Nomogram for predicting the risk of central lymph node metastasis in papillary thyroid microcarcinoma: a combination of sonographic findings and clinical factors
Highlight box
Key findings
• We developed and validated a predictive model for central lymph node metastasis (CLNM) in papillary thyroid microcarcinoma (PTMC).
• Risk stratification using nomogram informs clinicians of tailor-made strategy for PTMC.
What is known and what is new?
• Risk factors for lymph node metastasis of thyroid cancer include age, tumor size, gender, multifocality, and thyroid peroxidase antibodies (TPOAbs).
• We developed a predictive model for CLNM in PTMC consisting of free triiodothyronine, free thyroxine, and TPOAbs, with which we risk-stratified the affected population for clinicians to formulate personalized treatment plans.
What is the implication, and what should change now?
• This nomogram for predicting CLNM in patients with PTMC can provide quantitative information and reduce the burden of medical care.
Introduction
Papillary thyroid cancer (PTC) has been rapidly increasing and become the 11th most prevalent malignancy worldwide (1-8). A study using the Global Cancer Observatory (GLOBOCAN) database showed that the incidence rate of thyroid cancer in developed regions was higher than those in developing and underdeveloped legions; however, there was no significant difference in mortality because of high proportion of papillary thyroid microcarcinoma (PTMC) detected by fine needle aspiration with high resolution ultrasonography (US) (9). Furthermore, autopsies revealed that some patients who died from non-thyroid cancer already had PTMC in their lifetime (10). Therefore, PTMC is characterized by its indolent nature and have a tendency of slow growing. However, metastatic neck lymph node metastasis (LNM) was found in 24–64% of patients with PTMC (11). The earlier a surgery is performed, the lower the risk is; furthermore, for many patients who live in remote areas with limited transportation, it is simpler for them to go straight to surgery as opposed to active monitoring. Notably, as patients age, the incidence of postsurgical complications tends to increase. The issue of overdiagnosis centers primarily on whether PTMC cases are low-risk and whether central LNM (CLNM) is among the important criteria for diagnosis (11-13). To clarify, if the risk of CLNM is high, thyroidectomy and preventive central lymph node dissection (PCLND) are recommended. Conversely, if the risk of CLNM is low, active monitoring of disease progression or thyroidectomy without PCLND is recommended to significantly improve the patient’s prognosis and to reduce the surgery-related physiological and psychological complications. Given these circumstances, establishing a model that can accurately predict CLNM in patients with PTMC before surgery is crucial.
US is the primary method for evaluating the thyroid nodule and cervical lymph node as well (14-17). Despite the advantage of easy-accessible, cost effective, radiation-free, and non-invasive modality, US is operator dependent. Furthermore, detecting suspicious CLN might be an obstacle compared to lateral lymph node because of the anatomical correlation such as trachea, esophagus, and clavicle. Numerous studies on predictive models for CLNM in thyroid cancer have focused on PTC rather than PTMC, which has been less targeted and cannot adequately address problems (18-30). Prediction of CLNM is crucial process for treatment strategy in PTMC. Hence, we developed the nomogram combining the US findings and clinical factors for predicting CLND in patients with PTMC. We present this article in accordance with the TRIPOD reporting checklist (available at https://gs.amegroups.com/article/view/10.21037/gs-24-154/rc).
Methods
Patients
Data were collected from patients with pathologically confirmed PTMC who had undergone thyroidectomy and CLND at Tangdu Hospital and Xijing Hospital from January 1, 2017 to December 31, 2022. The exclusion criteria for patients were as follows: (I) concurrent and relapsed tumors, (II) distant metastasis, (III) ongoing administration of neoadjuvant or translational therapy, and (IV) incomplete US and clinicopathological data. Seven hundred and nine patients from Tangdu Hospital were included in the training set, whereas 320 patients from Xijing Hospital were included in the validation set. Clinicopathological data were obtained from the institutions’ medical databases and the US results were obtained from the medical imaging databases (Picture Archive and Communication Systems). The study was conducted in accordance with the Declaration of Helsinki (as revised in 2013). This study met the requirements of the Xijing Hospital Ethics Committee and Tangdu Hospital Ethics Committee for the waiver of ethical approval, and informed consent was taken from all the patients.
US examination and image analysis
All patients underwent examination with a US machine (Hitachi, Ltd., Tokyo, Japan) with a linear transducer operating in the 6- to 13-MHz frequency range. Each patient was placed in the supine position with the head tilted back and the neck completely exposed. Prior to the examination, we provided uniform training to the sonographers of both centers. During the examination, we sampled one-tenth of the patients from each center, performed the examination independently at the workstation of the other center, and compared the results to try to eliminate errors due to the operation of the sonographers. Two sonographers with over 5 years of thyroid US experience independently evaluated the patients’ US imaging characteristics. Both sonographers were blinded to the patient characteristics and findings. Any discrepancies between sonographers were resolved by a third sonographer with over 10 years of experience in the thyroid US, and a final report was generated. All US images and reports were stored in a hospital database. The imaging characteristics of each nodule were as follows: tumor shape was classified as regular or irregular; internal echo pattern was divided into heterogeneous or homogeneous; tumor margin was classified as smooth or ill-defined; multifocality was considered in cases where one or both lobes exhibited two or more foci; tumor size refers to the diameter of the largest tumor; color Doppler flow imaging (CDFI) in the article indicated peripheral or (and) internal blood flow signals, it was classified from 0 to 3; aspect ratio (height divided by width on transverse views, A/T); microcalcifications were defined as having a maximum diameter of less than 2 mm and were classified as present or absent, low-risk nodules’ means nodules classified as “1”, “2” and “3” according to Chinese Thyroid Imaging Reporting And Data System (C-TIRADS) (31); loss of fatty hilum was classified as yes or no.
Sample collection and laboratory evaluations
Blood samples were collected from patients between 6:30 AM and 8:30 AM on the day following admission and after an instructed 8-hour fasting period. Subsequently, the serum was immediately separated and preserved at −80 °C until examination. Triiodothyronine (T3), thyroxine (T4), free T3 (FT3), free T4 (FT4), thyroid-stimulating hormone (TSH), TPOAb, and thyroglobulin antibody (TgAb) were determined via electrochemical luminescent immunoassays using cobas® e 411 analyzers and their accompanying reagents and calibrators (Roche Diagnostics, Rotkreuz, Switzerland). These analyzers are functionally sensitive at levels <15 µIU/mL. All procedures were performed by strictly adhering to the manufacturers’ instructions.
Surgical treatment
The surgical extent of thyroid gland was determined by the number and location of tumor. Thyroid lobectomy was performed if the tumor(s) were in one lobe irrespective of numbers; while total thyroidectomy was performed if more than two tumors were in both lobes or tumor was infiltrated beyond the thyroid gland. The extent of CLND depended on the tumor location. Ipsilateral CLND was performed if the tumor(s) were limited in the one lobe, whereas bilateral CLND was performed if the tumors were presented in both lobes.
Statistical analysis and nomogram validation
Patients were classified as CLNM positive or CLNM negative according to the CLNM status in the pathology report. The categorical variables present count and percentage; while the continuous variables express median and interquartile ranges. Wilcoxon signed rank test for continuous variables and the Fisher exact test for categorical variables were used for comparison. Candidate variables identified with P<0.2 by univariate logistic regression analysis in the training set were selected for inclusion in the multiple regression model. To create the most efficient and compact model, we adopted backward stepwise selection with the Akaike information criterion (AIC) as the termination criterion. To accurately quantify the risk of CLNM in each patient with PTMC, a nomogram was developed in the training set via multivariate logistic regression analysis using the “rms” package in R version 4.1.1 (The Foundation for Statistical Computing; http://www.r-project.org). The overfitting bias was reduced by drawing a calibration curve with 1,000 bootstrap samples and calculating the concordance index (C-index). The predictive performance was measured through internal and external validations. We assessed the accuracy of the nomogram using likelihood ratios with specificity, sensitivity, and 95% confidence intervals (CIs) of the receiver operating characteristic (ROC) curve. Decision curve analysis (DCA) was used to examine the net benefits of the nomogram at different thresholds. Stata (RRID: SCR_012763; Stata Corp, College Station, TX, USA) and R software were used for data processing. Statistical significance was set at a two-sided P value of <0.05.
Results
Clinicopathologic characteristics
Of the 1,397 patients preoperatively diagnosed with PTMC, 1,029 met the inclusion criteria (Figure 1). The training set included 709 patients (318 CLNM positive and 391 CLNM negative) from Tangdu Hospital, while the validation set included 320 patients (147 CLNM positive and 173 CLNM negative) from Xijing Hospital. The sample size of this study met the criteria for each predictor variable of the 10 outcome events. Table 1 showed the baseline characteristics in the training and validation sets. There were no significant differences in age, body mass index, tumor size, FT3, FT4, T3, T4, TSH, TPOAb, TgAb, or US variables between the training and validation sets.
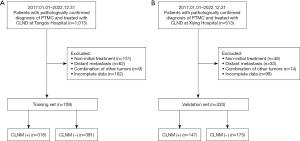
Table 1
Characteristics | Training data (n=709) | Validation data (n=320) | P value |
---|---|---|---|
Age (years) | 46.0 (36.0, 53.0) | 47.0 (36.5, 54.0) | 0.47 |
BMI (kg/m2) | 23.6 (21.7, 26.0) | 23.6 (21.2, 26.0) | 0.51 |
Tumor size (cm) | 0.7 (0.6, 0.9) | 0.7 (0.6, 0.9) | 0.82 |
FT3 (pmol/L) | 4.7 (4.3, 5.1) | 4.6 (4.4, 5.1) | 0.90 |
FT4 (pmol/L) | 16.9 (15.0, 18.3) | 16.7 (15.1, 18.4) | 0.44 |
T3 (pmol/L) | 1.7 (1.5, 1.9) | 1.7 (1.5, 1.9) | 0.59 |
T4 (pmol/L) | 101.1 (90.1, 113.0) | 100.0 (48.0, 155.0) | 0.44 |
TSH (μIU/mL) | 2.2 (1.6, 3.2) | 2.1 (1.6, 3.4) | 0.44 |
TgAb (U/mL) | 97.5 (2.0, 224.5) | 13.2 (10.0, 99.8) | 0.03 |
TPOAb (U/mL) | 12.8 (9.5, 20.4) | 12.8 (9.2, 21.5) | 0.55 |
Gender | 0.64 | ||
Female | 178 (25.1) | 76 (23.8) | |
Male | 531 (74.9) | 244 (76.3) | |
C-TIRADS | 0.89 | ||
4A | 217 (30.6) | 100 (31.3) | |
4B | 297 (41.9) | 128 (40.0) | |
4C | 124 (17.5) | 58 (18.1) | |
5 | 39 (5.5) | 17 (5.3) | |
6 | 32 (4.5) | 17 (5.3) | |
Multifocality | 0.42 | ||
No | 533 (75.2) | 233 (72.8) | |
Yes | 176 (24.8) | 87 (27.2) | |
Tumor shape | 0.43 | ||
Regular | 312 (44.0) | 144 (45.0) | |
Irregular | 397 (56.0) | 176 (55.0) | |
Tumor margin | 0.15 | ||
Smooth | 266 (37.5) | 105 (32.8) | |
Ill-defined | 443 (62.5) | 215 (67.2) | |
Internal echo | 0.77 | ||
Homogeneous | 159 (22.4) | 79 (24.7) | |
Heterogeneous | 550 (77.6) | 241 (75.3) | |
Microcalcification | 0.16 | ||
Present | 249 (35.1) | 127 (39.7) | |
Absent | 460 (64.9) | 193 (60.3) | |
CDFI blood flow | 0.97 | ||
0–1 | 108 (15.2) | 49 (15.3) | |
2–3 | 601 (84.8) | 271 (84.7) | |
Aspect ratio | 0.75 | ||
<1 | 382 (53.9) | 169 (52.8) | |
≥1 | 327 (46.1) | 151 (47.2) | |
Number of low-risk nodules | 0.98 | ||
0 | 353 (49.8) | 155 (48.4) | |
1 | 172 (24.3) | 89 (27.8) | |
≥2 | 184 (26.0) | 76 (23.8) | |
Number of enlarged lymph nodes | 0.71 | ||
0 | 317 (44.7) | 139 (43.4) | |
1 | 131 (18.5) | 60 (18.8) | |
≥2 | 261 (36.8) | 121 (37.8) | |
Loss of fatty hilum | 0.78 | ||
Yes | 655 (92.4) | 294 (91.9) | |
No | 54 (7.6) | 26 (8.1) |
Data are presented as median (IQR) or n (%). Number of low-risk nodules: nodules defined on ultrasound as having a low likelihood of malignancy. US, ultrasonography; BMI, body mass index; FT3, free triiodothyronine; FT4, free thyroxine; T3, triiodothyronine; T4, thyroxine; TSH, thyroid-stimulating hormone; TgAb, thyroglobulin antibody; TPOAb, thyroid peroxidase antibody; C-TIRADS, Chinese Thyroid Imaging Reporting and Data System; CDFI, color Doppler flow imaging; IQR, interquartile range.
The positivity rates for CLNM in the training and validation sets were 44.85% and 45.94%, respectively. The difference in the incidence of CLNM between the sets was not statistically significant (P=0.80). However, sex, tumor shape, age, multifocality, tumor size, loss of fatty hilum, microcalcification, aspect ratio, FT3, FT4, and TPOAb were significantly different between patients with and without CLNM (Table 2).
Table 2
Variables | OR (95% CI) | P value |
---|---|---|
Age | 0.97 (0.96–0.98) | <0.01* |
BMI | 0.97 (0.93–1.02) | 0.23 |
Tumor size | 7.03 (3.09–16.02) | <0.01* |
FT3 | 1.54 (1.20–1.97) | 0.01* |
FT4 | 1.10 (1.04–1.16) | 0.01* |
T3 | 0.97 (0.86–1.09) | 0.58 |
T4 | 1.01 (1.01–1.02) | 0.97 |
TSH | 0.92 (0.84–1.02) | 0.10* |
TgAb | 1.00 (1.00–1.00) | 0.39 |
TPOAb | 1.0 (1.0–1.0) | 0.04* |
Gender (male vs. female) | 1.68 (1.20–2.36) | <0.01* |
C-TIRADS | ||
4B vs. 4A | 0.73 (0.34–1.55) | 0.41 |
4C vs. 4A | 1.34 (0.64–2.81) | 0.44 |
5 vs. 4A | 2.24 (1.01–4.94) | 0.05* |
6 vs. 4A | 1.02 (0.39–2.63) | 0.97 |
Multifocality (multiple vs. single) | 0.61 (0.44–0.86) | <0.01* |
Tumor shape (irregular vs. regular) | 1.38 (1.02–1.87) | 0.03* |
Margin (smooth vs. ill-defined) | 0.86 (0.63–1.12) | 0.33 |
Internal echo (heterogeneous vs. homogeneous) | 0.73 (0.51–1.05) | 0.09* |
Microcalcification (present vs. absent) | 0.49 (0.36–0.68) | <0.01* |
CDFI blood flow (2–3 vs. 0–1) | 1.07 (0.71–1.62) | 0.74 |
Aspect ratio (<1 vs. ≥1) | 0.19 (0.14–0.27) | <0.01* |
Number of low-risk nodules | ||
1 vs. 0 | 1.26 (0.88–1.81) | 0.21 |
≥2 vs. 0 | 1.13 (0.74–1.71) | 0.58 |
Number of enlarged lymph nodes | ||
1 vs. 0 | 0.99 (0.71–1.37) | 0.94 |
≥2 vs. 0 | 1.57 (1.03–2.40) | 0.04* |
Loss of fatty hilum (no vs. yes) | 0.29 (0.15–0.53) | <0.01* |
These factors were selected as candidate variables for establishing the nomogram. *, P<0.2. OR, odds ratio; CI, confidence interval; BMI, body mass index; FT3, free triiodothyronine; FT4, free thyroxine; T3, triiodothyronine; T4, thyroxine; TSH, thyroid-stimulating hormone; TgAb, thyroglobulin antibody; TPOAb, thyroid peroxidase antibody; C-TIRADS, Chinese Thyroid Imaging Reporting and Data System; CDFI, color Doppler flow imaging.
Nomogram development
Candidate indicators selection via logistic regression analysis
Univariate logistic regression analysis showed that the P values of sex (P<0.01), age (P<0.01), multifocality (P<0.01), internal echo (P=0.09), tumor shape (P=0.03), microcalcification (P<0.01), aspect ratio (P<0.01), tumor size (P<0.01), FT3 (P=0.01), FT4 (P=0.01), TSH (P=0.14), and TPOAb (P=0.04) were less than 0.2 of CLNM (Table 2). These variables were incorporated into the multivariate regression model as candidate predictors of CLNM risk.
Stepwise backward selection and multivariate logistic analysis for nomogram construction
Multivariate regression analysis presented that odds ratio (OR) of age (OR =0.968; 95% CI: 0.953–0.984), FT4 (OR =1.101; 95% CI: 1.039–1.166), tumor size (OR =3.570; 95% CI: 1.361–9.363), multifocality (OR =1.755; 95% CI: 1.188–2.593), TPOAb (OR =0.998; 95% CI: 0.997–1.000), microcalcification (OR =1.701; 95% CI: 1.166–2.482), loss of fatty hilum (OR =2.769; 95% CI: 1.385–5.536), and aspect ratio (OR =5.334; 95% CI: 3.783–7.520) were identified as independent predictors for CLNM risk (Table 3). These factors were used to establish a CLNM risk evaluation nomogram (Figure 2).
Table 3
Variables | β† | OR (95% CI) | P value |
---|---|---|---|
Age | −0.032 | 0.968 (0.953–0.984) | <0.001 |
Multifocality‡ | 0.563 | 1.755 (1.188–2.593) | 0.005 |
Tumor size‡ | 1.273 | 3.570 (1.361–9.363) | 0.01 |
Microcalcification‡ (present vs. absent) | 0.531 | 1.701 (1.166–2.482) | 0.006 |
Aspect ratio‡ (≥1 vs. <1) | 1.674 | 5.334 (3.783–7.520) | <0.001 |
Loss of fatty hilum‡ (yes vs. no) | 1.019 | 2.769 (1.385–5.536) | 0.004 |
FT4 | 0.096 | 1.101 (1.039–1.166) | 0.001 |
TPOAb | −0.002 | 0.998 (0.997–1.000) | 0.03 |
Constant | −3.104 | 0.45 | <0.001 |
†, unstandardized β coefficients were calculated using multivariate logistic regression analysis based on stepwise regression (Akaike information criterion: 807.96); ‡, variables based on ultrasonography results. OR, odds ratio; CI, confidence interval; FT4, free thyroxine; TPOAb, thyroid peroxidase antibody.
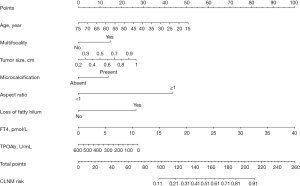
Validation of the nomogram
Calibration of the nomogram
Bootstrapping was used to conduct the internal and external validations of the nomogram. The nomogram showed excellent accuracy in estimating CLNM risk, with a C-index of 0.784 (95% CI: 0.750–0.817) in the training set. Furthermore, the calibration curve showed good consistency between the risk estimates and tissue pathology results of the surgical specimens. In the validation set, the C-index of the nomogram for estimating CLNM risk was 0.779 (95% CI: 0.729–0.830) (Figure 3).
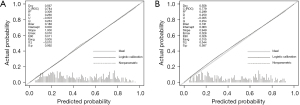
Accuracy assessment of the nomogram
The internal and external validation results for the ROC curve are 0.784 and 0.779 respectively (Figure 4). When the cutoff score was set to 0.438, we obtained the maximum Youden index. Therefore, a value of 0.438 was used for further analysis. With 0.438 as the cutoff score, the training set demonstrated a 73.9% sensitivity, a 73.5% specificity, a 71.3% accuracy, a 27.7% positive predictive value, and a 77.5% negative predictive value. In the validation set, these metrics were 75.8%, 70.0%, 70.3%, 37.0%, and 68.7%, respectively (Table 4). Our model can provide more benefit to patients with risk thresholds between 12% and 89% in the training group and between 12% and 85% in the validation group, without taking into account any risk factors, than with an “all treatment” or “no treatment” approach (Figure 5).
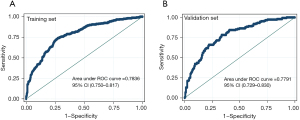
Table 4
Variables | Training set | Validation set |
---|---|---|
AUC/C-index (95% CI) | 0.784 (0.750, 0.817) | 0.779 (0.729, 0.830) |
Sensitivity (95% CI) | 0.739 (0.738, 0.739) | 0.758 (0.756, 0.760) |
Specificity (95% CI) | 0.735 (0.735, 0.735) | 0.700 (0.699, 0.702) |
Cutoff score | 0.438 | 0.438 |
Accuracy (95% CI) | 0.713 (0.713, 0.714) | 0.703 (0.702, 0.703) |
Positive predictive value (95% CI) | 0.277 (0.277, 0.278) | 0.370 (0.369, 0.371) |
Negative predictive value (95% CI) | 0.775 (0.775, 0.775) | 0.687 (0.687, 0.688) |
CLNM, central lymph node metastasis; AUC, area under the receiver operating characteristic curve; C-index, concordance index; CI, confidence interval.
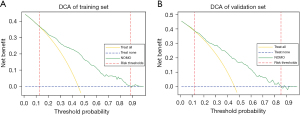
Risk stratification of CLNM based on the nomogram
As shown in Table 5, to more conveniently apply this nomogram to clinical practice, we determined two cutoff values through recursive partitioning based on the risk scores of all patients, thereby categorizing the risk of CLNM into three cohorts: (I) low risk (score <110), moderate risk (110≤ score ≤180), and high risk (score >180). In the training dataset, CLNM rates for the low-, moderate-, and high-risk cohorts were 11.8%, 41.4%, and 82.2%, respectively (P<0.001). Meanwhile, in the validation dataset, these rates were 11.7%, 43.2%, and 79.2%, respectively (P<0.001). Furthermore, pairwise comparisons indicated significant differences in the risk among these groups (P<0.001).
Table 5
Nomogram | LR (<110) | MR (110–180) | HR (>180) | Total | P value (total) |
---|---|---|---|---|---|
Training set | <0.001 | ||||
With CLNM | 15 (11.8) | 178 (41.4) | 125 (82.2) | 318 | |
Without CLNM | 112 (88.2) | 252 (58.6) | 27 (17.8) | 391 | |
Total | 127 | 430 | 152 | 709 | |
Validation set | <0.001 | ||||
With CLNM | 7 (11.7) | 79 (43.2) | 61 (79.2) | 147 | |
Without CLNM | 53 (88.3) | 104 (56.8) | 16 (20.8) | 173 | |
Total | 60 | 183 | 77 | 320 |
Data are presented as n or n (%). CLNM, central lymph node metastasis; LR, low risk; MR, moderate risk; HR, high risk.
Discussion
Prediction of CLNM could be a challenge and might have impact on the management strategy in patients with PTMC. The present study developed the predictive model for CLNM as a highly accurate, distinguishing, simple, and convenient tool for clinical applications. Previous models for predicting the risk of CLNM in PTMC have ignored biochemical indicators (32,33), and most are based on postoperative histopathological findings and genetic screening. Notably, they did not perform external validation, which may affect the accuracy of models and limit their preoperative application in treatment decisions. Our research was based on demographic characteristics, US findings, serum indicators of thyroid function, and serological indicators of autoimmune thyroid systems. Our study found that young age, larger tumor size, high FT3 and FT4 levels, low TPOAb levels, male sex, multifocality, microcalcification, an aspect ratio ≥1, and loss of fatty hilum were associated with increased CLNM in PTMC. Subsequently, using stepwise backward selection, we adopted AIC as the termination criterion to generate a nomogram with good accuracy and calibration. Additionally, this predictive model is a simple, easy-accessible and real-time manageable method. Our nomogram could provide the quantification for predicting CLNM in patients with PTMC to clinicians and patients.
Our findings corroborate previous studies, both indicating that young age is an important risk factor for the development of CLNM in PTMC (34,35). After reviewing 2,930 cases, Lee et al. (36) suggested that sex is not a prognosticator of PTMC but is an independent predictor of PTC prognosis. Although our study demonstrated the opposite results, the findings confirmed that sex is an independent predictor of CLNM in PTMC.
US imaging is a powerful and reliable method for diagnosing PTMC and determining the risk of CLNM. Several US imaging findings were significantly associated with CLNM and were incorporated into our nomogram. There is a consensus indicating that maximal tumor size based on US imaging is an essential predictor of CLNM (37-40). In a study by Weng et al. (41), the proportion of jumping LNM in PTMC cases with a diameter greater than 0.5 was 2.26 times higher than those with a diameter less than 0.5 cm, which implies that PTMC with a large diameter have a potency of more invasiveness. Microcalcification showed white opaque specks scattered or partially concentrated on the US image. Characteristics of microcalcification of thyroid nodule on US image is mainly used to determine whether the nodule has a potential malignancy. Microcalcification is a critical characteristic of PTCs. It may be related to rapid tumor growth, active metabolism, or coagulation necrosis in some tissues. Previous studies have found a correlation between US-detected microcalcification and LNM (42-44). In our model, the OR for microcalcification was 1.7, indicating that patients with calcified lesions are 1.7 times more susceptible to developing CLNM than are those without calcified lesions under similar conditions, which has not only been reported in previous thyroid cancers (29,45) but also in breast cancer (46,47).
Similar to our study, previous meta-analytic research described that multifocality was associated with CLNM (28,29,45,48,49). It is unclear whether multifocality is indicative of multiple newly developed tumors or lesions originating from a single thyroid tumor (50). There is evidence that multifocal lesions represent different tumors, as they often have different RET/PTC gene rearrangements and independent cloning sources. However, a study based on genome-wide allele genotypes have shown that over 80% of multifocal PTMC tumors may be monoclonal (51). This suggests that PTMCs develop from a single clone and then develop through intrathyroidal metastasis, in line with a theory that there is a rich network of microlymphatic tubes within the thyroid gland (52). PTMC spread through these lymphatic networks inside the thyroid gland and lymph nodes in the central region.
Limited reports exist regarding the relationship between LNM and the aspect ratio (53). The aspect ratio is an indicator that plays an important role in the judgment of the benign or malignant nature of thyroid nodules. It specifically refers to the ratio of the upper and lower diameters to the left and right diameters of a tumor lesion in longitudinal section, or the ratio of anterior-posterior to left-right diameters in transverse section. This ratio provides important clues about the morphology of the nodule, which in turn helps to determine its malignancy (22,54). Assessing the fatty hilum is a more intuitive means to determining the CLNM risk than are other US characteristics (55-60). However, due to the limitations of US susceptibility to interference and reliance on the skill of the practitioner, we had only 80 cases across 2 sets in which loss of fat hilum was present, accounting for 7.7% of the total cases.
TPO is the main antigen component of thyroid microsomes, and its function is related to thyroxine synthesis. Traditionally, TPOAb induces chronic inflammation of the thyroid gland and increases the risk of thyroid cancer. Therefore, TPOAb may be a promoting factor for the occurrence of CLNM (61,62). However, many reports have challenged this viewpoint (63-67), suggesting that TPOAb inhibits the occurrence of CLNM through complement- or antibody-mediated cytotoxicity. In our study, TPOAb emerged as a protective factor against CLNM, and we anticipate that this finding can be addressed in future research and applied in clinical practice. TgAb can cause chronic thyroid cell damage, may increase the risk of PTC (68-70), and may be associated with multifocal PTC, potentially leading to LNM (66). Compared to FT3 levels, higher FT4 levels are negatively correlated with PTC prevalence (71). While no previous reports have demonstrated a relationship between high FT4 levels and CLNM in PTC, our study found there to be a significant association of high FT4 levels with CLNM incidence. In recent years, more and more studies have begun to focus on the potential link between thyroid hormones and tumorigenesis (72,73). It has been reported that high levels of free thyroid hormones may promote the malignant transformation of tumor cells by activating key signaling pathways such as ERK1/ERK2 and PI3K in cancer cells, which in turn enhances their invasive and proliferative abilities. This interesting phenomenon is not limited to the thyroid gland itself, but has also been demonstrated in other types of tumors. For example, in a study of lung cancer (74), Ma et al. found that high levels of FT3 and FT4 may be associated with the development of lung malignant tumors through an in-depth analysis of thyroid function-related indicators in 527 cases.
In recent years, controversy over the treatment of PTMC has intensified due to its increasing detection rate. Several guidelines have suggested that active monitoring can be adopted for low-risk PTMC patients in place of traditional surgical treatment. As one of the important criteria for determining low-risk PTMC cases, the risk of CLNM is difficult to determine, and we applied logistic regression to analyze and organize the information of 1,023 cases, and finally established a risk prediction model for predicting the occurrence of CLNM in PTMC patients. It was categorized according to the scores and divided into high-risk, moderate-risk and low-risk groups, which has the reference value of potentially reducing over-treatment and avoiding under-treatment.
Our study has several limitations that should be mentioned. First, the data of this study were generated up to the point of pathological confirmation. Therefore, we could not analyze the predictive factors for survival outcomes. Second, performing US is likely an operator-dependent modality. US characteristics such as tumor size and aspect ratio could be impacted by the operator. Third, TPOAb and FT4 levels may fluctuate, and blood samples obtained at different times may be biased and interfere with nomogram results. Fourth, although our model has some application prospects, the positive predictive values were 28% and 37% in the training and validation cohorts, respectively, which are relatively low. Therefore, it is necessary to develop more detailed, accurate, and uniform US evaluation standards; conduct studies with larger, multicenter sets to further improve the model evaluation capabilities; and perform prospective investigations into CLND to strengthen the reliability of the model.
Conclusions
We established a comprehensive multivariate nomogram, including age, multifocality, microcalcification, loss of fatty hilum, aspect ratio, FT4 level, TPOAb level, and tumor size for predicting CLNM in PTMC to aid clinicians and patients. This tool could provide a valuable guidance for deciding on management in PTMC.
Acknowledgments
We acknowledge the patients, ultrasound, and pathologists who participated in this study.
Funding: This work was supported by
Footnote
Reporting Checklist: The authors have completed the TRIPOD reporting checklist. Available at https://gs.amegroups.com/article/view/10.21037/gs-24-154/rc
Data Sharing Statement: Available at https://gs.amegroups.com/article/view/10.21037/gs-24-154/dss
Peer Review File: Available at https://gs.amegroups.com/article/view/10.21037/gs-24-154/prf
Conflicts of Interest: All authors have completed the ICMJE uniform disclosure form (available at https://gs.amegroups.com/article/view/10.21037/gs-24-154/coif). The authors have no conflicts of interest to declare.
Ethical Statement: The authors are accountable for all aspects of the work in ensuring that questions related to the accuracy or integrity of any part of the work are appropriately investigated and resolved. The study was conducted in accordance with the Declaration of Helsinki (as revised in 2013). This study met the requirements of the Xijing Hospital Ethics Committee and Tangdu Hospital Ethics Committee for the waiver of ethical approval, and informed consent was taken from all the patients.
Open Access Statement: This is an Open Access article distributed in accordance with the Creative Commons Attribution-NonCommercial-NoDerivs 4.0 International License (CC BY-NC-ND 4.0), which permits the non-commercial replication and distribution of the article with the strict proviso that no changes or edits are made and the original work is properly cited (including links to both the formal publication through the relevant DOI and the license). See: https://creativecommons.org/licenses/by-nc-nd/4.0/.
References
- Sung H, Ferlay J, Siegel RL, et al. Global Cancer Statistics 2020: GLOBOCAN Estimates of Incidence and Mortality Worldwide for 36 Cancers in 185 Countries. CA Cancer J Clin 2021;71:209-49. [Crossref] [PubMed]
- Kaliszewski K, Diakowska D, Wojtczak B, et al. Cancer screening activity results in overdiagnosis and overtreatment of papillary thyroid cancer: A 10-year experience at a single institution. PLoS One 2020;15:e0236257. [Crossref] [PubMed]
- Back K, Lee J, Choe JH, et al. Total thyroidectomy can be overtreatment in cN1a papillary thyroid carcinoma patients whose tumor is smaller than 1 cm. Am J Surg 2022;223:635-40. [Crossref] [PubMed]
- Krajewska J, Kukulska A, Oczko-Wojciechowska M, et al. Early Diagnosis of Low-Risk Papillary Thyroid Cancer Results Rather in Overtreatment Than a Better Survival. Front Endocrinol (Lausanne) 2020;11:571421. [Crossref] [PubMed]
- Jegerlehner S, Bulliard JL, Aujesky D, et al. Overdiagnosis and overtreatment of thyroid cancer: A population-based temporal trend study. PLoS One 2017;12:e0179387. [Crossref] [PubMed]
- Solis-Pazmino P, Salazar-Vega J, Lincango-Naranjo E, et al. Thyroid cancer overdiagnosis and overtreatment: a cross- sectional study at a thyroid cancer referral center in Ecuador. BMC Cancer 2021;21:42. [Crossref] [PubMed]
- Issa PP, Munshi R, Albuck AL, et al. Recommend with caution: A meta-analysis investigating papillary thyroid carcinoma tumor progression under active surveillance. Am J Otolaryngol 2023;44:103994. [Crossref] [PubMed]
- Sugitani I, Ito Y, Takeuchi D, et al. Indications and Strategy for Active Surveillance of Adult Low-Risk Papillary Thyroid Microcarcinoma: Consensus Statements from the Japan Association of Endocrine Surgery Task Force on Management for Papillary Thyroid Microcarcinoma. Thyroid 2021;31:183-92. [Crossref] [PubMed]
- Lamartina L, Leboulleux S, Borget I, et al. Global thyroid estimates in 2020. Lancet Diabetes Endocrinol 2022;10:235-6. [Crossref] [PubMed]
- Soares P, Celestino R, Gaspar da Rocha A, et al. Papillary thyroid microcarcinoma: how to diagnose and manage this epidemic? Int J Surg Pathol 2014;22:113-9. [Crossref] [PubMed]
- Jiwang L, Yahong L, Kai L, et al. Clinicopathologic factors and preoperative ultrasonographic characteristics for predicting central lymph node metastasis in papillary thyroid microcarcinoma: a single center retrospective study. Braz J Otorhinolaryngol 2022;88:36-45. [Crossref] [PubMed]
- Lin JF, Jonker PKC, Cunich M, et al. Surgery alone for papillary thyroid microcarcinoma is less costly and more effective than long term active surveillance. Surgery 2020;167:110-6. [Crossref] [PubMed]
- Xing Z, Qiu Y, Yang Q, et al. Thyroid cancer neck lymph nodes metastasis: Meta-analysis of US and CT diagnosis. Eur J Radiol 2020;129:109103. [Crossref] [PubMed]
- Ito Y, Tomoda C, Uruno T, et al. Preoperative ultrasonographic examination for lymph node metastasis: usefulness when designing lymph node dissection for papillary microcarcinoma of the thyroid. World J Surg 2004;28:498-501. [Crossref] [PubMed]
- Wang Y, Guan Q, Xiang J. Nomogram for predicting central lymph node metastasis in papillary thyroid microcarcinoma: A retrospective cohort study of 8668 patients. Int J Surg 2018;55:98-102. [Crossref] [PubMed]
- Wen Q, Wang Z, Traverso A, et al. A radiomics nomogram for the ultrasound-based evaluation of central cervical lymph node metastasis in papillary thyroid carcinoma. Front Endocrinol (Lausanne) 2022;13:1064434. [Crossref] [PubMed]
- Leboulleux S, Girard E, Rose M, et al. Ultrasound criteria of malignancy for cervical lymph nodes in patients followed up for differentiated thyroid cancer. J Clin Endocrinol Metab 2007;92:3590-4. [Crossref] [PubMed]
- Chen Q, Liu Y, Liu J, et al. Development and validation of a dynamic nomogram based on conventional ultrasound and contrast-enhanced ultrasound for stratifying the risk of central lymph node metastasis in papillary thyroid carcinoma preoperatively. Front Endocrinol (Lausanne) 2023;14:1186381. [Crossref] [PubMed]
- Feng JW, Liu SQ, Qi GF, et al. Development and Validation of Clinical-Radiomics Nomogram for Preoperative Prediction of Central Lymph Node Metastasis in Papillary Thyroid Carcinoma. Acad Radiol 2024;S1076-6332(23)00682-7.
- Hu W, Zhuang Y, Tang L, et al. Preoperative Cervical Lymph Node Metastasis Prediction in Papillary Thyroid Carcinoma: A Noninvasive Clinical Multimodal Radiomics (CMR) Nomogram Analysis. J Oncol 2023;2023:3270137. [Crossref] [PubMed]
- Li Y, Gao X, Guo T, et al. Development and validation of nomograms for predicting the risk of central lymph node metastasis of solitary papillary thyroid carcinoma of the isthmus. J Cancer Res Clin Oncol 2023;149:14853-68. [Crossref] [PubMed]
- Lin X, Huo J, Zhang H, et al. Construction and validation of a nomogram for predicting cervical lymph node metastasis in diffuse sclerosing variant of papillary thyroid carcinoma. Langenbecks Arch Surg 2023;409:8. [Crossref] [PubMed]
- Tang J, Zhanghuang C, Yao Z, et al. Development and validation of a nomogram to predict cancer-specific survival in middle-aged patients with papillary thyroid cancer: A SEER database study. Heliyon 2023;9:e13665. [Crossref] [PubMed]
- Wang J, Gao Y, Zong Y, et al. Nomogram Model Based on Iodine Nutrition and Clinical Characteristics of Papillary Thyroid Carcinoma to Predict Lateral Lymph Node Metastasis. Cancer Control 2023;30:10732748231193248. [Crossref] [PubMed]
- Xiao W, Hu X, Zhang C, et al. Ultrasonic Feature Prediction of Large-Number Central Lymph Node Metastasis in Clinically Node-Negative Solitary Papillary Thyroid Carcinoma. Endocr Res 2023;48:112-9. [Crossref] [PubMed]
- Zhang M, Zhang Y, Qiu Y, et al. A nomogram based on ultrasound characteristics to predict large-number cervical lymph node metastasis in papillary thyroid carcinoma. Endocr J 2023;70:481-8. [Crossref] [PubMed]
- Zhong L, Xie J, Shi L, et al. Nomogram based on preoperative conventional ultrasound and shear wave velocity for predicting central lymph node metastasis in papillary thyroid carcinoma. Clin Hemorheol Microcirc 2023;83:129-36. [Crossref] [PubMed]
- Huang C, Cong S, Liang T, et al. Development and validation of an ultrasound-based nomogram for preoperative prediction of cervical central lymph node metastasis in papillary thyroid carcinoma. Gland Surg 2020;9:956-67. [Crossref] [PubMed]
- Sun J, Jiang Q, Wang X, et al. Nomogram for Preoperative Estimation of Cervical Lymph Node Metastasis Risk in Papillary Thyroid Microcarcinoma. Front Endocrinol (Lausanne) 2021;12:613974. [Crossref] [PubMed]
- Tong Y, Zhang J, Wei Y, et al. Ultrasound-based radiomics analysis for preoperative prediction of central and lateral cervical lymph node metastasis in papillary thyroid carcinoma: a multi-institutional study. BMC Med Imaging 2022;22:82. [Crossref] [PubMed]
- Zhou J, Yin L, Wei X, et al. 2020 Chinese guidelines for ultrasound malignancy risk stratification of thyroid nodules: the C-TIRADS. Endocrine 2020;70:256-79. [Crossref] [PubMed]
- Lee DW, Ji YB, Sung ES, et al. Roles of ultrasonography and computed tomography in the surgical management of cervical lymph node metastases in papillary thyroid carcinoma. Eur J Surg Oncol 2013;39:191-6. [Crossref] [PubMed]
- Sigrist RMS, Liau J, Kaffas AE, et al. Ultrasound Elastography: Review of Techniques and Clinical Applications. Theranostics 2017;7:1303-29. [Crossref] [PubMed]
- Ma T, Wang L, Zhang X, et al. A clinical and molecular pathology prediction model for central lymph node metastasis in cN0 papillary thyroid microcarcinoma. Front Endocrinol (Lausanne) 2023;14:1075598. [Crossref] [PubMed]
- Wang D, Zhu J, Deng C, et al. Preoperative and pathological predictive factors of central lymph node metastasis in papillary thyroid microcarcinoma. Auris Nasus Larynx 2022;49:690-6. [Crossref] [PubMed]
- Lee YH, Lee YM, Sung TY, et al. Is Male Gender a Prognostic Factor for Papillary Thyroid Microcarcinoma? Ann Surg Oncol 2017;24:1958-64. [Crossref] [PubMed]
- Genpeng L, Jianyong L, Jiaying Y, et al. Independent predictors and lymph node metastasis characteristics of multifocal papillary thyroid cancer. Medicine (Baltimore) 2018;97:e9619. [Crossref] [PubMed]
- Jiang LH, Yin KX, Wen QL, et al. Predictive Risk-scoring Model For Central Lymph Node Metastasis and Predictors of Recurrence in Papillary Thyroid Carcinoma. Sci Rep 2020;10:710. [Crossref] [PubMed]
- Park J, Fey JV, Naik AM, et al. A declining rate of completion axillary dissection in sentinel lymph node-positive breast cancer patients is associated with the use of a multivariate nomogram. Ann Surg 2007;245:462-8. [Crossref] [PubMed]
- Yang Q, Chen P, Hu HY, et al. Preoperative Sonographic and Clinicopathological Predictors for Solitary Lateral Neck Node Metastasis in Papillary Thyroid Carcinoma: A Retrospective Study. Cancer Manag Res 2020;12:1855-62. [Crossref] [PubMed]
- Weng HY, Yan T, Qiu WW, et al. The Prognosis of Skip Metastasis in Papillary Thyroid Microcarcinoma Is Better Than That of Continuous Metastasis. J Clin Endocrinol Metab 2022;107:1589-98. [Crossref] [PubMed]
- Liu C, Xiao C, Chen J, et al. Risk factor analysis for predicting cervical lymph node metastasis in papillary thyroid carcinoma: a study of 966 patients. BMC Cancer 2019;19:622. [Crossref] [PubMed]
- Gao X, Luo W, He L, et al. Predictors and a Prediction Model for Central Cervical Lymph Node Metastasis in Papillary Thyroid Carcinoma (cN0). Front Endocrinol (Lausanne) 2021;12:789310. [Crossref] [PubMed]
- Liu W, Wang S, Xia X. Risk Factor Analysis for Central Lymph Node Metastasis in Papillary Thyroid Microcarcinoma. Int J Gen Med 2021;14:9923-9. [Crossref] [PubMed]
- Jiwang L, Yahong L, Kai L, et al. Clinicopathologic factors and preoperative ultrasonographic characteristics for predicting central lymph node metastasis in papillary thyroid microcarcinoma: a single center retrospective study. Braz J Otorhinolaryngol 2022;88:36-45. [Crossref] [PubMed]
- James JJ, Evans AJ, Pinder SE, et al. Is the presence of mammographic comedo calcification really a prognostic factor for small screen-detected invasive breast cancers? Clin Radiol 2003;58:54-62. [Crossref] [PubMed]
- Nagashima T, Hashimoto H, Oshida K, et al. Ultrasound Demonstration of Mammographically Detected Microcalcifications in Patients with Ductal Carcinoma in situ of the Breast. Breast Cancer 2005;12:216-20. [Crossref] [PubMed]
- Kim SK, Park I, Woo JW, et al. Predictive Factors for Lymph Node Metastasis in Papillary Thyroid Microcarcinoma. Ann Surg Oncol 2016;23:2866-73. [Crossref] [PubMed]
- Qu N, Zhang L, Ji QH, et al. Risk Factors for Central Compartment Lymph Node Metastasis in Papillary Thyroid Microcarcinoma: A Meta-Analysis. World J Surg 2015;39:2459-70. [Crossref] [PubMed]
- Jung CK, Kang YG, Bae JS, et al. Unique patterns of tumor growth related with the risk of lymph node metastasis in papillary thyroid carcinoma. Mod Pathol 2010;23:1201-8. [Crossref] [PubMed]
- Jovanovic L, Delahunt B, McIver B, et al. Most multifocal papillary thyroid carcinomas acquire genetic and morphotype diversity through subclonal evolution following the intra-glandular spread of the initial neoplastic clone. J Pathol 2008;215:145-54. [Crossref] [PubMed]
- Katoh R, Sasaki J, Kurihara H, et al. Multiple thyroid involvement (intraglandular metastasis) in papillary thyroid carcinoma. A clinicopathologic study of 105 consecutive patients. Cancer 1992;70:1585-90. [Crossref] [PubMed]
- Guo JN, Song LH, Yu PY, et al. Ultrasound Elastic Parameters Predict Central Lymph Node Metastasis of Papillary Thyroid Carcinoma. J Surg Res 2020;253:69-78. [Crossref] [PubMed]
- Lei R, Wang Z, Qian L. Ultrasonic Characteristics of Medullary Thyroid Carcinoma: Differential From Papillary Thyroid Carcinoma and Benign Thyroid Nodule. Ultrasound Q 2021;37:329-35. [Crossref] [PubMed]
- Lin M, Tang X, Cao L, et al. Using ultrasound radiomics analysis to diagnose cervical lymph node metastasis in patients with nasopharyngeal carcinoma. Eur Radiol 2023;33:774-83. [Crossref] [PubMed]
- Zhao RN, Zhang B, Jiang YX. Sonographic evaluation of metastatic cervical lymph nodes. Zhongguo Yi Xue Ke Xue Yuan Xue Bao 2012;34:633-9. [PubMed]
- Chan JM, Shin LK, Jeffrey RB. Ultrasonography of abnormal neck lymph nodes. Ultrasound Q 2007;23:47-54. [Crossref] [PubMed]
- Zhang H, Hu S, Wang X, et al. Using Diffusion-Weighted MRI to Predict Central Lymph Node Metastasis in Papillary Thyroid Carcinoma: A Feasibility Study. Front Endocrinol (Lausanne) 2020;11:326. [Crossref] [PubMed]
- Zhu F, Li F, Xie X, et al. Investigating the impact of tumor location and size on the risk of recurrence for papillary thyroid carcinoma in the isthmus. Cancer Med 2023;12:13290-9. [Crossref] [PubMed]
- Zuo Q, Chen X, Yang J, et al. Analysis of the Clinical Value of Delphian Lymph Node Metastasis in Papillary Thyroid Carcinoma. J Oncol 2022;2022:8108256. [Crossref] [PubMed]
- Sun GH, Qu N, Hu JQ, et al. Risk for metastasis of lymph node between sternocleidomastoid and sternohyoid muscle in papillary thyroid cancer. Zhonghua Er Bi Yan Hou Tou Jing Wai Ke Za Zhi 2017;52:253-8. [PubMed]
- Wang Y, Zheng J, Hu X, et al. A retrospective study of papillary thyroid carcinoma: Hashimoto's thyroiditis as a protective biomarker for lymph node metastasis. Eur J Surg Oncol 2023;49:560-7. [Crossref] [PubMed]
- Huang DM, Zhi JT, Zhang JM, et al. Correlations of serum TgAb and TPOAb and clinicopathological features of PTC in children and adolescents. Zhonghua Er Bi Yan Hou Tou Jing Wai Ke Za Zhi 2022;57:1418-25. [PubMed]
- Li X, Zhang H, Zhou Y, et al. Risk factors for central lymph node metastasis in the cervical region in papillary thyroid carcinoma: a retrospective study. World J Surg Oncol 2021;19:138. [Crossref] [PubMed]
- Zhou SC, Liu TT, Zhou J, et al. An Ultrasound Radiomics Nomogram for Preoperative Prediction of Central Neck Lymph Node Metastasis in Papillary Thyroid Carcinoma. Front Oncol 2020;10:1591. [Crossref] [PubMed]
- Noel JE, Thatipamala P, Hung KS, et al. Pre-Operative Antithyroid Antibodies in Differentiated Thyroid Cancer. Endocr Pract 2021;27:1114-8. [Crossref] [PubMed]
- Li L, Shan T, Sun X, et al. Positive Thyroid Peroxidase Antibody and Thyroglobulin Antibody are Associated With Better Clinicopathologic Features of Papillary Thyroid Cancer. Endocr Pract 2021;27:306-11. [Crossref] [PubMed]
- Jia X, Pang P, Wang L, et al. Clinical Analysis of Preoperative Anti-thyroglobulin Antibody in Papillary Thyroid Cancer Between 2011 and 2015 in Beijing, China: A Retrospective Study. Front Endocrinol (Lausanne) 2020;11:452. [Crossref] [PubMed]
- Kim ES, Lim DJ, Baek KH, et al. Thyroglobulin antibody is associated with increased cancer risk in thyroid nodules. Thyroid 2010;20:885-91. [Crossref] [PubMed]
- Xiao Y, Zhou Q, Xu Y, et al. Positive thyroid antibodies and risk of thyroid cancer: A systematic review and meta-analysis. Mol Clin Oncol 2019;11:234-42. [Crossref] [PubMed]
- Guo X, Chen X, Zhang C, et al. Hyperinsulinemia and thyroid peroxidase antibody in Chinese patients with papillary thyroid cancer. Endocr J 2019;66:731-7. [Crossref] [PubMed]
- Duccini K, de Souza MVL, Delfim R, et al. High Serum Thyrotropin Concentrations within the Reference Range: A Predictor of Malignancy in Nodular Thyroid Disease. Med Princ Pract 2018;27:272-7. [Crossref] [PubMed]
- Petranović Ovčariček P, Verburg FA, Hoffmann M, et al. Higher thyroid hormone levels and cancer. Eur J Nucl Med Mol Imaging 2021;48:808-21. [Crossref] [PubMed]
- Ma Z, Song P, Ji D, et al. Thyroid hormones as biomarkers of lung cancer: a retrospective study. Ann Med 2023;55:2196088. [Crossref] [PubMed]
(English Language Editor: J. Gray)