Prediction of malignant risk stratification model for parotid gland nodules based on clinical and conventional ultrasound features: construction and validation
Highlight box
Key findings
• A prediction model was developed with the clinical and ultrasonic features for the precise and intuitive malignancy probability of parotid nodules. This could provide a reliable reference for further treatment.
What is known and what is new?
• Ultrasound is widely used in the examination of the parotid gland, but no single ultrasound feature has demonstrated satisfactory diagnostic performance in predicting the nature of parotid nodules.
• In this study, the malignancy probability of parotid nodules can be evaluated in real time by selecting relevant parameters, and the diagnostic efficacy performed well.
What is the implication, and what should change now?
• The inspection process should be optimized after the detection of suspected parotid nodules to reduce unnecessary suffering for patients.
Introduction
Salivary gland tumors are common tumors in the head and neck region, accounting for approximately 3–6% of all head and neck tumors (1). Regarding the incidence and location of salivary gland tumors, the “80 rule” is widely applied (2): 80% of salivary gland tumors are located in the parotid gland, 80% of parotid gland tumors are benign, and 80% of benign tumors are located in the superficial lobe. Identifying the tumor type can provide guidance for the surgery. For benign nodules, it is recommended to preserve the patient’s facial nerve as much as possible, and usually, partial glandular lobe resection or tumor excision is performed (3). However, it is necessary to avoid inadequate excision of malignant tumors, as this may lead to postoperative recurrence. Generally, total parotidectomy and neck lymph node dissection are performed, accompanied by postoperative radiotherapy or chemotherapy (4). Therefore, accurate preoperative diagnosis is crucial for selecting the appropriate surgical procedure.
Diagnostic methods for parotid nodules include ultrasonography, magnetic resonance imaging and computed tomography. High-frequency ultrasonography has advantages in the field of superficial organs due to its dynamic, portable, and non-ionizing radiation characteristics. Previous study has demonstrated that the shape, margin, internal echogenicity, cervical lymphadenopathy, and intranodular vascular distribution can aid in identifying the nature of parotid nodules (5). However, no single ultrasound feature has demonstrated satisfactory diagnostic performance in predicting the nature of parotid nodules. Unlike the established and widely used grading systems for breast and thyroid nodules, there is currently no standardized ultrasound-based grading system for parotid nodules, and there is a high degree of subjectivity and inter-rater variability in the ultrasound diagnosis.
Therefore, this study aims to develop a parotid gland malignant risk stratification model based on clinical and ultrasonographic features, which can improve the diagnostic efficiency of ultrasonography and facilitate homogeneous expression of the results for effective communication with clinicians (6). We present this article in accordance with the TRIPOD reporting checklist (available at https://gs.amegroups.com/article/view/10.21037/gs-24-119/rc).
Methods
Study population
Clinical data and ultrasound images of patients who underwent ultrasound examination of the salivary gland at the PLA General Hospital from January 2015 to July 2023 were selected. Inclusion criteria were as follows: (I) occupying lesions located within the parotid gland; (II) conventional ultrasound examination performed prior to fine-needle aspiration biopsy or surgical excision, with preserved and complete images; (III) nodules with definite pathological results obtained through fine-needle aspiration biopsy or postoperative examination. Exclusion criteria comprised: (I) poor ultrasound image quality with non-standardized image preservation; (II) parotid gland fine-needle aspiration biopsy performed before ultrasound examination; (III) postoperative recurrence of parotid gland lesions; (IV) non-nodular lesions such as sialolithiasis, Sjögren’s syndrome, and sialadenitis. A total of 736 patients were included, for those with multiple nodules, the largest nodules confirmed by pathology were analyzed. The patients were randomly divided into two groups in a 7:3 ratio: (I) training group: 515 cases; (II) validation group: 221 cases. The study was conducted in accordance with the Declaration of Helsinki (as revised in 2013). The study was approved by the ethics committee of PLA General Hospital (No. S2021-583-01) and individual consent for this study was waived due to the retrospective nature. The flowchart of patient selection is shown in Figure 1.
Clinical features
Clinical data of patients were collected through the medical record system, including gender, age, smoking history, course of disease, and facial symptoms (referring to facial pain, peripheral facial paralysis of unknown cause). If the patient had a disease that caused facial pain or peripheral facial paralysis in the past, this feature was not included.
Ultrasonography
The instruments used included Mindray Resone 7, Siemens Acuson Sequoia, and Phillips iU22 ultrasound diagnostic instruments, with a probe frequency of 5–12 MHz. The conventional ultrasound observation parameters include: (I) quantity and location: the number of nodules within the left and right glands are recorded separately, and if there are more than one, it is considered multifocal. If nodules are present bilaterally, it is considered bilateral. (II) Size and ratio of the short diameter to the long diameter (S/L ratio): the long and short diameters of the section with the largest diameter of the nodule are measured, and the S/L ratio is recorded. (III) Shape: divided into regular and irregular. Regular shapes include round (circular, spherical, or elliptical nodules) and shallow lobes (nodules with ≤3 blunt angles or depressions on the edge). Irregular shapes include heterogeneous shapes (nodules that are neither circular nor elliptical) and needle-shaped/deep lobes (sharp needle-shaped or spiky structures or gear-shaped undulations, with >3 waveforms and depressions on the edge of the nodule). (IV) Margin: when >50% of the nodule margin can be clearly observed on the ultrasound image, the margin is considered well-defined, otherwise it is considered ill-defined. (V) Internal echo intensity: the echo of normal parotid gland tissue is defined as isoechoic. Nodules with no internal echo (internal echo loss) are classified as anechoic, nodules with an echo intensity of more than 50% lower than the echo of the gland tissue are classified as hypoechoic, nodules with an echo intensity of more than 50% the same as the gland tissue are classified as isoechoic, and nodules with an echo intensity of more than 50% higher than the gland tissue are classified as hyperechoic. (VI) Internal echo texture: classified as homogeneous (internal echo of the nodule is consistent) or heterogeneous (internal echo of the nodule is inconsistent) based on whether the internal echo of the nodule is consistent. (VII) Internal echo structure: classified as cystic, predominantly cystic (cystic component >50%), solid, predominantly solid (solid component >50%), or reticular (multiple strands visible inside forming a mesh-like pattern). (VIII) Microcalcification: if strong echo points with a diameter of ≤1 mm are observed in the nodule, microcalcification is considered present. Otherwise, it is considered absent. (IX) Macrocalcification: if strong echo spots with a diameter >1 mm are observed in the nodule, coarse calcification is considered present. Otherwise, it is considered absent. (X) Posterior echo: if the posterior echo of the nodule is enhanced, it is classified as enhanced. If there is no posterior echo enhancement or the echo is attenuated, it is classified as not enhanced. (XI) Neck lymph nodes: if lymph nodes in the neck exhibit the following characteristics: round shape, disappearance of lymphatic hilum, clustered hyperechoic area, and internal speckled or punctate strong echoes, abnormal lymph nodes are considered present. (XII) Blood flow signal: graded according to the Adler semi-quantitative method. Grade 0 indicates no blood flow signal detected in the nodule; grade I indicates a small amount of blood flow, with 1–2 dot-shaped or thin rod-shaped blood vessels visible; grade II indicates moderate blood flow, with 3–4 dot-shaped blood vessels visible or a longer blood vessel entering the nodule, with a length approaching or exceeding the radius of the nodule; grade III indicates abundant blood flow, with ≥5 dot-shaped blood vessels or 2 longer blood vessels visible.
All ultrasound images were examined and obtained by physicians with more than 5 years of experience in superficial organ ultrasound examination. After extracting the images from the workstation, two ultrasound physicians with more than 5 years of experience jointly analyzed the ultrasound images without knowing the clinical pathological information. When there was a dispute, a deputy chief physician made the judgment. The pathological results were diagnosed and classified according to the 5th edition of the World Health Organization (WHO) in 2022 by doctors with more than 5 years of pathology experience.
Selection and scoring of malignant features
Single-factor analysis selected parameters with a P value less than 0.05 from a total of 19 clinical and ultrasound features. Multiple logistic regression was used to perform multivariate analysis on indicators with statistical significance to determine the independent risk factors for malignant parotid nodules and quantify the malignant score.
Malignant risk stratification was divided into grades 1–5. Grade 1: normal parotid gland; Grade 2: definitive benign lesion; Grade 3: possibly benign in nature, but the malignancy cannot be ruled out; Grade 4: suspected malignancy; Grade 5: high probability of malignancy.
Model construction and clinical value analysis
The calibration curves evaluated the calibration degree of the model, and the receiver operating characteristic (ROC) curve evaluated the discrimination degree of the model, including the area under the curve (AUC), sensitivity, specificity, and Youden index in the training and validation groups. Decision curve analysis (DCA) and clinical impact curve analysis (CICA) were used to validate the clinical applicability of the model.
Statistical analysis
SPSS 27.0 statistical software and R language were used for analysis. Measurement data were expressed as mean ± standard deviation, and t-test or Mann-Whitney U test was used. Count data were analyzed using chi-square test or Fisher’s exact test. Multiple factor analysis used logistic regression analysis, and the linear trend chi-square test was used to evaluate the correlation between the score and the probability of malignant tumors. All statistical tests were two-sided, and P<0.05 was considered statistically significant.
Results
Subject characteristics
A total of 736 nodules were analyzed, of which 669 nodules confirmed histopathologically after surgery and 67 nodules after fine-needle aspiration biopsy. Five hundred and eighty-two nodules (79.1%) were benign and 154 nodules (20.9%) were malignant, with specific pathological types and proportions shown in Table 1. The clinical characteristics of the training group patients are shown in Table 2, and there was a statistically significant difference in facial symptoms incidence between benign and malignant nodules (P<0.05), while the other factors were not statistically significant between the groups.
Table 1
Items | Pathological results | Number of cases |
---|---|---|
Benign (n=582) | Pleomorphic adenoma | 245 |
Warthin’s tumor | 193 | |
Basal cell adenoma | 92 | |
Myoepithelioma | 8 | |
Oncocytoma | 6 | |
Neurofibroma | 2 | |
Lipoma | 6 | |
Hemangioma | 3 | |
Neurilemmoma | 12 | |
Dermoid cyst | 2 | |
Kimura’s disease | 1 | |
IgG4-related sclerosing disease | 4 | |
Lymphoepithelial cyst | 1 | |
Lymph node hyperplasia | 4 | |
Cyst of duct | 3 | |
Malignant (n=154) | Acinar cell carcinoma | 15 |
Mucoepidermoid carcinoma | 27 | |
Adenoid cystic carcinoma | 20 | |
Myoepithelial carcinoma | 1 | |
Basal cell adenocarcinoma | 1 | |
Sebaceous gland carcinoma | 5 | |
Ductal carcinoma of the salivary gland | 14 | |
Carcinoma in pleomorphic adenoma | 7 | |
Lymphoma | 23 | |
Squamous cell carcinoma | 20 | |
Metastatic tumors | 9 | |
Adenocarcinoma | 8 | |
Neuroendocrine carcinoma | 2 | |
Carcinosarcoma | 1 | |
Secretory carcinoma | 1 |
Table 2
Features | Benign (n=407) | Malignant (n=108) | P value |
---|---|---|---|
Age (years), mean ± SD | 51.1±12.7 | 52.3±13.8 | 0.90 |
Sex, n | 0.27 | ||
Male | 217 | 64 | |
Female | 190 | 44 | |
Facial symptoms, n | <0.001 | ||
Yes | 8 | 24 | |
No | 399 | 84 | |
History of smoking, n | 0.27 | ||
Yes | 221 | 65 | |
No | 186 | 43 | |
Course of disease, n | 0.34 | ||
Within 1 year | 206 | 61 | |
1–5 years | 134 | 25 | |
More than 5 years | 67 | 22 |
SD, standard deviation.
Regarding ultrasound image features, there were statistically significant differences (P<0.05) between benign and malignant nodules in terms of shape, margin, microcalcification, posterior echo and abnormal lymph nodes in the neck (Table 3), while the other factors did not differ significantly between the benign and malignant nodules.
Table 3
Features | Benign (n=407) | Malignant (n=108) | P value |
---|---|---|---|
Number | 0.25 | ||
Single | 327 | 92 | |
Multiple | 80 | 16 | |
Location | 0.15 | ||
Left | 194 | 43 | |
Right | 213 | 65 | |
Long diameter | 0.39 | ||
<2 cm | 111 | 23 | |
2–4 cm | 249 | 71 | |
>4 cm | 47 | 14 | |
Shape | <0.001 | ||
Regular | 337 | 55 | |
Irregular | 70 | 53 | |
Margin | <0.001 | ||
Clear | 388 | 67 | |
Unclear | 19 | 41 | |
Macrocalcification | 0.10 | ||
Yes | 29 | 13 | |
No | 378 | 95 | |
Microcalcification | <0.001 | ||
Yes | 8 | 22 | |
No | 399 | 86 | |
S/L ratio | 0.07 | ||
<1 | 377 | 94 | |
≥1 | 30 | 14 | |
Internal echogenicity | 0.09 | ||
Anechoic | 6 | 0 | |
Hypoechoic | 367 | 103 | |
Isoechoic | 34 | 5 | |
Hyperechoic | 0 | 0 | |
Internal echo status | 0.08 | ||
Homogeneous | 70 | 11 | |
Non-uniform | 337 | 97 | |
Internal structure | 0.54 | ||
Cystic | 2 | 0 | |
Mainly cystic | 13 | 3 | |
Solid | 313 | 86 | |
Mainly solid | 39 | 14 | |
Reticular | 40 | 5 | |
Blood flow signal (type) | 0.57 | ||
0–I | 150 | 43 | |
II–III | 257 | 65 | |
Abnormal cervical lymph nodes | <0.001 | ||
Yes | 1 | 15 | |
No | 406 | 93 | |
Posterior echo | <0.001 | ||
Enhanced | 367 | 78 | |
Unenhanced | 40 | 30 |
S/L ratio, ratio of the short diameter to the long diameter.
Multivariate logistic regression analysis
Multivariate logistic regression analysis was performed on clinical and ultrasound indicators with P<0.05, and the results showed that facial symptoms, unclear margin, irregular shape, microcalcification and abnormal lymph nodes in the neck were independent risk factors for malignant parotid gland nodules (Table 4).
Table 4
Items | Coefficient of regression | Standard error | Wald | P value |
---|---|---|---|---|
Facial symptoms | 2.266 | 0.475 | 22.702 | <0.001 |
Unclear margin | 2.220 | 0.364 | 37.185 | <0.001 |
Irregular shape | 1.408 | 0.287 | 24.018 | <0.001 |
Microcalcification | 2.234 | 0.491 | 20.695 | <0.001 |
Abnormal cervical lymph nodes | 2.833 | 1.077 | 6.921 | 0.009 |
A malignancy score was calculated based on the ultrasound features, with irregular shape having the lowest coefficient (1.408) and being used as the reference value of 1. The scores of other features were calculated by dividing them by the reference value and rounding the resulting number, which was then used as the corresponding score. The total score ranged from 0 to 9. Each nodule in the training group was scored accordingly, and the corresponding risk grade was determined. The malignant score tables and the scores for benign and malignant nodules in the training group are shown in Tables 5,6, respectively.
Table 5
Risk factor | Classification | Score |
---|---|---|
Shape | Regular | 0 |
Irregular | 1 | |
Margin | Clear | 0 |
Unclear | 2 | |
Facial symptoms | No | 0 |
Yes | 2 | |
Abnormal cervical lymph nodes | No | 0 |
Yes | 2 | |
Microcalcification | No | 0 |
Yes | 2 |
Table 6
Score | Benign, n | Malignant, n | Proportion of malignant (%) |
---|---|---|---|
0 | 319 | 16 | 4.8 |
1 | 57 | 21 | 26.9 |
2 | 14 | 21 | 60.0 |
3 | 12 | 22 | 64.7 |
4 | 4 | 17 | 81.0 |
5 | 1 | 8 | 88.9 |
6 | 0 | 1 | 100.0 |
7 | 0 | 2 | 100.0 |
8 | 0 | 0 | – |
9 | 0 | 0 | – |
Construction of malignant risk stratification model
Considering the habits of radiologists and clinical applicability, this study referred to the classification systems of breast and thyroid nodules and divided parotid gland nodules into grades 1–5 based on malignant risk. Table 7 presents the ultrasound features, malignant scores, and probabilities based on the malignant risk stratification model. Figures 2-5 show the sonographic images of some cases classified as malignant risk grades 2–5.
Table 7
Malignant risk stratification | Categories of risk | Malignancy score | Probability of malignancy |
---|---|---|---|
1 | Normal parotid gland | – | 0 |
2 | Benign | – | 0 |
3 | Low suspicion | 0 | <5% |
4 | Intermediate suspicion | 1–3 | 5–80% |
5 | High suspicion | ≥4 | >80% |
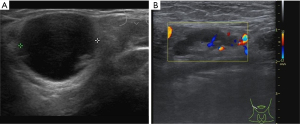
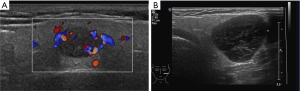
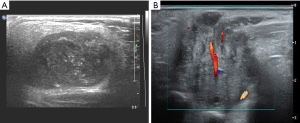
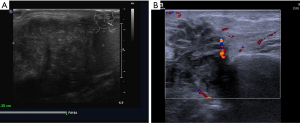
Diagnostic efficacy of the prediction model
The calibration curves of the training and validation groups are shown in Figure 6, and the Hosmer-Lemeshow goodness-of-fit test indicates that the model has a good fit (P=0.22 in the training group and P=0.31 in the validation group, both P>0.05). The AUC of the training group was 0.850 [95% confidence interval (CI): 0.816–0.879], with a sensitivity of 85.19% and a specificity of 74.94%, and the Youden index selected the optimal critical value of 0.601. In the validation group, the AUC was 0.846 (95% CI: 0.791–0.891), with a sensitivity of 80.43% and a specificity of 82.86%, and the Youden index selected the optimal critical value of 0.633 (Figure 7). The linear trend chi-square test result had a P value <0.001, indicating a correlation between nodule scores and malignant probabilities.
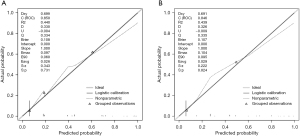
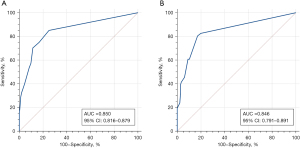
The value of the model in clinical practice can be evaluated using DCA and CICA. DCA plots the net benefit value on the y-axis against the threshold value on the x-axis by calculating the net benefit value of the model at different threshold values (Figure 8). CICA plots the number of high-risk individuals on the y-axis, the first horizontal axis is the threshold probability value, and the second horizontal axis is the loss-to-benefit ratio. The red curve represents the number of high-risk individuals, and the blue curve represents the number of outcome events in the high-risk population (Figure 9). The results of this study show that the model has good clinical applicability overall.
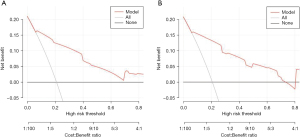
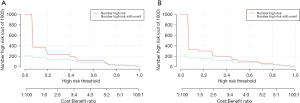
Discussion
Since the inception of the Image Reporting and Data System in the field of medical ultrasonography, it has found extensive utility in the evaluation of various organ systems (7), demonstrating notable accuracy in the assessment of malignant tumor risks (8). However, as of the present moment, there remains a conspicuous absence of a widely adopted and clinically endorsed risk stratification system for malignancies of the parotid glands. Abdel Razek et al. (9) have endeavored to categorize the ultrasound manifestations of parotid gland nodules into nine distinct patterns based on conventional ultrasonographic features, assigning respective risk levels to each pattern. To facilitate the development of a practical and expeditious method for parotid gland nodule analysis in the clinical setting, we have leveraged parotid gland nodule risk factors to streamline the diagnostic process and construct a predictive model.
Parotid gland nodules have a complex pathological classification, with 41 subtypes of parotid gland tumors, including 15 benign and 26 malignant, according to the WHO [2022] classification of head and neck tumors (10). The histological distribution is approximately 80% benign and 20% malignant (11). Benign tumors are mainly composed of pleomorphic adenoma, Warthin tumor, and basal cell adenoma (12), while malignant tumors are most commonly mucoepidermoid carcinoma and adenoid cystic carcinoma (13). Due to the lack of specific laboratory indicators (14), imaging examination has become an important way to evaluate parotid gland nodules. Fine needle aspiration biopsy is currently the only method for preoperative qualitative diagnosis of parotid gland nodules. However, for the risk of tumor cell dissemination as well as unsatisfactory specimens, inability to diagnose, its clinical utility (15) and safety (16) are controversial. This study used multivariate logistic regression analysis to identify independent risk factors for predicting malignant parotid gland tumors, quantified malignant indicators, and graded them. The results showed that this scoring system can be used to evaluate the risk of malignant parotid gland tumors and is positively correlated with the score.
Facial symptoms are statistically significant indicators of malignant parotid gland nodules. The facial nerve enters the parotid gland, and if the nodules invade the facial nerve, facial paralysis may occur. Pain, peripheral tissue adhesion, and facial nerve paralysis are considered important symptoms indicating malignant parotid gland nodules (17). However, previous study has suggested that only one-third of malignant nodules present with facial paralysis, adhesion to peripheral tissues and pain (18). The results of our study showed that only about 28% of malignant nodules had facial symptoms. At the same time, we noticed that the proportion of patients with facial pain was highest in patients with adenoid cystic carcinoma (13/20=65%), and Inaka et al. (19) suggested that the reason for the higher incidence of facial pain in parotid gland adenoid cystic carcinoma is that this tumor has obvious neurotropism compared to other malignant nodules and has strong invasiveness.
In contrast to the fact that women were more likely to suffer breast and thyroid nodules than men, this study found that both benign and malignant parotid gland nodules are more common in middle-aged and elderly men. Albright et al. (20) found that head and neck tumors accounted for as much as 12% of childhood malignant tumors, much higher than in adults. However, in this study, gender and age (both mean age and different age groups) were not statistically significant in distinguishing between benign and malignant nodules. Smoking may be one of the inducing factors of malignant nodules, but some studies have suggested that there is a strong correlation between smoking and Warthin tumor, with a incidence rate eight times higher in non-smokers (21), so smoking cannot be used as a distinguishing point between benign and malignant nodules.
This study showed that irregular shape, unclear margin, microcalcification and abnormal lymph nodes in the neck can be used as distinguishing features between benign and malignant nodules on conventional ultrasound. Benign nodules exhibit expansive growth, often with a capsule and clear margins (22), while malignant nodules often have an incomplete capsule or do not have a capsule at all, and causing unclear margin (23). Rzepakowska et al. have reported that only 30% of malignant parotid gland tumors show unclear margins on ultrasound (24), while our study found a higher proportion (38%), which may be related to the degree of differentiation and invasiveness of the tumor. When the tumor has not completely broken through the capsule or is in the early stage of invasion, it often has clear margins. At the same time, we also noticed that among the 79 benign cases with irregular shape or unclear margin in the training group, 46 cases were diagnosed as pleomorphic adenoma. Previous study has suggested that pleomorphic adenoma has the characteristics of tissue polymorphism (25), and the type with predominant epithelial tissue has more active growth which can cause unclear margin and irregular shape.
Schick et al. (26) believed that calcification cannot be used to distinguish between benign and malignant parotid gland tumors. Both benign and malignant groups in this study had macrocalcifications, and the difference was not statistically significant. Compared with macrocalcifications, we found that microcalcifications were more common in malignant nodules than in benign nodules, and could appear as punctate, granular, or dot-like calcifications. Previous study has suggested that the appearance of microcalcifications in malignant parotid gland nodules is related to the effect of nodules on local calcium and phosphorus metabolism, mainly manifested as punctate or needle-like calcifications (27).
After establishing the malignant signs of parotid gland nodules, it is crucial to quantify the corresponding signs. In our stratification model, the scoring criteria for each feature are based on its logistic regression coefficient (28). Therefore, different signs have different scores, and signs with higher scores indicate a higher probability of malignancy. The model showed high accuracy in both the training and validation groups, but misdiagnosed cases still exist. After analysis, we found that the ultrasound appearance of pleomorphic adenoma in benign nodules is often atypical, which may be related to its tissue polymorphism and transitional zones (25). Acinar cell carcinoma and lymphoma in malignant nodules are easily confused with benign nodules, and among the 38 cases, 18 cases had clear margins and regular shape, and no obvious facial symptoms appeared. Acinar cell carcinoma is a low-grade malignant tumor of the parotid gland, with slow growth and a benign growth process (29). B-cell lymphoma is more common in parotid gland lymphoma, often showing clear margins, uniform echoes, or multiple strands or mesh-like structures, making it difficult to distinguish from benign nodules, especially Warthin tumor (30). Although our developed malignant risk scoring and stratification model provides an objective prediction of the malignancy degree of parotid nodules, it is still a supplement to the clinical judgment of surgeons, and the final pathological results may differ from the scoring predictions.
It is necessary to acknowledge the limitations of this study: (I) as a retrospective single-center study, potential selection bias may exist. In future studies, multicenter samples can be considered for external validation. (II) The incidence of malignant parotid gland tumors is low, and some patients have not completed the ultrasound examination, resulting in a limited sample size. (III) In the training and validation groups, the sensitivity level is generally better than the specificity level. After analyzing the reasons, we find that irregular shape appears more frequently in benign nodules compared to other malignant signs, which is somewhat misleading. (IV) The accuracy of the model’s diagnostic performance is correlated to some extent with the experience level of the radiologists.
Conclusions
The study used multiple logistic regression analysis to screen for malignant signs of parotid gland nodules and quantify malignant scores and risk probabilities, developing a new, easy-to-use, and highly accurate malignant risk scoring and stratification model. This model can help stratify the malignant risk of parotid gland nodules and better guide clinical decision-making using ultrasound diagnosis.
Acknowledgments
Funding: None.
Footnote
Reporting Checklist: The authors have completed the TRIPOD reporting checklist. Available at https://gs.amegroups.com/article/view/10.21037/gs-24-119/rc
Data Sharing Statement: Available at https://gs.amegroups.com/article/view/10.21037/gs-24-119/dss
Peer Review File: Available at https://gs.amegroups.com/article/view/10.21037/gs-24-119/prf
Conflicts of Interest: All authors have completed the ICMJE uniform disclosure form (available at https://gs.amegroups.com/article/view/10.21037/gs-24-119/coif). The authors have no conflicts of interest to declare.
Ethical Statement: The authors are accountable for all aspects of the work in ensuring that questions related to the accuracy or integrity of any part of the work are appropriately investigated and resolved. The study was conducted in accordance with the Declaration of Helsinki (as revised in 2013). The study was approved by the ethics committee of PLA General Hospital (No. S2021-583-01) and individual consent for this study was waived due to the retrospective nature.
Open Access Statement: This is an Open Access article distributed in accordance with the Creative Commons Attribution-NonCommercial-NoDerivs 4.0 International License (CC BY-NC-ND 4.0), which permits the non-commercial replication and distribution of the article with the strict proviso that no changes or edits are made and the original work is properly cited (including links to both the formal publication through the relevant DOI and the license). See: https://creativecommons.org/licenses/by-nc-nd/4.0/.
References
- Tian Z, Li L, Wang L, et al. Salivary gland neoplasms in oral and maxillofacial regions: a 23-year retrospective study of 6982 cases in an eastern Chinese population. Int J Oral Maxillofac Surg 2010;39:235-42. [Crossref] [PubMed]
- Stoia S, Băciuț G, Lenghel M, et al. Ultrasonography techniques in the preoperative diagnosis of parotid gland tumors - an updated review of the literature. Med Ultrason 2021;23:194-202. [Crossref] [PubMed]
- Lee DH, Yoon TM, Lee JK, et al. Surgical treatment strategy in Warthin tumor of the parotid gland. Braz J Otorhinolaryngol 2019;85:546-50. [Crossref] [PubMed]
- Park YM, Koh YW. Current Issues in Treatment of Parotid Gland Cancer and Advanced Surgical Technique of Robotic Parotidectomy. Curr Oncol Rep 2022;24:203-8. [Crossref] [PubMed]
- Haidar YM, Moshtaghi O, Mahmoodi A, et al. The Utility of In-Office Ultrasound in the Diagnosis of Parotid Lesions. Otolaryngol Head Neck Surg 2017;156:511-7. [Crossref] [PubMed]
- Na DG, Baek JH, Sung JY, et al. Thyroid Imaging Reporting and Data System Risk Stratification of Thyroid Nodules: Categorization Based on Solidity and Echogenicity. Thyroid 2016;26:562-72. [Crossref] [PubMed]
- O'Connor SD, Kulkarni NM, Griffin MO Jr, et al. Structured Reporting in Ultrasound. Ultrasound Q 2020;36:1-5. [Crossref] [PubMed]
- Ganeshan D, Duong PT, Probyn L, et al. Structured Reporting in Radiology. Acad Radiol 2018;25:66-73. [Crossref] [PubMed]
- Abdel Razek AA, Ashmalla GA, Gaballa G, et al. Pilot study of ultrasound parotid imaging reporting and data system (PIRADS): Inter-observer agreement. Eur J Radiol 2015;84:2533-8. [Crossref] [PubMed]
- Skálová A, Hyrcza MD, Leivo I. Update from the 5th Edition of the World Health Organization Classification of Head and Neck Tumors: Salivary Glands. Head Neck Pathol 2022;16:40-53.
- Thielker J, Grosheva M, Ihrler S, et al. Contemporary Management of Benign and Malignant Parotid Tumors. Front Surg 2018;5:39. [Crossref] [PubMed]
- Reginelli A, Clemente A, Renzulli M, et al. Delayed enhancement in differential diagnosis of salivary gland neoplasm. Gland Surg 2019;8:S130-5. [Crossref] [PubMed]
- Cordesmeyer R, Kauffmann P, Laskawi R, et al. The incidence of occult metastasis and the status of elective neck dissection in salivary adenoid cystic carcinoma: a single center study. Oral Surg Oral Med Oral Pathol Oral Radiol 2018;125:516-9. [Crossref] [PubMed]
- Gabelloni M, Faggioni L, Attanasio S, et al. Can Magnetic Resonance Radiomics Analysis Discriminate Parotid Gland Tumors? A Pilot Study. Diagnostics (Basel) 2020;10:900. [Crossref] [PubMed]
- Correia-Sá I, Correia-Sá M, Costa-Ferreira P, et al. Fine-needle aspiration cytology (FNAC): is it useful in preoperative diagnosis of parotid gland lesions? Acta Chir Belg 2017;117:110-4. [Crossref] [PubMed]
- Liu CC, Jethwa AR, Khariwala SS, et al. Sensitivity, Specificity, and Posttest Probability of Parotid Fine-Needle Aspiration: A Systematic Review and Meta-analysis. Otolaryngol Head Neck Surg 2016;154:9-23. [Crossref] [PubMed]
- Stodulski D, Mikaszewski B, Stankiewicz C. Signs and symptoms of parotid gland carcinoma and their prognostic value. Int J Oral Maxillofac Surg 2012;41:801-6. [Crossref] [PubMed]
- Guntinas-Lichius O, Wendt TG, Buentzel J, et al. Incidence, treatment, and outcome of parotid carcinoma, 1996-2011: a population-based study in Thuringia, Germany. J Cancer Res Clin Oncol 2015;141:1679-88. [Crossref] [PubMed]
- Inaka Y, Kawata R, Haginomori SI, et al. Symptoms and signs of parotid tumors and their value for diagnosis and prognosis: a 20-year review at a single institution. Int J Clin Oncol 2021;26:1170-8. [Crossref] [PubMed]
- Albright JT, Topham AK, Reilly JS. Pediatric head and neck malignancies: US incidence and trends over 2 decades. Arch Otolaryngol Head Neck Surg 2002;128:655-9. [Crossref] [PubMed]
- Kuzenko YV, Romanuk AM, Dyachenko OO, et al. Pathogenesis of Warthin's tumors. Interv Med Appl Sci 2016;8:41-8. [Crossref] [PubMed]
- David E, Cantisani V, De Vincentiis M, et al. Contrast-enhanced ultrasound in the evaluation of parotid gland lesions: an update of the literature. Ultrasound 2016;24:104-10. [Crossref] [PubMed]
- Heřman J, Sedláčková Z, Vachutka J, et al. Differential Diagnosis of Parotid Gland Tumors: Role of Shear Wave Elastography. Biomed Res Int 2017;2017:9234672. [Crossref] [PubMed]
- Rzepakowska A, Osuch-Wójcikiewicz E, Sobol M, et al. The differential diagnosis of parotid gland tumors with high-resolution ultrasound in otolaryngological practice. Eur Arch Otorhinolaryngol 2017;274:3231-40. [Crossref] [PubMed]
- Rooker SA, Van Abel KM, Yin LX, et al. Risk factors for subsequent recurrence after surgical treatment of recurrent pleomorphic adenoma of the parotid gland. Head Neck 2021;43:1088-96. [Crossref] [PubMed]
- Schick S, Steiner E, Gahleitner A, et al. Differentiation of benign and malignant tumors of the parotid gland: value of pulsed Doppler and color Doppler sonography. Eur Radiol 1998;8:1462-7. [Crossref] [PubMed]
- Peng L, Li N, Luo Y, et al. Ultrasonographic prediction model for benign and malignant salivary gland tumors: a preliminary study. Oral Surg Oral Med Oral Pathol Oral Radiol 2022;134:758-67. [Crossref] [PubMed]
- Ruan J, Xu X, Cai Y, et al. A Practical CEUS Thyroid Reporting System for Thyroid Nodules. Radiology 2022;305:149-59. [Crossref] [PubMed]
- Sideris A, Rao A, Maher N, et al. Acinic cell carcinoma of the salivary gland in the adult and paediatric population: a survival analysis. ANZ J Surg 2021;91:1233-9. [Crossref] [PubMed]
- Mantsopoulos K, Koch M, Fauck V, et al. Primary parotid gland lymphoma: pitfalls in the use of ultrasound imaging by a great pretender. Int J Oral Maxillofac Surg 2021;50:573-8. [Crossref] [PubMed]