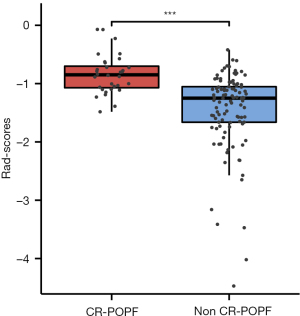
Models development and validation
The body composition measures, pancreatic duct diameter, and clinicopathological features were included in the univariate logistic regression. The variables with P<0.05 included pancreatic duct diameter, BMI, SFA, VFA, and VFA/TAMAI. The above variables except VFA were included in the multivariate logistic regression, as a result, the VFA/TAMAI and pancreatic duct diameter were variables with P<0.05 (Table 3).
Table 3
Variables | Univariate | Multivariate | |||
---|---|---|---|---|---|
OR (95% CI) | P value | OR (95% CI) | P value | ||
Sex | |||||
Male | 1.00 | – | – | ||
Female | 0.75 (0.32, 1.71) | 0.49 | – | – | |
Age (years) | 1.02 (0.99, 1.07) | 0.13 | – | – | |
Smoking history | |||||
No | 1.00 | – | – | ||
Yes | 0.68 (0.30, 1.54) | 0.36 | – | – | |
Drinking history | |||||
No | 1.00 | – | – | ||
Yes | 0.55 (0.22, 1.40) | 0.21 | – | – | |
History of abdominal surgery | |||||
No | 1.00 | – | – | ||
Yes | 1.09 (0.48, 2.47) | 0.84 | – | – | |
Preoperative pancreatitis | |||||
No | 1.00 | – | – | ||
Yes | 0.20 (0.03, 1.56) | 0.12 | – | – | |
Hypertension | |||||
No | 1.00 | – | – | ||
Yes | 0.95 (0.35, 2.59) | 0.91 | – | – | |
Diameter of pancreatic duct stent (Fr) | |||||
5 | 1.00 | – | – | ||
6 | 0.87 (0.22, 3.5) | 0.85 | – | – | |
7.5 | 0.77 (0.17, 3.49) | 0.73 | – | – | |
8 | 0.44 (0.08, 2.27) | 0.33 | – | – | |
9 | 0.28 (0.05, 1.56) | 0.15 | – | – | |
10.5 | 0 (0, Inf) | 0.99 | – | – | |
12 | 0 (0, Inf) | 0.99 | – | – | |
Diabetes | |||||
No | 1.00 | – | – | ||
Yes | 0.45 (0.13, 1.63) | 0.22 | – | – | |
BMI (kg/m2) | 1.23 (1.05, 1.44) | 0.01 | 1.32 (0.94, 1.37) | 0.20 | |
Albumin (g/L) | 0.93 (0.84, 1.03) | 0.15 | – | – | |
Total bilirubin (μmol/L) | 1.00 (1.00, 1.01) | 0.09 | – | – | |
CONUT grade | |||||
Normal | 1.00 | – | – | ||
Mild | 0.74 (0.3, 1.84) | 0.51 | – | – | |
Moderate | 1.4 (0.44, 4.41) | 0.57 | – | – | |
Severe | 0 (0, Inf) | 0.99 | – | – | |
HUAC | 0.98 (0.94, 1.03) | 0.46 | – | – | |
ONS | |||||
TPN | 1.00 | – | – | ||
SP | 0.97 (0.39, 2.42) | 0.94 | – | – | |
TP | 0.65 (0.07, 5.87) | 0.70 | – | – | |
Results of postoperative pathology | |||||
Neoplasm | 1.00 | – | – | ||
Chronic pancreatitis/pancreatic duct stone | 0.70 (0.14, 3.41) | 0.66 | – | – | |
Others | 0.45 (0.05, 3.80) | 0.46 | – | – | |
ASA grade | |||||
I | 1.00 | – | – | ||
II | 0.30 (0.02, 5.12) | 0.41 | – | – | |
III | 0.29 (0.02, 4.87) | 0.39 | – | – | |
IV | 0 (0, Inf) | 0.99 | – | – | |
Operation time (minutes) | 1.00 (0.99, 1.01) | 0.41 | – | – | |
Intraoperative blood loss (mL) | |||||
<700 | 1.00 | – | – | ||
≥700 | 0.84 (0.31, 2.29) | 0.74 | – | – | |
Pancreatic fluid drainage method | |||||
Internal drainage | 1.00 | – | – | ||
External drainage | 2.24 (0.97, 5.18) | 0.059 | – | – | |
Intraoperative blood transfusion | |||||
No | 1.00 | – | – | ||
Yes | 1.23 (0.54, 2.81) | 0.62 | – | – | |
SFA (cm2) | 1.01 (1.00, 1.01) | 0.03 | 0.99 (0.99, 1.01) | 0.91 | |
VFA (cm2) | 1.01 (1, 1.02) | 0.003 | – | – | |
TAMAI | 0.98 (0.92, 1.03) | 0.40 | – | – | |
VFA/TAMAI | 1.72 (1.22, 2.42) | 0.002 | 1.55 (1.02, 2.27) | 0.04 | |
Pancreatic duct diameter (mm) | 0.78 (0.67, 0.92) | 0.003 | 0.8 (0.68, 0.94) | 0.008 |
OR, odds ratio; CI, confidence interval; Inf, infinity; Fr, French; BMI, body mass index; CONUT, Controlling Nutritional status; HUAC, Hounsfield unit average calculation; ONS, oral nutritional supplements; TPN, total parenteral nutrition; SP, short peptide formula; TP, total protein formula; ASA, American Society of Anesthesiologists; SFA, subcutaneous fat area; VFA, visceral fat area; TAMAI, total abdominal muscle area index.
Finally, VFA/TAMAI and pancreatic duct diameter were included in the clinical model and the combined model with Rad-score. The ROC curve of the training set is shown in Figure 3A. Moreover, we validated the model internally through 1,000 bootstrap resampling and compared the predictive efficiency of the Rad-score, clinical model, and combined model. The ROC curve of each model after 1,000 bootstrap resampling is shown in Figure 3B, and the AUC values were 0.824 [95% confidence interval (CI): 0.73–0.889], 0.745 (95% CI: 0.642–0.832), and 0.839 (95% CI: 0.757–0.907), respectively. The accuracy, sensitivity, specificity, PPV, and NPV are listed in Table 4.
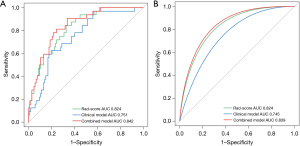
Table 4
Variables | AUC | P value | Accuracy | Sensitivity | Specificity | PPV | NPV |
---|---|---|---|---|---|---|---|
Training set | |||||||
Clinical model | 0.751 | 0.02§ | 0.755 | 0.625 | 0.804 | 0.429 | 0.934 |
Rad-score | 0.824 | 0.14† | 0.705 | 0.844 | 0.673 | 0.476 | 0.876 |
Combined model | 0.842 | 0.24‡ | 0.712 | 0.906 | 0.664 | 0.438 | 0.947 |
Validation set by 1,000 bootstrap resampling | |||||||
Clinical model | 0.745 | 0.02§ | 0.646 | 0.755 | 0.613 | 0.368 | 0.893 |
Rad-score | 0.824 | 0.14† | 0.725 | 0.789 | 0.706 | 0.445 | 0.917 |
Combined model | 0.839 | 0.24‡ | 0.738 | 0.802 | 0.719 | 0.46 | 0.924 |
§, the P value reflects the comparison between the clinical model and the combined model; †, the P value reflects the comparison between the clinical model and the Rad-score; ‡, the P value reflects the comparison between the Rad-score and the combined model. AUC, area under the receiver operating characteristic curve; PPV, positive predictive value; NPV, negative predictive value; Rad, radiomics.
The calibration curves of the three models are shown in Figure 4A. It can be seen that the three models had good calibration, but the calibration of the combined model and Rad-score were better than clinical model. The DCA curves of the three models are shown in Figure 4B. When the risk threshold was between 10% and 28%, the net benefits of the combined model were greater than those of the clinical model and Rad-score. The preoperative combined model is displayed in the form of a nomogram in Figure 5A. To facilitate validation by other researchers, we designed the nomogram as a web-based calculator (Figure 5B). The web-based calculator can be used directly by visiting https://whyyjyljz.shinyapps.io/DynNomapp/.
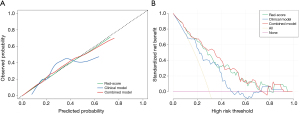
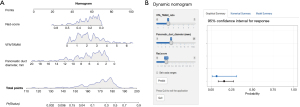
In addition, because the soft texture of pancreas is a recognized risk factor for CR-POPF (27), we added the data of pancreatic texture obtained by intraoperative palpation. Subsequently, we combined the pancreatic duct diameter, VFA/TAMAI, and Rad-score with pancreatic texture, and constructed the intraoperative clinical model and intraoperative combined model by logistic regression. The AUC values of the intraoperative clinical model and intraoperative combined model in the training set reached 0.808 (95% CI: 0.720–0.896) and 0.860 (95% CI: 0.793–0.927), respectively. The AUC values of these two models in the validation set reached 0.799 (95% CI: 0.709–0.879) and 0.857 (95% CI: 0.777–0.915), respectively (Figure S7A,S7B). Moreover, the efficacy of the intraoperative combined model was verified by DCA and calibration curve analysis (Figure S7C,S7D). In order to facilitate usage and external verification, we transformed it into a nomogram (Figure S7E).
Interestingly, we divided the patients into a soft pancreas group and a hard pancreas group according to the pancreas texture. Comparing the Rad-score between the two groups, we found that the Rad-score of the soft pancreas group was significantly higher than that of the hard pancreas group (P<0.001) (Figure S7F).
Discussion
In this study, the clinical model was established by pancreatic duct diameter and VFA/TAMAI, and the combined model was established by pancreatic duct diameter, VFA/TAMAI, and Rad-score. All variables included in the three models were collected from preoperative CT images. The preoperative combined model was superior to the Rad-score and clinical model in discrimination and net benefit. Furthermore, Rad-score and combined model were superior to clinical model in calibration.
Several studies have reported that imaging features including pancreatic duct diameter measured by preoperative CT are independently related to POPF (28-30). We measured the maximum of the pancreatic duct diameter by preoperative CT at the section revealing the maximum diameter of the pancreatic duct at the tail and body, and found that this variable was an independent risk factor for CR-POPF. When the R1 margin of PDAC is found by intraoperative frozen biopsy, it is often necessary to expand the scope of pancreatic resection. At this time, the position of the anastomosis will change compared with the preoperative estimated position (31,32). Therefore, the measurement of the maximum diameter of the pancreatic duct is more objective than the pre-operative estimated diameter of the pancreatic duct at the anastomosis. Moreover, 11 radiomics features extracted from the pancreatic parenchyma were used to establish the Rad-score. Zhang et al. used preoperative CT portal venous phase images to extract 1,219 radiomics features from pancreatic parenchyma and selected 11 features to develop a calculation formula to obtain a Rad-score to predict POPF (16). Subsequently, we found that the Rad-score of the soft pancreas group was significantly higher than that of the hard pancreas group, which showed that the radiomics features we obtained could reflect pancreatic texture. Lin et al. extracted 102 radiomics features from the proximal 20–60% from the head to the tail of pancreas and the nomogram was developed to predict CR-POPF with 11 selected radiomics features, demographic information, and radiological features (17). However, we found that the radiomics features extracted in our study were different from the radiomics features in the studies of Lin et al. and Zhang et al. This difference may be related to different outcomes, image data sources, image quality, and feature extraction methods between these studies. This difference illustrates that more studies are needed to improve the biological interpretability of radiomics for predicting CR-POPF and to assess the heterogeneity of features between different models.
BMI, SFA, VFA, and VFA/TAMAI were included as body composition analysis indicators and we found that BMI, SFA, and TAMAI were not independent risk factors for CR-POPF. BMI defines the overall obesity status of the body, including different states dominated by subcutaneous fat and visceral fat. Visceral fat includes intraperitoneal fat and retroperitoneal peripancreatic fat, which reflect pancreatic fatty infiltration related to POPF (33,34). Thus, correlation between VFA and POPF is stronger than that between BMI and POPF. In the same way, SFA is not an independent risk factor of CR-POPF, which partly explains why visceral obesity is a more rational risk factor of CR-POPF. VFA could not be included in the multivariate logistic-regression analysis simultaneously with VFA/TAMAI because there is collinear between VFA and VFA/TAMAI. A previous study compared VFA and TAMAI alone with VFA/TAMAI, and confirmed that VFA/TAMAI had better for CR-POPF (22). When combined with visceral obesity as defined by VFA/TAMAI, skeletal muscle loss produces insulin resistance related to metabolic syndrome and surgical stress response (35). Moreover, biochemical leak (BL) is more likely to progress to more dangerous CR-POPF due to severe celiac lipolysis in patients with sarcopenic obesity (22). Thus, VFA/TAMAI was included as a body composition analysis predictor in the clinical model and the combined model. However, since the patients in this cohort did not undergo bioimpedance electric analysis and functional assessment of dynamometer, our model did not have these two potential CR-POPF-related body composition analyses (36,37).
The better performance of the preoperative combined model than the other models in the validation set illustrates that the information provided by radiomics, pancreatic duct diameter, or body composition analysis alone is still incomplete. Radiomics or pancreatic duct diameter only provides the characteristics of the pancreas itself, but in fact, CR-POPF is closely related to the patient’s general condition and the visceral environment of the pancreas. Therefore, a combined model is more valuable for predicting CR-POPF.
This study also has some limitations. First, this study is a single-center retrospective study, which needs to be validated by a large multicenter prospective cohort. Second, in this study, only internal validation by 1,000 bootstrap resamples of three models was carried out, and no external validation was conducted. Third, we did not compare the combined model with the currently widely used FRS model due to the lack of necessary clinical information in some patients such as intraoperative direct measurement of pancreatic duct diameter. The pancreatic duct diameter used in our model was the maximum diameter of pancreatic duct measured by CT, rather than the actual diameter of the pancreatic duct at the anastomosis. However, previous studies (16,17) have confirmed that Rad-score is superior to the FRS model in predicting POPF. In addition, limited by our sample size, we did not analyze patients with grade B POPF or grade C POPF. Moreover, the current study shows that the risk factors of pancreatic fistula after distal pancreatectomy include combined organ resection, the closure methods of pancreatic stump, and diabetes (38,39), so our model is not suitable for patients with distal pancreatectomy.
Conclusions
We explored a potential approach of combining radiomics features, pancreatic duct diameter, and VFA/TAMAI in preoperative assessment for risk of CR-POPF and established a combined model based on these factors. The combined model outperformed a single Rad-score and clinical model, but larger sample-sized studies are needed to validate the generalization and stability of this model and show the biological interpretability of radiomics and body composition analysis in predicting CR-POPF.
Acknowledgments
We would like to thank Dr. Feng Yang from the University College London Institute for Liver and Digestive Health for his help in polishing our paper.
Funding: This study was funded by
Footnote
Reporting Checklist: The authors have completed the TRIPOD reporting checklist. Available at https://gs.amegroups.com/article/view/10.21037/gs-24-167/rc
Data Sharing Statement: Available at https://gs.amegroups.com/article/view/10.21037/gs-24-167/dss
Peer Review File: Available at https://gs.amegroups.com/article/view/10.21037/gs-24-167/prf
Conflicts of Interest: All authors have completed the ICMJE uniform disclosure form (available at https://gs.amegroups.com/article/view/10.21037/gs-24-167/coif). D.Y. reports that funding for the study was provided by Chongqing Medical Scientific Research Project of Chongqing Health Commission (No. 2023WSJK096). J.L. reports that funding for the study was provided by the Kuanren Talents Program of the Second Affiliated Hospital of Chongqing Medical University, Senior Medical Talents Program of Chongqing for Young and Middle-aged, and Joint Project of Chongqing Health Commission and Science and Technology Bureau (No. 2022GDRC004). The other authors have no conflicts of interest to declare.
Ethical Statement: The authors are accountable for all aspects of the work in ensuring that questions related to the accuracy or integrity of any part of the work are appropriately investigated and resolved. The study was conducted in accordance with the Declaration of Helsinki (as revised in 2013). The study was approved by the Ethics Committee of Wushan County People’s Hospital of Chongqing (No. WSEC2023-06) and the requirement for written informed consent to participate was waived due to its retrospective nature.
Open Access Statement: This is an Open Access article distributed in accordance with the Creative Commons Attribution-NonCommercial-NoDerivs 4.0 International License (CC BY-NC-ND 4.0), which permits the non-commercial replication and distribution of the article with the strict proviso that no changes or edits are made and the original work is properly cited (including links to both the formal publication through the relevant DOI and the license). See: https://creativecommons.org/licenses/by-nc-nd/4.0/.
References
- Chang YR, Kang JS, Jang JY, et al. Prediction of Pancreatic Fistula After Distal Pancreatectomy Based on Cross-Sectional Images. World J Surg 2017;41:1610-7. [Crossref] [PubMed]
- Murakami Y, Uemura K, Hayashidani Y, et al. No mortality after 150 consecutive pancreatoduodenctomies with duct-to-mucosa pancreaticogastrostomy. J Surg Oncol 2008;97:205-9. [Crossref] [PubMed]
- Enestvedt CK, Diggs BS, Cassera MA, et al. Complications nearly double the cost of care after pancreaticoduodenectomy. Am J Surg 2012;204:332-8. [Crossref] [PubMed]
- Fujii T, Kanda M, Nagai S, et al. Excess Weight Adversely Influences Treatment Length of Postoperative Pancreatic Fistula: A Retrospective Study of 900 Patients. Pancreas 2015;44:971-6. [Crossref] [PubMed]
- Bassi C, Marchegiani G, Dervenis C, et al. The 2016 update of the International Study Group (ISGPS) definition and grading of postoperative pancreatic fistula: 11 Years After. Surgery 2017;161:584-91. [Crossref] [PubMed]
- Cao Z, Qiu J, Guo J, et al. A randomised, multicentre trial of somatostatin to prevent clinically relevant postoperative pancreatic fistula in intermediate-risk patients after pancreaticoduodenectomy. J Gastroenterol 2021;56:938-48. [Crossref] [PubMed]
- Trudeau MT, Casciani F, Ecker BL, et al. The Fistula Risk Score Catalog: Toward Precision Medicine for Pancreatic Fistula After Pancreatoduodenectomy. Ann Surg. 2022;275:e463-72. [Crossref] [PubMed]
- Mungroop TH, van Rijssen LB, van Klaveren D, et al. Alternative Fistula Risk Score for Pancreatoduodenectomy (a-FRS): Design and International External Validation. Ann Surg 2019;269:937-43. [Crossref] [PubMed]
- Gnanasekaran S, Durgesh S, Gurram R, et al. Do preoperative pancreatic computed tomography attenuation index and enhancement ratio predict pancreatic fistula after pancreaticoduodenectomy? World J Radiol 2022;14:165-76. [Crossref] [PubMed]
- Xu H, Meng QC, Hua J, et al. Identifying the risk factors for pancreatic fistula after laparoscopic pancreaticoduodenectomy in patients with pancreatic cancer. World J Gastrointest Surg 2024;16:1609-17. [Crossref] [PubMed]
- Avanzo M, Stancanello J, El Naqa I. Beyond imaging: The promise of radiomics. Phys Med 2017;38:122-39. [Crossref] [PubMed]
- Guiot J, Vaidyanathan A, Deprez L, et al. A review in radiomics: Making personalized medicine a reality via routine imaging. Med Res Rev 2022;42:426-40. [Crossref] [PubMed]
- Avanzo M, Wei L, Stancanello J, et al. Machine and deep learning methods for radiomics. Med Phys 2020;47:e185-202. [Crossref] [PubMed]
- Berbís MÁ, Godino FP, Rodríguez-Comas J, et al. Radiomics in CT and MR imaging of the liver and pancreas: tools with potential for clinical application. Abdom Radiol (NY) 2024;49:322-40. [Crossref] [PubMed]
- Sofue K, Ueshima E, Masuda A, et al. Estimation of pancreatic fibrosis and prediction of postoperative pancreatic fistula using extracellular volume fraction in multiphasic contrast-enhanced CT. Eur Radiol 2022;32:1770-80. [Crossref] [PubMed]
- Zhang W, Cai W, He B, et al. A radiomics-based formula for the preoperative prediction of postoperative pancreatic fistula in patients with pancreaticoduodenectomy. Cancer Manag Res 2018;10:6469-78. [Crossref] [PubMed]
- Lin Z, Tang B, Cai J, et al. Preoperative prediction of clinically relevant postoperative pancreatic fistula after pancreaticoduodenectomy. Eur J Radiol 2021;139:109693. [Crossref] [PubMed]
- Gaujoux S, Cortes A, Couvelard A, et al. Fatty pancreas and increased body mass index are risk factors of pancreatic fistula after pancreaticoduodenectomy. Surgery 2010;148:15-23. [Crossref] [PubMed]
- Lee MW, Jeon SK, Paik WH, et al. Prognostic value of initial and longitudinal changes in body composition in metastatic pancreatic cancer. J Cachexia Sarcopenia Muscle 2024;15:735-45. [Crossref] [PubMed]
- Tranchart H, Gaujoux S, Rebours V, et al. Preoperative CT scan helps to predict the occurrence of severe pancreatic fistula after pancreaticoduodenectomy. Ann Surg 2012;256:139-45. [Crossref] [PubMed]
- Jang M, Park HW, Huh J, et al. Predictive value of sarcopenia and visceral obesity for postoperative pancreatic fistula after pancreaticoduodenectomy analyzed on clinically acquired CT and MRI. Eur Radiol 2019;29:2417-25. [Crossref] [PubMed]
- Yue Y, Li M, Zhang X, et al. Prediction of clinically relevant pancreatic fistula after pancreatic surgery using preoperative CT scan: A systematic review and meta-analysis. Pancreatology 2020;20:1558-65. [Crossref] [PubMed]
- Mathur A, Marine M, Lu D, et al. Nonalcoholic fatty pancreas disease. HPB (Oxford) 2007;9:312-8. [Crossref] [PubMed]
- Ruiz M, Cefalu C, Reske T. Frailty syndrome in geriatric medicine. Am J Med Sci 2012;344:395-8. [Crossref] [PubMed]
- Pedersen BK. The diseasome of physical inactivity--and the role of myokines in muscle--fat cross talk. J Physiol 2009;587:5559-68. [Crossref] [PubMed]
- Bulut G, Atci N. Sarcopenic obesity in early breast cancer patients with metabolic syndrome: a cross-sectional study. Future Oncol 2022;18:2489-98. [Crossref] [PubMed]
- Yang F, Windsor JA, Fu DL. Optimizing prediction models for pancreatic fistula after pancreatectomy: Current status and future perspectives. World J Gastroenterol 2024;30:1329-45. [Crossref] [PubMed]
- Iseki M, Noda H, Watanabe F, et al. A deep pancreas is a novel predictor of pancreatic fistula after pancreaticoduodenectomy in patients with a nondilated main pancreatic duct. Surgery 2021;169:1471-9. [Crossref] [PubMed]
- Kolbinger FR, Lambrecht J, Leger S, et al. The image-based preoperative fistula risk score (preFRS) predicts postoperative pancreatic fistula in patients undergoing pancreatic head resection. Sci Rep 2022;12:4064. [Crossref] [PubMed]
- Ohgi K, Okamura Y, Sugiura T, et al. Pancreatic attenuation on computed tomography predicts pancreatic fistula after pancreaticoduodenectomy. HPB (Oxford) 2020;22:67-74. [Crossref] [PubMed]
- Petrucciani N, Nigri G, Debs T, et al. Frozen section analysis of the pancreatic margin during pancreaticoduodenectomy for cancer: Does extending the resection to obtain a secondary R0 provide a survival benefit? Results of a systematic review. Pancreatology 2016;16:1037-43. [Crossref] [PubMed]
- Jung JH, Yoon SJ, Lee OJ, et al. Intraoperative Positive Pancreatic Parenchymal Resection Margin: Is It a True Indication of Completion Total Pancreatectomy after Partial Pancreatectomy for Pancreatic Ductal Adenocarcinoma? Curr Oncol 2022;29:5295-305. [Crossref] [PubMed]
- Lee JS, Kim SH, Jun DW, et al. Clinical implications of fatty pancreas: correlations between fatty pancreas and metabolic syndrome. World J Gastroenterol 2009;15:1869-75. [Crossref] [PubMed]
- Zyromski NJ, Mathur A, Pitt HA, et al. Obesity potentiates the growth and dissemination of pancreatic cancer. Surgery 2009;146:258-63. [Crossref] [PubMed]
- Sato H, Carvalho G, Sato T, et al. The association of preoperative glycemic control, intraoperative insulin sensitivity, and outcomes after cardiac surgery. J Clin Endocrinol Metab 2010;95:4338-44. [Crossref] [PubMed]
- Nakajima T, Ikuta S, Fujikawa M, et al. High hand grip strength is a significant risk factor and a useful predictor of postoperative pancreatic fistula following pancreaticoduodenectomy. Langenbecks Arch Surg 2024;409:85. [Crossref] [PubMed]
- Angrisani M, Sandini M, Cereda M, et al. Preoperative adiposity at bioimpedance vector analysis improves the ability of Fistula Risk Score (FRS) in predicting pancreatic fistula after pancreatoduodenectomy. Pancreatology 2020;20:545-50. [Crossref] [PubMed]
- Ecker BL, McMillan MT, Allegrini V, et al. Risk Factors and Mitigation Strategies for Pancreatic Fistula After Distal Pancreatectomy: Analysis of 2026 Resections From the International, Multi-institutional Distal Pancreatectomy Study Group. Ann Surg 2019;269:143-9. [Crossref] [PubMed]
- Bonsdorff A, Ghorbani P, Helanterä I, et al. Development and external validation of DISPAIR fistula risk score for clinically relevant postoperative pancreatic fistula risk after distal pancreatectomy. Br J Surg 2022;109:1131-9. [Crossref] [PubMed]
(English Language Editor: J. Jones)