Predicting papillary thyroid microcarcinoma in American College of Radiology Thyroid Imaging Reporting and Data System (ACR TI-RADS) 3 nodules: radiomics analysis based on intratumoral and peritumoral ultrasound images
Highlight box
Key findings
• The ultrasound-based nomogram consisting of intratumoral and peritumoral radiomics features as well as clinical information has high diagnostic performance in the early discrimination of papillary thyroid microcarcinoma in American College of Radiology Thyroid Imaging Reporting and Data System (ACR TI-RADS) 3 nodules.
What is known and what is new?
• Only a limited number of published studies have specifically addressed the application of ultrasound radiomics in predicting malignancy of thyroid micronodules. Besides, previous radiomics studies in thyroid nodules mainly focused on the intratumoral region alone. There still exists a research gap in the field of ultrasound radiomics as applied to predicting papillary thyroid microcarcinoma (PTMC).
• This study is the first to construct and assess the performance of the ultrasound-based radiomics model, combined intratumoral and peritumoral radiomics features as well as clinical features, for predicting PTMC in patients with ACR TI-RADS 3 nodules.
What is the implication, and what should change now?
• The ultrasound-based nomogram, incorporating both intratumoral and peritumoral radiomics features, along with clinical data, demonstrates effectiveness in PTMC in ACR TI-RADS 3 nodules. Early and accurate detection of papillary thyroid microcarcinoma can help to promote optimal treatment and alleviate the burden on patients. To strengthen the reliability of our nomogram predictive model, it is imperative to conduct a multicenter survey with a larger sample size for further validation.
Introduction
Papillary thyroid microcarcinoma (PTMC) is defined by the World Health Organization as a small papillary thyroid cancer measuring 10 mm or less in the greatest dimension of the tumor (1). In the past several decades, there has been a substantial increase in the incidence of thyroid cancer (2). The rise has been almost entirely due to papillary carcinoma (3). PTMC accounts for a significant proportion of this rise (4). Some investigators have suggested that the increasing incidence may be attributed to overdiagnosis resulting from enhanced surveillance and diagnostic capabilities (5,6). Moreover, the majority of PTMC patients remain asymptomatic, with inert behavior and a generally favorable prognosis (7). Active surveillance (AS) has been suggested as a management strategy for low-risk PTMC, but controversy exists with its adoption as not all PTMCs exhibit a uniformly benign prognosis (8). Besides, a growing body of evidence substantiates a true increase in the occurrence of thyroid cancer (9). Lim et al. found that incidence-based thyroid cancer mortality was also increasing (9). It is important to underscore that while many patients with PTMC have favorable prognosis, a subset of them faces worse prognosis with tumor recurrence or distant metastasis (10). Distant metastasis in PTMC is rare but fatal (11). Ultrasonography is widely used as a noninvasive and easily accessible first-step diagnostic method for the detection and evaluation of nodular thyroid disease (12). The American College of Radiology Thyroid Imaging Reporting and Data System (ACR TI-RADS) is proposed as a method for stratifying the risk of malignancy in thyroid nodules, founded upon the comprehensive assessment of ultrasound (US) features that are categorized into five distinct domains: composition, echogenicity, shape, margin, and echogenic foci (12). All thyroid nodules categorized as ACR TI-RADS 3 exhibited non-solid characteristics, lacked significant hypoechogenicity, and demonstrated well-defined margins, absence of microcalcifications, and an aspect ratio of ≤1 (12). For an ACR TI-RADS 3 lesion, follow-up imaging is available at the first, third, and fifth year. If there is no change in size, imaging can stop at the fifth year, as stability over this time reliably indicates that the nodule has a benign behavior (13). However, a study found a proportion of PTMCs in patients who underwent thyroid surgery for presumed benign thyroid disease (14). As a result, it is still necessary to find an effective method for early recognition of PTMC. Fine-needle aspiration biopsy (FNAB) of the thyroid is considered to be the most effective diagnostic tool for thyroid nodules (15). While FNAB is invaluable for detecting thyroid malignancy, it is both a costly and invasive method (16). Moreover, the size of micropapillary thyroid carcinoma is too small, resulting in low accuracy of FNAB.
Radiomics, defined as quantitative features extracted from radiological images through sophisticated data characterization algorithms, serves the purpose of developing prognostic prediction tools and therapeutic decision support tools for cancer (17). Since it was proposed, the field of radiomics has witnessed a remarkable surge in research endeavors, with a growing body of literature dedicated to enhancing accurate cancer diagnosis and therapeutic strategies (18). To our current knowledge, only one study has specifically addressed the application of US radiomics in predicting malignancy of thyroid micronodules (19). There exists a research gap in the field of US radiomics as applied to PTMC. In the present study, we aimed to develop an US-based nomogram that combines intratumoral and peritumoral radiomics features as well as clinical features for predicting PTMC in ACR TI-RADS 3 nodules, and validate its performance. We present this article in accordance with the TRIPOD reporting checklist (available at https://gs.amegroups.com/article/view/10.21037/gs-24-30/rc).
Methods
Study population and data acquisition
Between January 2019 and October 2022, a retrospective review was conducted on a cohort of 221 patients who presented with ACR TI-RADS 3 nodules. These patients were subsequently pathologically diagnosed with either PTMC or benign thyroid nodules. The study was conducted in accordance with the Declaration of Helsinki (as revised in 2013) and was approved by the institutional ethics committee of The Second Affiliated Hospital of Wenzhou Medical University (No. 2023-K-43-01). Individual consent for this retrospective analysis was waived. However, prior to surgery or biopsy, written consent was obtained from all patients. The inclusion criteria comprised: (I) patients with confirmed pathological results of either PTMC or benign thyroid nodules; (II) patients with clear B-mode US images available for analysis; (III) patients with comprehensive clinical information. The exclusion criterion is the presence of a prior history of other malignancies or coexisting malignancies among the patients (Figure 1).
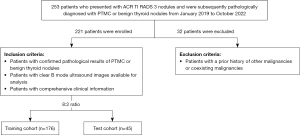
US examination and interpretation of US features
The US examinations were conducted by board-certified radiologists who possessed expertise in superficial tissue US imaging and had more than five years of experience in the field. Multiple US machines were utilized to conduct these examinations, including the Resona7 by Mindray (Shenzhen, China), the Aplio 500 by Toshiba Medical Systems (Tokyo, Japan), and the ESAOTE (MyLab 90 X-vision, Genoa, Italy), all outfitted with suitable high-frequency probes. Images capturing the largest cross-section of the nodules in the longitudinal plane were acquired for further analysis.
Two seasoned radiologists, each with at last 5 years of experience in superficial tissue ultrasonography, independently assessed all images without access to the patients’ clinical data or final diagnoses. The reassessment of the US features involved evaluating tumor dimensions, echogenicity (hypoechoic, iso/hyperechoic, or mixed), echotexture (homogeneous or heterogeneous), margin characteristics (well-defined or ill-defined), and the identification of calcifications (absent, macrocalcification, or microcalcification), as well as determining the aspect ratio (>1 or ≤1). The radiomics models were subsequently constructed based on manually-segmented regions of interest that were confirmed by two radiologists. Prior to feature extraction, the intensities of all images were normalized.
Lesion segmentation and radiomics features extraction
The radiomics analysis workflow encompassed several pivotal steps, including lesion segmentation, feature extraction, feature selection, and model construction, as depicted in Figure 2. The study cohort was randomly split into a training cohort and a test cohort, following an 8:2 ratio. The region of interest (ROI) was meticulously delineated by an experienced radiologist and subsequently verified by another, both of whom were kept blind to any clinical information. To achieve this, the tumor region in each US image was manually outlined using ITK-SNAP software (http://www.itksnap.org; version 4.0.1) around the lesion’s contour. To obtain the peritumoral regions, a standard morphological dilation operation was applied to the delineated tumor contour, expanding it by 5 mm. This operation was executed through a custom program developed in Matlab 2016b (MathWorks, Natick, MA, USA). Consequently, for each US slice, three distinct ROI images were obtained: the intratumor ROI, the peritumor ROI, and a combined ROI merging both intratumoral and peritumoral regions. Prior to feature extraction, the intensities of all US images were normalized to standardize the gray intensity values. Following this, a comprehensive total of 3,122 radiomics features were subsequently extracted. These features were categorized into three distinct groups: geometry, intensity, and texture. Geometry features characterize the three-dimensional (3D) shape attributes of the tumor, while intensity features encapsulate first-order statistical distributions of voxel intensities within the tumor. Texture features, on the other hand, encapsulate patterns derived from second- and high-order spatial distributions of intensities. A variety of methods were employed to extract texture features, including gray-level dependence matrix (GLDM), gray-level size zone matrix (GLSZM), gray-level run length matrix (GLRLM), gray-level co-occurrence matrix (GLCM) and neighbouring gray tone difference matrix (NGTDM). For the implementation of feature extraction, we utilized the Pyradiomics 2.2.0 open-source python package. More detailed information about this package can be found at http://www.radiomics.io/pyradiomics.html.
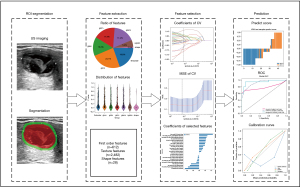
Feature selection and radiomics model establishment
In order to analyze our data statistically, we employed different tests based on the distribution of the features. For features that followed a normal distribution, we utilized the Student t-test, while for non-normally distributed features, we applied the Mann-Whitney U test. Features with a P value below 0.05 were retained for further analysis. To assess the correlation between features with high repeatability, Spearman’s rank correlation coefficient was employed. If the correlation coefficient exceeded 0.9 between any two features, we retained one of them. In order to ensure a comprehensive depiction of the features, we implemented a greedy recursive deletion strategy for feature filtering. This involved iteratively removing features with the highest redundancy in the current set. Ultimately, we identified and retained a total of 23 relevant features.
For the construction of the radiomics signature, we utilized the least absolute shrinkage and selection operator (LASSO) regression model to the discovery dataset. Based on the regularization weight λ, the LASSO model effectively shrinks regression coefficients towards zero and effectively setting the coefficients of many irrelevant features to exactly zero. To find the optimal value of λ, we performed 10-fold cross-validation with minimum criteria, selecting the λ value that minimized the cross-validation error. Features with nonzero coefficients were used for regression model fitting and amalgamated to conduct the radiomics signature. Each patient was assigned a radiomics score by calculating a linear combination of the retained features, weighted by their corresponding model coefficients. We used the Python scikit-learn package for LASSO regression modeling. Following the LASSO feature screening, we input the final features into the machine learning model. We used K-nearest neighbor (KNN) algorithm to construct a risk model based on the intratumor ROI, the peritumor ROI, and the combined ROI, respectively. For obtaining the final Rad Signature, we adopted five-fold cross verification was adopted.
The building of the clinical model and radiomics-clinical nomogram
Given that thyroid nodules categorized as ACR TI-RADS 3 exhibited clearly defined margins, an aspect ratio of ≤1 and absence of microcalcifications, we deliberately chose to utilize US features such as tumor dimension, echotexture and echogenicity in order to distinguish between benign thyroid nodules and PTMC. The process of constructing the clinical model followed a similar approach to the radiomics model. The features used for clinical modeling were selected based on the univariate and multivariate analysis with a P value <0.05. We utilized the same machine learning model as the one used in the radiomics model construction process. Additionally, we set the test cohort to fixed for a fair comparison and performed five-fold cross-validation. By integrating the radiomics signature and the important clinical predictor, we created an easy-to-use radiomics-clinical nomogram model for potential clinical applications.
Statistical analysis
In order to assess the equivalence of patient attributes across different cohorts, we employed independent t-tests to analyze normally distributed data and used Mann-Whitney U tests to express non-normally distributed data as medians (interquartile range). Categorical variables were analyzed using Chi-squared tests. To assess the predictive power of the models, we plotted receiver operating characteristic (ROC) curves, calculated the area under the curve (AUC), and determined the equilibrium sensitivity and specificity at the cut-off point that maximized the value of the Youden index. We tested the performance of the models in both the training and test cohorts. To compare the AUCs, we employed Delong’s test between the predictive models. Furthermore, we conducted the calibration curve to evaluate the clinical applicability of these models. This analysis assessed the potential benefits of using the models in clinical decision-making. All statistical analyses were performed using SPSS (version 21.0; IBM Corp.), and statistical significance was determined as a two-sided P value <0.05.
Results
Baseline characteristics of patients
The study enrolled a total of 130 patients with benign thyroid nodules and 91 patients with PTMC. In order to compare the clinical characteristics of these patients, we performed relevant statistical analyses such as independent sample t-tests, Mann-Whitney U tests, or Chi-squared tests, as deemed appropriate for each variable. The baseline clinical information of all patients is presented in Table 1.
Table 1
Parameter | Training cohort (n=176) | Testing cohort (n=45) | |||||
---|---|---|---|---|---|---|---|
Benign (n=105) | Malignant (n=71) | P value | Benign (n=25) | Malignant (n=20) | P value | ||
Age (years) | 49.86±12.03 | 50.44±10.09 | 0.75 | 50.00±13.65 | 51.20±11.63 | 0.70 | |
Tumor dimension (mm) | 29.15±13.35 | 16.61±13.59 | <0.001* | 26.80±12.94 | 13.54±12.39 | <0.001* | |
Sex | 0.28 | 0.43 | |||||
Female | 88 (83.81) | 54 (76.06) | 20 (80.00) | 13 (65.00) | |||
Male | 17 (16.19) | 17 (23.94) | 5 (20.00) | 7 (35.00) | |||
Echogenicity | <0.001* | 0.11 | |||||
Hypoechoic | 35 (33.33) | 48 (67.61) | 9 (36.00) | 13 (65.00) | |||
Iso/hyperechoic | 8 (7.62) | 7 (9.86) | 2 (8.00) | 2 (10.00) | |||
Mixed | 62 (59.05) | 16 (22.54) | 14 (56.00) | 5 (25.00) | |||
Echotexture | 0.009* | 0.79 | |||||
Homogeneous | 9 (8.57) | 16 (22.54) | 2 (8.00) | 3 (15.00) | |||
Heterogeneous | 96 (91.43) | 55 (77.46) | 23 (92.00) | 17 (85.00) |
Data are presented as a number (%) or mean ± standard deviation. *, P<0.05.
Establishment and evaluation of the radiomics model
We manually extracted a total of 3,122 features from both the tumoral and peritumoral regions, encompassing 612 first-order features, 28 shape features, and the last are texture features, as depicted in Figure 3A. A detailed list of these handcrafted features can be found in https://cdn.amegroups.cn/static/public/gs-24-30-1.xlsx, https://cdn.amegroups.cn/static/public/gs-24-30-2.xlsx. These features were extracted using an in-house feature analysis program implemented in Pyradiomics, accessible at http://pyradiomics.readthedocs.io. Figure 3B provides an overview of all the features along with their corresponding P value results.
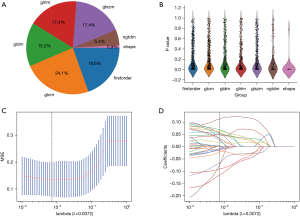
For constructing the Rad-score, we employed a LASSO logistic regression model to identify the nonzero coefficients. The coefficients and mean standard error (MSE) obtained from 10-fold validation process are depicted in Figure 3C,3D. Following the selection process, a cumulative of 23 features exhibited non-zero coefficient values, illustrated in detail in Figure 4.
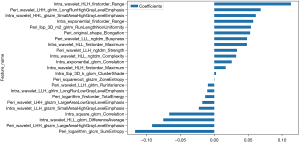
We used KNN model as the base model for constructing both the radiomics signature and clinical signature. Figure 5 shows favorable predictive performance of radiomics models in both training and test cohorts. In the training cohort, the combined-region model achieved a sensitivity of 0.887, a specificity of 0.895 and an AUC value of 0.955, which was higher than that of intratumor-region model (AUC =0.948) and peritumor-region model (AUC =0.889) (Figure 5A, Table 2). In the corresponding test cohort, the combined-region model also obtained an excellent result, with highest AUC value of 0.923 [95% confidence interval (CI): 0.854–0.992], followed by the intratumor-region model (AUC =0.851, 95% CI: 0.730–0.972) and the peritumor-region model (AUC =0.798, 95% CI: 0.669–0.927) (Figure 5B, Table 2). In addition, sensitivity and specificity value of the combined-region model in training cohort were 0.887 and 0.895, which in test cohort were 0.850 and 0.800, respectively.
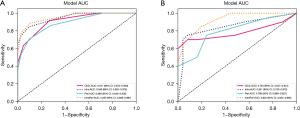
Table 2
Model | AUC | 95% CI | Sensitivity | Specificity | Cohort |
---|---|---|---|---|---|
Training cohort | |||||
Clinical model | 0.911 | 0.872–0.950 | 0.915 | 0.733 | Train |
IntraPeri model | 0.955 | 0.929–0.981 | 0.887 | 0.895 | Train |
Nomogram | 0.961 | 0.934–0.988 | 0.930 | 0.895 | Train |
Testing cohort | |||||
Clinical model | 0.784 | 0.627–0.941 | 0.700 | 0.920 | Test |
IntraPeri model | 0.923 | 0.854–0.992 | 0.850 | 0.800 | Test |
Nomogram | 0.950 | 0.883–1.000 | 0.950 | 0.920 | Test |
Sensitivity and specificity were evaluated at the cutoff value that produced the highest Youden index value. AUC, area under receiver operating curve; CI, confidence interval; IntraPeri model, intratumoral and peritumoral combined-region model.
Establishment and performance of the clinical model and radiomics-clinical nomogram
In univariate and multivariate analysis, we employed the P value (P<0.05) of the features in the training cohort to select the features to establish the clinical model. Among these features, only tumor dimension and echogenicity met this condition (Table 3). Therefore, the two features were utilized to construct the clinical model, which achieved an AUC of 0.911 (95% CI: 0.872–0.950) in the training cohort. In the test cohort, the AUC was 0.784 (95% CI: 0.627–0.941) (Figure 6, Table 2).
Table 3
Parameter | Univariate analysis | Multivariate analysis | |||
---|---|---|---|---|---|
OR (95% CI) | P value | OR (95% CI) | P value | ||
Age | 1.001 (0.997–1.006) | 0.65 | |||
Tumor dimension | 0.986 (0.982–0.989) | <0.001* | 0.989 (0.985–0.992) | <0.001* | |
Sex | 1.149 (1.004–1.314) | 0.09 | |||
Echogenicity | 0.834 (0.790–0.880) | <0.001* | 0.886 (0.839–0.934) | <0.001* | |
Echotexture | 0.774 (0.661–0.906) | 0.008* | 0.882 (0.765–1.018) | 0.15 |
*, P<0.05. PTMC, papillary thyroid microcarcinoma; OR, odds ratios; CI, confidence interval.
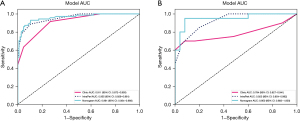
Based on clinical and radiomics model, we successfully constructed a nomogram combined intratumoral and peritumoral radiomics features as well as clinical features (Figure 7). The utilization of intratumoral and peritumoral radiomics features significantly improved the predictive ability for PTMC in ACR TI-RADS 3 nodules based on the significant clinical characteristics. In the training cohort, the radiomics-clinical nomogram model yielded an AUC of 0.961 (95% CI: 0.934–0.988), which were higher than clinical factor (0.961 vs. 0.911) and radiomics signature models (0.961 vs. 0.955). In the test cohort, the AUC values of the nomogram model (0.950, 95% CI: 0.883–1.000) were higher than that of clinical factor (0.950 vs. 0.784) and radiomics signature models (0.950 vs. 0.923) (Figure 6, Table 2). The sensitivity and specificity of the clinical model, intratumor-peritumor combined-region model, and nomogram in the training and test cohorts were demonstrated in the Table 2, which showed the radiomics-clinical nomogram had favorable discrimination efficiency.
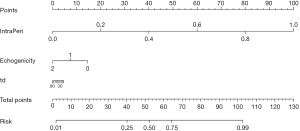
Figure 8 displays calibration curves for the clinical model, combined-region model, and nomogram. Results showed good agreement between the prediction curve of the radiomics-clinical nomogram and standard curve. Thus, the nomogram demonstrated an excellent performance for identifying PTMC in ACR TI-RADS 3 nodules. In a comparison against scenarios without prediction model implementation (i.e., treat-all or treat-none scheme), the nomogram provided greater benefit in most cases (Figure 8).
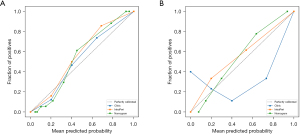
DeLong’s test was used for the comparisons between the AUCs of the models. Notably, there were significant differences in the value of AUC between the combined-region model and both intratumor-region model (P<0.05) and peritumor-region model (P<0.05) in the test cohort. Overall, compared with other radiological models, the performance of the combined-region model was better. As depicted in Figure 6, the nomogram demonstrated the best predictive ability, surpassing the standalone radiomics and clinical models. The calibration curve also showed favorable prediction efficiency of the proposed combined nomogram.
Discussion
In this study, a predictive nomogram consisting of intratumoral and peritumoral radiomics features as well as clinical features was developed and validated for the individualized prediction of PTMC in patients with ACR TI-RADS 3 nodules.
Although most PTMCs have an indolent course, the literature has reported some cases of PTMC with locoregional recurrences and distant metastases (20). Therefore, early and accurate detection of PTMC can help to promote optimal treatment and relieve the burden of patients. US still plays an important role in the diagnosis of thyroid-related diseases. However, the method has limitations associated with small nodules, resulting the accuracy of diagnosing PTMC being inefficient. Zhang et al. (21) found that US in identifying PTMC has a low sensitivity and specificity, implicating that a proportion of patients would be mistreated or misdiagnosed. Although FNAB demonstrates an effective method for diagnosing thyroid nodules ≤1 cm with a low nondiagnostic rate, it is still a costly and invasive method (22). Thus, it is crucial to find a nondestructive and effective method to make a reliable and precise diagnosis early.
Radiomics is an accurate, objective and highly efficient method to enhance conventional imaging diagnosis. It extracts a multitude of quantitative, high-dimensional features from the image, and carries on self-training and learning based on the pathological results. Several published studies have delved into the application of radiomics in predicting the malignancy of thyroid micronodules. For example, Zhang et al. (19) constructed a multimodal US radiomics nomogram, showing favorable predictive efficiency with an AUC of 0.881. Wu et al. (23) explored the potential ability of CT-based radiomics in accurate classification of thyroid micronodules, and their model achieved an AUC value of 0.851. Previous radiomics studies in thyroid nodules mainly focused on the intratumoral region alone. Nonetheless, there is growing evidence that the predictive model should not be limited to mere tumor regions, and that the surrounding areas may provide complementary information about tumor heterogeneity in other cancers. So, in this study, we developed US-based radiomics models to identify PTMC in ACR TI-RADS 3 nodules using intratumoral and peritumoral radiomics features, separately or in combination, and validated their performances.
In our study, there were 23 radiomics features which were significantly correlated with the malignancy of PTMC, including one shape feature, five first-order features, five gray-level co-occurrence matrix (GLCM) textural features, four gray-level run-length matrix (GLRLM) textural features, five gray-level size zone matrix (GLSZM) textural features and three neighbouring gray tone difference matrix (NGTDM) textural features. The textural radiomics features accounted for the greatest weight. It has been shown that the textural features measure tumor heterogeneity and further reflect cancer pathophysiology, which was also confirmed in our study (24). Based on these features, we constructed three radiomics models: intratumor-region model, peritumor-region model and the combined-region model. All three showed favorable discriminative performance. This further confirms that radiomics analysis is an effective tool for calculating the spatial heterogeneity of tumors. The combined-region model showed an excellent result, with higher AUC value (AUC =0.923) in the independent test cohort compared to that of the intratumor-region model and the peritumor-region model (0.851 and 0.798, respectively). This suggests that the surrounding areas is able to provide complementary information about tumor heterogeneity, and that the combined-region model will yield more accurate predicting results than the individual models.
In this study, we also considered clinical characteristics, such as echogenicity and tumor dimension. However, the inclusion of only clinical characteristic is inadequate for achieving a high diagnostic accuracy rate. The clinical model had a subpar predictive performance in the identification of PTMC (AUC =0.784). But we found that the addition of radiomics had a strong and independent effect on prediction accuracy. By combining intratumoral and peritumoral radiomics features as well as clinical features, we constructed a nomogram that yielded a significant improvement in diagnostic performance, raising the AUC from 0.784 to 0.950. As compared to the methods of Zhang et al. (19) and Wu et al. (23), our method showed better performance. The calibration curve also indicated the clinical applicability of the proposed combined nomogram. Overall, the developed radiomics-clinical nomogram contributed to improve the identification of PTMC from ACR TI-RADS 3 nodules.
There are limitations in our study. Firstly, there is a lack of external verification, because the sample size was insufficient and it was developed and validated in the same center. Thus, a multicenter survey using a larger sample size is necessary to further validate the nomogram predictive model. Secondly, since this study is retrospective, selection bias is inevitable, which affected the results of our study. In the future, we aim to carry out prospective studies to control for confounding variables. Thirdly, the lesion’s contour was delineated manually, which was labor-intensive and time-consuming. Further research on automatic volume segmentation is needed to simplify this process. Fourthly, US images were captured utilizing diverse US machines, necessitating a standardization process to ensure consistency across the dataset. Fifthly, the utilization of various versions of the TI-RADS by different centers could potentially introduce discrepancies in the classification of thyroid nodules, thereby affecting the accuracy and reliability of diagnostic outcomes.
Conclusions
As a noninvasive prediction tool, the US-based nomogram consisting of intratumoral and peritumoral radiomics features as well as clinical information has high diagnostic performance in the early discrimination of PTMC in ACR TI-RADS 3 nodules.
Acknowledgments
Funding: This work was supported by
Footnote
Reporting Checklist: The authors have completed the TRIPOD reporting checklist. Available at https://gs.amegroups.com/article/view/10.21037/gs-24-30/rc
Data Sharing Statement: Available at https://gs.amegroups.com/article/view/10.21037/gs-24-30/dss
Peer Review File: Available at https://gs.amegroups.com/article/view/10.21037/gs-24-30/prf
Conflicts of Interest: All authors have completed the ICMJE uniform disclosure form (available at https://gs.amegroups.com/article/view/10.21037/gs-24-30/coif). The authors have no conflicts of interest to declare.
Ethical Statement: The authors are accountable for all aspects of the work in ensuring that questions related to the accuracy or integrity of any part of the work are appropriately investigated and resolved. The study was conducted in accordance with the Declaration of Helsinki (as revised in 2013). The study was approved by the institutional ethics committee of The Second Affiliated Hospital of Wenzhou Medical University (No. 2023-K-43-01) and individual consent for this retrospective analysis was waived.
Open Access Statement: This is an Open Access article distributed in accordance with the Creative Commons Attribution-NonCommercial-NoDerivs 4.0 International License (CC BY-NC-ND 4.0), which permits the non-commercial replication and distribution of the article with the strict proviso that no changes or edits are made and the original work is properly cited (including links to both the formal publication through the relevant DOI and the license). See: https://creativecommons.org/licenses/by-nc-nd/4.0/.
References
- Won HR, Koo BS. Active Surveillance or Surgery in Papillary Thyroid Microcarcinoma: An Ongoing Controversy. Clin Exp Otorhinolaryngol 2022;15:123-4. [Crossref] [PubMed]
- Kitahara CM, Sosa JA. The changing incidence of thyroid cancer. Nat Rev Endocrinol 2016;12:646-53. [Crossref] [PubMed]
- Davies L, Morris LG, Haymart M, et al. American Association of Clinical Endocrinologists and American College of Endocrinology Disease State Clinical Review: The Increasing Incidence of Thyroid Cancer. Endocr Pract 2015;21:686-96. [Crossref] [PubMed]
- Zheng X, Wei S, Han Y, et al. Papillary microcarcinoma of the thyroid: clinical characteristics and BRAF(V600E) mutational status of 977 cases. Ann Surg Oncol 2013;20:2266-73. [Crossref] [PubMed]
- Davies L, Welch HG. Current thyroid cancer trends in the United States. JAMA Otolaryngol Head Neck Surg 2014;140:317-22. [Crossref] [PubMed]
- Sugitani I. Active surveillance of low-risk papillary thyroid microcarcinoma. Best Pract Res Clin Endocrinol Metab 2023;37:101630. [Crossref] [PubMed]
- Li Q, Feng T, Zhu T, et al. Multi-omics profiling of papillary thyroid microcarcinoma reveals different somatic mutations and a unique transcriptomic signature. J Transl Med 2023;21:206. [Crossref] [PubMed]
- Kim KJ, Kim SG, Tan J, et al. BRAF V600E status may facilitate decision-making on active surveillance of low-risk papillary thyroid microcarcinoma. Eur J Cancer 2020;124:161-9. [Crossref] [PubMed]
- Lim H, Devesa SS, Sosa JA, et al. Trends in Thyroid Cancer Incidence and Mortality in the United States, 1974-2013. JAMA 2017;317:1338-48. [Crossref] [PubMed]
- Yang F, Zhong Q, Huang Z, et al. Survival in Papillary Thyroid Microcarcinoma: A Comparative Analysis Between the 7th and 8th Versions of the AJCC/UICC Staging System Based on the SEER Database. Front Endocrinol (Lausanne) 2019;10:10. [Crossref] [PubMed]
- Huang H, Xu S, Wang X, et al. Patient Age Is Significantly Related to Distant Metastasis of Papillary Thyroid Microcarcinoma. Front Endocrinol (Lausanne) 2021;12:748238. [Crossref] [PubMed]
- Alexander EK, Cibas ES. Diagnosis of thyroid nodules. Lancet Diabetes Endocrinol 2022;10:533-9. [Crossref] [PubMed]
- Tessler FN, Middleton WD, Grant EG, et al. ACR Thyroid Imaging, Reporting and Data System (TI-RADS): White Paper of the ACR TI-RADS Committee. J Am Coll Radiol 2017;14:587-95. [Crossref] [PubMed]
- Slijepcevic N, Zivaljevic V, Marinkovic J, et al. Retrospective evaluation of the incidental finding of 403 papillary thyroid microcarcinomas in 2466 patients undergoing thyroid surgery for presumed benign thyroid disease. BMC Cancer 2015;15:330. [Crossref] [PubMed]
- Baloch ZW. LiVolsi VA. Current role and value of fine-needle aspiration in nodular goitre. Best Pract Res Clin Endocrinol Metab 2014;28:531-44. [Crossref] [PubMed]
- Soyer Güldoğan E, Ergun O, Taşkın Türkmenoğlu T, et al. The impact of TI-RADS in detecting thyroid malignancies: a prospective study. Radiol Med 2021;126:1335-44. [Crossref] [PubMed]
- Li G, Li L, Li Y, et al. An MRI radiomics approach to predict survival and tumour-infiltrating macrophages in gliomas. Brain 2022;145:1151-61. [Crossref] [PubMed]
- Liu Z, Wang S, Dong D, et al. The Applications of Radiomics in Precision Diagnosis and Treatment of Oncology: Opportunities and Challenges. Theranostics 2019;9:1303-22. [Crossref] [PubMed]
- Zhang XY, Zhang D, Han LZ, et al. Predicting Malignancy of Thyroid Micronodules: Radiomics Analysis Based on Two Types of Ultrasound Elastography Images. Acad Radiol 2023;30:2156-68. [Crossref] [PubMed]
- Ardito G, Revelli L, Giustozzi E, et al. Aggressive papillary thyroid microcarcinoma: prognostic factors and therapeutic strategy. Clin Nucl Med 2013;38:25-8. [Crossref] [PubMed]
- Zhang Y, Pan J, Xu D, et al. Combination of serum microRNAs and ultrasound profile as predictive biomarkers of diagnosis and prognosis for papillary thyroid microcarcinoma. Oncol Rep 2018;40:3611-24. [Crossref] [PubMed]
- Gao L, Ma B, Zhou L, et al. The impact of presence of Hashimoto's thyroiditis on diagnostic accuracy of ultrasound-guided fine-needle aspiration biopsy in subcentimeter thyroid nodules: A retrospective study from FUSCC. Cancer Med 2017;6:1014-22. [Crossref] [PubMed]
- Wu X, Li J, Mou Y, et al. Radiomics Nomogram for Identifying Sub-1 cm Benign and Malignant Thyroid Lesions. Front Oncol 2021;11:580886. [Crossref] [PubMed]
- Rizzo S, Botta F, Raimondi S, et al. Radiomics: the facts and the challenges of image analysis. Eur Radiol Exp 2018;2:36. [Crossref] [PubMed]