Prognostic prediction of breast cancer patients using machine learning models: a retrospective analysis
Highlight box
Key findings
• This study developed and evaluated machine learning models to predict breast cancer prognosis using data from 6,477 patients. Among the models tested, Extreme Gradient Boosting (XGBoost) demonstrated superior performance with a receiver operating characteristic area under the curve of 0.813, accuracy of 0.739, sensitivity of 0.815, and specificity of 0.735. Significant prognostic factors identified include age, tumor size, lymph node status, and hormone receptor status.
What is known and what is new?
• Traditional prognostic models such as the Nottingham Prognostic Index and Predict Breast provide valuable insights but have limitations in prediction accuracy and personalization.
• This manuscript introduces the XGBoost algorithm, which surpasses these established models in predictive performance. It offers a more accurate and personalized tool for prognosis prediction in breast cancer, potentially leading to better-informed treatment decisions.
What is the implication, and what should change now?
• The improved predictive accuracy of the XGBoost model could significantly enhance personalized treatment strategies for breast cancer patients, leading to better outcomes.
• Further research and validation of the XGBoost model in diverse clinical settings are needed to support its integration into routine clinical practice and to optimize patient-specific treatment plans.
Introduction
Breast cancer is one of the most prevalent and significant health issues affecting women globally (1). Accurate prognostic prediction plays a crucial role in determining appropriate treatment strategies and improving patient outcomes. Traditional prognostic models, such as the Nottingham Prognostic Index (NPI) (2) and Predict Breast (3,4), have been widely used; however they may have limitations in terms of accuracy and personalized prediction (5). Accurately estimating the mortality risk for all patients diagnosed with breast cancer, regardless of stage and molecular subtype, can have important clinical implications. It may help to stratify follow-up plans, provide patients with valuable information about their prognosis, and identify high-risk individuals who might benefit from participation in clinical trials.
In recent years, machine learning models have demonstrated significant potential in various medical applications, including the prediction of breast cancer prognosis (5-7). These models excel at integrating multiple clinical features and detecting complex patterns that might pose challenges for conventional statistical methods. The potential for machine learning approaches in clinical prediction modeling has generated considerable interest within the medical community. By leveraging these advanced techniques, we hope to enhance our capacity to accurately predict breast cancer prognosis, enabling clinicians to develop personalized treatment strategies and improve patient outcomes.
Breast cancer is a multifaceted disease with a variety of prognostic factors, making accurate prediction challenging. While machine learning approaches have shown potential, it is crucial to evaluate the performance of each specific machine learning method and compare it with conventional regression-based methods, which have consistently demonstrated good performance in stratified follow-up and other clinical applications (2-4). In conclusion, while machine learning approaches hold promise, it is vital to evaluate and compare their performance with conventional regression-based methods to ensure accurate clinical decision-making.
Therefore, in this study, we aim to compare the performance of machine learning approaches with regression-based methods in predicting breast cancer prognosis using a comprehensive dataset. We leveraged the predictors available in the dataset to evaluate the effectiveness and consistency of both approaches. Through an analysis of the performance of these models, we can identify the most reliable and clinically relevant method for predicting breast cancer prognosis. We present this article in accordance with the TRIPOD reporting checklist (available at https://gs.amegroups.com/article/view/10.21037/gs-24-106/rc).
Methods
Study participants
In this retrospective study, we collected electronic medical records (EMRs) of 6,477 breast cancer patients admitted to the Department of Surgical Oncology, Affiliated Sir Run Run Shaw Hospital, Zhejiang University School of Medicine, between August 1998 and November 2021. These EMRs for each patient included a wealth of demographic and clinical feature information such as age, diagnosis date, recurrence and death dates, stage, estrogen receptor (ER as negative or positive), progesterone receptor (PR as negative or positive), human epidermal growth factor receptor 2 (HER2 ER as negative or positive), tumor size, Ki67, intravascular cancer emboli, operating time, menopausal status, histology (ductal/lobular carcinoma in situ, invasive lobular carcinoma and invasive ductal carcinoma), surgical approach and treatment. The primary outcome was the 5-year overall survival (OS), represented in binary form (1= death, 0= survival). The exclusion criteria for this study were as follows: (I) male participants; (II) received neoadjuvant chemotherapy; (III) missing records for birth, diagnosis, surgery, and follow-up date; (IV) interested feature misses more than 30%; (V) the follow-up duration of less than 5 years from the date of enrollment for the survival. This study was conducted in accordance with the Declaration of Helsinki (as revised in 2013) and was approved by Ethics Committee of the Affiliated Sir Run Run Shaw Hospital, Zhejiang University School of Medicine (No. S20210910-30). Informed consent was waived considering the retrospective nature of the study.
Feature selection and data processing
We collected 15 easily obtainable clinical features that are believed to be associated with the survival of breast cancer patients from the EMRs according to features importance (8). The percentage of missing values for all features was less than 30%. Before the model development, the categorical features were converted by using either the label or one-hot encoding method, as appropriate and the numerical variables remained unchanged. As for the missing data, we applied the following imputation strategy to deal with:
- If the missing data was a categorical feature encoded using one-hot encoding, we replaced it with zeros.
- If the missing data was a continuous feature or a categorical feature encoded using label encoding, we utilized the Multiple Imputation by Chained Equations (MICE) method with a linear regression model for imputation.
Subsequently, all features were normalized to avoid within-subject differences among features. Figure 1 shows the flow chart of the study protocol.
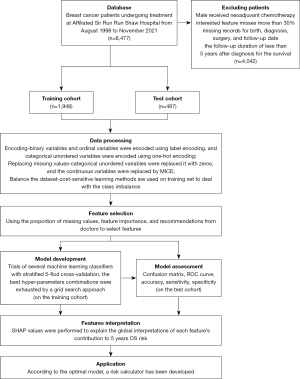
Model development and assessment
The dataset was randomly divided into a training set and a test cohort without following any sequences. To investigate the impact of train/test split ratio on the performance of prediction models, we examined five sets of ratios: 50:50, 60:40, 70:30, 80:20 and 90:10. The training set was used to develop and train models, while the test set was used to evaluate the performance of the models. Eight machine learning models were developed in this study, i.e., Logistic Regression (LR) (9), Support Vector Machine (SVM) (10), Random Forest (RF) (11), Extreme Gradient Boosting (XGBoost; XGB) (12), Multinomial Naïve Bayes (MNB) (13), K Nearest Neighbors (KNN) (14), Multi-layer Perceptron (MLP) (15) and LightGBM (LGBM) (16) which are commonly applied in medical binary classification problems and each model was supplied with the same input variables. The models were implemented utilizing Python and the Scikit-learn machine learning toolkit, and the best hyper-parameter combinations of each model were exhausted by a grid search with 5-fold cross-validation to build the optimal model.
To quantify the predictive capabilities of each model, we plotted the receiver operating characteristic (ROC) curves and then calculated the area under the curve (AUC) as the main metric to assess the model’s performance. Furthermore, the accuracy, sensitivity, and specificity, G-mean as shown in the formulas based on the confusion matrix were used to evaluate the model performance from multiple perspectives, and the Youden index was used as the threshold selection for classification. Finally, we compared the best-performing model with internationally recognized breast cancer prognostic statistical models such as the NPI and Predict Breast to assess whether machine learning methods exhibit significantly better predictive performance compared to statistical methods in the context of breast cancer prognosis.
Statistical analysis
The descriptive and continuous variables were expressed as actual numbers (n) (percentages, %), and means ± standard deviations, respectively. A Chi-squared test was used to compare the categorical variables, while Student’s t-test was used to compare the continuous variables. The P<0.05 was considered statistically significant. Data were processed and analyzed using IBM SPSS Statistics 26.0 (IBM Corp., Armonk, NY, USA).
Results
Study population characteristics
A total of 2,435 patients met the inclusion criteria for the analysis. The mean age of the participants was 51.85±0.43 years, and 136 (5.59%) and 2,299 (94.41%) of the patients died and survived within 5 years after diagnosis, respectively. A total of 15 input features were extracted from each EMR, including age, tumor location, tumor size, menstruation status, histology, number of metastatic lymph nodes, number of dissected lymph nodes, presence of intravascular tumor emboli, number of chemotherapy cycles, radiation therapy, and endocrine therapy. The characteristics of each clinical variable are shown in Table 1. It could be seen from the descriptive statistics that seven features (age, tumor size, menstruation, number of metastatic lymph nodes, intravascular tumor emboli, endocrine therapy, Ki67) were significantly different among the output classes (survival and death).
Table 1
Features | Total (N=2,435) | Survival (N=2,299) | Death (N=136) | P value |
---|---|---|---|---|
Age (years), mean ± SD | 51.85±0.43 | 51.55±0.43 | 57.05±2.45 | <0.001 |
Tumor location, n (%) | 0.68 | |||
Inner upper | 545 (22.4) | 514 (94.3) | 31 (5.7) | |
Inner lower | 107 (4.4) | 100 (93.5) | 7 (6.5) | |
Outer upper | 445 (18.3) | 420 (94.4) | 25 (5.6) | |
Outer lower | 646 (26.5) | 617 (95.5) | 29 (4.5) | |
Overlapping | 571 (23.4) | 537 (94.0) | 34 (6.0) | |
Center | 91 (3.7) | 84 (92.3) | 7 (7.7) | |
Accessory breasts | 5 (0.2) | 4 (80.0) | 1 (20.0) | |
Missing | 25 (1.0) | 23 (92.0) | 2 (8.0) | |
Tumor size (cm), mean ± SD | 2.1±0.05 | 2.12±0.05 | 2.76±0.28 | <0.001 |
Menstruation, n (%) | <0.001 | |||
Postmenopausal | 1,232 (50.6) | 1,188 (96.4) | 44 (3.6) | |
Premenopausal | 1,203 (49.4) | 1,111 (92.4) | 92 (7.6) | |
Histology, n (%) | 0.045 | |||
Invasive ductal carcinoma | 1,895 (77.8) | 1,782 (94.0) | 113 (6.0) | |
Ductal carcinoma in situ | 189 (7.8) | 185 (97.9) | 4 (2.1) | |
Mucinous carcinoma | 48 (2.0) | 48 (100.0) | 0 | |
Invasive lobular carcinoma | 70 (2.9) | 64 (91.4) | 6 (8.6) | |
Lobular carcinoma in situ | 1 (0.04) | 1 (100.0) | 0 | |
Other invasive carcinoma | 231 (9.5) | 218 (94.4) | 13 (5.6) | |
Missing | 1 (0.04) | 1 (100.0) | 0 | |
Number of metastatic lymph nodes, mean ± SD | 1.44±0.1419 | 1.25±0.1286 | 4.71±1.19 | <0.001 |
Number of dissected lymph nodes, mean ± SD | 15.87±0.3874 | 15.82±0.3974 | 16.8088±1.7253 | 0.25 |
Intravascular tumor emboli | 126 (5.2) | 111 (88.1) | 15 (11.9) | 0.002 |
Chemotherapy cycle number, mean ± SD | 4.1865±0.1816 | 4.1795±0.186 | 4.3052±0.8285 | 0.76 |
Radiation therapy, n (%) | 0.43 | |||
Yes | 1,100 (45.2) | 1,043 (94.8) | 57 (5.2) | |
No | 1,335 (54.8) | 1,256 (94.1) | 79 (5.9) | |
Endocrine therapy, n (%) | <0.001 | |||
Aromatase inhibitor | 614 (25.2) | 582 (94.8) | 32 (5.2) | |
Tamoxifen | 589 (24.2) | 567 (96.3) | 22 (3.7) | |
Toremifene | 359 (14.7) | 352 (98.1) | 7 (1.9) | |
Medical castration + aromatase inhibitor | 25 (1.0) | 23 (92.0) | 2 (8.0) | |
Medical castration + TAM | 17 (0.7) | 17 (100.0) | 0 | |
Other | 99 (4.1) | 94 (94.9) | 5 (5.1) | |
Not used | 327 (13.4) | 288 (88.1) | 39 (11.9) | |
Missing | 405 (16.6) | 376 (92.8) | 29 (7.2) | |
ER, n (%) | 0.93 | |||
Positive | 634 (26.0) | 599 (94.5) | 35 (5.5) | |
Negative | 1,801 (74.0) | 1,700 (94.4) | 101 (5.6) | |
PR, n (%) | 0.31 | |||
Positive | 702 (28.8) | 668 (95.2) | 34 (4.8) | |
Negative | 1,733 (71.2) | 1,631 (94.1) | 102 (5.9) | |
Her2, n (%) | 0.10 | |||
Positive | 639 (26.2) | 595 (93.1) | 44 (6.9) | |
Negative | 1,796 (73.8) | 1,704 (94.9) | 92 (5.1) | |
Ki67, mean ± SD | 28.69±0.81 | 28.37±0.83 | 34.23±3.64 | 0.001 |
SD, standard deviation; TAM, tamoxifen; ER, estrogen receptor; PR, progesterone receptor.
Model performance
We compared eight algorithms for breast cancer prognosis prediction. Table 2 presents the performance metrics of each model, including AUC value derived from the ROC curve (Figure 2), accuracy, sensitivity, specificity, and G-mean, across various training and testing splits. The results demonstrated that the XGBoost algorithm maintained good performance at all different ratios. Meanwhile, as anticipated, when the training set ratio was relatively small (e.g., 50:50), the performance of several machine learning algorithms improved with an increase in the training set ratio, which suggests that the models learned more effectively. However, when the training set ratio was further increased to 90%, a decrease in model performance was observed, indicating potential overfitting effects. Ultimately, the best performance was obtained by the XGBoost algorithm in the primary evaluation metric, AUC value of 0.813 on the 80:20 training/testing split, along with fairly high values in other evaluation metrics, suggesting good classification performance. However, we found that no classification model achieved the highest scores across all evaluation metrics. While the MNB model achieved the highest accuracy value of 0.848 and the highest specificity value of 0.865, it achieved the lowest sensitivity value of 0.556. Similarly, the LR model exhibited extremely high sensitivity value of 0.889, but it performed comparatively poorer in terms of accuracy and specificity metrics.
Table 2
Model | AUC | Accuracy | Sensitivity | Specificity | G-mean |
---|---|---|---|---|---|
Training/testing (50:50) | |||||
LR | 0.745 | 0.805 | 0.529 | 0.821 | 0.659 |
SVM | 0.735 | 0.811 | 0.559 | 0.826 | 0.679 |
RF | 0.781 | 0.869 | 0.515 | 0.890 | 0.677 |
XGB | 0.774 | 0.873 | 0.397 | 0.901 | 0.598 |
MNB | 0.679 | 0.715 | 0.515 | 0.727 | 0.612 |
KNN | 0.614 | 0.944 | 0.0 | 1.0 | 0.000 |
MLP | 0.703 | 0.775 | 0.471 | 0.793 | 0.611 |
LGBM | 0.755 | 0.865 | 0.382 | 0.893 | 0.584 |
Training/testing (60:40) | |||||
LR | 0.746 | 0.786 | 0.500 | 0.803 | 0.634 |
SVM | 0.767 | 0.854 | 0.444 | 0.878 | 0.625 |
RF | 0.783 | 0.801 | 0.611 | 0.812 | 0.704 |
XGB | 0.782 | 0.861 | 0.500 | 0.883 | 0.664 |
MNB | 0.661 | 0.732 | 0.500 | 0.746 | 0.611 |
KNN | 0.615 | 0.945 | 0.000 | 1.000 | 0.000 |
MLP | 0.732 | 0.805 | 0.481 | 0.824 | 0.630 |
LGBM | 0.759 | 0.858 | 0.426 | 0.884 | 0.614 |
Training/testing (70:30) | |||||
LR | 0.754 | 0.724 | 0.683 | 0.726 | 0.704 |
SVM | 0.772 | 0.654 | 0.756 | 0.648 | 0.700 |
RF | 0.786 | 0.837 | 0.659 | 0.848 | 0.747 |
XGB | 0.778 | 0.806 | 0.634 | 0.816 | 0.719 |
MNB | 0.718 | 0.686 | 0.659 | 0.688 | 0.673 |
KNN | 0.652 | 0.725 | 0.585 | 0.733 | 0.655 |
MLP | 0.759 | 0.748 | 0.683 | 0.752 | 0.717 |
LGBM | 0.777 | 0.713 | 0.780 | 0.709 | 0.744 |
Training/testing (80:20) | |||||
LR | 0.789 | 0.643 | 0.889 | 0.628 | 0.747 |
SVM | 0.795 | 0.741 | 0.815 | 0.737 | 0.775 |
RF | 0.809 | 0.745 | 0.815 | 0.741 | 0.777 |
XGB | 0.813 | 0.739 | 0.815 | 0.735 | 0.774 |
MNB | 0.758 | 0.848 | 0.556 | 0.865 | 0.693 |
KNN | 0.751 | 0.741 | 0.778 | 0.739 | 0.758 |
MLP | 0.746 | 0.694 | 0.703 | 0.693 | 0.699 |
LGBM | 0.786 | 0.649 | 0.852 | 0.637 | 0.737 |
Training/testing (90:10) | |||||
LR | 0.776 | 0.664 | 0.786 | 0.657 | 0.718 |
SVM | 0.806 | 0.615 | 1.0 | 0.591 | 0.769 |
RF | 0.803 | 0.770 | 0.857 | 0.765 | 0.810 |
XGB | 0.781 | 0.758 | 0.857 | 0.752 | 0.803 |
MNB | 0.774 | 0.861 | 0.643 | 0.874 | 0.750 |
KNN | 0.756 | 0.734 | 0.857 | 0.726 | 0.789 |
MLP | 0.788 | 0.664 | 0.857 | 0.652 | 0.748 |
LGBM | 0.789 | 0.803 | 0.714 | 0.809 | 0.760 |
AUC, area under the curve; LR, Logistic Regression; SVM, Support Vector Machine; RF, Random Forest; XGB, Extreme Gradient Boosting; MNB, Multinomial Naïve Bayes; KNN, K-Nearest Neighbors; MLP, Multi-Layer Perceptron; LGBM, Light Gradient Boosting Machine.
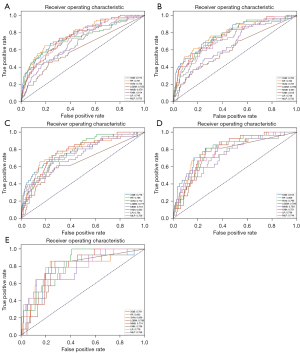
Model explanations
In this research, we utilized the Shapley Additive Explanations (SHAP) tool to reveal the individual contributions of each feature to the model predictions which is useful in model interpretation. Figure 3A presents the feature importance plot for the XGB model, in order of importance based on the average absolute SHAP values. The beeswarm plot depicted in Figure 3B describes the specific impact of each feature on the prediction of the 5-year mortality risk. Each dot represents the SHAP value of each feature for all individual patients, with the colors ranging from blue (low feature value) to red (high feature value). These points are distributed relative to a vertical line at zero, where all the feature values on the left side of zero exert a negative effect on clinical outcomes, while the feature values on the right side exert a positive effect on clinical outcomes. The features on the right indicated by red dots are positively correlated with outcomes, while the features indicated by blue dots are negatively correlated with outcomes.
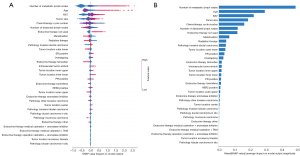
Number of metastatic lymph nodes, age and Ki67 were determined to be the four most important features with the highest SHAP values (0.483, 0.289 and 0.220, respectively; Figure 2A), followed by tumor size, chemotherapy cycle number, number of dissected lymph nodes, endocrine therapy not used, menstruation, radiation therapy, pathology invasive ductal carcinoma, tumor location outer lower, ER positive and overlapping and so on. Furthermore, as indicated in Figure 2B, the higher number of metastatic lymph nodes, older age, higher Ki67 value, larger tumor size, and not using endocrine therapy were associated with an increased risk of mortality within 5 years for breast cancer patients, while the greater number of dissected lymph nodes, higher chemotherapy cycle number, menopause and radiation therapy can reduce the risk of death. Although the SHAP value distribution was highly dispersed, the correlations of the features with 5-year OS remained consistent with domain knowledge of most of the features.
Model comparison
Due to a high proportion of missing data in the Histologic Tumor Grading within the EMRs used in this study, this feature was not included in the constructed model. However, the NPI and Predict Breast models incorporate this feature. In order to facilitate a meaningful comparison, we selected the subset (n=206, survival =193, death =13) of patient samples from the test set that presented with available Histologic Tumor Grading value to compare the performance of the best-performing XGB model with the NPI and Predict Breast models. The NPI was calculated using the formula NPI = (0.2 × S) + N + G, where S denotes tumor size, N denotes lymph node stage, and G denotes histological grade. On the other hand, the Predict Breast model’s prediction was calculated based on its openly available source code and risk coefficients. The ROC curves and AUC values for the three models are depicted in Figure 4. The results indicated that the XGB model achieved the best predictive performance (AUC =0.733), followed by NPI (AUC =0.637), and Predict Breast performed the least accurately (AUC =0.592).
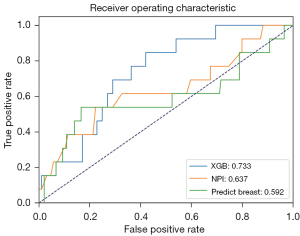
Model application
Based on the XGB model, we have developed a postoperative prognosis prediction tool for breast cancer patients, as shown in Figure 5, which can be accessed via https://t54e757334.vicp.fun/tool. By inputting the patient’s clinical features and treatment regimen, this tool can assist doctors in gaining a better understanding of the patient’s disease progression, which facilitates precision treatment for breast cancer, allowing for more targeted and efficient interventions.
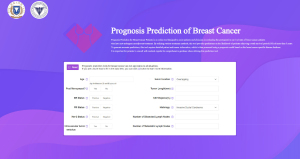
Discussion
The study focused on developing machine learning models for predicting prognosis in breast cancer patients. Eight different machine learning methods were employed and evaluated to forecast the survival of patient. The study used the AUC value, accuracy, sensitivity, and specificity to evaluate the predictive performance of the model. The AUC value was used as the main indicator for overall classification performance, regardless of how the classification threshold was set. The accuracy was used to evaluate the classification accuracy of the model in the overall sample. However, on our imbalanced data, the model may tend to predict the majority of categories, resulting in high accuracy and poor actual performance. The sensitivity measured the modell’s ability to recognize positive examples, while specificity indicators measure the model’s ability to recognize negative examples. Analysis based on the AUC values indicates that multiple machine learning methods exhibit strong predictive performance in forecasting the survival rates of breast cancer within the test dataset. Notably, the XGBoost model outperformed the other seven algorithms, boasting an impressive AUC of 0.813 on the 80:20 training/testing radio. While its performance may vary in other metrics, including accuracy (0.739), sensitivity (0.815), and specificity (0.735), it demonstrated superior overall efficacy, which allows it to balance positive and negative samples well. This highlights the XGBoost model’s robust discriminatory ability in identifying patients with varying prognoses. Most importantly, based on the XGBoost model with the highest AUC value, we can modify its threshold to adapt to different prediction needs, such as increasing the threshold to improve the recognition ability for negative cases, i.e., increasing specificity, or decreasing the threshold to improving the recognition ability for positive cases, i.e., improving sensitivity.
In contrast to prior studies (17-22) focusing solely on pathological characteristics, our research utilized a comprehensive dataset spanning a broader timeframe, representing the Chinese population. Notably, age, tumor size, lymph node status, and hormone receptor status were identified as key predictors in our analysis. These findings align with established knowledge in the field, emphasizing the importance of these factors in breast cancer prognosis, and also validating the reliability of our model (8). Furthermore, treatment modalities such as the number of dissected lymph node, postoperative chemotherapy, and endocrine therapy were factors that had a higher impact on patient survival compared to some pathological features, indicating the importance of treatment.
Previous research has shown that machine learning approaches do not necessarily outperform appropriate statistical models in low-dimensional clinical settings (23). Considering the potential risks associated with suboptimal medical decisions, thorough evaluation of clinical prediction models is essential to assess their performance and utility (24). Efforts should focus on enhancing generalizability through multi-center collaborations and ensuring transparency by incorporating model explanations in clinical applications (25).
Evaluation against established prognostic models like the NPI (2) and Predict Breast (3,4) demonstrated the superior efficacy of our machine learning model. This highlights the potential of machine learning algorithms to improve prognostic prediction accuracy in breast cancer patients. Classical methods are often faced with issues such as collinearity, heteroscedasticity, complex interactions between variables, and higher-order interactions between predictors, all of which machine learning approaches can effectively overcome.
The development of a postoperative prognosis prediction tool based on the XGBoost model represents a significant advancement in personalized treatment decision-making. Clinicians can leverage this tool to gain valuable insights into the progression of diseases and guide tailored treatment strategies, ultimately improving patient outcomes and optimizing the allocation of resources in healthcare settings.
Despite yielding promising results, it is important to acknowledge certain limitations. Firstly, this study utilized retrospective data from a singular institution, potentially introducing biases. Future studies should consider multi-center collaborations to enhance generalizability. Additionally, although machine learning models provide accurate predictions, their complex nature can limit interpretability. Efforts should focus on incorporating model explanations and ensuring transparency in clinical applications.
Conclusions
In conclusion, our study demonstrates the potential of machine learning models, particularly XGBoost, in providing precise prognostic predictions for breast cancer patients. By incorporating easily obtainable clinical features, these models can provide valuable insights to clinicians, assisting in personalized treatment decision-making and ultimately improving patient outcomes. Further research and validation are warranted to fully integrate these models into clinical practice.
Acknowledgments
Funding: This work was supported by
Footnote
Reporting Checklist: The authors have completed the TRIPOD reporting checklist. Available at https://gs.amegroups.com/article/view/10.21037/gs-24-106/rc
Data Sharing Statement: Available at https://gs.amegroups.com/article/view/10.21037/gs-24-106/dss
Peer Review File: Available at https://gs.amegroups.com/article/view/10.21037/gs-24-106/prf
Conflicts of Interest: All authors have completed the ICMJE uniform disclosure form (available at https://gs.amegroups.com/article/view/10.21037/gs-24-106/coif). The authors have no conflicts of interest to declare.
Ethical Statement: The authors are accountable for all aspects of the work in ensuring that questions related to the accuracy or integrity of any part of the work are appropriately investigated and resolved. This study was conducted in accordance with the Declaration of Helsinki (as revised in 2013) and was approved by Ethics Committee of the Affiliated Sir Run Run Shaw Hospital, Zhejiang University School of Medicine (No. S20210910-30). Informed consent was waived considering the retrospective nature of the study.
Open Access Statement: This is an Open Access article distributed in accordance with the Creative Commons Attribution-NonCommercial-NoDerivs 4.0 International License (CC BY-NC-ND 4.0), which permits the non-commercial replication and distribution of the article with the strict proviso that no changes or edits are made and the original work is properly cited (including links to both the formal publication through the relevant DOI and the license). See: https://creativecommons.org/licenses/by-nc-nd/4.0/.
References
- Wilkinson L, Gathani T. Understanding breast cancer as a global health concern. Br J Radiol 2022;95:20211033. [Crossref] [PubMed]
- Gray E, Donten A, Payne K, et al. Survival estimates stratified by the Nottingham Prognostic Index for early breast cancer: a systematic review and meta-analysis of observational studies. Syst Rev 2018;7:142. [Crossref] [PubMed]
- Wishart GC, Bajdik CD, Dicks E, et al. PREDICT Plus: development and validation of a prognostic model for early breast cancer that includes HER2. Br J Cancer 2012;107:800-7. [Crossref] [PubMed]
- Wishart GC, Azzato EM, Greenberg DC, et al. PREDICT: a new UK prognostic model that predicts survival following surgery for invasive breast cancer. Breast Cancer Res 2010;12:R1. [Crossref] [PubMed]
- Clift AK, Dodwell D, Lord S, et al. Development and internal-external validation of statistical and machine learning models for breast cancer prognostication: cohort study. BMJ 2023;381:e073800. [Crossref] [PubMed]
- Cutillo CM, Sharma KR, Foschini L, et al. Machine intelligence in healthcare-perspectives on trustworthiness, explainability, usability, and transparency. NPJ Digit Med 2020;3:47. [Crossref] [PubMed]
- Alaa AM, Gurdasani D, Harris AL, et al. Machine learning to guide the use of adjuvant therapies for breast cancer. Nat Mach Intell 2021;3:716-26. [Crossref]
- Coates AS, Winer EP, Goldhirsch A, et al. Tailoring therapies--improving the management of early breast cancer: St Gallen International Expert Consensus on the Primary Therapy of Early Breast Cancer 2015. Ann Oncol 2015;26:1533-46. [Crossref] [PubMed]
- Elkahwagy DMAS, Kiriacos CJ, Mansour M. Logistic regression and other statistical tools in diagnostic biomarker studies. Clin Transl Oncol 2024;26:2172-80. [Crossref] [PubMed]
- Medjahed SA, Boukhatem F. Applying Support Vector Machines with Different Kernel to Breast Cancer Diagnosis. Computación y Sistemas 2024;28:659-67. [Crossref]
- Dinesh P, Vickram AS, Kalyanasundaram P. Medical Image Prediction for Diagnosis of Breast Cancer Disease Comparing the Machine Learning Algorithms: SVM, KNN, Logistic Regression, Random Forest, and Decision Tree to Measure Accuracy. AIP Conf Proc 2024;2853:020140. AIP Publishing.
- Hoque R, Das S, Hoque M, et al. Breast Cancer Classification using XGBoost. World Journal of Advanced Research and Reviews. 2024;21:1985-94. [Crossref]
- Abnoosian K, Farnoosh R, Behzadi MH. Prediction of diabetes disease using an ensemble of machine learning multi-classifier models. BMC Bioinformatics 2023;24:337. [Crossref] [PubMed]
- Assegie TA, Suresh T, Purushothaman R, et al. Early prediction of gestational diabetes with parameter-tuned K-Nearest Neighbor Classifier. Journal of Robotics and Control 2023;4:452-7. (JRC). [Crossref]
- Safar AA, Salih DM, Murshid AM. Pattern recognition using the multi-layer perceptron (MLP) for medical disease: A survey. International Journal of Nonlinear Analysis and Applications 2023;14:1989-98.
- Yang H, Chen Z, Yang H, et al. Predicting coronary heart disease using an improved LightGBM model: Performance analysis and comparison. IEEE Access 2023;11:23366-80.
- Boeri C, Chiappa C, Galli F, et al. Machine Learning techniques in breast cancer prognosis prediction: A primary evaluation. Cancer Med 2020;9:3234-43. [Crossref] [PubMed]
- Ferroni P, Zanzotto FM, Riondino S, et al. Breast Cancer Prognosis Using a Machine Learning Approach. Cancers (Basel) 2019;11:328. [Crossref] [PubMed]
- Tapak L, Shirmohammadi-Khorram N, Amini P, et al. Prediction of survival and metastasis in breast cancer patients using machine learning classifiers. Clin Epidemiol Glob Heal 2019;7:293-9. [Crossref]
- Mihaylov I, Nisheva M, Vassilev D. Application of Machine Learning Models for Survival Prognosis in Breast Cancer Studies. Information 2019;10:93. [Crossref]
- Naji MA, Filali S, El , Aarika K. Bet al. Machine Learning Algorithms For Breast Cancer Prediction And Diagnosis. Procedia Comput Sci 2021;191:487-92. [Crossref]
- Kalafi EY, Nor NAM, Taib NA, et al. Machine Learning and Deep Learning Approaches in Breast Cancer Survival Prediction Using Clinical Data. Folia Biol (Praha) 2019;65:212-20. [Crossref] [PubMed]
- Christodoulou E, Ma J, Collins GS, et al. A systematic review shows no performance benefit of machine learning over logistic regression for clinical prediction models. J Clin Epidemiol 2019;110:12-22. [Crossref] [PubMed]
- Van Calster B, Wynants L, Timmerman D, et al. Predictive analytics in health care: how can we know it works? J Am Med Inform Assoc 2019;26:1651-4. [Crossref] [PubMed]
- Riley RD, Ensor J, Snell KI, et al. External validation of clinical prediction models using big datasets from e-health records or IPD meta-analysis: opportunities and challenges. BMJ 2016;353:i3140. [Crossref] [PubMed]