Radiomics and deep learning for large volume lymph node metastasis in papillary thyroid carcinoma
Highlight box
Key findings
• The Thy-deep learning (DL)-Radiomics model was developed to predict large volume lymph node metastasis (LVLNM) in patients with papillary thyroid carcinoma (PTC), demonstrating an area under the curve of 0.839 in internal validation and 0.789 in external validation. By integrating radiomics and DL features, this model provides personalized diagnostic and therapeutic insights for PTC patients.
What is known and what is new?
• Current knowledge encompasses the use of ultrasound imaging and clinical features to predict lymph node metastasis (LNM), though the accuracy (ACC) of these methods requires enhancement.
• The innovative Thy-DL-Radiomics model signifies a significant advancement by integrating radiomics and DL techniques to improve the risk assessment of LNM in patients with PTC.
What is the implication, and what should change now?
• The implications of the Thy-DL-Radiomics model include the enhanced identification of high-risk LVLNM patients, which could potentially inform personalized treatment strategies and improve patient outcomes. It is recommended that clinicians incorporate advanced imaging and machine learning models into clinical practice to enhance the ACC of preoperative assessments in PTC.
Introduction
Thyroid cancer is the fastest-growing endocrine malignancy globally, with papillary thyroid carcinoma (PTC) accounting for over 90% of cases (1). Upon diagnosis, 25–80% of PTC patients already exhibit lymph node metastasis (LNM) in the neck, even when tumor diameters are ≤1 cm, with a metastatic rate of 12–64% (2-4). Although PTC generally has a favorable prognosis, with a 10-year survival rate exceeding 88%, cervical LNM significantly increases the risk of recurrence and mortality (5). Specifically, if more than five lymph nodes are involved, the postoperative recurrence rate exceeds 30% (6). The American Thyroid Association (ATA) guidelines stratify the risk of recurrence and suggest that PTC patients with more than five metastatic lymph nodes are at moderate or high recurrence risk, indicating a preoperative inclination towards proactive preventative lymph node dissection (7). Previous research has reported that when the number of metastatic lymph nodes exceeds five, it is typically defined as large volume LNM (LVLNM) (8). Currently, lymph node dissection is indisputably required for patients with moderate- or high-risk of recurrence, but the necessity of prophylactic dissection for patients with a low-risk of recurrence remains controversial. Recent opinions suggest that active surveillance (AS) could replace surgical treatment for low-risk PTC to avoid unnecessary harm from overtreatment (9). Therefore, accurate preoperative assessment of LNM is crucial.
Ultrasound is commonly used preoperatively to assess the presence of LNM in PTC (10). However, due to the deep location and obstruction by the surrounding tissue in the central neck region, the sensitivity (SEN) of ultrasound to detect central LNM is low, ranging from only 31% to 35% (11). Computed tomography (CT), with its higher spatial resolution, can clearly display suspicious metastatic lymph nodes and their relationship with surrounding structures, with a SEN of 47–63% and specificity (SPE) of 90–95% (12). However, the evaluation of cervical LNM by radiologists is time-consuming and subjective, often depending on the radiologist’s experience and imaging equipment. In recent years, radiomics has garnered significant attention in precise diagnosis. Li et al. proposed a radiomics-based approach predicting LNM in PTC patients by converting ultrasound image features such as intensity, boundary, texture, and wavelet into extractable data (13). Concurrently, deep learning (DL), a new scientific technology, has been used in medical imaging research to overcome limitations in manual image analysis and information retrieval.
This study aims to develop a combined DL-Radiomics model to enhance the preoperative prediction of LVLNM in patients with PTC, striving to provide more precise individualized diagnostic and therapeutic plans. By integrating the strengths of radiomics and DL, we expect this model to improve the accuracy (ACC) of LNM quantification, thus aiding in better risk stratification and treatment decisions for PTC patients. We present this article in accordance with the TRIPOD reporting checklist (available at https://gs.amegroups.com/article/view/10.21037/gs-24-308/rc).
Methods
Patients
This study collected data from patients with PTC who underwent surgery at Hangzhou Hospital of Traditional Chinese Medicine, Cancer Hospital of the University of Chinese Academy of Sciences, Affiliated Yantai Yuhuangding Hospital, Qingdao University from January 2023 to December 2023. The data were divided into a training set, an internal validation set, and an external validation set. The inclusion criteria were as follows: (I) pathological confirmation of PTC; (II) patients who underwent primary surgery accompanied by cervical lymph node dissection; (III) postoperative pathological reports including detailed information on the number of lymph nodes dissected and the number of metastatic lymph nodes; and (IV) availability of comprehensive preoperative thyroid ultrasound images for analysis. The exclusion criteria were as follows: (I) postoperative pathological diagnosis indicating sub-types of PTC; (II) history of neck trauma, previous tumor surgery, or adjuvant chemoradiotherapy; and (III) fewer than three lymph nodes dissected during surgery. The surgical procedures were listed in Appendix 1. The study was conducted in accordance with the Declaration of Helsinki (as revised in 2013). The study was approved by the Ethics Committee of Hangzhou Hospital of Traditional Chinese Medicine (No. 2022KY153-CX1). All participating hospitals were informed and agreed with this study. Individual consent for this retrospective analysis was waived.
Imaging acquisition and thyroid nodules segmentation
A color Doppler ultrasound machine equipped with a real-time, high-frequency (5–12 MHz) linear-array probe was used in this study. The ultrasound image data was collected by two ultrasound radiologists with 5 years of thyroid ultrasound experience. The images of the thyroid nodules were stored in JPG format. The regions of interest (ROIs) of the PTCs were manually delineated using ITK-SNAP (version 3.6.0). A radiologist with 10 years of experience outlined the ROI borders for the thyroid nodules.
Radiomics feature extraction and selection
The data extracted included first-order features, intensity histogram statistics, shape and size statistics, and (filtered) texture features. All handcrafted features were extracted using an in-house feature analysis program implemented in Pyradiomics (http://pyradiomics.readthedocs.io). The explanation was listed in Appendix 2. The least absolute shrinkage and selection operator (LASSO) regression model was applied to the training dataset for signature construction. LASSO shrinks all regression coefficients towards zero and sets the coefficients of many irrelevant features to zero depending on the regulation weight λ. To find the optimal λ, a 5-fold cross-validation with minimum criteria was employed, wherein the final value of λ yielded the minimum cross-validation error. The retained features with nonzero coefficients were used for fitting the regression model and were combined into a radiomics feature.
The construction of a DL model and DL feature extraction
In thyroid ultrasound images, each image has a corresponding mask image containing the cancerous region, where the pixels of the cancerous area are set to 255, and other non-cancerous areas are set to 0. We extracted the ROI of the nodule area based on the image mask, defining the minimum bounding rectangle. We utilized two-dimensional (2D) ultrasound grayscale images as input. The model output was the probability of LVLNM in PTC. The training parameters are presented in Appendix 3. To enhance the network’s generalization ability, random data transformations were applied during training batches based on different task characteristics. This allowed the network to learn geometric invariance, such as size changes, rotations, affine transformations, contrast variations, noise interference, to reduce variations arising from the diverse physical properties of different ultrasound devices. We derived a set of DL features from the previously described pre-trained DL model. Initially, each image in the dataset underwent processing through the model, and the output activations of the second-to-last fully-connected neural network (FCNN) layer were retained and utilized as the DL features of the images. Principal component analysis (PCA) was conducted to further reduce the dimensionality of DL features to 128. We utilized the Gradient-weighted Class Activation Map (Grad-CAM) algorithm to visualize heatmaps that highlight the image regions contributing most to the model prediction. Specifically, we applied Grad-CAM to the final convolutional layer in our model to generate heatmaps with appropriate spatial resolution (14).
Combined radiomics and DL features
To construct the integrated DL-Radiomics model, DL features were merged with the radiomics features to create a combined set of DL-radiomics features for each image. For classifier selection, the development dataset was split into training dataset and internal validation dataset portions for the examination and evaluation of seven different classifiers: logistic regression (LR), support vector machine (SVM), K-nearest neighbors (KNN), multi-layer perceptron (MLP), random forest (RF), ExtraTrees, extreme gradient boosting (XGBoost), and light gradient boosting machine (LightGBM). The machine learning (ML) classifier demonstrated the highest area under the curve (AUC) in the highest average and lowest standard deviation (SD) across 5-fold cross-validations, leading to its adoption for the optimal model development.
Statistical analysis
By employing descriptive statistics, mean values and SDs characterized the ages and sizes of the nodules. The classification outcomes from each method were detailed through both absolute quantities and percentages relative to the gold standard. The statistical evaluation each model regarding the gold standard included metrics such as AUC, ACC, SEN, SPE, positive predictive value (PPV), negative predictive value (NPV), and F1-score to assess result consistency. Statistical tests for the κ coefficient across different groups were performed, and group comparisons utilized the χ2 test, alongside the analysis of receiver operating characteristic (ROC) curves. DeLong tests were conducted to compare the AUCs. For statistical significance, a P value <0.05 was considered. R software version 4.0.2 (R Foundation for Statistical Computing, Vienna, Austria) was utilized for all statistical analyses. The statistical analyses were executed using R software (version 4.3.1).
Results
Patient cohort
This study enrolled 854 patients diagnosed with PTC, comprising 212 males and 642 females, with an age distribution of 45.89±12.19 years, ranging from 18 to 85 years. Of the 854 PTC patients, there were 414 patients without LNM, 340 patients with LNM but less than 5, and 120 patients with LVLNM. These patients were segregated into three cohorts: a training set (n=350), internal validation set (n=150), and external validation set (n=374). Figures 1,2 illustrate the study’s flow diagram. Clinical data are detailed in Table 1.
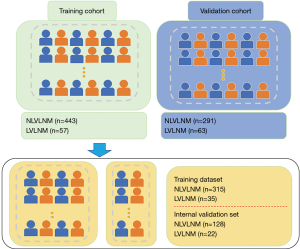
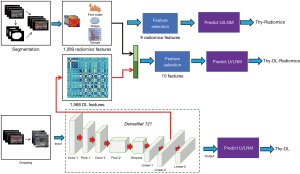
Table 1
Characteristics | Training set (n=350) | Internal validation set (n=150) | External verification set (n=374) | |||||
---|---|---|---|---|---|---|---|---|
NLVLNM | LVLNM | NLVLNM | LVLNM | NLVLNM | LVLNM | |||
Sex | ||||||||
Female | 246 | 21 | 96 | 16 | 222 | 41 | ||
Male | 69 | 14 | 32 | 6 | 69 | 22 | ||
Age (years) | 42.11±15.34 | 47.32±9.27 | 41.61±14.54 | 39.45±9.35 | 46.31±12.51 | 42.11±13.21 | ||
Size (mm) | 7.36±3.26 | 12.63±5.38 | 9.38±4.25 | 12.35±3.51 | 8.96±5.13 | 13.43±4.67 | ||
Multifocality | ||||||||
No | 200 | 17 | 50 | 15 | 227 | 45 | ||
Yes | 115 | 18 | 78 | 7 | 64 | 18 | ||
Capsular invasion | ||||||||
No | 263 | 5 | 84 | 4 | 141 | 9 | ||
Yes | 52 | 30 | 44 | 18 | 150 | 54 |
Data are presented as number or mean ± SD. PTC, papillary thyroid carcinoma; NLVLNM, non-large volume lymph node metastasis; LVLNM, large volume lymph node metastasis; SD, standard deviation.
Radiomics features selection and radiomics model building
A total of seven categories and 1,357 radiomics features were extracted, including 374 first-order, 14 shape, and 969 texture features. We included 1–3 representative ultrasound images for each patient without limitation of transverse and longitudinal cuts, and satisfactory reproducibility of radiomics feature extraction was achieved. LASSO was employed in the training cohort to determine the optimal regulation weight, and 9 RFs were chosen for predicting LVLNM (Figure 3A). The Thy-Radiomics model was obtained by ExtraTrees algorithm compared with eight classical ML model classifiers (Table 2). Figure 3B shows the AUC of each ML model on the internal validation set cohort of the Thy-Radiomics model. The Thy-Radiomics model achieved the best value of AUC reaching 0.787 [95% confidence interval (CI): 0.715–0.858] for predicting the LVLNM by ExtraTrees. The ACC, SEN, SPE, PPV, NPV, and F1-score of the ExtraTrees model were 0.718, 0.738, 0.714, 0.33, 0.935, and 0.456 respectively (Figure 3C). Then, we conducted a five random cross-validation of the internal dataset (Table S1).
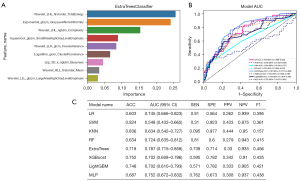
Table 2
Model name | ACC | AUC (95% CI) | SEN | SPE | PPV | NPV | F1 |
---|---|---|---|---|---|---|---|
AlexNet | 0.641 | 0.735 (0.652–0.818) | 0.738 | 0.623 | 0.272 | 0.738 | 0.397 |
DenseNet121 | 0.721 | 0.766 (0.683–0.848) | 0.714 | 0.723 | 0.33 | 0.714 | 0.451 |
Inception_v3 | 0.34 | 0.492 (0.411–0.572) | 0.857 | 0.241 | 0.177 | 0.857 | 0.294 |
ResNet50 | 0.676 | 0.686 (0.600–0.772) | 0.619 | 0.686 | 0.274 | 0.619 | 0.38 |
ViT | 0.794 | 0.614 (0.528–0.700) | 0.19 | 0.909 | 0.286 | 0.19 | 0.229 |
DL, deep learning; ACC, accuracy; AUC, area under the curve; CI, confidence interval; SEN, sensitivity; SPE, specificity; PPV, positive predictive value; NPV, negative predictive value; F1, F1-score; ViT, vision transformer.
DL model building and DL features selection
We further tried five DL models to assess the risk of LVLNM in PTC, including AlexNet, DenseNet121, inception_v3, ResNet50, and transformer algorithm to select the optimal DL model. Table 2 shows the AUC of performance of DL model. The Thy-DL model by DenseNet121 showed the best result with an AUC of 0.766 (95% CI: 0.683–0.848). The ACC, SEN, SPE, PPV, NPV, and F1-score of the DenseNet121 model were 0.721, 0.714, 0.723, 0.33, 0.714, and 0.451, respectively, in predicting LVLNM (Table 2). We further extracted the DenseNet121 model of DL features, in which 1,568 DL features were extracted. The dimensionality of DL features was reduced to 128 features by PCA.
Combined model and performance evaluation
We further integrated the aforementioned RFs and DL features. After a screening process, we selected seven traditional radiomics features and three DL features to predict LVLNM (Figure 4). We constructed a combined model, named the Thy-DL-Radiomics model, for which the internal validation set results showed an AUC of 0.839 (95% CI: 0.758–0.920), and the ACC, SEN, SPE, PPV, NPV, and F1-score of MLP were 0.727, 0.8, 0.719, 0.24, 0.97, and 0.369, respectively. To assess the performance of the Thy-DL-Radiomics model on an external test set, we included PTC patients as an external validation set, which yielded an AUC of 0.789 (95% CI: 0.718–0.859). The ACC, SEN, SPE, PPV, NPV, and F1-score of the Thy-DL-Radiomics model were 0.658, 0.833, 0.624, 0.297, 0.952, and 0.437, respectively, in predicting LVLNM. Additionally, the DeLong test results indicated that the Thy-DL-Radiomics model in the internal validation set showed better performance in predicting LVLNM (P<0.05, Figure 5).
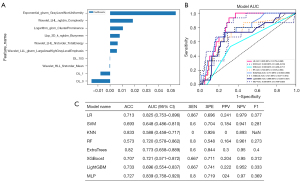
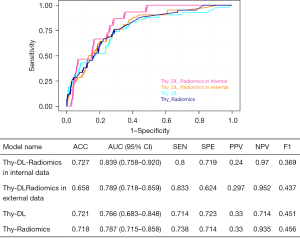
Model interpretability
We extracted visual inspection information of images processed in the final convolutional layer of the DL algorithm, showcasing the capability to handle ultrasound imaging data in diverse ways. Simultaneously, we characterized the distinct features of diverse imaging radiomics, as shown in Figure 6.
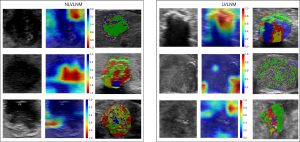
Discussion
This study developed a new preoperative method for assessing LVLNM based on PTC ultrasound. By analyzing ultrasound radiomics features and DL algorithms, we successfully constructed a model combining radiomics features and DL features to predict LVLNM. Our results demonstrate the feasibility of predicting LNM in PTC through the fusion of convolutional neural network (CNN) algorithms and radiomics features.
The literature reports that qualitative ultrasound features extracted from PTC primary lesions in ultrasound images (such as tumor size, location, and echogenicity) achieved an ACC of 71.2% (15). Wu et al. used ultrasound images to evaluate LNM in PTC patients, and concluded that tumor size, blood flow, and Hashimoto’s thyroiditis were independent factors for LNM (16). Nie et al. found that tumor size, tumor invasiveness, and tumor location were significantly associated with LNM in the univariate analysis (17). This confirmed the value of using ultrasound image features of primary lesions to predict LNM. In this study, radiomics features, imperceptible to the naked eye, were extracted, revealing clinical information that might evade detection by clinicians. Various ML algorithms were further employed to reduce feature complexity and select the optimal feature combinations (18). Unlike traditional methods, DL as a data-driven end-to-end learning approach typically does not require preprocessing. Its training process inherently involves feature extraction and optimal selection, eliminating the need for classifier selection. With the advancement of hardware-like graphic processors, DL excels in handling big data, enabling rapid processing and computation. Additionally, DL can automatically extract high-dimensional, high-level features, superior to traditionally designed features, and does not require subsequent selection (19). This study utilized 1,568 DL features to evaluate the risk of LVLNM metastasis, offering a more accurate and objective approach to image feature extraction as compared to human observation, reducing inter-observer variability and saving substantial manpower.
The Thyroid Imaging Reporting and Data System (TI-RADS) scoring system based on different ultrasound characteristics such as calcification, echo patterns, and margins, was developed to assess the malignancy of thyroid nodules (20). However, radiomics features, as high-dimensional features, lack interpretability (21). This study identified nine radiomics features closely associated with LVLNM using ML algorithms. Therefore, we introduced a new habitat analysis technique. By characterizing the differential features between the LVLNM group and the non-LVLNM (NLVLNM) group, the optimal category was identified through cluster analysis (22). Visual analysis of the results indicated that calcification in hypoechoic regions was correlated with LVLNM, consistent with previous research (23). DL, being a black-box model, also faces challenges with interpretability. Grad-CAM weights and integrates gradients of the neural network’s output to visualize the model’s focus on specific regions and crucial predictive information, aiding in the understanding and interpretation of the model’s operational principles (24). Jia et al. found that the GAFM-HAIbrid model may help identify novel diagnosis-relevant second-order features beyond ultrasonography to assess the malignancy of thyroid nodules (25). Further selection of DL features related to LVLNM using DL algorithms requires future research.
This study also has certain limitations. Firstly, to meet the demands of large sample sizes required for DL, it is necessary to further incorporate samples from multiple centers to increase the sample size, assess the model’s external generalization ability, and further enhance the model’s robustness. This study included 1–3 ultrasound images from different planes to evaluate LVLNM. In future research, it is essential to investigate the impact of the quantity of ultrasound images and planes on the results. The LNM of PTC is complex; this study was limited to the presence of LVLNM in lymph nodes. Further exploration is needed regarding the location, region, and quantity of LNM to meet precise clinical requirements.
Conclusions
A combined Thy-DL-Radiomics model utilizing thyroid ultrasound images surpasses the performance of standalone DL or radiomics models in predicting LVLNM in PTC patients, serving as an effective screening tool potentially informing decisions about surgery administration. These AI models hold promise for providing noninvasive and convenient preoperative guidance. However, despite their potential, the clinical utility of such screening models is currently limited, necessitating further research to enhance their ACC and generalizability.
Acknowledgments
Some of our experiments were carried out on the OnekeyAI platform. We thank OnekeyAI and their developers’ help in this scientific research work.
Funding: This study was funded by
Footnote
Reporting Checklist: The authors have completed the TRIPOD reporting checklist. Available at https://gs.amegroups.com/article/view/10.21037/gs-24-308/rc
Data Sharing Statement: Available at https://gs.amegroups.com/article/view/10.21037/gs-24-308/dss
Peer Review File: Available at https://gs.amegroups.com/article/view/10.21037/gs-24-308/prf
Conflicts of Interest: All authors have completed the ICMJE uniform disclosure form (available at https://gs.amegroups.com/article/view/10.21037/gs-24-308/coif). The authors have no conflicts of interest to declare.
Ethical Statement: The authors are accountable for all aspects of the work in ensuring that questions related to the accuracy or integrity of any part of the work are appropriately investigated and resolved. The study was conducted in accordance with the Declaration of Helsinki (as revised in 2013). The study was approved by the Ethics Committee of Hangzhou Hospital of Traditional Chinese Medicine (No. 2022KY153-CX1). All participating hospitals were informed and agreed with this study. Individual consent for this retrospective analysis was waived.
Open Access Statement: This is an Open Access article distributed in accordance with the Creative Commons Attribution-NonCommercial-NoDerivs 4.0 International License (CC BY-NC-ND 4.0), which permits the non-commercial replication and distribution of the article with the strict proviso that no changes or edits are made and the original work is properly cited (including links to both the formal publication through the relevant DOI and the license). See: https://creativecommons.org/licenses/by-nc-nd/4.0/.
References
- Siegel RL, Miller KD, Wagle NS, et al. Cancer statistics, 2023. CA Cancer J Clin 2023;73:17-48. [Crossref] [PubMed]
- Xu S, Huang H, Huang Y, et al. Comparison of Lobectomy vs Total Thyroidectomy for Intermediate-Risk Papillary Thyroid Carcinoma With Lymph Node Metastasis. JAMA Surg 2023;158:73-9. [Crossref] [PubMed]
- van Dijk SPJ, Coerts HI, Gunput STG, et al. Assessment of Radiofrequency Ablation for Papillary Microcarcinoma of the Thyroid: A Systematic Review and Meta-analysis. JAMA Otolaryngol Head Neck Surg 2022;148:317-25. [Crossref] [PubMed]
- Chen DW, Lang BHH, McLeod DSA, et al. Thyroid cancer. Lancet 2023;401:1531-44. [Crossref] [PubMed]
- Xiao L, Zhou J, Tan W, et al. Contrast-enhanced US with Perfluorobutane to Diagnose Small Lateral Cervical Lymph Node Metastases of Papillary Thyroid Carcinoma. Radiology 2023;307:e221465. [Crossref] [PubMed]
- Machens A, Dralle H. Correlation between the number of lymph node metastases and lung metastasis in papillary thyroid cancer. J Clin Endocrinol Metab 2012;97:4375-82. [Crossref] [PubMed]
- Haugen BR, Alexander EK, Bible KC, et al. 2015 American Thyroid Association Management Guidelines for Adult Patients with Thyroid Nodules and Differentiated Thyroid Cancer: The American Thyroid Association Guidelines Task Force on Thyroid Nodules and Differentiated Thyroid Cancer. Thyroid 2016;26:1-133. [Crossref] [PubMed]
- Oh HS, Park S, Kim M, et al. Young Age and Male Sex Are Predictors of Large-Volume Central Neck Lymph Node Metastasis in Clinical N0 Papillary Thyroid Microcarcinomas. Thyroid 2017;27:1285-90. [Crossref] [PubMed]
- Cho SJ, Suh CH, Baek JH, et al. Active Surveillance for Small Papillary Thyroid Cancer: A Systematic Review and Meta-Analysis. Thyroid 2019;29:1399-408. [Crossref] [PubMed]
- Kambalapalli M, Gupta A, Prasad UR, et al. Ultrasound characteristics of the thyroid in children and adolescents with goiter: a single center experience. Thyroid 2015;25:176-82. [Crossref] [PubMed]
- Lee DW, Ji YB, Sung ES, et al. Roles of ultrasonography and computed tomography in the surgical management of cervical lymph node metastases in papillary thyroid carcinoma. Eur J Surg Oncol 2013;39:191-6. [Crossref] [PubMed]
- Wang C, Yu P, Zhang H, et al. Artificial intelligence-based prediction of cervical lymph node metastasis in papillary thyroid cancer with CT. Eur Radiol 2023;33:6828-40. [Crossref] [PubMed]
- Li F, Pan D, He Y, et al. Using ultrasound features and radiomics analysis to predict lymph node metastasis in patients with thyroid cancer. BMC Surg 2020;20:315. [Crossref] [PubMed]
- Iqbal S, Qureshi AN, Alhussein M, et al. AD-CAM: Enhancing Interpretability of Convolutional Neural Networks with a Lightweight Framework - From Black Box to Glass Box. IEEE J Biomed Health Inform 2023; Epub ahead of print. [Crossref] [PubMed]
- Liu T, Zhou S, Yu J, et al. Prediction of Lymph Node Metastasis in Patients With Papillary Thyroid Carcinoma: A Radiomics Method Based on Preoperative Ultrasound Images. Technol Cancer Res Treat 2019;18:1533033819831713. [Crossref] [PubMed]
- Wu Q, Li Y, Wang Y, et al. Sonographic features of primary tumor as independent predictive factors for lymph node metastasis in papillary thyroid carcinoma. Clin Transl Oncol 2015;17:830-4. [Crossref] [PubMed]
- Nie X, Tan Z, Ge M, et al. Risk factors analyses for lateral lymph node metastases in papillary thyroid carcinomas: a retrospective study of 356 patients. Arch Endocrinol Metab 2016;60:492-9. [Crossref] [PubMed]
- Yu B, Li Y, Yu X, et al. Differentiate Thyroid Follicular Adenoma from Carcinoma with Combined Ultrasound Radiomics Features and Clinical Ultrasound Features. J Digit Imaging 2022;35:1362-72. [Crossref] [PubMed]
- Peng S, Liu Y, Lv W, et al. Deep learning-based artificial intelligence model to assist thyroid nodule diagnosis and management: a multicentre diagnostic study. Lancet Digit Health 2021;3:e250-9. [Crossref] [PubMed]
- Tessler FN, Middleton WD, Grant EG. Thyroid Imaging Reporting and Data System (TI-RADS): A User's Guide. Radiology 2018;287:29-36. [Crossref] [PubMed]
- Jiang L, Guo S, Zhao Y, et al. Predicting Extrathyroidal Extension in Papillary Thyroid Carcinoma Using a Clinical-Radiomics Nomogram Based on B-Mode and Contrast-Enhanced Ultrasound. Diagnostics (Basel) 2023;13:1734. [Crossref] [PubMed]
- Wu J, Gensheimer MF, Zhang N, et al. Tumor Subregion Evolution-Based Imaging Features to Assess Early Response and Predict Prognosis in Oropharyngeal Cancer. J Nucl Med 2020;61:327-36. [Crossref] [PubMed]
- Lu J, Liao J, Chen Y, et al. Risk factor analysis and prediction model for papillary thyroid carcinoma with lymph node metastasis. Front Endocrinol (Lausanne) 2023;14:1287593. [Crossref] [PubMed]
- Zhang H, Ogasawara K. Grad-CAM-Based Explainable Artificial Intelligence Related to Medical Text Processing. Bioengineering (Basel) 2023;10:1070. [Crossref] [PubMed]
- Jia X, Ma Z, Kong D, et al. Novel Human Artificial Intelligence Hybrid Framework Pinpoints Thyroid Nodule Malignancy and Identifies Overlooked Second-Order Ultrasonographic Features. Cancers (Basel) 2022;14:4440. [Crossref] [PubMed]
(English Language Editor: J. Jones)