Preoperative prediction of central lymph node metastasis in follicular variant of papillary thyroid carcinoma using clinical and ultrasound features
Highlight box
Key findings
• Preoperative thyroid ultrasound (US) features, integrated with clinical characteristics, enhance the prediction of central lymph node metastasis (CLNM), offering a valuable nomogram for improved risk assessment in clinical decision-making.
What is known and what is new?
• The study presents a novel nomogram model incorporating age, thyroid peroxidase antibody (TPOAb), and various US features for predicting CLNM in follicular variant of papillary thyroid carcinoma (FVPTC).
• The combination of preoperative US and clinical features demonstrates enhanced predictive efficiency for CLNM, emphasizing the importance of risk assessment for potential neck lymph node dissection.
What is the implication, and what should change now?
• The study suggests improved prediction of lymph node metastasis in FVPTC with the developed nomogram, pointing to a potential shift in clinical practice towards more accurate preoperative risk assessment. The findings imply the need for an updated approach, emphasizing preoperative US and clinical features for better risk assessment in FVPTC patients, especially regarding neck lymph node dissection.
Introduction
Thyroid nodules are extremely common manifestations and high-resolution sonography, the preferred method for detecting thyroid nodules, has considerably increased the number of cases of incidentally spotted thyroid nodules (1). Follicular epithelial cells are the most common site of thyroid carcinoma, with papillary thyroid carcinoma (PTC) being the most common pathological type; it accounts for approximately 80% of thyroid carcinoma and 85% of differentiated thyroid carcinoma cases (2). PTC can be divided into different subtypes, including the canonical subtype [classic variant of papillary thyroid carcinoma (CVPTC)], follicular subtype [follicular variant of papillary thyroid carcinoma (FVPTC)] and the highly invasive high-cell subtype (tall cell variant). FVPTC is the most common subtype besides CVPTC, accounting for 30% of all PTCs (3). The most metastasis-prone lymph nodes in patients with FVPTC are the central lymph nodes.
The ultrasound (US) characteristics and size of the thyroid nodules determine the need for fine needle aspiration (FNA), and the cytological results can help decide the therapeutic strategies for patients. However, FVPTC can exhibit cytological features similar to those of follicular lesions; therefore, FNA alone cannot accurately distinguish between them (4,5). Based on the 2015 American Thyroid Association management guidelines for adult patients with thyroid nodules and differentiated thyroid cancer, preoperative US can detect suspicious cervical lymph nodes in 20–31% of patients (1). A previous study indicated that (5), compared to PTC, FVPTC has a lower rate of CLMN (26.3% vs. 8.3%). In case of definite lymph node or distant metastasis in clinical settings, total or near-total glandular resection should be performed. Therefore, an appropriate and non-invasive tool to quantify the risk of central neck lymph node metastasis (CLNM) is warranted and may help in optimally treating patients with FVPTC. However, studies on a unified standard to measure the advantages and disadvantages of routine central neck dissection (CND) are lacking.
Therefore, herein, we constructed and validated a nomogram model and a precise, simple and objective scoring system to preoperatively predict CLNM based on clinical and US features with a quantifiable probability of CLNM. We present this article in accordance with the TRIPOD reporting checklist (available at https://gs.amegroups.com/article/view/10.21037/gs-23-492/rc).
Methods
The study design and protocol were approved by both the Ethics Committee of the Zhejiang Cancer Hospital (No. IRB-2020-287) and the Ethics Committee of the Zhejiang Provincial People’s Hospital (No. QT-2024-023). The requirement for written informed consent was waived due to the retrospective nature of this study. The study was conducted in accordance with the Declaration of Helsinki (as revised in 2013).
Patient selection
In this study, 447 participants (92 men and 355 women) who were pathologically diagnosed with FVPTC at Zhejiang Cancer Hospital and Zhejiang Provincial People’s Hospital between January 2013 and December 2022 were enrolled.
The inclusion criteria were as follows: (I) patients who underwent thyroid US at our hospitals 2 weeks before surgery; (II) those who presented with thyroid nodules and underwent CND or modified radical neck dissection and had a confirmed pathological diagnosis of FVPTC; and (III) those who did not receive any other treatment modalities for thyroid nodules before surgery.
The exclusion criteria were as follows: (I) patients with any other type of thyroid cancer; (II) those with incomplete clinical and thyroid US imaging data; and (III) those with discrepancy in the US images of the nodule and pathological diagnosis.
Based on the above-mentioned criteria, 315 patients (66 men and 249 women, mean aged 46.7±12.6 years) were finally included. From the screened patients, 221 (70%) and 94 (30%) patients were randomly divided into the training and validation cohorts, respectively. Figure 1 illustrates the flowchart of the patients participating in the present study.
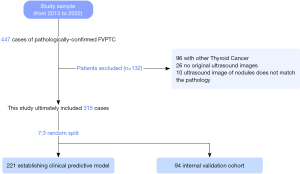
US examination and image analysis
The US images obtained from the picture archiving and communication system workstations adhered to the following requirements: (I) they encompassed most of the malignant tumour characteristics in both longitudinal and transverse planes; (II) they displayed the level of contact with the surrounding capsule; and (III) US revealed tumour characteristics such as size, location, presence of solid components, margin, echogenicity, echotexture, extracapsular invasion, calcifications and suspicious metastatic lymph nodes.
In a previous study (6), US images were retrospectively and independently reviewed by two experienced radiologists in performing thyroid US. The reviewers were blinded to the FNA results and final diagnosis.
The dimensions (diameter) of the largest lesion of multifocal FVPTCs were used to determine the tumour size in the patients. The nodules were assessed based on the US guidelines regarding composition, echogenicity, orientation, margin and echogenic foci (1). Finally, the nodules were classified based on the American College of Radiology Thyroid Imaging Reporting and Data System (ACR-TI-RADS), which is based on the classification criteria developed by Kwak et al. (7).
The clinical characteristics, including sex, age, chronic lymphocytic thyroiditis, thyroid peroxidase antibody (TPOAb), thyroglobulin (TG), thyroid-stimulating hormone (TSH), triiodothyronine (T3), thyroxine (T4), free triiodothyronine (FT3), free thyroxine (FT4) and thyroglobulin antibody (TGAb) were retrieved from the electronic medical records of the patients. The thresholds set for TPOAb is 0.0–34.0 U/mL, TG is 1.4–78 ng/mL, TSH is 0.35–5.50 µg/dL, T3 is 0.60–1.81 ng/mL, T4 is 4.50–10.90 µg/dL, FT3 is 2.30–4.20 pg/mL, FT4 is 0.89–1.76 ng/dL and TGAb is 0.0–115.0 U/mL.
Statistical analysis
R software (version 4.2.0) was used to analyse the data. In case of normal distribution, continuous variables were represented as means and standard deviations. In contrast, if the distribution was not normal, data were provided using median and interquartile range (IQR). Furthermore, frequencies and percentages were used to report categorical variables. The Student’s t-test was used for comparison between groups of quantitative variables with normal distribution, whereas the Mann-Whitney U test was performed when the distribution was not normal. Additionally, qualitative variable groups were compared using the Chi-squared test or the Fisher exact test. The agreement between the two reviewers was assessed based on weighted k statistics for qualitative variables and Pearson’s correlation coefficient for quantitative variables.
A multivariate logistic model was constructed to assess the probability of CLNM in FVPTC. This model incorporated all relevant clinical and biological factors for CLNM in FVPTC. A P value of <0.20 was considered statistically significant and used for backward regression analysis. Another multivariate logistic regression model was constructed using the same methodology; however, it was limited to US-related variables. Finally, a third model was constructed by merging the clinical and US models. The performance of these multivariate models was assessed by calculating their areas under the curve (AUCs) and confidence intervals (CIs), which were determined using the bootstrap method with 500 bootstrap replicates. AUCs were compared using the DeLong test. A predictive nomogram model for CLNM in FVPTC was developed based on the most accurate multivariate model. The assessment of the nomogram model included both discrimination and calibration, which are significant attributes when evaluating the performance of multivariate models (8).
All tests were two-sided, and a P value of <0.05 was considered statistically significant.
Results
Characteristics of the study cohorts
From January 2013 to December 2022, 447 patients who were pathologically diagnosed with FVPTC were enrolled. After excluding 132 patients, 315 patients were included in this study. All patients were randomly divided into the training and validation cohorts in a 7:3 ratio (Figure 1).
The characteristics of both cohorts are presented in Table 1. Among the 315 participants included, 249 (79.0%) were women and 66 (21.0%) were men; their average age was 46.7 (±12.6) years. Furthermore, 50 patients (15.9%) exhibited Hashimoto’s thyroiditis (chronic lymphocytic thyroiditis).
Table 1
Characteristic | Training cohort | Comparison with validation cohort | |||||
---|---|---|---|---|---|---|---|
No central lymph node metastasis (n=163) | Central lymph node metastasis (N=58) | P value | Training cohort (N=221) | Validation cohort (N=94) | P value | ||
Sex | 0.28 | 0.72 | |||||
Men | 32 (19.6) | 16 (27.6) | 48 (21.7) | 18 (19.1) | |||
Women | 131 (80.4) | 42 (72.4) | 173 (78.3) | 76 (80.9) | |||
Age (years) | 48.5±12.9 | 41.9±13.1 | 0.001 | 46.8±13.2 | 46.6±11.0 | 0.87 | |
CLT | 0.98 | 0.89 | |||||
No | 137 (84.0) | 48 (82.8) | 185 (83.7) | 80 (85.1) | |||
Yes | 26 (16.0) | 10 (17.2) | 36 (16.3) | 14 (14.9) | |||
Blood tests | |||||||
TGAb (U/mL) | 17.9 (14.6–62.3) | 23.5 (15.5–119) | 0.03 | 18.6 (15.0–90.7) | 19.0 (15.0–41.9) | 0.77 | |
TPOAb (U/mL) | 20.5 (12.0–35.8) | 25.8 (12.8–151) | 0.07 | 21.4 (12.3–42.1) | 22.2 (12.6–41.9) | 0.92 | |
FT4 (ng/dL) | 1.22 (1.14–1.37) | 1.25 (1.18–1.35) | 0.20 | 1.23 (1.15–1.37) | 1.24 (1.15–1.33) | 0.87 | |
FT3 (pg/mL) | 3.20 (2.98–3.54) | 3.24 (3.03–3.63) | 0.26 | 3.22 (3.00–3.56) | 3.16 (2.95–3.40) | 0.29 | |
T3 (ng/mL) | 1.08±0.19 | 1.07±0.19 | 0.64 | 1.07 (0.95–1.18) | 1.06 (0.96–1.17) | 0.71 | |
TSH (μg/dL) | 1.80 (1.18–2.48) | 1.62 (1.08–2.83) | 0.54 | 1.70 (1.14–2.53) | 1.71 (1.10–2.52) | 0.78 | |
TG (ng/mL) | 17.5 (6.75–44.5) | 17.8 (3.27–38.5) | 0.54 | 17.5 (5.91–42.9) | 15.0 (5.85–34.6) | 0.41 | |
T4 (μg/dL) | 8.53 (7.30–10.0) | 8.89 (7.90–10.0) | 0.50 | 8.70 (7.31–10.0) | 8.45 (7.13–9.33) | 0.13 |
Data are presented as number (percentage), mean ± standard deviation, or median (interquartile range). CLT, chronic lymphocytic thyroiditis; TPOAb, thyroid peroxidase antibody; TGAb, thyroglobulin antibody; FT4, free thyroxine; FT3, free triiodothyronine; T3, triiodothyronine; TSH, thyroid-stimulating hormone; TG, thyroglobulin; T4, thyroxine.
Table 2 summarizes the US features. The maximum nodule diameter measured using the US images of patients with CLNM was higher than that of those without CLNM, with median values of 14.0 (IQR, 10.2–24.0) mm and 10.0 (IQR, 6.0–14.5) mm, respectively (P=0.009). CLNM-associated factors were multifocal nodules (P=0.04), nodule edge invasion of the thyroid capsule (P<0.001), microcalcifications within the nodule (P<0.001) and if the nodule belonged to ACR TI-RADS category 5 (P=0.01).
Table 2
Characteristic | No central lymph node metastasis (N=163) | Central lymph node metastasis (N=58) | P value | OR (95% CI) |
---|---|---|---|---|
Diameter (mm) | 10.0 (6.0–14.5) | 14.0 (10.2–24.0) | 0.009 | 1.031 (1.008–1.056) |
Multifocal tumor | 0.04 | 2.622 (1.066–6.447) | ||
No | 151 (92.6) | 48 (82.8) | ||
Yes | 12 (7.36) | 10 (17.2) | ||
Nodule location | ||||
Left lobe of thyroid gland | 64 (39.3) | 24 (41.4) | ||
Right lobe of thyroid gland | 91 (55.8) | 29 (50.0) | 0.61 | 0.850 (0.453–1.593) |
Thyroid-isthmus | 8 (4.91) | 5 (8.62) | 0.41 | 1.667 (0.496–5.600) |
Composition | 0.30 | 0.348 (0.048–2.528) | ||
Mixed solid-cystic | 2 (1.23) | 2 (3.45) | ||
Solid | 161 (98.8) | 56 (96.6) | ||
Echogenicity | ||||
Isoechoic/hyperechoic | 7 (4.29) | 4 (6.90) | ||
Hypoechoic | 155 (95.1) | 54 (93.1) | 0.44 | 0.610 (0.172–2.164) |
Very hypoechoic | 1 (0.61) | 0 (0.00) | 0.99 | 0 (0–Inf) |
Margin | ||||
Smooth | 91 (55.8) | 12 (20.7) | ||
Irregular/lobulated | 5 (3.07) | 3 (5.17) | 0.056 | 4.550 (0.963–21.498) |
Extrathyroidal extension | 67 (41.1) | 43 (74.1) | <0.001 | 4.867 (2.385–9.934) |
Calcifications | ||||
None | 83 (50.9) | 8 (13.8) | ||
Macrocalcification | 8 (4.91) | 5 (8.62) | 0.006 | 6.484 (1.711–24.574) |
Rim calcification | 1 (0.61) | 0 (0.00) | 0.99 | 0 (0–Inf) |
Microcalcification | 71 (43.6) | 45 (77.6) | <0.001 | 6.576 (2.908–14.872) |
ACR TI-RADS | ||||
3 | 19 (11.7) | 2 (3.45) | ||
4 | 98 (60.1) | 20 (34.5) | 0.40 | 1.939(0.418-8.991) |
5 | 46 (28.2) | 36 (62.1) | 0.01 | 7.435(1.625-34.019) |
Data are presented as number (percentage) or median (interquartile range). OR, odds ratio; CI, confidence interval; ACR, American College of Radiology; TI-RADS, Thyroid Imaging Reporting and Data System.
Multivariate analysis
Table 3 presents the results of multivariate analysis of clinical and US features. Based on the clinical multivariate model, age [odds ratio (OR), 0.961; 95% CI: 0.938–0.985; P=0.001) and TPOAb (OR, 1.001; 95% CI: 1.000–1.002; P=0.11) were significantly associated with CLNM. According to the US model, diameter (OR, 1.032; 95% CI: 1.009–1.065; P=0.047), irregular/lobulated margin (OR, 3.385; 95% CI: 0.647–17.714: P=0.15), extrathyroidal extension (OR, 3.870; 95% CI: 1.720–8.707; P=0.001), nodules with macrocalcifications (OR, 6.854; 95% CI: 1.613–29.117; P=0.009), nodules with microcalcification (OR, 4.026; 95% CI: 1.617–10.023; P=0.003) and ACR TI-RADS category 5 (OR, 2.484; 95% CI: 0.398–15.512; P=0.33) were associated with CLNM. The integration of both clinical and US variables in the model revealed that age, TPOAb, maximum nodule diameter, irregular/lobulated margin, extrathyroidal extension, nodules with macrocalcifications, nodules with microcalcification and ACR TI-RADS category 5 were the factors associated with CLNM. The above variables were used to develop the nomogram model (Figure 2).
Table 3
Features | Clinical model | US model | Combined model (clinical + US) | |||||
---|---|---|---|---|---|---|---|---|
OR (95% CI) | P value | OR (95% CI) | P value | OR (95% CI) | P value | |||
Clinical | ||||||||
Age | 0.961 (0.938–0.985) | 0.001 | 0.958 (0.931–0.986) | 0.003 | ||||
TPOAb | 1.001 (1.000–1.002) | 0.11 | 1.001 (1.000–1.002) | 0.06 | ||||
US | ||||||||
Diameter (mm) | 1.032 (1.009–1.065) | 0.047 | 1.031 (1.002–1.060) | 0.03 | ||||
Irregular/lobulated | 3.385 (0.647–17.714) | 0.149 | 4.246 (0.747–24.137) | 0.10 | ||||
Extrathyroidal extension | 3.870 (1.720–8.707) | 0.001 | 4.393 (1.851–10.423) | 0.001 | ||||
Macrocalcification | 6.854 (1.613–29.117) | 0.009 | 7.838 (1.839–33.399) | 0.005 | ||||
Microcalcification | 4.026 (1.617–10.023) | 0.003 | 3.690 (1.480–9.198) | 0.005 | ||||
ACR TI–RADS category 5 | 2.484 (0.398–15.512) | 0.330 | 3.505 (0.540–22.745) | 0.19 |
US, ultrasound; OR, odds ratio; CI, confidence interval; TPOAb, thyroid peroxidase antibody; ACR, American College of Radiology; TI-RADS, Thyroid Imaging Reporting and Data System.
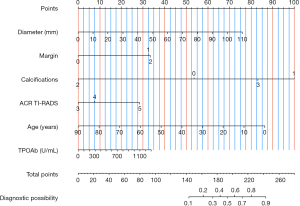
Evaluation of the prognostic performance of the constructed models
For predicting CLNM in FVPTC, the AUC values were as follows: 0.841 (95% CI: 0.788–0.895), combined model; 0.655 (95% CI: 0.580–0.744), clinical model and 0.811 (95% CI: 0.755–0.875), US model. This result suggests that the combined model exhibits a significantly better predictive performance than the clinical and US models (P<0.001 and P=0.072, respectively).
The net reclassification improvement of the combined model compared with the US model was 0.065 (95% CI: −0.057 to 0.187; P=0.30); the integrated discrimination improvement was 0.055 (95% CI: 0.017–0.093; P=0.004). No evidence regarding differences between both reviewers and the consensus reading was discovered in the US or combination model.
Predictive nomogram model and internal validation
The ability of the combined model to predict CLNM in FVPTC was evaluated in the validation cohort comprising 94 patients (Table 1 and Figure 3). The AUC values in the validation cohort were as follows: 0.735 (95% CI: 0.621–0.872), combined model; 0.486 (95% CI: 0.349–0.642), clinical model and 0.750 (95% CI: 0.646–0.880), US model. The calibration plots illustrated in Figure 3 display a good agreement between the predicted and observed probabilities in both study cohorts.
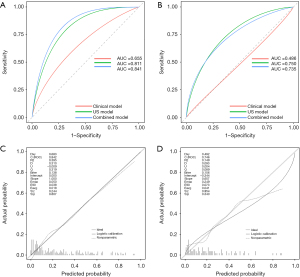
In the prediction of CLNM in FVPTC, the accuracy (ACC) was 0.751 (95% CI: 0.749–0.753) for the training cohort and 0.745 (95% CI: 0.741–0.749) for the validation cohort; sensitivity (SEN) was 0.776 (95% CI: 0.669–0.883) and 0.636 (95% CI: 0.435–0.837); square prediction error (SPE) was 0.742 (95% CI: 0.675–0.809) and 0.778 (95% CI: 0.682–0.874) and kappa was 0.446 (95% CI: 0.327–0.556) and 0.368 (95% CI: 0.165–0.571).
Figure 4 illustrates the decision curve and suggests that the combined model exhibits higher predictability than the clinical and US models, within a probability threshold range of 0.1–0.9. Figure 5 illustrates the instances of the clinical utilisation of the nomogram.
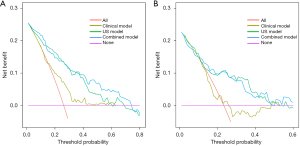
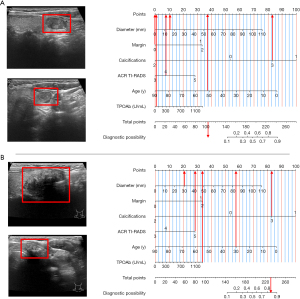
Discussion
In the present study, we constructed and validated a nomogram model based on the clinical and US characteristics of patients with FVPTC for customized preoperative prediction of CLNM. Compared with the clinical or US prediction models, the combined nomogram model demonstrated a notable discriminatory ability for CLNM prediction in both the training and validation cohorts. Furthermore, the nomogram displayed a favourable concordance in calibration and exhibited the most optimal net benefit within a reasonable spectrum of the threshold probabilities. These findings substantiate the advantages of our radiomic nomogram model in aiding the personalised and preoperative prediction of CLNM in patients with FVPTC.
Preoperative imaging in patients with FVPTC to identify CLNM is critical for their preoperative planning and management. Among the various existing imaging modalities, US imaging is the preferred modality for assessing thyroid nodules and lymph nodes in patients suspected of having thyroid cancer. FVPTC, characterized by its follicular growth pattern, poses a challenge in preoperative imaging because of its potential for vascular invasion and distant metastasis. Therefore, imaging techniques play vital roles in determining the extent of the disease and identifying the presence of CLNM. In recent years, US imaging has garnered considerable popularity owing to its non-invasive nature, cost-effectiveness and ability to provide real-time imaging. Regarding the evaluation of lymph nodes in individuals diagnosed with thyroid cancer, US imaging possesses several advantages, including high-resolution imaging, practicability, sensitivity in identifying calcifications and capability to assess nodal vascularity. However, the effectiveness of US imaging relies on the expertise of the operator, and limitations exist in the evaluation of retropharyngeal and mediastinal lymph nodes. Regrettably, the detection rate of CLNM remains unsatisfactory.
In our dataset, 92 tumours exhibited CLNM; however, US imaging only identified 76.1% (70 of 92 tumours) of such cases as positive. The AUC for the imaging diagnosis alone was only 0.799. On the other hand, the clinical- and US feature-based nomogram model, developed by integrating six independent risk factors, including age, TPOAb, tumour diameter, margin, calcification and TI-RADS, exhibited a higher AUC value of 0.841. Differentiating between malignant and benign nodules based on morphological features continues to be difficult because of the limited number of lymph node metastasis cases and the presence of nonspecific inflammatory hyperplasia (9).
Although several studies have identified the independent risk factors that contribute to the development of a predictive model for lymph node metastasis in PTC (10-14), only a few studies have classified FVPTC as a separate category to predict the risk factors for CLNM. In the present study, we investigated FVPTC because it belongs to the follicular tumour type and has different US features from PTC. Hence, the construction and validation of a novel model, different from that of PTC, was necessary. Therefore, we constructed the prediction model based on a comprehensive assessment of preoperative clinical indicators and US imaging features as well as four independent US characteristics to provide a more precise evaluation of the risk of lymph node metastasis in FVPTC.
A study has reported that a ‘U-shaped’ association is present between age and probability of lymph node metastasis in PTC, suggesting that both younger and older patients are susceptible to lymph node metastasis (15). However, our results indicated that younger patients were more likely to develop CLNM, which is consistent with the results of Shukla et al. (16), Zhao et al. (17), and Xu et al. (18). This can be linked to the increased basal metabolic rate observed in younger patients and their propensity towards events such as genetic abnormalities (19). Many studies have consistently reported that there is no notable disparity in histological type between patients diagnosed with FVPTC exhibiting lymph node metastasis and those who do not (19,20); this is consistent with the results of our study. Previous studies have shown that the aspect ratio in ARC TI-RADS classification is not suitable for follicular tumors because of their large tumor diameter (21,22). Therefore, in our study, diameter was used to represent the shape of nodules. Furthermore, we found that maximum nodule diameter was an independent risk factor for FVPTC lymph node metastasis, which is consistent with the findings reported by Sun et al. (23) and Feng et al. (24). Many studies have investigated the association between CLNM and US features of tumours. In addition, the presence of calcification and a shorter distance between the tumour and capsule have been reported to be indicated of lymph node metastasis (25). Many studies have reported that the formation of microcalcifications is caused by the rapid proliferation of cancer cells and is significantly associated with the incidence of CLNM (25,26). The psammoma body is the main component of histological results in thyroid cancer. A study showed that there is a close relationship between the psammoma body and calcification (26), which makes calcified FVPTC nodules more likely to have the characteristics of PTC and more likely to produce CLNM. Microcalcification is positively linked to the invasiveness of PTC, our findings also suggested that calcification and nodules with extrathyroidal extension were associated with CLNM. Moreover, studies have demonstrated that in patients with PTC, the quantity and positioning of PTC can affect the occurrence of lymph node metastasis (27,28). However, in our study, the number of nodules and their location were not considered independent factors.
We aimed to establish a clinical- and US feature-based nomogram model to predict the preoperative occurrence of CLNM. We examined the correlation between CLNM and clinically accessible characteristics via univariate analysis. The independent risk factors for CLNM in patients with FVPTC were identified as US characteristics via multivariate logistic regression analysis. Subsequently, the nomogram model was constructed based on the results of multivariate analysis. The predictive ability of the nomogram model to generate a personalised CLNM probability was established. Both surgeons and radiologists can individually and preoperatively predict CLNM risk. This aligns with the contemporary trajectory of personalised precision medicine (28). Regarding the clinical application of the nomogram, we used decision curve analysis (DCA) to assess the potential advantages of using nomogram-assisted predictions for patient outcomes. DCA quantifies net benefit by considering threshold probability. The overall benefit is determined by summing the advantages (true positives) and subtracting the disadvantages (false positives) while considering the comparative harm caused by false-positive and false-negative outcomes. In the present study, we observed that patients with a nomogram score of ≤240 constituted the subset of patients with FVPTC with a low risk of CLNM. Subsequently, these patients may benefit from abstaining from CLNM dissection, indicating a favourable clinical utility.
Our study has three limitations. First, the study was retrospective in nature, and after applying the inclusion/exclusion criteria, the sample size was relatively small (315 patients). Second, a potential for variability was observed in the interpretation of histopathological examination results, specifically between observers or centres (namely SMC and SNUH). Third, our nomogram model solely relies on histopathologically verified postoperative data, thereby introducing a predisposition towards patients presenting with FVPTC-related aggressive features.
Conclusions
We constructed a novel nomogram model based on age, TPOAb and US features, namely maximum nodule diameter, margin, calcifications and ACR TI-RADS, to predict CLNM. We have shown that preoperative US provides useful features for the prediction of CLNM in FVPTC. The combination of US and clinical model demonstrated the better predictive efficiency on CLNM. The risk assessment of CLNM is crucial as it may require neck lymph node dissection. Our developed model exhibits modest sensitivity and specificity for predicting CLNM in our patient cohort with FVPTC.
Acknowledgments
Funding: This work was supported by
Footnote
Reporting Checklist: The authors have completed the TRIPOD reporting checklist. Available at https://gs.amegroups.com/article/view/10.21037/gs-23-492/rc
Data Sharing Statement: Available at https://gs.amegroups.com/article/view/10.21037/gs-23-492/dss
Peer Review File: Available at https://gs.amegroups.com/article/view/10.21037/gs-23-492/prf
Conflicts of Interest: All authors have completed the ICMJE uniform disclosure form (available at https://gs.amegroups.com/article/view/10.21037/gs-23-492/coif). The authors have no conflicts of interest to declare.
Ethical Statement: The authors are accountable for all aspects of the work in ensuring that questions related to the accuracy or integrity of any part of the work are appropriately investigated and resolved. The study was approved by both the Ethics Committee of the Zhejiang Cancer Hospital (No. IRB-2020-287) and the Ethics Committee of the Zhejiang Provincial People’s Hospital (No. QT-2024-023). The requirement for written informed consent was waived due to the retrospective nature of this study. The study was conducted in accordance with the Declaration of Helsinki (as revised in 2013).
Open Access Statement: This is an Open Access article distributed in accordance with the Creative Commons Attribution-NonCommercial-NoDerivs 4.0 International License (CC BY-NC-ND 4.0), which permits the non-commercial replication and distribution of the article with the strict proviso that no changes or edits are made and the original work is properly cited (including links to both the formal publication through the relevant DOI and the license). See: https://creativecommons.org/licenses/by-nc-nd/4.0/.
References
- Haugen BR, Alexander EK, Bible KC, et al. 2015 American Thyroid Association Management Guidelines for Adult Patients with Thyroid Nodules and Differentiated Thyroid Cancer: The American Thyroid Association Guidelines Task Force on Thyroid Nodules and Differentiated Thyroid Cancer. Thyroid 2016;26:1-133. [Crossref] [PubMed]
- Schneider DF, Chen H. New developments in the diagnosis and treatment of thyroid cancer. CA Cancer J Clin 2013;63:374-94. [Crossref] [PubMed]
- Baloch ZW, Shafique K, Flanagan M, et al. Encapsulated classic and follicular variants of papillary thyroid carcinoma: comparative clinicopathologic study. Endocr Pract 2010;16:952-9. [Crossref] [PubMed]
- Cibas ES, Ali SZ. The 2017 Bethesda System for Reporting Thyroid Cytopathology. Thyroid 2017;27:1341-6. [Crossref] [PubMed]
- Durante C, Hegedüs L, Czarniecka A, et al. 2023 European Thyroid Association Clinical Practice Guidelines for thyroid nodule management. Eur Thyroid J 2023;12:e230067. [Crossref] [PubMed]
- Raffaelli M, De Crea C, Sessa L, et al. Risk factors for central neck lymph node metastases in follicular variant vs. classic papillary thyroid carcinoma. Endocrine 2018;62:64-70. [Crossref] [PubMed]
- Kwak JY, Han KH, Yoon JH, et al. Thyroid imaging reporting and data system for US features of nodules: a step in establishing better stratification of cancer risk. Radiology 2011;260:892-9. [Crossref] [PubMed]
- Alba AC, Agoritsas T, Walsh M, et al. Discrimination and Calibration of Clinical Prediction Models: Users' Guides to the Medical Literature. JAMA 2017;318:1377-84. [Crossref] [PubMed]
- Ji GW, Zhang YD, Zhang H, et al. Biliary Tract Cancer at CT: A Radiomics-based Model to Predict Lymph Node Metastasis and Survival Outcomes. Radiology 2019;290:90-8. [Crossref] [PubMed]
- Liu W, Cheng R, Ma Y, et al. Establishment and validation of the scoring system for preoperative prediction of central lymph node metastasis in papillary thyroid carcinoma. Sci Rep 2018;8:6962. [Crossref] [PubMed]
- Li X, Zhang H, Zhou Y, et al. Risk factors for central lymph node metastasis in the cervical region in papillary thyroid carcinoma: a retrospective study. World J Surg Oncol 2021;19:138. [Crossref] [PubMed]
- Zheng CM, Ji YB, Song CM, et al. Number of Metastatic Lymph Nodes and Ratio of Metastatic Lymph Nodes to Total Number of Retrieved Lymph Nodes Are Risk Factors for Recurrence in Patients With Clinically Node Negative Papillary Thyroid Carcinoma. Clin Exp Otorhinolaryngol 2018;11:58-64. [Crossref] [PubMed]
- Xue S, Zhang L, Pang R, et al. Predictive Factors of Central-Compartment Lymph Node Metastasis for Clinical N0 Papillary Thyroid Carcinoma With Strap Muscle Invasion. Front Endocrinol (Lausanne) 2020;11:511. [Crossref] [PubMed]
- Deng Y, Zhang J, Wang J, et al. Risk factors and prediction models of lymph node metastasis in papillary thyroid carcinoma based on clinical and imaging characteristics. Postgrad Med 2023;135:121-7. [Crossref] [PubMed]
- Thompson AM, Turner RM, Hayen A, et al. A preoperative nomogram for the prediction of ipsilateral central compartment lymph node metastases in papillary thyroid cancer. Thyroid 2014;24:675-82. [Crossref] [PubMed]
- Shukla N, Osazuwa-Peters N, Megwalu UC. Association Between Age and Nodal Metastasis in Papillary Thyroid Carcinoma. Otolaryngol Head Neck Surg 2021;165:43-9. [Crossref] [PubMed]
- Zhao W, He L, Zhu J, et al. A nomogram model based on the preoperative clinical characteristics of papillary thyroid carcinoma with Hashimoto's thyroiditis to predict central lymph node metastasis. Clin Endocrinol (Oxf) 2021;94:310-21. [Crossref] [PubMed]
- Xu SY, Yao JJ, Zhou W, et al. Clinical characteristics and ultrasonographic features for predicting central lymph node metastasis in clinically node-negative papillary thyroid carcinoma without capsule invasion. Head Neck 2019;41:3984-91. [Crossref] [PubMed]
- Yan C, He X, Chen Z, et al. Central Compartment Lymph Nodes Have Distinct Metastatic Patterns in Different Age Groups. Front Endocrinol (Lausanne) 2022;13:807431. [Crossref] [PubMed]
- Wang L, Chen J, Yuan X, et al. Lymph node metastasis of papillary thyroid carcinoma in the context of Hashimoto's thyroiditis. BMC Endocr Disord 2022;22:12. [Crossref] [PubMed]
- Lin Y, Lai S, Wang P, et al. Performance of current ultrasound-based malignancy risk stratification systems for thyroid nodules in patients with follicular neoplasms. Eur Radiol 2022;32:3617-30. [Crossref] [PubMed]
- Yang J, Sun Y, Li X, et al. Diagnostic performance of six ultrasound-based risk stratification systems in thyroid follicular neoplasm: A retrospective multi-center study. Front Oncol 2022;12:1013410. [Crossref] [PubMed]
- Sun F, Zou Y, Huang L, et al. Nomogram to Assess the Risk of Central Cervical Lymph Node Metastasis in Patients With Clinical N0 Papillary Thyroid Carcinoma. Endocr Pract 2021;27:1175-82. [Crossref] [PubMed]
- Feng JW, Hong LZ, Wang F, et al. A Nomogram Based on Clinical and Ultrasound Characteristics to Predict Central Lymph Node Metastasis of Papillary Thyroid Carcinoma. Front Endocrinol (Lausanne) 2021;12:666315. [Crossref] [PubMed]
- Han DY, Sohn YM, Seo M, et al. Shear-wave elastography in thyroid ultrasound: Can be a predictor of extrathyroidal extension and cervical lymph node metastasis in papillary thyroid carcinoma? Medicine (Baltimore) 2020;99:e23654. [Crossref] [PubMed]
- Chang L, Zhang Y, Zhu J, et al. An integrated nomogram combining deep learning, clinical characteristics and ultrasound features for predicting central lymph node metastasis in papillary thyroid cancer: A multicenter study. Front Endocrinol (Lausanne) 2023;14:964074. [Crossref] [PubMed]
- Yu QA, Ma DK, Liu KP, et al. Clinicopathologic risk factors for right paraesophageal lymph node metastasis in patients with papillary thyroid carcinoma. J Endocrinol Invest 2018;41:1333-8. [Crossref] [PubMed]
- Choudhury P, Gupta M. Personalized & Precision Medicine in Cancer: A Theranostic Approach. Curr Radiopharm 2017;10:166-70. [Crossref] [PubMed]