Contrast-enhanced ultrasound image sequences based on radiomics analysis for diagnosis of metastatic cervical lymph nodes from thyroid cancer
Highlight box
Key findings
• The support vector machine learning based on contrast-enhanced ultrasound (CEUS) videos is helpful for the diagnosis of whether metastasis occurs in the cervical lymph nodes of thyroid cancer patients.
What is known and what is new?
• Thyroid cancer prone to cervical lymph node metastasis both before and after surgery. Ultrasonography (US) is the first-line imaging method for evaluating the thyroid gland and cervical lymph nodes. However, this assessment relies mainly on the subjective judgment of the sonographer and is very much dependent on the sonographer’s experience.
• Artificial intelligence assisted diagnosis can reduce dependence on US operators. In recent years, it has become popular in ultrasonic diagnoses.
What is the implication, and what should change now?
• The study tried three machine learning based on CEUS videos for the diagnosis of whether metastasis occurs in the cervical lymph nodes of thyroid cancer patients. The support vector machine had the best diagnostic efficacy, with a sensitivity of 93.0%, a specificity of 93.8%, and an accuracy of 93.3%. Further multi-center studies are needed for more conformation.
Introduction
Thyroid cancer (TC) is the fastest growing tumor in terms of incidence in recent years and differentiated thyroid carcinoma has been widely considered a malignant tumor with good prognosis (1). However, TC is prone to cervical lymph node (CLN) metastasis both before and after surgery. It is reported that papillary thyroid carcinoma (PTC) involves CLN metastasis in 20–50% (2), and more than half of TCs are associated with CLN metastasis at the time of initial diagnosis (1). Evaluation of CLN metastasis is important for TC patients, not only for long-term clinical outcomes, treatment planning and overall prognosis assessment, but also in relation to local recurrence and distant metastasis (3,4). Preoperative assessment of CLN status in patients with TC is critical in guiding the extent of lymph node dissection. Accurate preoperative assessment helps to minimize secondary surgeries due to missed diagnosis and reduces the risk of laryngeal recurrent nerve injury and complications such as recurrent laryngeal nerve injury and parathyroid decompensation. Accurate assessment depends primarily on the results of CLN imaging.
Ultrasonography (US) is the first-line imaging method for evaluating the thyroid gland and CLNs. However, the specificity of US in detecting abnormal lymph nodes varies widely, ranging from 25% to 90% (5). Factors affecting sensitivity include the location of the lymph node and the expertise of the physician. In routine clinical work, physicians classify lymph nodes into benign or malignant groups based on their morphology, echogenicity, presence calcification or not, and blood flow patterns (1,6,7), however, this assessment relies mainly on the subjective judgment of the sonographer and is very much dependent on the sonographer’s experience (8).
Contrast-enhanced ultrasound (CEUS) provides new technique for differential diagnosis of CLN. It is used to assess tissue and organ microcirculatory perfusion by injecting US contrast agent through a peripheral venous mass. By CEUS technique, the microcirculatory perfusion of lymph nodes can be assessed both qualitatively and quantitatively, providing additional information compared with conventional US. Previous reports have shown that CEUS could contribute to more accurate detection of lymph node metastasis (9-11).
Artificial intelligence (AI)-assisted diagnosis can reduce dependence on US operators. In recent years, it has become popular in ultrasonic diagnoses (12-14). There are several studies reported that AI related ultrasonic image analysis has been applied in the field of thyroid, breast, liver, obstetrics and gynecology, and so on (15,16). Some commercial AI systems have been integrated into ultrasonic machines for real-time diagnosis (17). However, there is no machine learning study to predict the risk of CLN metastasis from TC based on CEUS image sequences. Therefore, in this prospective study, we focused on the use of CEUS videos to develop a computer aided diagnosis (CAD) system to correctly identify benign and malignant lymph nodes in TC patients. We present this article in accordance with the TRIPOD reporting checklist (available at https://gs.amegroups.com/article/view/10.21037/gs-24-98/rc).
Methods
Patients
The study was conducted in accordance with the Declaration of Helsinki (as revised in 2013). The study was approved by the Ethics Office of West China Hospital (No. 1341). All patients signed an informed consent form.
Between August 2019 and May 2020, hospital patients who met the inclusion criteria were included in the study. Inclusion criteria: patients agreed to participate in the study; age older than 18 years, patients had pathologically confirmed TC; patients with no serious cardiopulmonary dysfunction, and patients had no history of other malignancies.
Exclusion criteria: patients were pregnant or lactating; patients with a mental disorder hindering comprehensive assessment; patients had intense breathing or extensive movement; patients who refused to participate in this study.
CEUS examination
The examination equipment was Mindray RESONA7T machine with L14–5 and L9–3 probe, and the contrast agent was SonoVue (Brocco, Italy).
Prior to operation, conventional US and CEUS were performed. An informed consent form was signed by each patient before the examination. Patients were maintained at supine position and breathed at a normal and regular pace without swallowing. Firstly, the L14–5 probe was used to scan the suspicious lymph nodes from multiple angles, and the most suspicious or the largest plane was selected for CEUS. CEUS was performed using an L9–3 linear array transducer, and a mechanical index of 0.1. The frame rate was set to 10 frame/s. 2.4 mL of SonoVue agent was injected through the elbow vein mass, followed by rinsing with 5 mL of saline, while video recording was started. A 2-minute duration video was recorded and stored in digital imaging and communications in medicine (DICOM) mode.
US-guided fine-needle aspiration of lymph nodes was performed within one week of imaging, cytopathologic examination and thyroglobulin (Tg) testing of washout were detected. Malignancy was diagnosed when the pathology was abnormal or the washout Tg level was significantly higher than serum Tg level, and when pathology was normal and Tg concentration was not risen, the CLN was classified into benign group.
Machine learning
The radiomics evaluation included target tracking, feature extraction, feature selection and classification. The workflow is shown in Figure 1.
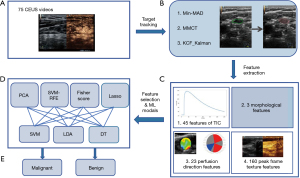
Target tracking
US images of lymph nodes contain various sources of noise, such as blood vessels and muscle tissues, which results in difficulty to detect CLN correctly during the development of CAD system (18). Therefore, we decided to extract only the lymph node region for use as a training set.
Firstly, the CEUS video was divided into frame images and the peak frames were selected. This part of the work was done on Matlab 2019. The CEUS video contains two windows: the left window is the US image, and the right window is the CEUS image. The position of the lymph node in the two windows is consistent, and it is easier to detect the lymph node from the US window than the contrast window. So the CLN region was manually tagged by a radiologist with 10-year experience in the US window in the peak frames. While recording the US video, the lymph node images moved back and forth with breathing, thus requiring target tracking.
Three different target tracking algorithms, namely minimum mean absolute deviation (Min-MAD) target tracking, multi-model compressive tracking (MMCT), and Kalman filtering combined with kernel correlation filter (KCF) targets, were tested. Three different methods of MAD contrast plot, dx vs. dy curve, and schematic diagram of target box were used to evaluate the effectiveness of these target tracking methods, and target tracking algorithm with best performance was used for target tracking in the modal.
Feature extraction
Using imageomics, the motion-compensated CEUS images were subjected to temporal and spatial feature extraction, and a total of 231-dimensional features were obtained.
CEUS videos record the concentration of contrast agent perfusion in lymph nodes over time, and the intensity values of video pixels are commonly used to represent the contrast agent concentration in a previous study (19). In this study, the Hexcone model (HSV color model) was utilized for the conversion of intensity values. HSV is a color model where H stands for hue, S for saturation, and V for value. In this study, the value of H ranges from 0 to 360, S from 0 to 1, and V from 0 to 1. According to Figure 2, the value of hue (H) hardly changes during CEUS, and the color saturation (S) cannot be used to reflect the change of contrast concentration. Therefore, the value (V) was used as an independent indicator of the change in contrast concentration. The average value curve was obtained by scaling the V value to [0, 255] and then calculating the average gray value for each image frame. A combined gamma-Gaussian fitting model was also used to fit the time intensity curve (TIC) curves.
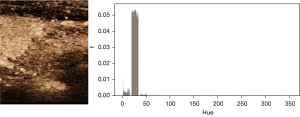
Based on the radiomics, gray level dependence matrix was used for feature extraction based on pixel level of TIC. Nine perfusion time-domain features were extracted for basic intensity (BI), arrival time (AT), time to peak (TTP), peak intensity (PI), ascending slope (AS), descending half-time (HT), descending slope (DS), area under the curve (AUC), and mean transit time (MTT). The parameter BI was defined as the intensity before the agent was flowed into the region of interest (ROI). AT was defined as the first point of the curve clearly above the baseline intensity. TTP was the time from the start of the injection to the maximum intensity of the curve. PI was the maximal intensity. AS was the slope between the beginning point and the peak point. HT was the time when the intensity descended from peak to half. DS was defined as the slope between the peak point to half descent of intensity. The mean, standard deviation, median, 75th percentile and 90th percentile of each parameter were selected for the study. Thus, a total of 45 domain features were extracted for every pixel in this study.
The spatial features included morphological features, perfusion direction features, and peak frame texture features. Three of the morphological features included lymph node roundness, lymph node length-to-diameter ratio, and the ratio of the number of successful coordinate points based on pixel-level TIC fitting to the total number of coordinate points within the ROI. Twenty-three perfusion direction features were extracted using a two-dimensional matrix of TTP parameters.
According to expert experience, the intensity of near peak frames should be more valuable for diagnosis. So we tried to extract the texture features near contrast peak frames. Taking the peak frame as the center, a total of 20 frame images forward and backward were selected to form a new image sequence, and a time-domain averaged map was obtained by calculating average intensity of these frames. Four parameters of contrast, energy, homogeneity and dissimilarity were extracted based on gray level co-occurrence matrix (GLCM). Finally, a total of 160 peak frame texture features were extracted.
Due to the reproducibility and redundancy of the radiomics features, four different feature selection methods were employed to reduce the dimensions of the feature set, with principal component analysis (PCA), recursive feature elimination based on support vector machines (SVM-RFE), least absolute shrinkage and selection operator (LASSO) and Fisher score including.
PCA is a common data dimensionality reduction technique that projects the initial data onto a lower dimensional space where the main variation modes are highlighted (20). Mathematically, PCA determines a linear mapping M which maximizes the , where S is the covariance matrix of the dataset U. The mapping is then computed and the covariance matrix of the dataset U is then computed. Each eigenvalue is computed and M more significant eigenvalues are selected.
The SVM-RFE method is one of the recursive feature elimination methods (21). The least effective feature parameters are removed during an iterative loop and the subset of more important features is obtained by ranking the importance of the self-features according to the order in which they were removed.
The Fisher score method works by mapping the high dimensional data onto the low dimensional data space so that the low dimensional data has the minimum same class distance and maximum inter-class distance. In the feature selection process, a value is assigned to each feature, and the higher the feature assignment, the greater the contribution to classification (22).
The LASSO algorithm is a linear regression analysis method, the principle of which is to minimize the residual sum of squares under the constraint that the sum of the absolute values of the regression coefficients is less than a constant, thus making some of the regression coefficients go to zero, and achieving the purpose of feature selection (23).
Machine learning models
Three robust machine learning algorithms, linear SVM, linear discriminant analysis (LDA), and decision tree (DT), were applied to the CEUS data to predict CLN categories.
Therefore, four feature selection algorithms and three machine learning algorithms were used in this study to categorize lymph nodes. The four feature selection algorithms were used in combination with each machine learning algorithm in turn to select the best combination.
Statistical analysis
In this study, leave-one-out cross-validation (LOOCV) was used for model training. LOOCV (24) is a special case of k-times cross validation with k=n, the number of observations. Each time (n−1) samples are used to train the model and the remaining one sample is used to test the model and n analyses are performed to obtain the average classification accuracy. LOOCV is more efficient but due to the expensive computational cost, it is applicability to small sample sizes.
We trained the classification models for diagnosis. The number of true positive samples, true negative samples, false negative samples and false positive samples were calculated, and the accuracy, sensitivity, specificity, F1-score were statistically calculated. The machine learning modeling and statistical analyses were implemented by using Python (version 3.0) and SPSS (version 25.0).
Results
A total of 75 cases were included in the study. All patients were PTC patients and confirmed by postoperative pathology. The age ranged from 21 to 73 years, with a mean age of 42.6±11.4 years, of which 25 were male and 50 were female. Of the 75 lymph nodes, 42 were benign and 33 were malignant. The range of the long diameter of the lymph nodes was 6–28 mm, with a mean value of 12.1±4.6 mm, and the range of the short diameter was 4–15 mm, with a mean value of 6.0±2.2 mm.
The results of target tracking are shown in Figures 3-5. Through comprehensive analysis, the kernel correlation filtering algorithm combined with Kalman filtering was used in this study for experimentation.
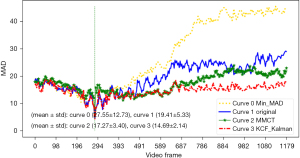
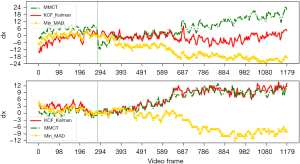
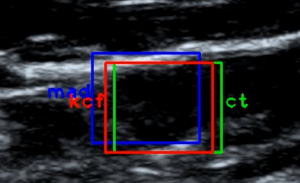
The results of sensitivity, specificity and accuracy of the model to diagnose lymph nodes are shown in Table 1.
Table 1
Classifier combined feature selection | Sensitivity (%) | Specificity (%) | Accuracy (%) | F1-score |
---|---|---|---|---|
SVM-RFE + SVM | 93.0 | 93.8 | 93.3 | 0.941 |
LASSO + LDA | 80.4 | 82.8 | 81.3 | 0.711 |
Fisher + DT | 78.4 | 65.8 | 72.0 | 0.667 |
SVM-RFE, recursive feature elimination based on support vector machine; SVM, support vector machine; LASSO, least absolute shrinkage and selection operator; LDA, linear discriminant; DT, decision tree.
When the SVM classification model was selected, the relationship graph between the diagnostic accuracy and each feature selection algorithm is shown in Figure 6.
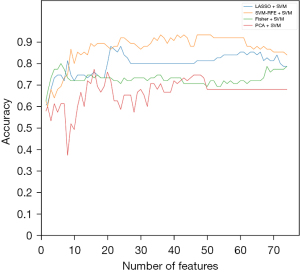
When the LDA classification model was selected, the correspondence graph between the diagnostic accuracy and each feature selection algorithm is shown in Figure 7.
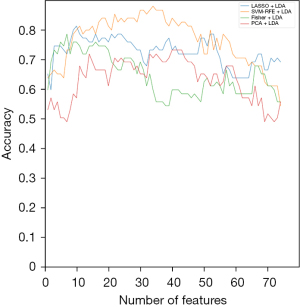
When the DT classification model was selected, the graph of diagnostic accuracy versus each feature selection algorithm was shown in Figure 8.
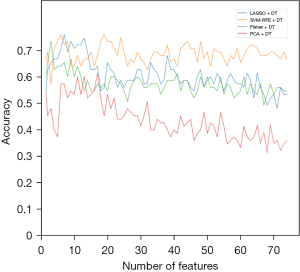
Among these three classification algorithms, SVM-RFE showed the best accuracy as a feature elimination method. Twenty-two key radiomic features were selected by SVM-RFE to build the DT classifier. For LDA classification modeling, the highest accuracy was achieved when 34 key radiomic features were selected. For the SVM modeling, the best accuracy was achieved when 47 key radiomic features were selected. Among these combinations, the SVM-RFE combination SVM classifier showed the best results, with 93.3% accuracy, 93.0% sensitivity and 93.8% specificity.
Discussion
In this study, the visual image information of lymph node CEUS image sequence was transformed into deep-level features for quantitative research, and a large amount of image feature information was extracted in a high-throughput manner and mined at a deep level, and three of machine learning models were constructed based on the feature information. To our knowledge, this is the first research involving the development and validation of a CAD system specific for lymph node metastases of TCs using CEUS image sequence. By comparison, the SVM machine learning model combined with SVM-RFE feature selection method had the best diagnostic performance, with 93.3% accuracy, 93.0% sensitivity, and 93.8% specificity.
Routine high-frequency US is the basic examination for assessing the status of CLNs in patients with TC. Ultrasonographic features of lymph node metastases in patients with PTC include micro-calcifications, a partially cystic appearance, peripheral blood flow, and the absence of lymphatic hilar structures (6,25). However, conventional US examination of CLNs has some limitations. Firstly, CLNs are vulnerable sites of lymphadenitis, lymphoma, and tumor metastasis. Sometimes it is a great challenge to distinguish these diseases only based on conventional ultrasonic features (26,27). Secondly, US could be time consuming and with a substantial learning curve. These result in the yield of the US exam being highly dependent on the experience of the radiologist (28,29). CEUS is a new ultrasonic technique that reflects microcirculatory perfusion in real time. Several studies have reported CEUS as a potential imaging modality to assess lymph node metastasis in patients with PTC (9,11). This correlates positively with pathology. In benign lymph nodes, normal vessels enter through gates and spread evenly in the branches. US in benign lymph nodes shows uniform hyper- or iso-enhancement with centrifugal perfusion. In contrast, when CLNs are involved in TC, tumor cells first invade the subperitoneal sinus, inducing neovascularization locally from the incoming lymphatic vessels before affecting the lymphatic gates. Therefore, on CEUS, metastatic lymph nodes show heterogeneous areas of low or no enhancement, centripetal perfusion, and areas of abnormal hyperperfusion (30). Some studies on quantitative and qualitative analysis of lymph node CEUS have shown that CEUS parameters are helpful in the differential diagnosis of benign and malignant lymph nodes (9,31,32). Based on previous study results, in this study, we simultaneously extracted lymph node morphological features, temporal enhancement curve time-domain features, perfusion direction features, and peak frame texture features to construct a machine learning model, and the diagnostic effect was better than that of conventional US (18), which proved that CEUS-based AI analysis is more helpful in assisting the clinician’s diagnosis.
There have been several radiomics studies which rely on manual thyroid nodules segmentation to predict metastasis to the CLNs (33,34). The accuracy ranged 72.0% to 82.8%. Only a limited number of studies have focused on the role of deep learning solution in differentiating benign and malignant CLN in the setting of TC. Lee et al. developed a deep learning-based CAD system to localize and differentiate metastatic lymph nodes from TC on US (18). The CAD system achieved an acceptable diagnostic performance, with accuracy of 83.0%, sensitivity of 89.0%, and specificity of 77.0%. Abbasian Ardakani et al. (35) developed a “ClymphNet” deep learning model based on B-mode US images. The ClymphNet model achieved an accuracy of 91.77%, sensitivity of 92.68%, and specificity of 90.79%. There was only one study which reported to design a lymph node convolutional neural network (LN-Net) to predict lymph node metastasis based on CEUS video. The LN-Net system had the highest accuracy of 84.9% (36). Different from this study, our study developed the CAD system based on CEUS image sequence of lymph nodes from TC patients. Compared with previous studies, accuracy was improved by 1.7–10%. The intelligent diagnosis method has good clinical interpretability.
There are some limitations of this study. First, this is a single-center study, and multicenter studies are also required to improve the robustness and accuracy of model classification. Second, we only used CEUS videos of lymph nodes to categorize them. It may be useful for developing a model more accurately if clinical data and epidemiologic considerations were included.
Conclusions
In conclusion, we developed a deep learning-based CAD system for differentiation of CLN metastases from TC based on CEUS videos. This CAD system was able to achieve a sensitivity of 93.0%, specificity of 93.8%, the accuracy of 93.3%, which was superior to the other deep learning models with conventional ultrasonic images. Our study confirmed CAD system may be useful as a screening tool for distinguishing benign and malignant lymph nodes. Additionally, more clinical and imaging data will be required for further validation to determine the real-world relative efficacy.
Acknowledgments
Funding: This study was supported by grants from
Footnote
Reporting Checklist: The authors have completed the TRIPOD reporting checklist. Available at https://gs.amegroups.com/article/view/10.21037/gs-24-98/rc
Data Sharing Statement: Available at https://gs.amegroups.com/article/view/10.21037/gs-24-98/dss
Peer Review File: Available at https://gs.amegroups.com/article/view/10.21037/gs-24-98/prf
Conflicts of Interest: All authors have completed the ICMJE uniform disclosure form (available at https://gs.amegroups.com/article/view/10.21037/gs-24-98/coif). The authors have no conflicts of interest to declare.
Ethical Statement: The authors are accountable for all aspects of the work in ensuring that questions related to the accuracy or integrity of any part of the work are appropriately investigated and resolved. The study was conducted in accordance with the Declaration of Helsinki (as revised in 2013). The study was approved by the Ethics Office of West China Hospital (No. 1341). All patients signed an informed consent form.
Open Access Statement: This is an Open Access article distributed in accordance with the Creative Commons Attribution-NonCommercial-NoDerivs 4.0 International License (CC BY-NC-ND 4.0), which permits the non-commercial replication and distribution of the article with the strict proviso that no changes or edits are made and the original work is properly cited (including links to both the formal publication through the relevant DOI and the license). See: https://creativecommons.org/licenses/by-nc-nd/4.0/.
References
- Haddad RI, Bischoff L, Ball D, et al. Thyroid Carcinoma, Version 2.2022, NCCN Clinical Practice Guidelines in Oncology. J Natl Compr Canc Netw 2022;20:925-51. [Crossref] [PubMed]
- Haugen BR, Alexander EK, Bible KC, et al. 2015 American Thyroid Association Management Guidelines for Adult Patients with Thyroid Nodules and Differentiated Thyroid Cancer: The American Thyroid Association Guidelines Task Force on Thyroid Nodules and Differentiated Thyroid Cancer. Thyroid 2016;26:1-133. [Crossref] [PubMed]
- Feng JW, Yang XH, Wu BQ, et al. Predictive factors for central lymph node and lateral cervical lymph node metastases in papillary thyroid carcinoma. Clin Transl Oncol 2019;21:1482-91. [Crossref] [PubMed]
- Wang C, Yu P, Zhang H, et al. Artificial intelligence-based prediction of cervical lymph node metastasis in papillary thyroid cancer with CT. Eur Radiol 2023;33:6828-40. [Crossref] [PubMed]
- Albuck AL, Issa PP, Hussein M, et al. A combination of computed tomography scan and ultrasound provides optimal detection of cervical lymph node metastasis in papillary thyroid carcinomas: A systematic review and meta-analysis. Head Neck 2023;45:2173-84. [Crossref] [PubMed]
- Lee JY, Baek JH, Ha EJ, et al. 2020 Imaging Guidelines for Thyroid Nodules and Differentiated Thyroid Cancer: Korean Society of Thyroid Radiology. Korean J Radiol 2021;22:840-60. [Crossref] [PubMed]
- Rago T, Cantisani V, Ianni F, et al. Thyroid ultrasonography reporting: consensus of Italian Thyroid Association (AIT), Italian Society of Endocrinology (SIE), Italian Society of Ultrasonography in Medicine and Biology (SIUMB) and Ultrasound Chapter of Italian Society of Medical Radiology (SIRM). J Endocrinol Invest 2018;41:1435-43. [Crossref] [PubMed]
- Kravchenko T, Chen V, Hsu D, et al. Which Ultrasound Characteristics Predict Lymphatic Spread of Papillary Thyroid Cancer? J Surg Res 2024;299:263-8. [Crossref] [PubMed]
- Chen L, Chen L, Liu J, et al. Value of Qualitative and Quantitative Contrast-Enhanced Ultrasound Analysis in Preoperative Diagnosis of Cervical Lymph Node Metastasis From Papillary Thyroid Carcinoma. J Ultrasound Med 2020;39:73-81. [Crossref] [PubMed]
- Liu X, Wang M, Wang Q, et al. Diagnostic value of contrast-enhanced ultrasound for sentinel lymph node metastasis in breast cancer: an updated meta-analysis. Breast Cancer Res Treat 2023;202:221-31. [Crossref] [PubMed]
- Yang Z, Wang X, Tao T, et al. Diagnostic value of contrast-enhanced ultrasonography in the preoperative evaluation of lymph node metastasis in papillary thyroid carcinoma: a single-center retrospective study. BMC Surg 2023;23:325. [Crossref] [PubMed]
- Zhang Q, Zhang S, Pan Y, et al. Deep learning to diagnose Hashimoto's thyroiditis from sonographic images. Nat Commun 2022;13:3759. [Crossref] [PubMed]
- Wang J, Dong C, Zhang YZ, et al. A novel approach to quantify calcifications of thyroid nodules in US images based on deep learning: predicting the risk of cervical lymph node metastasis in papillary thyroid cancer patients. Eur Radiol 2023;33:9347-56. [Crossref] [PubMed]
- Zhang B, Jin Z, Zhang S. A deep-learning model to assist thyroid nodule diagnosis and management. Lancet Digit Health 2021;3:e410. [Crossref] [PubMed]
- Shen YT, Chen L, Yue WW, et al. Artificial intelligence in ultrasound. Eur J Radiol 2021;139:109717. [Crossref] [PubMed]
- Yu ZH, Hong YT, Chou CP. Enhancing Breast Cancer Diagnosis: A Nomogram Model Integrating AI Ultrasound and Clinical Factors. Ultrasound Med Biol 2024;50:1372-80. [Crossref] [PubMed]
- Choi YJ, Baek JH, Park HS, et al. A Computer-Aided Diagnosis System Using Artificial Intelligence for the Diagnosis and Characterization of Thyroid Nodules on Ultrasound: Initial Clinical Assessment. Thyroid 2017;27:546-52. [Crossref] [PubMed]
- Lee JH, Baek JH, Kim JH, et al. Deep Learning-Based Computer-Aided Diagnosis System for Localization and Diagnosis of Metastatic Lymph Nodes on Ultrasound: A Pilot Study. Thyroid 2018;28:1332-8. [Crossref] [PubMed]
- Greis C. Quantitative evaluation of microvascular blood flow by contrast-enhanced ultrasound (CEUS). Clin Hemorheol Microcirc 2011;49:137-49. [Crossref] [PubMed]
- Mitrea D, Badea R, Mitrea P, et al. Hepatocellular Carcinoma Automatic Diagnosis within CEUS and B-Mode Ultrasound Images Using Advanced Machine Learning Methods. Sensors (Basel) 2021;21:2202. [Crossref] [PubMed]
- Sanz H, Valim C, Vegas E, et al. SVM-RFE: selection and visualization of the most relevant features through non-linear kernels. BMC Bioinformatics 2018;19:432. [Crossref] [PubMed]
Gu Q Li Z Han J. Generalized Fisher Score for feature selection. 2012 . arXiv:1202.3725.- Kang J, Choi YJ, Kim IK, et al. LASSO-Based Machine Learning Algorithm for Prediction of Lymph Node Metastasis in T1 Colorectal Cancer. Cancer Res Treat 2021;53:773-83. [Crossref] [PubMed]
- Kurogi S, Toidani M, Shigematsu R, et al. Performance improvement via bagging in probabilistic prediction of chaotic time series using similarity of attractors and LOOCV predictable horizon. Neural Comput Appl 2018;29:341-9. [Crossref] [PubMed]
- Zhao H, Li H. Meta-analysis of ultrasound for cervical lymph nodes in papillary thyroid cancer: Diagnosis of central and lateral compartment nodal metastases. Eur J Radiol 2019;112:14-21. [Crossref] [PubMed]
- Białek EJ, Jakubowski W. Mistakes in ultrasound diagnosis of superficial lymph nodes. J Ultrason 2017;17:59-65. [Crossref] [PubMed]
- Ling W, Nie J, Zhang D, et al. Role of Contrast-Enhanced Ultrasound (CEUS) in the Diagnosis of Cervical Lymph Node Metastasis in Nasopharyngeal Carcinoma (NPC) Patients. Front Oncol 2020;10:972. [Crossref] [PubMed]
- Liu Y, Zhao J, Luo Q, et al. Automated classification of cervical lymph-node-level from ultrasound using Depthwise Separable Convolutional Swin Transformer. Comput Biol Med 2022;148:105821. [Crossref] [PubMed]
- Konishi M, Kakimoto N. Radiomics analysis of intraoral ultrasound images for prediction of late cervical lymph node metastasis in patients with tongue cancer. Head Neck 2023;45:2619-26. [Crossref] [PubMed]
- Liu Z, Wang R, Zhou J, et al. Ultrasound lymphatic imaging for the diagnosis of metastatic central lymph nodes in papillary thyroid cancer. Eur Radiol 2021;31:8458-67. [Crossref] [PubMed]
- Zhu AQ, Li XL, An LW, et al. Predicting Axillary Lymph Node Metastasis in Patients With Breast Invasive Ductal Carcinoma With Negative Axillary Ultrasound Results Using Conventional Ultrasound and Contrast-Enhanced Ultrasound. J Ultrasound Med 2020;39:2059-70. [Crossref] [PubMed]
- Zhang YX, Wang XM, Kang S, et al. Contrast-enhanced ultrasonography in qualitative diagnosis of sentinel lymph node metastasis in breast cancer: A meta-analysis. J Cancer Res Ther 2015;11:697-703. [Crossref] [PubMed]
- Wang Z, Qu L, Chen Q, et al. Deep learning-based multifeature integration robustly predicts central lymph node metastasis in papillary thyroid cancer. BMC Cancer 2023;23:128. [Crossref] [PubMed]
- Lee JH, Ha EJ, Kim D, et al. Application of deep learning to the diagnosis of cervical lymph node metastasis from thyroid cancer with CT: external validation and clinical utility for resident training. Eur Radiol 2020;30:3066-72. [Crossref] [PubMed]
- Abbasian Ardakani A, Mohammadi A, Mirza-Aghazadeh-Attari M, et al. Diagnosis of Metastatic Lymph Nodes in Patients With Papillary Thyroid Cancer: A Comparative Multi-Center Study of Semantic Features and Deep Learning-Based Models. J Ultrasound Med 2023;42:1211-21. [Crossref] [PubMed]
- Yu H, Liang X, Zhang M, et al. LN-Net: Perfusion Pattern-Guided Deep Learning for Lymph Node Metastasis Diagnosis Based on Contrast-Enhanced Ultrasound Videos. Ultrasound Med Biol 2023;49:1248-58. [Crossref] [PubMed]