Combing superb microvascular imaging with shear wave elastography for risk stratification of Thyroid Imaging Reporting and Data System (TI-RADS) 4 thyroid nodules
Highlight box
Key findings
• In this study, we used multimodal ultrasound imaging, including the conventional ultrasound (US), superb microvascular imaging (SMI), and shear wave elastography (SWE) to establish a dynamic nomogram for the risk assessment of Thyroid Imaging Reporting and Data System (TI-RADS) 4 thyroid nodules. The nomogram showed good performance in both training and verification sets and could provide significant risk stratification in TI-RADS 4 thyroid nodules, which can assist clinical decision-making and reduce unnecessary needle biopsies.
What is known and what is new?
• Fine-needle aspiration (FNA) pathological result is still one of the gold standards for diagnosing benign and malignant thyroid nodules, but it is invasive for patients. Due to the difficulty of the US in accurately diagnosing the benign and malignant nature of TI-RADS 4 thyroid nodules, many unnecessary FNA have been made.
• Although previous studies have reported that either SMI or SWE could improve the diagnostic accuracy of TI-RADS 4 thyroid nodules, the predictive value of combining SMI with SWE on TI-RADS 4 thyroid nodules remains elusive. This study aimed to explore the potential value of SMI and SWE on the risk of TI-RADS 4 thyroid nodule, thereby establishing a dynamic nomogram to present a precise and useful tool.
What is the implication, and what should change now?
• The dynamic nomogram could accurately stratify the malignancy risk of TI-RADS 4 thyroid nodules to assist clinical decision-making.
Introduction
In recent years, the incidence rate of thyroid nodules has increased significantly due to the improvement of detection technology (1,2). Conventional ultrasound (US) remains the mainstay in detection and diagnosis of thyroid nodules, and the Thyroid Imaging Reporting and Data System (TI-RADS), proposed by the American College of Radiology (ACR) in 2017, has been established based on the ultrasonographic features and has proposed five categories to stratify risk of malignancy of thyroid nodules (3). TI-RADS 4 and TI-RADS 5 represent moderately suspicious nodules and highly suspicious ones, respectively. It is difficult to accurately assess the risk of TI-RADS 4 thyroid nodules, due to the fact that the probability of TI-RADS 4 nodules being malignant is 5–20%, which leads to overlapping of benign and malignant US features of nodules (4-6). At present, fine-needle aspiration (FNA) is still one of the commonly used methods for the diagnosis of suspected thyroid nodules, but it is invasive for patients and sometimes the results are inaccurate (7,8). Therefore, it is essential to assess the risk of TI-RADS 4 nodules to reduce unnecessary FNA.
Angiogenesis has been underlined in tumor growth, invasion, and distant metastasis (9). Assessment of lesion blood flow characteristics is widely utilized in the diagnosis of various tumors (10,11). Color doppler flow imaging (CDFI) is widely used for detecting blood flow in lesions, but it lacks sensitivity in identifying small blood vessels and slow blood flow, thus limiting its ability to recognize malignant thyroid nodules (2,12). Superb microvascular imaging (SMI) as an innovative technique could visualize the tumor microvascular blood flow without clutter using an advanced algorithm, and utilizing new algorithms and enhanced wall filters to effectively preserve tiny blood flow signals (10,13). Prior research has shown that SMI provides a significantly better depiction of blood flow characteristics within lesions than CDFI (14,15). In addition to the characteristic of blood flow, the progression of tumors is often accompanied by changes in tissue pathological structure, with malignant lesions typically exhibiting greater stiffness than benign lesions (16). Shear wave elastography (SWE) has emerged as a non-invasive tool in evaluating lesion stiffness to provide additional information in differentiating benign and malignant thyroid nodules (17). Previous research has shown that combining SMI and SWE can enhance the diagnostic performance for distinguishing between benign and malignant breast lesions, indicating their potential complementarity in aiding physicians to evaluate lesions more comprehensively (18). However, to our knowledge, there is currently very limited research in the field of thyroid nodules on this matter.
In this study, we aimed to explore the potential value of SMI and SWE on the risk prediction of TI-RADS 4 thyroid nodule, thereby establishing a dynamic nomogram to present a precise and useful tool. We present this article in accordance with the TRIPOD reporting checklist (available at https://gs.amegroups.com/article/view/10.21037/gs-24-87/rc).
Methods
Patients
The study was conducted in accordance with the Declaration of Helsinki (as revised in 2013). This retrospective study was approved by the ethics committee of Beijing Tongren Hospital (No. TREC2022-KY138). This study only analyzes retrospective images, so no additional clinical risks are imposed on the patients during this process, and patient data is kept strictly confidential. Therefore, individual consent for this study was waived.
Patients who underwent FNA of thyroid nodules in our hospital from January 2020 to September 2022 were retrospectively evaluated from the institutional database. Patients were enrolled according to the following inclusion criteria: (I) all thyroid nodules had histopathological results; (II) US, SMI, and SWE images were complete. Exclusion criteria: (I) poor image quality; (II) presence of following-up lose. A total of 248 patients (mean age 44.19±12.35 years; range, 24–73 years) with solitary TI-RADS 4 thyroid nodules, determined by pathological diagnosis, underwent US, SMI, and SWE were included in this study retrospectively. Figure 1 shows the patient screening process. All patients were randomly divided into the training (174 patients; mean age 43.9±13.9 years; range, 24–73 years) and validation (74 patients; mean age 44.8±13.3 years; range, 25–72 years) cohorts in a 7:3 ratio.
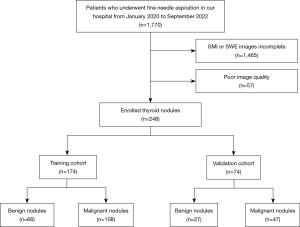
Image acquisition and analysis
All images were acquired with an Aplio i900 ultrasound system (Canon Medical Systems, Tokyo, Japan) equipped with the i18LX5 linear probe (5–18 MHz).
First, the US features of the thyroid nodules including size (defined as the maximum diameter of the thyroid nodule), location, shape, echogenicity, aspect ratio (defined as anteroposterior diameter divided by transverse diameter, >1 or ≤1), and calcification were evaluated. And then blood flow distribution of the thyroid nodules was also evaluated by SMI when the patients were asked to hold their breath and not to swallow. The SMI of thyroid nodules was evaluated in the longitudinal section of the thyroid nodules and the setting of velocity scale of 1.0–2.5 cm/s, mechanical index 1.2, and a frame frequency of 26–60 frames/s. Analysis of blood flow distribution by SMI as suggested by Shin et al. (17): type I, blood flow rarefaction or absence of blood flow; type II, predominantly peri-nodular vascularity; type III, predominantly intra-nodular vascularity; type IV, penetrating vascularity (Figure 2). Penetrating vascularity was defined as blood vessels extending from the edge of the nodule to the inside.
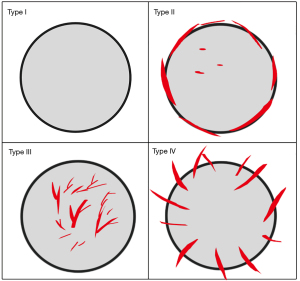
Finally, the longitudinal plane with the clear lesion was selected for SWE. During elastography, the probe was placed perpendicular to the skin and waited for the sampling box to be filled with color, parallel propagation lines were observed at the same time. The region of interest (ROI) was outlined along the nodule’s outer margin, and the stiffness values (kpa) were obtained. The average value of three measurements was taken as the individual result. The image analysis by two experienced radiologists (with more than 5 years of experience) was blinded to pathological outcomes. If the radiologists disagreed, a consensus was obtained by discussion. Representative cases in this study are illustrated in Figure 3.
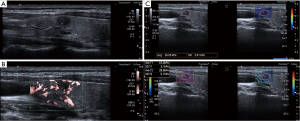
Nomogram construction
First, we used multivariate logistic analyses to identify the risk factors of malignant thyroid nodules. Second, the least absolute shrinkage and selection operator (LASSO) logistic regression algorithm with penalty parameter tuning conducted by 10-fold cross-validation was performed to select the most useful predictive features. Finally, to weigh the odds ratio (OR) by combing multivariate logistics with LASSO regression and the nomogram was obtained. The predicted probability (defined as Nomo-score) of each patient was calculated and the cut-off value of the Nomo-score was obtained to divide high-risk and low-risk groups.
Statistical analysis
Statistical analysis were conducted with SPSS Statistics version 26.0 (IBM Corp.), R software version 4.1.0 (The R Foundation for Statistical Computing), and GraphPad Prism 9.0. Quantitative data were compared by means of the Student’s t-test or Mann-Whitney U test, and categorical data were compared using the χ2 test or Fisher’s exact test. The receiver operating characteristic (ROC) curves were plotted to evaluate the performance of the nomogram and TI-RADS, and the De-Long test was used to compare the area under the curve (AUC). The cut-off values were computed with the maximal Youden index. All statistical tests were 2-sided. P<0.05 was considered significant. Calibration of the nomogram was evaluated using the calibration curve and Hosmer-Lemeshow test. The decision curve analysis (DCA) was performed to determine the clinical significance of the nomogram.
Results
Patient characteristics
The clinical characteristics and US, SMI, and SWE features of all patients are summarized in Table 1. There was no significant statistical difference between the training and validation cohorts in the presence of malignant thyroid nodules (P>0.05). In addition, there were no significant differences between the two cohorts in other characteristics (Table 1). It was suggested that the patients in the training and validation cohorts were comparable (P>0.05).
Table 1
Characteristics | Training cohort (n=174) | Validation cohort (n=74) | P value |
---|---|---|---|
Pathology | 0.83 | ||
Benign | 66 (37.9) | 27 (36.5) | |
Malignant | 108 (62.1) | 47 (63.5) | |
Sex | 0.20 | ||
Male | 47 (27.0) | 26 (35.1) | |
Female | 127 (73.0) | 48 (64.9) | |
Age (years) | 43.9±13.9 | 44.8±13.3 | 0.60 |
Size (cm) | 1.0±0.6 | 1.1±0.6 | 0.85 |
Location | 0.58 | ||
Left lobe | 80 (46.0) | 29 (39.2) | |
Right lobe | 91 (52.3) | 44 (59.5) | |
Isthmic | 3 (1.7) | 1 (1.4) | |
Shape | 0.63 | ||
Regular | 33 (19.0) | 16 (21.6) | |
Irregular | 141 (81.0) | 58 (78.4) | |
A/T | 0.10 | ||
>1 | 76 (43.7) | 24 (32.4) | |
≤1 | 98 (56.3) | 50 (67.6) | |
Calcification | 0.09 | ||
Yes | 131 (75.3) | 48 (64.9) | |
No | 43 (24.7) | 26 (35.1) | |
Echogenicity | 0.79 | ||
Hypoechogenicity | 156 (89.7) | 67 (90.5) | |
Isoechogenicity | 15 (8.6) | 5 (6.8) | |
Hyperechogenicity | 3 (1.7) | 2 (2.7) | |
SMI | 0.057 | ||
I | 5 (2.9) | 7 (9.5) | |
II | 77 (44.3) | 25 (33.8) | |
III | 47 (27.0) | 17 (23.0) | |
IV | 45 (25.9) | 25 (33.8) | |
SWE (kpa) | 34.9±17.1 | 36.5±21.3 | 0.99 |
≥26.91 kpa | 92 (52.9) | 44 (59.5) | 0.34 |
<26.91 kpa | 82 (47.1) | 30 (40.5) |
Continuous data are expressed as means ± standard deviations and for categorical data as n (%). A/T, aspect ratio; SMI, superb microvascular imaging; SWE, shear wave elastography.
Nomogram construction
Table 2 shows the clinical characteristics, US, SMI, and SWE features of patients in the training and validation cohorts. Univariate analysis indicates that there were significant differences in age, size, shape, SMI, and SWE measurement between patients with or without malignant lesions in the training cohort (P<0.05). The optimal cut-off value of SWE for differentiating benign and malignant thyroid nodules was 26.91 kpa, with an AUC being 0.678 [95% confidence interval (CI): 0.594–0.762], and the sensitivity and specificity being 76.9% and 56.1% respectively.
Table 2
Characteristics | Training cohort (n=174) | Validation cohort (n=74) | |||||
---|---|---|---|---|---|---|---|
Benign (n=66) | Malignant (n=108) | P value | Benign (n=27) | Malignant (n=47) | P value | ||
Sex | 0.95 | 0.80 | |||||
Male | 18 (27.3) | 29 (26.9) | 10 (37.0) | 16 (34.0) | |||
Female | 48 (72.7) | 79 (73.1) | 17 (63.0) | 31 (66.0) | |||
Age (years) | 48.0±12.4 | 41.4±11.1 | 0.001 | 49.8±12.9 | 42.0±12.8 | 0.02 | |
Size (cm) | 0.9±0.3 | 1.1±0.7 | 0.044 | 1.2±0.7 | 1.1±0.6 | 0.92 | |
Location | 0.64 | 0.52 | |||||
Left lobe | 29 (43.9) | 55 (50.9) | 9 (33.3) | 20 (42.6) | |||
Right lobe | 36 (54.5) | 51 (47.2) | 18 (66.7) | 26 (55.3) | |||
Isthmic | 1 (1.5) | 2 (1.9) | 0 | 1 (2.1) | |||
Shape | 0.003 | 0.21 | |||||
Regular | 20 (30.3) | 13 (12.0) | 8 (29.6) | 8 (17.0) | |||
Irregular | 46 (69.7) | 95 (88.0) | 19 (70.4) | 39 (83.0) | |||
A/T | 0.07 | 0.52 | |||||
>1 | 23 (34.8) | 53 (49.1) | 10 (37.0) | 14 (29.8) | |||
≤1 | 43 (65.2) | 55 (50.9) | 17 (63.0) | 33 (70.2) | |||
Calcification | 0.91 | 0.44 | |||||
Yes | 50 (75.8) | 81 (75.0) | 16 (59.3) | 32 (68.1) | |||
No | 16 (24.2) | 27 (25.0) | 11 (40.7) | 15 (31.9) | |||
Echogenicity | 0.056 | 0.10 | |||||
Hypoechogenicity | 55 (83.3) | 101 (93.5) | 22 (81.5) | 45 (95.7) | |||
Isoechogenicity | 10 (15.2) | 5 (4.6) | 4 (14.8) | 1 (2.1) | |||
Hyperechogenicity | 1 (1.5) | 2 (1.9) | 1 (3.7) | 1 (2.1) | |||
SMI | <0.001 | <0.001 | |||||
I | 1 (1.5) | 4 (3.7) | 5 (18.5) | 2 (4.3) | |||
II | 5 (7.6) | 27 (25.0) | 16 (59.3) | 9 (19.1) | |||
III | 10 (15.2) | 37 (34.3) | 2 (7.4) | 15 (31.9) | |||
IV | 50 (75.8) | 40 (37.0) | 4 (14.8) | 21 (44.7) | |||
SWE (kpa) | 29.0±14.4 | 38.5±17.7 | <0.001 | 30.0±15.1 | 40.3±23.5 | 0.04 | |
≥26.91 kpa | 29 (43.9) | 83 (76.9) | <0.001 | 12 (44.4) | 32 (68.1) | 0.046 | |
<26.91 kpa | 37 (56.1) | 25 (23.1) | 15 (55.6) | 15 (31.9) |
Continuous data are expressed as means ± standard deviations and for categorical data as n (%). US, conventional ultrasound; SMI, superb microvascular imaging; SWE, shear wave elastography; A/T: aspect ratio.
Then, the LASSO regression result suggested that age, shape, aspect ratio, SMI, and SWE measurement were the significant predictive features for malignant thyroid nodules in the training cohort (Figure 4). The nomogram was obtained by combing multivariate logistic with LASSO regression results and the dynamic nomogram was further constructed (https://dynnomogram11.shinyapps.io/Riskstratification/) (Figure 5) (Table 3). The Nomo-score of each patient was calculated and the cut-off value of the Nomo-score was 0.607, the sensitivity and specificity were 85.2% and 78.8% in the training cohort, 78.7% and 81.5% in the validation cohort. According to the cut-off value of the Nomo-score, we divided patients into low-risk (100 patients) and high-risk groups (116 patients). The proportion of patients with malignant thyroid nodules in the high-risk group was higher than that in the low-risk group in both the training and validation cohorts (P<0.05) (Figure 6).
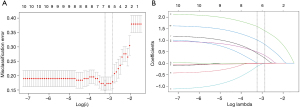
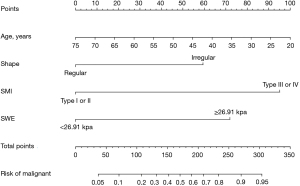
Table 3
Intercept and variables | β | OR (95% CI) | P value |
---|---|---|---|
Age | −0.047 | 0.954 (0.922−0.986) | 0.005 |
Shape | −1.064 | 0.345 (0.124−0.959) | 0.041 |
SMI | 2.252 | 9.511 (4.235−21.357) | <0.001 |
SWE | 1.300 | 3.670 (1.652−8.150) | 0.001 |
Intercept | 1.964 | 7.125 (–) | 0.08 |
OR, odds ratio; CI, confidence interval; SMI, superb microvascular imaging; SWE, shear wave elastography.
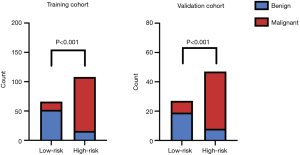
Evaluation and validation of the nomogram
The ROC curves were used to evaluate the performance of the nomogram in the training and validation cohorts (Figure 7). The nomogram shows excellent discrimination both in the training (AUC: 0.848; 95% CI: 0.784–0.911) and validation (AUC: 0.862; 95% CI: 0.780–0.944) cohorts. Our results show that the sensitivity and specificity of the nomogram were 85.2%, and 78.8% in the training cohort and 78.7%, and 81.5% in the validation cohort, respectively (Table 4). The performance of the nomogram was better than US, SMI, and SWE in the training and validation cohorts (P<0.05) (Table 4). The calibration curve and Hosmer-Lemeshow test showed that the nomogram had a good agreement in the training (P=0.15) and validation (P=0.82) cohorts (Figure 8).
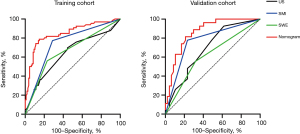
Table 4
Methods | Training cohort | Validation cohort | |||||
---|---|---|---|---|---|---|---|
AUC (95% CI) | Sensitivity (%) | Specificity (%) | AUC (95% CI) | Sensitivity (%) | Specificity (%) | ||
US | 0.638 (0.550−0.726) | 55.6 | 69.7 | 0.689 (0.568−0.810) | 38.3 | 92.6 | |
SMI | 0.743 (0.666−0.820) | 71.3 | 77.3 | 0.772 (0.657−0.887) | 76.6 | 77.8 | |
SWE | 0.665 (0.579−0.750) | 76.9 | 56.1 | 0.618 (0.483−0.753) | 68.1 | 55.6 | |
Nomogram | 0.848 (0.784−0.911) | 85.2 | 78.8 | 0.862 (0.780−0.944) | 78.7 | 81.5 |
US, conventional ultrasound; SMI, superb microvascular imaging; SWE, shear wave elastography; AUC, area under the curve; CI, confidence interval.
Clinical use
The DCA curves showed that the nomogram presented a greater net benefit than US in a wide range of threshold probability in discriminating between benign and malignant thyroid nodules (Figure 9).
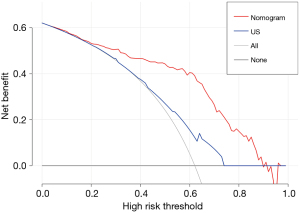
Discussion
In this study, we used multimodal ultrasound imaging, including the US, SMI, and SWE to establish a dynamic nomogram for the risk assessment of TI-RADS 4 thyroid nodules. The nomogram has shown good performance in both training and validation cohorts and could provide significant risk stratification in TI-RADS 4 thyroid nodules, which can assist clinical decision-making and reduce unnecessary needle biopsies.
Thyroid cancer is a kind of tumor with rich blood supply, angiogenesis plays an important role in the development of thyroid cancer (19). As a new ultrasound technology, SMI can clearly display the distribution of blood vessels in the lesions without motion artifacts (20). In this study, SMI was performed to observe the vascularity distribution of thyroid nodules. In line with previous studies (14,15), SMI performed better in displaying details of blood flow distribution. Our results showed that malignant lesions are presented more frequently as intra-nodular and penetrating vascularity, which is consistent with a previous study (10). It is noteworthy that SMI plays the most important role in the risk assessment of TI-RADS 4 thyroid nodules in the dynamic nomogram, and the diagnostic sensitivity and specificity were 71.3% and 77.3% respectively. We believe that this once again illustrates the significant role of angiogenesis in tumor genesis and development.
As it is generally agreed that the stiffness is related to the malignant risk of the lesion (21), this enables SWE to provide valuable information for evaluating the risk of thyroid nodules. SWE is a non-invasive and promising technique to significantly improve the diagnostic accuracy of US (22,23). Gao et al. (21) combined SWE with US features to diagnose thyroid nodules, and the results showed that SWE significantly improved the diagnostic value of US alone. The SWE cut-off value obtained in this study was 26.91 kpa, which is close to 27.49 kpa in Chao et al. (24) study, but it is significantly lower than the 51 kpa obtained by Gao et al. (21). As far as we know, there is no agreement on the cut-off value for the diagnosis of malignant thyroid nodules (21,25). This may be due to differences of the patients’ characteristics and the pathological types of thyroid cancer selected in different studies. The diagnostic sensitivity and specificity of the SWE cut-off value were 76.9% and 56.1% respectively. In this study, the specificity of SMI is significantly better than that of SWE, likely because the SWE value is affected by the calcifications in the lesion to some degree (6,26).
This study established a dynamic nomogram incorporating SWE and SMI for the risk evaluation of TI-RADS 4 thyroid nodules. Our results showed that the AUC, sensitivity, and specificity of the nomogram were 0.848, 85.2%, and 78.8% in the training cohort and 0.862, 78.7%, and 81.5% in the validation cohort, respectively. Both in training and validation cohorts, this dynamic nomogram was significantly superior to US, SMI, or SWE alone in predicting the risk of TI-RADS 4 nodules (P<0.05), possibly suggesting that SMI and SWE could complement each other. It was speculated that SMI could partially compensate for the effect of calcification on SWE, and furthermore, SWE could also provide elasticity information at the lesion. The advantage of dynamic nomogram is that clinicians can easily obtain the Nomo-score of each patient. We conclude that the optimal cut-off value of risk probability is 0.607, patients with Nomo-score more than 0.607 are classified as high-risk group, and patients with Nomo-score lower than 0.607 are classified as low-risk groups. The proportion of patients with malignant thyroid nodules in the high-risk group was higher than that in the low-risk group in the training and validation cohorts, which indicates that the cut-off value can be more effectively used on risk stratification.
There are several limitations in our study. First, it was a retrospective study, which may lead to some degree of selection bias. Second, the sample size of our study is relatively small and it needs to be further expanded. Finally, this study did not collect quantitative data on SMI which seems to provide more information.
Conclusions
The dynamic nomogram has been established by including additional US-derived modalities SMI and SWE, and it can accurately stratify the malignancy risk of TI-RADS 4 thyroid nodules in order to assist clinical decision-making.
Acknowledgments
Funding: This work was supported by
Footnote
Reporting Checklist: The authors have completed the TRIPOD reporting checklist. Available at https://gs.amegroups.com/article/view/10.21037/gs-24-87/rc
Data Sharing Statement: Available at https://gs.amegroups.com/article/view/10.21037/gs-24-87/dss
Peer Review File: Available at https://gs.amegroups.com/article/view/10.21037/gs-24-87/prf
Conflicts of Interest: All authors have completed the ICMJE uniform disclosure form (available at https://gs.amegroups.com/article/view/10.21037/gs-24-87/coif). The authors have no conflicts of interest to declare.
Ethical Statement:
Open Access Statement: This is an Open Access article distributed in accordance with the Creative Commons Attribution-NonCommercial-NoDerivs 4.0 International License (CC BY-NC-ND 4.0), which permits the non-commercial replication and distribution of the article with the strict proviso that no changes or edits are made and the original work is properly cited (including links to both the formal publication through the relevant DOI and the license). See: https://creativecommons.org/licenses/by-nc-nd/4.0/.
References
- Li Y, Teng D, Ba J, et al. Efficacy and Safety of Long-Term Universal Salt Iodization on Thyroid Disorders: Epidemiological Evidence from 31 Provinces of Mainland China. Thyroid 2020;30:568-79. [Crossref] [PubMed]
- Zhang L, Gu J, Zhao Y, et al. The role of multimodal ultrasonic flow imaging in Thyroid Imaging Reporting and Data System (TI-RADS) 4 nodules. Gland Surg 2020;9:1469-77. [Crossref] [PubMed]
- Tessler FN, Middleton WD, Grant EG, et al. ACR Thyroid Imaging, Reporting and Data System (TI-RADS): White Paper of the ACR TI-RADS Committee. J Am Coll Radiol 2017;14:587-95. [Crossref] [PubMed]
- Zhang Y, Zhou P, Tian SM, et al. Usefulness of combined use of contrast-enhanced ultrasound and TI-RADS classification for the differentiation of benign from malignant lesions of thyroid nodules. Eur Radiol 2017;27:1527-36. [Crossref] [PubMed]
- Tessler FN, Middleton WD, Grant EG. Thyroid Imaging Reporting and Data System (TI-RADS): A User's Guide. Radiology 2018;287:29-36. [Crossref] [PubMed]
- Pei S, Cong S, Zhang B, et al. Diagnostic value of multimodal ultrasound imaging in differentiating benign and malignant TI-RADS category 4 nodules. Int J Clin Oncol 2019;24:632-9. [Crossref] [PubMed]
- Singh Ospina N, Brito JP, Maraka S, et al. Diagnostic accuracy of ultrasound-guided fine needle aspiration biopsy for thyroid malignancy: systematic review and meta-analysis. Endocrine 2016;53:651-61. [Crossref] [PubMed]
- Scappaticcio L, Trimboli P, Iorio S, et al. Repeat thyroid FNAC: Inter-observer agreement among high- and low-volume centers in Naples metropolitan area and correlation with the EU-TIRADS. Front Endocrinol (Lausanne) 2022;13:1001728. [Crossref] [PubMed]
- Sun C, Li J, Wang B, et al. Tumor angiogenesis and bone metastasis - Correlation in invasive breast carcinoma. J Immunol Methods 2018;452:46-52. [Crossref] [PubMed]
- Chen L, Zhan J, Diao XH, et al. Additional Value of Superb Microvascular Imaging for Thyroid Nodule Classification with the Thyroid Imaging Reporting and Data System. Ultrasound Med Biol 2019;45:2040-8. [Crossref] [PubMed]
- Zhan J, Diao XH, Jin JM, et al. Superb Microvascular Imaging-A new vascular detecting ultrasonographic technique for avascular breast masses: A preliminary study. Eur J Radiol 2016;85:915-21. [Crossref] [PubMed]
- Bonacchi G, Becciolini M, Seghieri M. Superb microvascular imaging: a potential tool in the detection of FNH. J Ultrasound 2017;20:179-80. [Crossref] [PubMed]
- Li W, Gao L, Du Y, et al. Ultrasound microflow patterns help in distinguishing malignant from benign thyroid nodules. Cancer Imaging 2024;24:18. [Crossref] [PubMed]
- Machado P, Segal S, Lyshchik A, et al. A Novel Microvascular Flow Technique: Initial Results in Thyroids. Ultrasound Q 2016;32:67-74. [Crossref] [PubMed]
- Lu R, Meng Y, Zhang Y, et al. Superb microvascular imaging (SMI) compared with conventional ultrasound for evaluating thyroid nodules. BMC Med Imaging 2017;17:65. [Crossref] [PubMed]
- Nowicki A, Dobruch-Sobczak K. Introduction to ultrasound elastography. J Ultrason 2016;16:113-24. [Crossref] [PubMed]
- Shin JH, Baek JH, Chung J, et al. Ultrasonography Diagnosis and Imaging-Based Management of Thyroid Nodules: Revised Korean Society of Thyroid Radiology Consensus Statement and Recommendations. Korean J Radiol 2016;17:370-95. [Crossref] [PubMed]
- Lee EJ, Chang YW. Combination of Quantitative Parameters of Shear Wave Elastography and Superb Microvascular Imaging to Evaluate Breast Masses. Korean J Radiol 2020;21:1045-54. [Crossref] [PubMed]
- Fu Z, Zhang J, Lu Y, et al. Clinical Applications of Superb Microvascular Imaging in the Superficial Tissues and Organs: A Systematic Review. Acad Radiol 2021;28:694-703. [Crossref] [PubMed]
- Zhu YC, Zhang Y, Shan J, et al. Added Value of Superb Microvascular Imaging and Virtual Touch Imaging Quantification in Assisting Thyroid Cancer Classification. Ultrasound Med Biol 2021;47:3364-71. [Crossref] [PubMed]
- Gao XQ, Ma Y, Peng XS, et al. Diagnostic performance of C-TIRADS combined with SWE for the diagnosis of thyroid nodules. Front Endocrinol (Lausanne) 2022;13:939303. [Crossref] [PubMed]
- Bora Makal G, Aslan A. The Diagnostic Value of the American College of Radiology Thyroid Imaging Reporting and Data System Classification and Shear-Wave Elastography for the Differentiation of Thyroid Nodules. Ultrasound Med Biol 2021;47:1227-34. [Crossref] [PubMed]
- Li HJ, Sui GQ, Teng DK, et al. Incorporation of CEUS and SWE parameters into a multivariate logistic regression model for the differential diagnosis of benign and malignant TI-RADS 4 thyroid nodules. Endocrine 2024;83:691-9. [Crossref] [PubMed]
- Fu C, Cui KF, Qin SC, et al. Clinical application of real-time shear wave elastography in diagnosis of benign and malignant thyroid solid nodules. Chinese Journal of Ultrasonography 2012;21:49-51.
- Kim HJ, Kwak MK, Choi IH, et al. Utility of shear wave elastography to detect papillary thyroid carcinoma in thyroid nodules: efficacy of the standard deviation elasticity. Korean J Intern Med 2019;34:850-7. [Crossref] [PubMed]
- Wang B, Ou X, Yang J, et al. Contrast-enhanced ultrasound and shear wave elastography in the diagnosis of ACR TI-RADS 4 and 5 category thyroid nodules coexisting with Hashimoto's thyroiditis. Front Oncol 2022;12:1022305. [Crossref] [PubMed]