The value of diffusion kurtosis imaging and dynamic contrast-enhanced magnetic resonance imaging in the differential diagnosis of parotid gland tumors
Highlight box
Key findings
• The combined use of diffusion kurtosis imaging (DKI) and dynamic contrast-enhanced magnetic resonance imaging (DCE-MRI) parameters and their relative parameters can improve the diagnostic accuracy of distinguishing parotid gland tumors (PGTs).
What is known and what is new?
• It is known that DKI and DCE-MRI have certain advantages in the diagnosis of PGTs.
• In our study, only the combination of DKI and DCE-MRI parameters could reliably distinguish basal cell adenomas from other PGTs, and the relative values of DKI and DCE-MRI parameters can also improve their diagnostic ability for PGTs.
What is the implication, and what should change now?
• Our data suggested that the combined use of DKI and DCE-MRI and their relative values can improve the diagnostic performance of PGTs. And this suggested that the use of DKI and DCE-MRI may help to optimize the surgical mode decision for patients with PGTs in the future clinical work.
Introduction
Only 3–6% of head and neck tumors are salivary gland tumors, making them uncommon, with the parotid gland being the most frequent location (79%) (1). Parotid gland tumors (PGTs) are typically benign. However, the incidence of symptoms and signs in malignant tumors (MTs) is significantly higher than that in benign tumors (BTs), and it is more common in high-grade MTs (2). Pleomorphic adenomas (PAs), Warthin tumors (WTs), and basal cell adenomas (BCAs) are the three most prevalent types of BTs (3). Complete surgical resection typically treats MTs and PAs to prevent recurrence and malignant transformation, while WTs and BCAs may benefit from local or supra-fascial resection or conservative patient monitoring (4-6). The imaging presentation of most BCAs is similar to that of BTs. However, some BCAs should be handled cautiously because they could develop a slight chance of becoming malignant and might have imaging characteristics that resemble those of MTs (7). Therefore, as this information affects the surgical strategy, a precise preoperative diagnosis is crucial for its creation.
Fine needle aspiration cytology (FNAC) for differentiating malignant from benign parotid lesions has been reported to have moderate sensitivity and high specificity (8). Despite its advantages, however, the accuracy of FNAC remains contested (9). And even if the probability is low, there is still the possibility of tumor cell seeding after FNAC (10).
Conventional magnetic resonance imaging (MRI) can clearly show PGTs and their surrounding tissues due to their strong tissue contrast resolution (11). Increasingly, advanced techniques have been used to aid in the differential diagnosis of PGTs (4,12-14). The hemodynamic data and quantitative metrics provided by dynamic contrast-enhanced MRI (DCE-MRI) can be used to differentiate between BTs and MTs. In addition, quantitative DCE-MRI has been shown in a few studies to be helpful in detecting PTs; however, several inherent limitations remain, including small sample sizes or poor temporal resolution (15-18). Diffusion kurtosis imaging (DKI) can produce two parameters by measuring the non-Gaussian behavior of water molecule diffusion, including diffusion kurtosis (K) and diffusion coefficient (D). DKI has a strong diagnostic potential for the differential diagnosis of PGTs with various pathogenic features (19,20).
Only one study has demonstrated the increased benefit of integrating DKI and DCE-MRI in identifying PGTs (21). However, this study is limited because of its small sample size and lack of a differential diagnosis for BCAs. In addition, the MRI characteristics of BCAs have only been described briefly in a few studies, particularly when compared to other PGTs (3,7).
Therefore, this study also conducted a differentiation between BCAs and other tumors, aiming to explore whether DKI and DCE-MRI alone or in combination can effectively differentiate the four types of tumors. This manuscript is written following the STARD reporting checklist (available at https://gs.amegroups.com/article/view/10.21037/gs-24-78/rc).
Methods
Patients
In this retrospective study, we reviewed the DKI and DCE-MRI imaging data from patients with PGTs between June 2018 and August 2022 that were available before the surgery. The exclusion criteria were (I) individuals whose largest tumors were below 5 mm in diameter; (II) MRI images with poor quality and motion artifacts; and (III) recurrent tumors. A total of 142 patients, including 68 males and 74 females, were collected, and PGTs were divided into two groups: BTs (n=98) and MTs (n=44). Based on pathological findings, and then all patients were divided into four groups: PAs (n=68), WTs (n=19), BCAs (n=11), and MTs (n=44).
This single-center retrospective investigation was conducted in accordance with the Declaration of Helsinki (as revised in 2013). The study was approved by the Ethics Committee of The First Affiliated Hospital of Zhengzhou University (No. 2019-KY-0015-001), and individual consent for this retrospective analysis was waived.
MRI acquisition protocols
All MRI examinations were conducted using a 3 T MRI scanner (MAGNETOM Skyra, Siemens Healthcare, Erlangen, Germany) with a 20-channel head and neck coil. The main scan parameters were axial/sagittal/coronal Dixon T2-weighted image (T2WI): repetition time (TR) =4,000–4,500 ms, echo time (TE) =82 ms, slice thickness =4.0 mm, and field of view (FOV) =230 mm × 230 mm; axial T1-weighted image (T1WI): TR =250 ms, TE =2.5 ms, slice thickness =4.0 mm, and FOV =230 mm × 230 mm; DKI: TR =5,690 ms, TE1 =69 ms, TE2 =105 ms, slice thickness =4.0 mm, FOV =240 mm × 240 mm, and b-values =0, 1,000, 1,600 s/mm2, and acquisition time =6 minutes; and axial/sagittal/coronal Dixon contrast-enhanced T1WI (CE-T1WI): TR =565–884 ms, TE =6.9 ms, slice thickness =4.0 mm, FOV =230 mm × 230 mm. Notably, DCE-MRI was performed after DKI. In addition, 0.1 mmol/kg gadobenate dimeglumine (Gd-DTPA; Magnevist, Bayer Healthcare, Berlin, Germany) was intravenously injected through the median cubital vein at 3 mL/s, followed by a 20-mL saline flush, with the following parameters: TR =4.93 ms, TE =1.93 ms, slice thickness =3.5 mm, and FOV =260 mm × 260 mm, flip angle =12°, temporal resolution =5.48 s. The acquisition time was 5 minutes 56 seconds.
Image processing and analysis
A Siemens post-processing workstation received the captured images for processing. DCE-MRI processing was conducted using a processing workstation (syngo MR Tissue4D, Siemens Healthcare). Motion correction, alignment, and processing were included in the post-processing procedure. The Diffusional Kurtosis Estimator (DKE; http://academicdepartments.musc.edu/cbi/dki/) and an additional research application software (MR Body Diffusion Toolbox, Siemens Healthcare) were used to process the DKI data. The following equation describes the DKI model:
where K is the diffusion kurtosis, and D is the diffusion coefficient of the non-Gaussian distribution. Relative K (rK) and relative D (rD) were calculated by the following formula:
Quantitative parameters [rate constant from extravascular extracellular space to plasma (Kep), transfer constant from plasma to extravascular extracellular space (Ktrans), initial area under curve (iAUC), and extravascular extracellular volume fraction (Ve)] were obtained from the DCE-MRI using the Tofts model. Relative Kep (rKep), relative Ktrans (rKtrans), relative iAUC (riAUC), and relative Ve (rVe) were calculated by the following formula:
To evaluate the DCE-MRI data, necrotic, cystic, and hemorrhagic areas within the tumor were avoided, and the values of the quantitative parameters were measured by placing a single region of interest (ROI) at the highest level of the tumor, specifically at the solid part of the tumor with a more uniform signal, then manually outlining the ROIs. Each tumor with an area of not less than 30 mm2 was measured by two radiologists (B.W. and Yan Zhang, each with over 8 years of experience in head and neck MRI), respectively, taking the average of both as the final value. The same method was used to measure the quantitative parameters of the contralateral normal gland. The radiologists were blinded to the pathology results. Finally, quantitative measurements from both readers were used to evaluate interobserver agreement.
Statistical analysis
MedCalc® Statistical Software version 20.022 (MedCalc Software Ltd., Ostend, Belgium; https://www.medcalc.org; 2021) and SPSS software version 26.0 were used for the statistical analyses. The study employed two-sided P values, and statistical significance was determined at a significance level of P<0.05. The normality and homogeneity of variance of all numerical data were tested using Kolmogorov-Smirnov’s and Levene’s tests. The means ± standard deviations (SDs) were used to convey normally distributed variables, while medians [interquartile ranges (IQRs)] were used to express non-normally distributed variables. The chi-squared test was used to compare the sex differences between benign tumors and MTs. In addition, the age difference between the two groups was compared using an independent samples t-test. The quantitative DCE-MRI and DKI characteristics and their relative values were compared between BTs and MTs using the Mann-Whitney U test. Depending on the normality distribution and homogeneity of variance of the quantitative parameters, the Kruskal-Wallis H test, Bonferroni-corrected post-hoc test, one-way analysis of variance (ANOVA), or post-hoc test by the least significant difference (LSD) method were used to analyze the quantitative parameters of DKI and DCE-MRI, and their relative values between the four groups, respectively.
The receiver operating characteristic (ROC) curve was created to determine the diagnostic performance and the best cutoff values for the quantitative parameters. Then, additional calculations for the area under the ROC curve (AUC), sensitivity, and specificity were made. Select the DKI and DCE-MRI parameters with the highest AUC value, use the logistic regression study to determine whether the combination of DKI and DCE-MRI will increase the discriminatory ability for parotid tumors.
The Delong test was used to examine comparisons of the AUCs. The intraclass correlation coefficient (ICC) with 95% confidence intervals (CIs) was calculated to assess the consistency of the two radiologists’ interpretations of the imaging. The ICC was evaluated as follows: r<0.50, poor; r=0.50–0.75, moderate; r=0.75–0.90, good; and r>0.90, excellent (22).
Results
One hundred and forty-two patients were reviewed, including benign (n=98) and malignant patients (n=44). And all patients were divided into four groups based on pathological findings: PAs (n=68), WTs (n=19), BCAs (n=11), and MTs (n=44). The mean age of the BTs (43.54±15.81 years) was significantly lower than that of the MTs (52.73±16.94 years) (P<0.05), and there was no significant difference between the two groups in terms of gender.
Statistical results showed that all parameter data were non-normally distributed except for the D value and age, and the variance of the Kep, Ve, rK, and riAUC values was not constant. In addition, the DKI and DCE-MRI parameters determined by the two readers were consistent, according to the ICC analysis, with the ICC ranging from 0.836 to 0.982. Figures 1-4 show representative cases of each of the four types of PGTs.
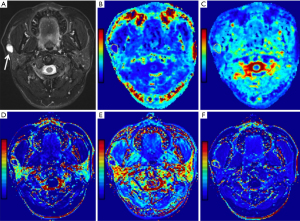
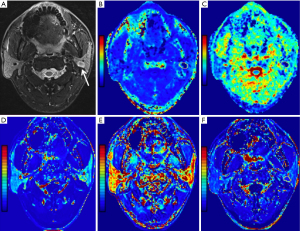
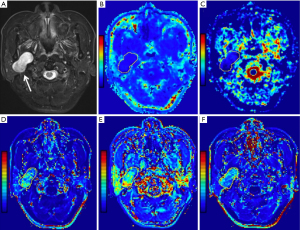
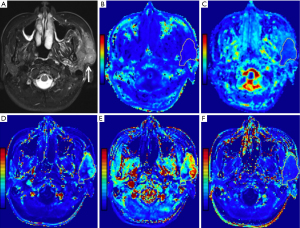
Parameter comparison of the benign tumors and MTs
The D value of the BTs [(1.65±0.44)×10−3 mm2/s] was substantially higher than that of the MTs [(1.21±0.44)×10−3 mm2/s], and the cutoff D value was 1.38×10−3 mm2/s (AUC, 0.76). The K value for the BTs was significantly lower than that of the MTs [0.55 (0.44, 0.72); P<0.001], and the cutoff value for the K value was 0.66 (AUC, 0.78). The rD value of the BTs [0.43 (0.10, 0.85)] was substantially higher than that of the MTs [0.06 (−0.20, 0.42)], and the cutoff rD value was 0.097 (AUC, 0.69). The rK value for the BTs [−0.51 (−0.65, −0.28)] was significantly lower than that of the MTs [−0.23 (−0.42, 0.17); P<0.001], and the cutoff value for the rK value was –0.444 (AUC, 0.74).
In addition, no DCE-MRI parameters were substantially different between them (all P>0.05). However, we found that BTs has a lower rKep value [−0.60 (−0.75, 0.12)] than MTs [−0.16 (−0.51, 0.22)], and the difference was significant (P=0.003).
Comparison of the DCE-MRI parameters of the four subtypes
Except for Ve (P=0.001), rVe (P=0.042), and riAUC (P=0.004), the Kruskal-Wallis H test revealed statistically significant differences in all quantitative parameters across the groups of PGTs (P<0.001). An overview of the analyses of the PGTs’ quantitative parameters and relative values are shown in Table 1. Whether the differences between the four subgroups reached difference can be observed in Table 2.
Table 1
Parameters | BT | MT | P value† | PA | WT | BCA | MT | P value‡ |
---|---|---|---|---|---|---|---|---|
K | 0.55 (0.44, 0.72) | 0.83 (0.70, 1.22) | <0.001 | 0.49 (0.39, 0.63) | 1.01 (0.91, 1.28) | 0.61 (0.58, 0.87) | 0.83 (0.70, 1.22) | <0.001 |
rK | −0.51 (−0.65, −0.28) | −0.23 (−0.42, 0.17) | <0.001 | −0.53 (−0.67, −0.39) | −0.15 (−0.37, 0.13) | −0.49 (−0.61, −0.35) | −0.23 (−0.42, 0.17) | <0.001 |
D (×10−3mm2/s) | 1.65±0.44 | 1.21±0.44 | <0.001 | 1.77±0.40 | 1.20±0.37 | 1.71±0.29 | 1.21±0.44 | <0.001 |
rD | 0.43 (0.10, 0.85) | 0.06 (−0.20, 0.42) | <0.001 | 0.54 (0.16, 1.04) | 0.09 (−0.13, 0.36) | 0.62 (0.28, 0.63) | 0.06 (−0.20, 0.42) | <0.001 |
Ktrans (min−1) | 0.16 (0.08, 0.35) | 0.17 (0.10, 0.31) | 0.48 | 0.09 (0.06, 0.20) | 0.43 (0.20, 0.83) | 0.41 (0.16, 0.49) | 0.17 (0.10, 0.31) | <0.001 |
rKtrans | 0.17 (−0.53, 2.10) | 0.65 (−0.33, 3.36) | 0.19 | −0.24 (−0.64, 1.00) | 2.04 (−0.22, 6.71) | 1.20 (0.54, 3.18) | 0.65 (−0.33, 3.36) | <0.001 |
Kep (min−1) | 0.46 (0.26, 1.32) | 0.71 (0.52, 1.28) | 0.07 | 0.32 (0.22, 0.53) | 2.31 (1.04, 4.22) | 1.60 (1.26, 2.70) | 0.71 (0.52, 1.28) | <0.001 |
rKep | −0.60 (−0.75, 0.12) | −0.16 (−0.51, 0.22) | 0.003 | −0.71 (−0.81, −0.56) | 1.07 (0.03, 4.38) | 0.83 (0.07, 1.27) | −0.16 (−0.51, 0.22) | <0.001 |
Ve | 0.27 (0.20, 0.41) | 0.25 (0.17, 0.33) | 0.14 | 0.31 (0.24, 0.49) | 0.18 (0.14, 0.22) | 0.21 (0.07, 0.35) | 0.25 (0.17, 0.33) | 0.001 |
rVe | 0.85 (0.11, 3.31) | 0.99 (−0.19, 3.73) | 0.68 | 1.41 (0.12, 4.99) | 0.59 (−0.38, 1.14) | 0.64 (0.35, 0.93) | 0.99 (−0.19, 3.73) | 0.042 |
iAUC (mmol·s/kg) | 0.21 (0.12, 0.38) | 0.26 (0.15, 0.44) | 0.18 | 0.15 (0.09, 0.26) | 0.33 (0.25, 0.40) | 0.38 (0.20, 0.47) | 0.26 (0.15, 0.44) | <0.001 |
riAUC | 0.28 (−0.43, 1.72) | 1.14 (−0.31, 3.10) | 0.055 | −0.77 (−0.64, 1.05) | 1.32 (−0.36, 2.04) | 1.10 (0.59, 2.05) | 1.14 (−0.31, 3.10) | 0.004 |
The normally distributed variables are expressed as mean ± SD, and non-normally distributed variables are expressed as median (IQR). †, P values from independent t-test/Mann-Whitney U test; ‡, P values Kruskal-Wallis H test or from one-way analysis of variance. DKI, diffusion kurtosis imaging; DCE-MRI, dynamic contrast-enhanced magnetic resonance imaging; PGT, parotid gland tumor; BT, benign tumors; MT, malignant tumor; PA, pleomorphic adenoma; WT, Warthin tumor; BCA, basal cell adenoma; K, diffusion kurtosis; rK, relative diffusion kurtosis; D, diffusion coefficient; rD, relative diffusion coefficient; Ktrans, transfer constant from plasma to extravascular extracellular space; rKtrans, relative transfer constant from plasma to extravascular extracellular space; Kep, rate constant from extravascular extracellular space to plasma; rKep, relative rate constant from extravascular extracellular space to plasma; Ve, extravascular extracellular volume fraction; rVe, relative extravascular extracellular volume fraction; iAUC, initial area under curve; riAUC, relative initial area under curve; SD, standard deviation; IQR, interquartile range.
Table 2
Subgroups | DKI parameters, P value | DCE-MRI parameters, P value | |||||||||||
---|---|---|---|---|---|---|---|---|---|---|---|---|---|
K | rK | D (×10−3 mm2/s) | rD | Ktrans (min−1) | rKtrans | Kep (min−1) | rKep | Ve | rVe | iAUC (mmol·s/kg) | riAUC | ||
PA vs. WT | <0.001 | <0.001 | <0.001 | 0.007 | <0.001 | 0.005 | <0.001 | <0.001 | 0.001 | 0.043 | 0.001 | 0.12 | |
PA vs. BCA | 0.12 | >0.99 | 0.66 | >0.99 | <0.001 | 0.02 | <0.001 | <0.001 | 0.78 | >0.99 | 0.008 | 0.09 | |
PA vs. MT | <0.001 | <0.001 | <0.001 | <0.001 | 0.03 | 0.048 | <0.001 | <0.001 | 0.049 | >0.99 | 0.03 | 0.03 | |
WT vs. BCA | 0.30 | 0.17 | <0.001 | 0.15 | >0.99 | >0.99 | >0.99 | >0.99 | >0.99 | >0.99 | >0.99 | >0.99 | |
WT vs. MT | >0.99 | >0.99 | 0.89 | >0.99 | 0.01 | >0.99 | 0.01 | 0.055 | 0.54 | 0.66 | 0.69 | >0.99 | |
BCA vs. MT | 0.52 | 0.07 | <0.001 | 0.09 | 0.14 | >0.99 | 0.03 | 0.22 | >0.99 | >0.99 | 0.85 | >0.99 |
Statistical significance was set at P<0.05. PGT, parotid gland tumor; DKI, diffusion kurtosis imaging; DCE-MRI, dynamic contrast-enhanced magnetic resonance imaging; K, diffusion kurtosis; rK, relative diffusion kurtosis; D, diffusion coefficient; rD, relative diffusion coefficient; Ktrans, transfer constant from plasma to extravascular extracellular space; rKtrans, relative transfer constant from plasma to extravascular extracellular space; Kep, rate constant from extravascular extracellular space to plasma; rKep, relative rate constant from extravascular extracellular space to plasma; Ve, extravascular extracellular volume fraction; rVe, relative extravascular extracellular volume fraction; iAUC, initial area under curve; riAUC, relative initial area under curve; PA, pleomorphic adenoma; WT, Warthin tumor; BCA, basal cell adenoma; MT, malignant tumor.
Compared to MTs, BCAs, and WTs, the Ktrans values [0.09 (0.06, 0.20) min−1], rKtrans values [−0.24 (−0.64, 1.00)], Kep values [0.32 (0.22, 0.53) min−1], rKep values [−0.71 (−0.81, −0.56)] and iAUC values [0.15 (0.09, 0.26) mmol·s/kg] of PAs were considerably lower (all corrected P<0.05). In addition, as shown in Figures 1-4, both Ktrans and Kep maps derived from DCE-MRI demonstrated low Kep and Ktrans values of PA compared to other types of PGTs. Compared to WTs and MTs, the Ve values of PAs [0.31 (0.24, 0.49)] were considerably higher (all corrected P<0.05).
In addition, the Ktrans values for MTs were substantially lower [0.17 (0.10, 0.31) min−1] than those for WTs (adjusted P=0.01). The Kep values for MTs [0.71 (0.52, 1.28) min−1] were substantially lower (all corrected P<0.05) than those for WTs and BCAs. Figures 2-4 show that mucoepidermoid carcinoma had a lower Kep value than WTs and BCAs.
Comparison of DKI parameters of the four subtypes
We found that the K value and rK value of PAs were significantly lower than those of the MTs and WTs (all corrected P<0.001). The D values of PAs and BCAs were significantly higher than those of WTs and MTs (all corrected P<0.001). And the rD value of PAs was significantly higher than those of WTs and MTs (all corrected P<0.05). However, the D and K values, even their relative values of PAs and BCAs did not differ significantly.
Diagnostic results comparing the groups
Optimal cutoff values and diagnostic performance of DKI and DCE-MRI parameters for differentiating four groups of PGTs are shown in Tables 3,4, respectively. The ROC analyses showed that the rKep’s cutoff value of −0.329 (AUC, 0.957) demonstrated the best excellent diagnostic ability in separating PAs from WTs, superior to other quantitative parameters and relative values. In addition, the optimal diagnostic performance was demonstrated by the Kep’s cutoff value of 0.988 min−1 in discriminating BCAs from MTs (AUC, 0.874).
Table 3
Parameters | Cutoff value | AUC (95% CI) | Sensitivity (%) | Specificity (%) |
---|---|---|---|---|
PAs vs. WTs | ||||
D | 1.450 | 0.847 (0.754–0.915) | 80.88 | 78.95 |
rD | 0.376 | 0.742 (0.637–0.830) | 63.24 | 89.47 |
K | 0.890 | 0.872 (0.783–0.934) | 100.00 | 78.95 |
rK | −0.195 | 0.788 (0.688–0.869) | 92.65 | 63.16 |
PAs vs. MTs | ||||
D | 1.380 | 0.818 (0.734–0.884) | 88.24 | 65.91 |
rD | 0.440 | 0.729 (0.637–0.809) | 57.35 | 79.55 |
K | 0.660 | 0.896 (0.824–0.945) | 83.82 | 84.09 |
rK | −0.444 | 0.809 (0.724–0.877) | 72.06 | 79.55 |
WTs vs. BCAs | ||||
D | 1.35 | 0.861 (0.686–0.960) | 68.42 | 90.91 |
BCAs vs. MTs | ||||
D | 1.39 | 0.833 (0.708–0.920) | 90.91 | 68.18 |
DKI, diffusion kurtosis imaging; PGT, parotid gland tumor; AUC, area under the receiver operating characteristic curve; CI, confidence interval; PA, pleomorphic adenoma; WT, Warthin tumor; D, diffusion coefficient; rD, relative diffusion coefficient; K, diffusion kurtosis; rK, relative diffusion kurtosis; MT, malignant tumor; BCA, basal cell adenoma.
Table 4
Parameters | Cutoff value | AUC (95% CI) | Sensitivity (%) | Specificity (%) |
---|---|---|---|---|
PAs vs. WTs | ||||
Ktrans | 0.184 | 0.882 (0.795–0.941) | 72.06 | 94.74 |
rKtrans | 1.410 | 0.745 (0.640–0.832) | 85.29 | 57.89 |
Kep | 0.680 | 0.942 (0.870–0.981) | 91.18 | 89.47 |
rKep | −0.329 | 0.957 (0.890–0.989) | 92.65 | 89.47 |
Ve | 0.219 | 0.779 (0.677–0.861) | 82.35 | 78.95 |
rVe | 1.471 | 0.700 (0.593–0.794) | 50.00 | 94.74 |
iAUC | 0.250 | 0.802 (0.703–0.880) | 75.00 | 84.21 |
PAs vs. BCAs | ||||
Ktrans | 0.147 | 0.881 (0.789–0.943) | 67.65 | 100.00 |
rKtrans | 0.165 | 0.798 (0.693–0.880) | 61.76 | 90.91 |
Kep | 0.849 | 0.969 (0.903–0.995) | 94.12 | 100.00 |
rKep | −0.329 | 0.980 (0.920–0.998) | 92.65 | 100.00 |
iAUC | 0.171 | 0.799 (0.694–0.881) | 58.82 | 100.00 |
PAs vs. MTs | ||||
Ktrans | 0.095 | 0.667 (0.572–0.754) | 54.41 | 77.27 |
rKtrans | 1.288 | 0.647 (0.551–0.735) | 83.82 | 43.18 |
Kep | 0.490 | 0.784 (0.697–0.857) | 72.06 | 79.55 |
rKep | −0.471 | 0.851 (0.771–0.911) | 85.23 | 75.00 |
Ve | 0.283 | 0.651 (0.555–0.738) | 55.88 | 70.45 |
iAUC | 0.282 | 0.654 (0.558–0.741) | 82.35 | 50.00 |
riAUC | 1.205 | 0.653 (0.557–0.741) | 79.41 | 50.00 |
WTs vs. MTs | ||||
Ktrans | 0.345 | 0.767 (0.643–0.864) | 63.16 | 84.09 |
Kep | 1.916 | 0.815 (0.697–0.901) | 57.89 | 97.73 |
BCAs vs. MTs | ||||
Kep | 0.988 | 0.874 (0.757–0.948) | 100.00 | 68.18 |
DCE-MRI, dynamic contrast-enhanced magnetic resonance imaging; PGT, parotid gland tumor; AUC, area under the receiver operating characteristic curve; CI, confidence interval; PA, pleomorphic adenoma; WT, Warthin tumor; Ktrans, transfer constant from plasma to extravascular extracellular space; rKtrans, relative transfer constant from plasma to extravascular extracellular space; Kep, rate constant from extravascular extracellular space to plasma; rKep, relative rate constant from extravascular extracellular space to plasma; Ve, extravascular extracellular volume fraction; rVe, relative extravascular extracellular volume fraction; iAUC, initial area under curve; riAUC, relative initial area under curve; BCA, basal cell adenoma; MT, malignant tumor.
rKep’s cutoff value of −0.329 (AUC, 0.980) demonstrated the ideal diagnostic performance was equivalent to Kep (AUC, 0.969) in distinguishing PAs from BCAs, superior to Ktrans, rKtrans, and iAUC (both P<0.05). In addition, the Kep’s cutoff value of 1.916 min−1 (AUC, 0.815) demonstrated a higher AUC than Ktrans when separating WTs from MTs, although there was no statistically significant difference between the AUCs of Kep and Ktrans.
The K value demonstrated superior diagnostic efficacy in separating PAs from MTs, outperforming other parameters. Furthermore, the D value’s cutoff value of 1.35×10−3 mm2/s (AUC, 0.861) demonstrated the best diagnostic performance for separating BCAs from WTs. Besides, only D value can only divide WTs and BCAs. Figures 2,3 demonstrate how the WT has a lower D value than BCAs.
Comparison of the combination of DKI and DCE-MRI parameters of the four subtypes
Based on Tables 3,4, we selected the DKI and DCE-MRI parameters with higher diagnostic capability between each pair of tumor groups. By using logistic regression, we calculated whether the combination of DKI and DCE-MRI parameters could improve the diagnostic ability of DKI or DCE-MRI when used alone, and thus plotted Figure 5. When comparing PAs and WTs, K value (AUC, 0.872) and rKep value (AUC, 0.957) demonstrated excellent diagnostic ability. The combination of these two parameters (AUC, 0.986) further enhanced their discriminatory power between PAs and WTs, although no significant difference was reached. The K value (AUC, 0.896) and rKep value (AUC, 0.851) showed strong diagnostic ability when comparing PAs and MTs. Their discriminating strength between PAs and MTs was further enhanced by the combination of K and rKep value (AUC, 0.915), and it was significantly higher than the discriminatory ability of rKep alone (P=0.02). Excellent diagnostic capacity was demonstrated by D value (AUC, 0.833) and Kep value (AUC, 0.874) when comparing BCAs and MTs. Even if there was not a discernible difference, the combination of these two factors (AUC, 0.909) improved their ability to distinguish between BCAs and MTs. Nevertheless, only one of the DKI or DCE-MRI parameters can be utilized to differentiate between any other two types of tumors.
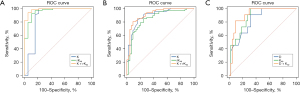
Discussion
Distinct types of PGTs have different treatment philosophies and prognoses. Low-grade MTs are challenging to identify from benign tumors by physical characteristics of conventional MRI alone. Studies have shown that combining diffusion-weighted imaging (DWI) and DCE-MRI sequences aids in detecting PGTs (5,18,23-25). DWI is based on the hypothetical model of a Gaussian distribution of water molecule motion in tissue, which is non-Gaussian. As a modified version of the DWI technique, DKI is based on a non-Gaussian distribution of water molecule motion. The hypothetical model based on the non-Gaussian distribution of water molecule mobility would offer more useful data to identify parotid malignancies. However, to our knowledge, no studies have used DKI or DCE-MRI to distinguish BCAs from other PGTs. Therefore, this study aimed to evaluate the benefits of using DKI and DCE-MRI to distinguish four types of PGTs, including BCAs.
In the actual measurement process of DKI and DCE-MRI parameters, it is often affected by individual differences, basal metabolism and other factors. A previous article used the proximity of the lesion to the gland as a reference and found that normalized apparent diffusion coefficient (ADC) was easier to distinguish benign from malignant parotid tumors than ADC (26). So far, we have not found any articles that studied the relative values of DKI and DCE-MRI parameters in PGTs. Therefore, the concept of relative value was introduced in this study. The contralateral normal gland of the same patient was used as a reference to calculate the relative values of each parameter, so as to improve the stability of the diagnostic ability of DKI and DCE-MRI parameters.
The primary results of our research revealed that only the combination of DKI and DCE-MRI parameters could reliably distinguish BCAs from other PGTs. No DKI parameters could reliably separate BCAs from PAs and no DCE-MRI parameters could reliably distinguish BCAs from WTs. Therefore, we investigated the diagnostic effectiveness of using DCE-MRI and DKI parameters separately or together to differentiate PGTs.
We found stark contrasts in the D values between BTs and MTs; either WTs or MTs had lower D values than PAs and BCAs. The findings of earlier investigations are inconsistent on whether D values can distinguish BTs and MTs in the parotid gland (20,21). The larger sample sizes of PAs and BCAs in our study may have impacted the difference in D values between MTs and BTs, because we discovered that D values could be utilized to distinguish MTs from PAs and BCAs. Tumor cell density and the nuclear-cytoplasmic ratio have a substantial negative connection with the D values derived by DKI, which are non-Gaussian-biased adjusted fusion correlation coefficients (27).
Our results showed that either WTs or MTs had lower D values than PAs, consistent with prior investigations (21). Furthermore, we discovered WTs or MTs had lower D values than BCAs, and it was impossible to differentiate between PAs and BCAs by using D values. This finding has not previously been reported. Interestingly, the low D value appeared likely because WTs contain an abundance of densely arranged small lymphocytes, and MTs are characterized by much cell proliferation (28-30). The high D values may have been due to the rich myxoid and chondroid matrices in PAs. The interstitial tissue of some pathological types of BCAs may show edema due to dilated lymphatic and tiny blood vessels, exhibiting high D values in our study (20,31). Upon comparing the four subgroups, the D values could be used to distinguish PAs and BCAs from WTs and MTs, indicating that D values aid in differentiating between parotid tumor subtypes.
K values are associated with cell heterogeneity and tissue complexity (30). We found that the K values were considerably greater for MTs than for BTs, consistent with Yu et al. (19), but inconsistent with Huang et al. (21). However, when comparing the four subgroups, the K values could not distinguish BCAs from other PGTs, suggesting that K values did not help distinguish BCAs. One possible explanation is that different pathological types of BCAs have different proportions of cystic or hemorrhagic elements within them, affecting their K values (32).
In our study, no DCE-MRI quantitative parameters could reliably discriminate between BTs and MTs, consistent with earlier research by Huang et al. (21). However, we found that the rKep value for the BTs was significantly lower than that of the MTs, which has not been reported previously. This maybe because the use of relative values eliminates individual effects as much as possible, thereby increasing the stability of Kep’s ability to discriminate between tumor categories.
In addition, we found that PAs had the lowest mean Ktrans and Kep, consistent with a study by Xu et al. (17). This finding suggested that the mean Ktrans and Kep could be used to distinguish PAs from other tumors. In addition, we also found that PAs had the lowest mean rKtrans and rKep values. And PAs had the lowest K value than WTs and MTs. To increase the diagnostic accuracy, we found that combining the analyzed parameters (K and rKep values) could improve PAs diagnosis, and it showed that the use of combined values can improve the discriminatory ability to some extent. Similarly, when comparing BCAs and MTs, the use of combined values (D and Kep values) also improved the discriminatory ability.
Moreover, in our investigation, lower Kep values helped differentiate MTs from BCAs and WTs, suggesting that DCE-MRI characteristics assisted in differentiating PGTs. However, we also found it challenging to distinguish between BCAs and WTs using any DCE-MRI features, making it necessary to combine DKI parameters for doing so. To our knowledge, Ktrans and Kep, as DCE-MRI pharmacokinetic parameters, increase with microvascular blood flow, vascular permeability, and microvascular density in the sick tissue (33). Histologically, most vascular channels with small capillaries and veins are prominent in BCAs. In contrast, the heterogeneous distribution of vessels and a small extracellular and extravascular space in WTs could explain their similar Ktrans and Kep values (34-36).
There are some limitations in this study. First, despite the fact that the existing DKI technique collected more parameters, the scanning time was lengthy. The scanning time could be somewhat decreased with ongoing technological updating and advancement. Second, future BCA studies should address the many pathological types of tumors in groups because the sample size of different pathological BCA subtypes may affect their overall DKI and DCE-MRI parameters. Last, a study with a more evenly distributed sample size is required to validate our findings because the sample sizes for each subtype in our study were not sufficient.
Conclusions
In summary, DKI and DCE-MRI showed good diagnostic performance in the differential diagnosis of different PGT types. In addition, the combined use of DKI and DCE-MRI parameters is more advantageous in distinguishing BCAs from other tumors, and the relative values of DKI and DCE-MRI parameters can also improve their diagnostic ability for PGTs.
Acknowledgments
We express our gratitude to all individuals who have provided assistance and support throughout the course of this study. Their contributions have been invaluable and greatly appreciated. Parts of the manuscript was presented as an EPOS Radiologist (scientific) at the European Congress Radiology (ECR) 2024 annual meeting.
Funding: This research was supported by
Footnote
Reporting Checklist: The authors have completed the STARD reporting checklist. Available at https://gs.amegroups.com/article/view/10.21037/gs-24-78/rc
Data Sharing Statement: Available at https://gs.amegroups.com/article/view/10.21037/gs-24-78/dss
Peer Review File: Available at https://gs.amegroups.com/article/view/10.21037/gs-24-78/prf
Conflicts of Interest: All authors have completed the ICMJE uniform disclosure form (available at https://gs.amegroups.com/article/view/10.21037/gs-24-78/coif). F.Q. and Y.W. are employees of Siemens Healthineer Ltd. R.G. is an employee of Siemens Healthcare GmbH. The other authors have no conflicts of interest to declare.
Ethical Statement: The authors are accountable for all aspects of the work in ensuring that questions related to the accuracy or integrity of any part of the work are appropriately investigated and resolved. This single-center retrospective investigation was conducted in accordance with the Declaration of Helsinki (as revised in 2013). The study was approved by the Ethics Committee of The First Affiliated Hospital of Zhengzhou University (No. 2019-KY-0015-001), and individual consent for this retrospective analysis was waived.
Open Access Statement: This is an Open Access article distributed in accordance with the Creative Commons Attribution-NonCommercial-NoDerivs 4.0 International License (CC BY-NC-ND 4.0), which permits the non-commercial replication and distribution of the article with the strict proviso that no changes or edits are made and the original work is properly cited (including links to both the formal publication through the relevant DOI and the license). See: https://creativecommons.org/licenses/by-nc-nd/4.0/.
References
- Santana BW, Silva LP, Serpa MS, et al. Incidence and profile of benign epithelial tumors of salivary glands from a single center in Northeast of Brazil. Med Oral Patol Oral Cir Bucal 2021;26:e108-13. [Crossref] [PubMed]
- Inaka Y, Kawata R, Haginomori SI, et al. Symptoms and signs of parotid tumors and their value for diagnosis and prognosis: a 20-year review at a single institution. Int J Clin Oncol 2021;26:1170-8. [Crossref] [PubMed]
- Murayama Y, Kamitani T, Sagiyama K, et al. Evaluation of MR imaging findings differentiating parotid basal cell adenomas from other parotid tumors. Eur J Radiol 2021;144:109980. [Crossref] [PubMed]
- Khalek Abdel Razek AA. Characterization of salivary gland tumours with diffusion tensor imaging. Dentomaxillofac Radiol 2018;47:20170343. [Crossref] [PubMed]
- Gökçe E. Multiparametric Magnetic Resonance Imaging for the Diagnosis and Differential Diagnosis of Parotid Gland Tumors. J Magn Reson Imaging 2020;52:11-32. [Crossref] [PubMed]
- Hellquist H, Paiva-Correia A, Vander Poorten V, et al. Analysis of the Clinical Relevance of Histological Classification of Benign Epithelial Salivary Gland Tumours. Adv Ther 2019;36:1950-74. [Crossref] [PubMed]
- Chen G, Wen X, Chen XJ, et al. Imaging Features and Pathological Analysis of 43 Parotid Basal Cell Adenomas. Comput Math Methods Med 2021;2021:7906058. [Crossref] [PubMed]
- Lee DH, Jung EK, Lee JK, et al. Comparative analysis of benign and malignant parotid gland tumors: A retrospective study of 992 patients. Am J Otolaryngol 2023;44:103690. [Crossref] [PubMed]
- Gudmundsson JK, Ajan A, Abtahi J. The accuracy of fine-needle aspiration cytology for diagnosis of parotid gland masses: a clinicopathological study of 114 patients. J Appl Oral Sci 2016;24:561-7. [Crossref] [PubMed]
- Shah KS, Ethunandan M. Tumour seeding after fine-needle aspiration and core biopsy of the head and neck--a systematic review. Br J Oral Maxillofac Surg 2016;54:260-5. [Crossref] [PubMed]
- Orhan Soylemez UP, Atalay B. Differentiation of Benign and Malignant Parotid Gland Tumors with MRI and Diffusion Weighted Imaging. Medeni Med J 2021;36:138-45. [Crossref] [PubMed]
- Baohong W, Jing Z, Zanxia Z, et al. T2 mapping and readout segmentation of long variable echo-train diffusion-weighted imaging for the differentiation of parotid gland tumors. Eur J Radiol 2022;151:110265. [Crossref] [PubMed]
- Wang Y, Wang L, Huang H, et al. Amide proton transfer-weighted magnetic resonance imaging for the differentiation of parotid gland tumors. Front Oncol 2023;13:1223598. [Crossref] [PubMed]
- Chen Y, Huang N, Zheng Y, et al. Characterization of parotid gland tumors: Whole-tumor histogram analysis of diffusion weighted imaging, diffusion kurtosis imaging, and intravoxel incoherent motion - A pilot study. Eur J Radiol 2024;170:111199. [Crossref] [PubMed]
- Patella F, Franceschelli G, Petrillo M, et al. A multiparametric analysis combining DCE-MRI- and IVIM -derived parameters to improve differentiation of parotid tumors: a pilot study. Future Oncol 2018;14:2893-903. [Crossref] [PubMed]
- Patella F, Sansone M, Franceschelli G, et al. Quantification of heterogeneity to classify benign parotid tumors: a feasibility study on most frequent histotypes. Future Oncol 2020;16:763-78. [Crossref] [PubMed]
- Xu Z, Zheng S, Pan A, et al. A multiparametric analysis based on DCE-MRI to improve the accuracy of parotid tumor discrimination. Eur J Nucl Med Mol Imaging 2019;46:2228-34. [Crossref] [PubMed]
- Stoia S, Lenghel M, Dinu C, et al. The Value of Multiparametric Magnetic Resonance Imaging in the Preoperative Differential Diagnosis of Parotid Gland Tumors. Cancers (Basel) 2023;15:1325. [Crossref] [PubMed]
- Yu S, Zhang Z, Bao Q, et al. Diffusion kurtosis imaging in the differential diagnosis of parotid gland disease and parotid adenolymphoma: preliminary results. Dentomaxillofac Radiol 2018;47:20170388. [Crossref] [PubMed]
- Qian W, Xu XQ, Zhu LN, et al. Preliminary study of using diffusion kurtosis imaging for characterizing parotid gland tumors. Acta Radiol 2019;60:887-94. [Crossref] [PubMed]
- Huang N, Chen Y, She D, et al. Diffusion kurtosis imaging and dynamic contrast-enhanced MRI for the differentiation of parotid gland tumors. Eur Radiol 2022;32:2748-59. [Crossref] [PubMed]
- Koo TK, Li MY. A Guideline of Selecting and Reporting Intraclass Correlation Coefficients for Reliability Research. J Chiropr Med 2016;15:155-63. Erratum in: J Chiropr Med 2017;16:346. [Crossref] [PubMed]
- Coudert H, Mirafzal S, Dissard A, et al. Multiparametric magnetic resonance imaging of parotid tumors: A systematic review. Diagn Interv Imaging 2021;102:121-30. [Crossref] [PubMed]
- Piludu F, Marzi S, Ravanelli M, et al. MRI-Based Radiomics to Differentiate between Benign and Malignant Parotid Tumors With External Validation. Front Oncol 2021;11:656918. [Crossref] [PubMed]
- Tao X, Yang G, Wang P, et al. The value of combining conventional, diffusion-weighted and dynamic contrast-enhanced MR imaging for the diagnosis of parotid gland tumours. Dentomaxillofac Radiol 2017;46:20160434. [Crossref] [PubMed]
- Zhang W, Zuo Z, Huang X, et al. Value of Diffusion-Weighted Imaging Combined with Susceptibility-Weighted Imaging in Differentiating Benign from Malignant Parotid Gland Lesions. Med Sci Monit 2018;24:4610-6. [Crossref] [PubMed]
- Xiao Z, Tang Z, Qiang J, et al. Differentiation of olfactory neuroblastomas from nasal squamous cell carcinomas using MR diffusion kurtosis imaging and dynamic contrast-enhanced MRI. J Magn Reson Imaging 2018;47:354-61. [Crossref] [PubMed]
- Munhoz L, Ramos EADA. Application of diffusion-weighted magnetic resonance imaging in the diagnosis of salivary gland diseases: a systematic review. Oral Surg Oral Med Oral Pathol Oral Radiol 2019;128:280-310. [Crossref] [PubMed]
- Yamamoto T, Kimura H, Hayashi K, et al. Pseudo-continuous arterial spin labeling MR images in Warthin tumors and pleomorphic adenomas of the parotid gland: qualitative and quantitative analyses and their correlation with histopathologic and DWI and dynamic contrast enhanced MRI findings. Neuroradiology 2018;60:803-12. [Crossref] [PubMed]
- Ma G, Xu XQ, Hu H, et al. Utility of Readout-Segmented Echo-Planar Imaging-Based Diffusion Kurtosis Imaging for Differentiating Malignant from Benign Masses in Head and Neck Region. Korean J Radiol 2018;19:443-51. [Crossref] [PubMed]
- Nagao K, Matsuzaki O, Saiga H, et al. Histopathologic studies of basal cell adenoma of the parotid gland. Cancer 1982;50:736-45. [Crossref] [PubMed]
- Mukai H, Motoori K, Horikoshi T, et al. Basal cell adenoma of the parotid gland; MR features and differentiation from pleomorphic adenoma. Dentomaxillofac Radiol 2016;45:20150322. [Crossref] [PubMed]
- Ahn SJ, An CS, Koom WS, et al. Correlations of 3T DCE-MRI quantitative parameters with microvessel density in a human-colorectal-cancer xenograft mouse model. Korean J Radiol 2011;12:722-30. [Crossref] [PubMed]
- Shi L, Wang YX, Yu C, et al. CT and ultrasound features of basal cell adenoma of the parotid gland: a report of 22 cases with pathologic correlation. AJNR Am J Neuroradiol 2012;33:434-8. [Crossref] [PubMed]
- Tofts PS, Brix G, Buckley DL, et al. Estimating kinetic parameters from dynamic contrast-enhanced T(1)-weighted MRI of a diffusable tracer: standardized quantities and symbols. J Magn Reson Imaging 1999;10:223-32. [Crossref] [PubMed]
- Parwani AV, Ali SZ. Diagnostic accuracy and pitfalls in fine-needle aspiration interpretation of Warthin tumor. Cancer 2003;99:166-71. [Crossref] [PubMed]