Deep convolutional neural network model ResNeSt for discrimination of papillary thyroid carcinomas and benign nodules in thyroid nodules diagnosed as atypia of undetermined significance
Highlight box
Key findings
• Deep convolutional neural network ResNeSt can achieve high sensitivity in diagnosing malignancy in atypia of undetermined significance (AUS) nodules.
What is known and what is new?
• Compared with typical cytological images, AUS cases are heterogeneous, both diagnostic surgery and molecular testing are costly and time-consuming.
• We proposed a machine-learning method to distinguish AUS thyroid nodules based on ResNeSt. Our method has the potential to provide promising diagnostic efficiency.
What is the implication, and what should change now?
• With its high sensitivity, ResNeSt can be used as a preliminary screening tool for AUS in the future.
Introduction
The most prevalent cancer in the endocrine system is thyroid cancer (1), among which papillary thyroid carcinoma (PTC) remains the most common type, accounting for 84% of all malignancies (2). The main clinical challenge is to rule out malignancy as most thyroid nodules are asymptomatic, benign, and do not require surgical treatment. Fine-needle aspiration (FNA) biopsy is currently the gold standard test for the preoperative diagnosis of malignancies (3). Although the cytologic features of PTC are well established (4), FNA is still unable to give definitive results in approximately 10–30% of thyroid nodules (5). Thyroid lesions with such an indeterminate cytology are malignant in 10–40% of cases (6). Among these indeterminate lesions, the category “Atypia of Undetermined Significance (AUS)” according to the 2023 Bethesda System for Reporting Thyroid Cytopathology (TBSRTC), previously termed as “Atypia of Undetermined Significance/Follicular Lesion of Undetermined Significance (AUS/FLUS)”, remains heterogeneous, with a risk of malignancy (ROM) range of 13–30% (7). This indeterminate cytology is mainly due to low cellularity owing to various preanalytical factors for FNA, as it cannot assess invasive features which can be assessed on histology and also the overlap of morphology of borderline malignant thyroid neoplasms, such as follicular variant of papillary thyroid cancer (FVPTC) and noninvasive follicular thyroid neoplasm with papillary-like nuclear features (NIFTPs) (8-10). The new edition of Bethesda thyroid cytology classification also has also refined ROM estimates and proposed a more formalized subcategorization of AUS based on ROM: AUS with nuclear atypia vs. AUS-other to assist clinical intervention (7). Suggested options for identifying these nodules include a second FNA biopsy (FNAB), molecular testing, and diagnostic lobectomy. A repeat FNA can only definitively diagnosed 40% of class I and class III nodules (11), while diagnostic surgery will inevitably increase risks and costs (12). Molecular testing of the FNAB samples is currently the state-of-art approach to distinguish malignancy in AUS nodules (13-15). However, it is still costly and time-consuming. Therefore, new approaches that can generate both positive health outcomes and positive economic outputs are needed.
Recently, artificial intelligence (AI)-aided techniques have been increasingly employed to overcome the limitations of manual and complex diagnosis processes (16,17). The deep convolutional neural network (DCNN) is a branch of machine learning (ML) simulating the multilayered human cognition system (18,19). Currently, DCNN has gradually emerged to enhance diagnostic efficiency in medical practice, such as skin cancer (17), colorectal polyps (20), psychiatric problems (21), and so on. There is already abundant literature on the application of these computer tools to distinguish digital images of thyroid nodules (22-27). However, most studies have focused on radiomics, and only a small number of studies have focused on cytology and pathology. Recently, using cytology microphotographs, Sanyal et al. (28) successfully trained a convolutional neural network to classify PTC and non-PTC. Our team (29) exploited a VGG-16 DCNN model to identify PTCs from benign thyroid nodules using cytological images. We also found Inception-ResNet-v2 and VGG-19 achieved excellent diagnostic efficiencies in differentiating different histologic types of thyroid malignancy, including PTC, follicular thyroid carcinoma (FTC), medullary thyroid carcinoma (MTC), and anaplastic thyroid carcinoma (ATC) (30). More recently, using formalin-fixed paraffin-embedded (FFPE) tissue specimen, Sun et al. (22) developed an AI-defined protein-based biomarker panel for the diagnostic classification of thyroid nodules. According to these studies, DCNN has shown promising ability in automatically diagnosing and classifying thyroid nodules. However, to the best of our knowledge, DCNNs have not been applied to distinguish nodules diagnosed as AUS; thus, our work is the first study to employ a DCNN model to identify PTC and benign nodules within AUS nodules.
ResNeSt, the model we chose to classify thyroid AUS nodules, is an automated DCNN scheme which achieved 83.0% top-1 accuracy on ImageNet 2012 (31). VGG-16, another DCNN model we pretrained to compare with ResNeSt, was named after its proposal lab, Visual Geometry Group from Oxford University, achieving 92.7% top-5 test accuracy in ImageNet (29). The training results of these two models were compared at the end of this study. We present this article in accordance with the STARD reporting checklist (available at https://gs.amegroups.com/article/view/10.21037/gs-23-486/rc).
Methods
Patients and cytological images
A retrospective study was designed to assess the diagnostic efficiency of DCNN model. Well-preserved thyroid FNAs, which were performed from January 1, 2016, to July 31, 2017, in Fudan University Shanghai Cancer Center, were consecutively selected. Our dataset was divided into a training set and a test set. Eligibility criteria of the training set were nodules cytologically and histopathologically confirmed as PTC or benign nodules confirmed combined cytology with other clinical, laboratory, and imaging evaluations. Eligibility criteria of the test set were nodules cytologically fit class III according to the 2023 Bethesda system with post-surgery histopathology. Cases without qualified cytological images were excluded from the study. All images were manually segmented into several 224×224 fragments that contained at least three complete cells. In total, as shown in Table 1, the training set contained 1,330 hematoxylin and eosin (H&E)-stained slides with definitive cytology (534 benign nodules and 796 PTC, class II, V, or VI according to the Bethesda system), while the testing set contained 173 H&E-stained slides of AUS cases (class III according to the Bethesda system), statistical result of AUS nodules are shown in Table 2. Detailed cytological and pathological characteristics of AUS nodules are shown in appendix available at https://cdn.amegroups.cn/static/public/gs-23-486-1.xlsx. All AUS and PTC cases had postoperational pathology and doubled confirmed by the two pathologists, while benign cases in the training group were confirmed combined cytology with other clinical information. Fragmented pictures were trained and tested by ResNeSt and VGG-16, and the results were compared with pathology, which is widely considered as the gold standard. Figure 1 shows several slides of AUS in the test set.
Table 1
Cytological type | Training data | Test data | Total |
---|---|---|---|
PTC | 796 | 95 | 891 |
Benign nodule | 534 | 78 | 612 |
All types | 1,330 | 173 | 1,503 |
PTC, papillary thyroid carcinoma.
Table 2
Characteristics | Malignant (n=31) | Benign (n=15) |
---|---|---|
Subcategorization of cytology, n (%) | ||
AUS with nuclear atypia (n=30) | 27 (87.1) | 3 (20.0) |
AUS-other (n=16) | 4 (12.9) | 12 (80.0) |
Pathology, n (%) | ||
PTC | 31 (100.0) | – |
Follicular adenoma | – | 2 (13.3) |
Nodular goiter | – | 5 (33.3) |
Hashimoto’s thyroiditis | – | 4 (26.7) |
Granulomatous thyroiditis | – | 1 (6.67) |
Oxyphilic cell adenoma | – | 1 (6.67) |
Fibrotic nodules with calcifications | – | 1 (6.67) |
Adenomatous hyperplastic nodule | – | 1 (6.67) |
AUS was divided into benign and malignant groups according to post-surgery pathology. AUS, atypia of undetermined significance; PTC, papillary thyroid carcinoma.
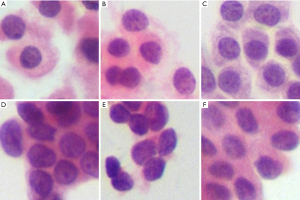
The study was conducted in accordance with the Declaration of Helsinki (as revised in 2013). Tissue bank ethical approvals were obtained from the research ethics committee of Fudan University Shanghai Cancer Center (No. IRB1612167-18), and the data were deidentified without impacting patient care. Oral and written informed consent was obtained from all patients after the nature of the procedures had been fully explained. All H&E-stained thin layer liquid-based cytology (LBC) preparations were scanned using a digital still camera (DP27, Olympus, Tokyo, Japan) at 400× objective magnification (29).
From a patient wise viewpoint, the nodule of which the fragmented images were all diagnosed as benign by DCNN was diagnosed as a benign one; otherwise, the nodule was considered to be malignant.
Network architecture
ResNeSt and VGG-16 were used in the experiments. The default image size of ResNeSt and VGG-16 was 224×224. ResNeSt leverages a special split-attention block, which contains several feature map groups and split attention operations (31). The cardinality hyperparameter K controls the number of feature map groups in each ResNeSt block, and the radix hyperparameter R indicates the number of splits in each cardinal group. The structure of a ResNeSt block is shown in Figure 2. The detailed structure of a split attention block is shown in Figure 3. The architecture of VGG-16 is shown in Figure 4.
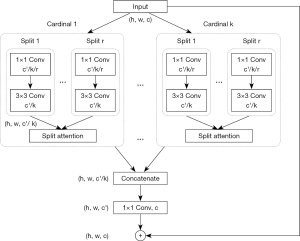
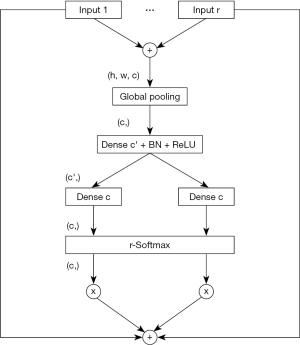
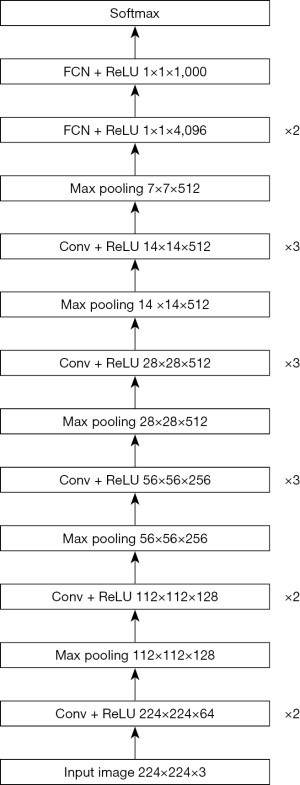
Dataset settings and training details
Our work was built upon MMClassification as a codebase, and if it was not specified, we applied the default settings (32). We set R=2 and K=2. To minimize the parameters, we adopted ResNeSt with 50 layers followed by a global averaging pooling layer as the neck. The final layer of prediction output only two possibilities, as there were only two categories in our dataset. The setting split the whole dataset at a ratio of 6:1, which was consistent with common practice.
Quantification of tumor cells
We converted the images into grayscale images and applied the Laplacian operator on the grayscale images, which calculated the second derivatives of pixels across the contour, and we employed powerful threshold segmentation algorithms, including Otsu (33), to filter out the contours. Otsu is a method for automatic image thresholding named after Nobuyuki Otsu. By minimizing the intraclass intensity variance, the Otsu algorithm determines the threshold and returns an intensity threshold to filter out the contours. Contour features, including perimeter, area, and average gray scale of cells per fragmented image, were calculated and compared between benign AUS cells and malignant AUS cells. In particular, it should be noted that gray scale, referring to the brightness of a single pixel, is a numerical value that indicates the brightness and darkness of the image, 255 for white and 0 for black. Higher grayscale values indicate brighter colors and lower grayscale values indicate darker colors.
Statistical analysis
Accuracy, sensitivity, specificity, positive predictive value, and negative predictive value were calculated to evaluate the diagnostic efficiency of DCNN. To estimate the agreement between the DCNN and pathological results, we used Cohen’s κ statistics. The Wilcoxon test was used to compare variables between benign AUS cases and malignant AUS cases. A P value less than 0.05 was considered significant. Statistical analyses were performed using IBM SPSS 25.0 for Windows (SPSS Inc., Chicago, IL, USA).
Results
Table 3 shows the cross tabulation of ResNeSt and VGG-16 result by the results of pathology. Table 4 shows diagnostic efficiency of the two models, such as sensitivity and specificity. ResNeSt achieved an accuracy of 92.49% on the fragmented images and 84.78% (39/46) from a patient wise viewpoint. Using the VGG-16 model, an accuracy of 88.44% was achieved on the fragmented images, and the accuracy rate from a patient wise viewpoint was 80.43% (37/46). In these experiments, ResNeSt outperformed the VGG-16 model.
Table 3
Model | Result | Pathology | |
---|---|---|---|
Malignant | Benign | ||
ResNeSt | Malignant | 91 | 9 |
Benign | 4 | 69 | |
VGG-16 | Malignant | 87 | 12 |
Benign | 8 | 66 |
Table 4
Diagnostic efficiency | ResNeSt | VGG-16 |
---|---|---|
Accuracy (%) | 92.49 | 88.44 |
Sensitivity (95% CI) (%) | 95.79 (88.96–98.64) | 91.58 (83.61–96.03) |
Specificity (95% CI) (%) | 88.46 (78.74–94.26) | 84.62 (74.27–91.46) |
Positive predictive value (95% CI) (%) | 91.00 (83.17–95.54) | 87.88 (79.41–93.31) |
Negative predictive value (95% CI) (%) | 94.52 (85.84–98.23) | 89.19 (79.28–94.88) |
κ value (95% CI), P value | 0.847 (0.767–0.927), <0.001 | 0.765 (0.669–0.861), <0.001 |
CI, confidence interval.
We used Cohen’s κ statistics to evaluate the agreement between ResNeSt and the pathological results. The κ value was 0.847 (P<0.001), indicating that ResNeSt had almost perfect agreement with the pathological results, while the κ value between VGG-16 and the pathological results was 0.765 (P<0.001).
We also further analyzed ResNeSt and VGG-16’s diagnostic efficiency in the subcategorization of AUS. Table 5 shows the number of fragmented images and nodules accord with each subcategorization. Table 6 shows the diagnostic efficiency of two models.
Table 5
Subcategorization | Fragmented images | Nodules |
---|---|---|
AUS with nuclear atypia | 111 | 30 |
Benign | 26 | 3 |
PTC | 85 | 27 |
AUS-other | 62 | 16 |
Benign | 52 | 12 |
PTC | 10 | 4 |
AUS, atypia of undetermined significance; PTC, papillary thyroid carcinoma.
Table 6
Diagnostic efficiency | ResNeSt | VGG-16 | |||
---|---|---|---|---|---|
AUS with nuclear atypia | AUS-other | AUS with nuclear atypia | AUS-other | ||
Accuracy (%) | 94.59 | 88.71 | 90.99 | 83.87 | |
Sensitivity (%) | 95.29 | 100.00 | 91.76 | 90.00 | |
Specificity (%) | 92.31 | 86.54 | 88.46 | 82.70 | |
Positive predictive value (%) | 97.59 | 58.82 | 96.30 | 50.00 | |
Negative predictive value (%) | 85.71 | 100.00 | 76.67 | 97.72 |
AUS, atypia of undetermined significance.
The quantification data are shown in Table 7. The area [2,340.00 (1,769.00, 2,807.00) vs. 1,941.00 (1,567.50, 2,455.75), P<0.001], perimeter [190.46 (167.64, 208.46) vs. 171.71 (154.95, 193.65), P<0.001] and mean grayscale values [37.52 (31.41, 46.67) vs. 45.84 (31.88, 57.36), P<0.001] were found to have significant differences between malignant AUS cell nuclei and benign AUS ones, indicating that malignant ones had larger nuclei and deeper staining.
Table 7
Characteristics | Malignant | Benign | Z value | P value |
---|---|---|---|---|
Area (pixel × pixel) | 2,340.00 (1,769.00, 2,807.00) | 1,941.00 (1,567.50, 2,455.75) | 6.824 | <0.001 |
Perimeter (pixel) | 190.46 (167.64, 208.46) | 171.71 (154.95, 193.65) | 7.798 | <0.001 |
Grayscale | 37.52 (31.41, 46.67) | 45.84 (31.88, 57.36) | 5.748 | <0.001 |
Data are presented as median (IQR). IQR, interquartile range.
Compared with VGG-16, ResNeSt exhibited better performance in overall diagnostic efficiency; thus, we decided to use ResNeSt for future investigation. Two misdiagnosed AUS fragmented pictures are shown in Figure 5. Figure 5A is pathologically confirmed to be benign nodules but falsely diagnosed as PTC by ResNeSt. Figure 5B is confirmed to be PTC but mistakenly diagnosed as benign nodules by ResNeSt.
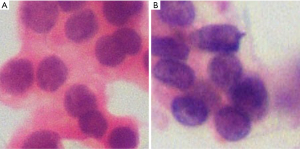
Discussion
As the ROM of AUS ranges from 13–30%, it’s of great significance to distinguish malignant cases from benign cases. However, both diagnostic operation and molecular testing are costly and time-consuming, and patients may assume additional risks with diagnostic operation. In contrast, DCNN may be served as a fast and non-invasive screening method to assist such a demanding task. Computer-aided methods have been employed in atypical cases in other malignancy. For example, using cytological images, multilayer neural network (MNN) achieved 100% accuracy in distinguish benign from malignant pancreatic nodules, and it achieved 77% accuracy in categorizing atypical cases into benign or malignant (34).
In our previous work, we found that the VGG-16 model of DCNN demonstrated great performance in distinguishing typical thyroid cytological images (Bethesda classes II, V, and VI) (29). In this study, we would like to investigate the ability of DCNN to classify AUS images. After training, ResNeSt achieved a specificity of 88.46%, better than previous state-of-the-art gene-expression classifier methods (a specificity of 80%) for identifying thyroid nodules with indeterminate cytology (35,36). With its high sensitivity of 94.51%, ResNeSt can be used as a preliminary screening tool for AUS in the future, as ML become maturer and expand to more subtypes of thyroid nodules, it may be served as a substitute for molecular testing because it’s more cost-efficient and less time-consuming.
Then we analyzed further the diagnostic efficiency of ResNeSt and VGG-16 in subcategorization of AUS. In both AUS with nuclear atypia and AUS-other, ResNeSt achieved a better performance than VGG-16. As malignancy rate was much higher in AUS with nuclear atypia than AUS-other, subcategorization of AUS can provide more information for clinical management, therefore, future studies can investigate DCNN’s capability in automatic subcategorization of AUS.
There is still room for improvement. We analyzed some misdiagnosed fragmented images. With selected pictures, we found that the images were indeed indistinguishable. In that case, we assumed that ResNeSt made the diagnosis based on the shape but not the size and staining of the nucleus, as the PTC misdiagnosed as benign nodules demonstrated a more regular shape, although the staining and size of the nucleus were much closer to those of PTC. As our previous study assumed that VGG-16 made the diagnosis based on the size and staining of the nucleus, it is likely that different DCCN models make the diagnosis based on different characteristics; thus, a combination of different models may provide better categorization. Future studies could investigate this hypothesis.
We also further analyzed the intrinsic factors of the models. ResNeSt belongs to the ResNet family, which introduces residual learning. To enlarge the receptive field of the network and improve interactions between channels, ResNeSt leverages split-attention blocks to hierarchically and gradually adjust the weights of channel attention. This improvement empowers the network with remarkable feature extraction ability. VGG-16 uses several consecutive 3×3 convolutional kernels along with repeated VGG blocks to obtain better image feature representation. In regard to the deep network, VGG shows inferior results compared to ResNeSt because of the problem of degradation. Our experimental results further consolidated our analysis.
However, as we only included AUS of which the postoperational pathology were PTC or benign nodules in this study, different pathological subtypes can be included to broaden the application of ResNeSt. We have already achieved a satisfactory result in selected patients, and we could test the model in wider range of crowds in the future.
Conclusions
We proposed a machine-learning method to distinguish AUS thyroid nodules based on ResNeSt. Our method has the potential to provide promising diagnostic efficiency.
Acknowledgments
Funding: This study was funded by
Footnote
Reporting Checklist: The authors have completed the STARD reporting checklist. Available at https://gs.amegroups.com/article/view/10.21037/gs-23-486/rc
Data Sharing Statement: Available at https://gs.amegroups.com/article/view/10.21037/gs-23-486/dss
Peer Review File: Available at https://gs.amegroups.com/article/view/10.21037/gs-23-486/prf
Conflicts of Interest: All authors have completed the ICMJE uniform disclosure form (available at https://gs.amegroups.com/article/view/10.21037/gs-23-486/coif). The authors have no conflicts of interest to declare.
Ethical Statement: The authors are accountable for all aspects of the work in ensuring that questions related to the accuracy or integrity of any part of the work are appropriately investigated and resolved. The study was conducted in accordance with the Declaration of Helsinki (as revised in 2013). Tissue bank ethical approvals were obtained from the research ethics committee of Fudan University Shanghai Cancer Center (No. IRB1612167-18), and the data were deidentified without impacting patient care. Oral and written informed consent was obtained from all patients after the nature of the procedures had been fully explained.
Open Access Statement: This is an Open Access article distributed in accordance with the Creative Commons Attribution-NonCommercial-NoDerivs 4.0 International License (CC BY-NC-ND 4.0), which permits the non-commercial replication and distribution of the article with the strict proviso that no changes or edits are made and the original work is properly cited (including links to both the formal publication through the relevant DOI and the license). See: https://creativecommons.org/licenses/by-nc-nd/4.0/.
References
- Boucai L, Zafereo M, Cabanillas ME. Thyroid Cancer: A Review. JAMA 2024;331:425-35. [Crossref] [PubMed]
- Kang YJ, Lee HW, Stybayeva G, et al. Comparison of Liquid-Based Preparations with Conventional Smears in Thyroid Fine-Needle Aspirates: A Systematic Review and Meta-Analysis. Cancers (Basel) 2024;16:751. [Crossref] [PubMed]
- Alyusuf EY, Alhmayin L, Albasri E, et al. Ultrasonographic predictors of thyroid cancer in Bethesda III and IV thyroid nodules. Front Endocrinol (Lausanne) 2024;15:1326134. [Crossref] [PubMed]
- Mendes JM, Elsheikh TM, Di Marco J, et al. Are there specific cytologic features that can predict BRAF(V600E) mutational status of papillary thyroid carcinoma in fine-needle aspiration specimens? Diagn Cytopathol 2024;52:295-302. [Crossref] [PubMed]
- Luong G, Idarraga AJ, Hsiao V, et al. Risk Stratifying Indeterminate Thyroid Nodules With Machine Learning. J Surg Res 2022;270:214-20. [Crossref] [PubMed]
- Hier J, Avior G, Pusztaszeri M, et al. Molecular testing for cytologically suspicious and malignant (Bethesda V and VI) thyroid nodules to optimize the extent of surgical intervention: a retrospective chart review. J Otolaryngol Head Neck Surg 2021;50:29. [Crossref] [PubMed]
- Ali SZ, Baloch ZW, Cochand-Priollet B, et al. The 2023 Bethesda System for Reporting Thyroid Cytopathology. Thyroid 2023;33:1039-44. [Crossref] [PubMed]
- Huang D, Zhang H, Li L, et al. Proteotypic Differences of Follicular-Patterned Thyroid Neoplasms. Front Endocrinol (Lausanne) 2022;13:854611. [Crossref] [PubMed]
- Baloch ZW, Asa SL, Barletta JA, et al. Overview of the 2022 WHO Classification of Thyroid Neoplasms. Endocr Pathol 2022;33:27-63. [Crossref] [PubMed]
- Xu B, Serrette R, Tuttle RM, et al. How Many Papillae in Conventional Papillary Carcinoma? A Clinical Evidence-Based Pathology Study of 235 Unifocal Encapsulated Papillary Thyroid Carcinomas, with Emphasis on the Diagnosis of Noninvasive Follicular Thyroid Neoplasm with Papillary-Like Nuclear Features. Thyroid 2019;29:1792-803. [Crossref] [PubMed]
- Allen L, Al Afif A, Rigby MH, et al. The role of repeat fine needle aspiration in managing indeterminate thyroid nodules. J Otolaryngol Head Neck Surg 2019;48:16. [Crossref] [PubMed]
- Stewart R, Leang YJ, Bhatt CR, et al. Quantifying the differences in surgical management of patients with definitive and indeterminate thyroid nodule cytology. Eur J Surg Oncol 2020;46:252-7. [Crossref] [PubMed]
- Nikiforova MN, Mercurio S, Wald AI, et al. Analytical performance of the ThyroSeq v3 genomic classifier for cancer diagnosis in thyroid nodules. Cancer 2018;124:1682-90. [Crossref] [PubMed]
- Steward DL, Carty SE, Sippel RS, et al. Performance of a Multigene Genomic Classifier in Thyroid Nodules With Indeterminate Cytology: A Prospective Blinded Multicenter Study. JAMA Oncol 2019;5:204-12. [Crossref] [PubMed]
- Patel KN, Angell TE, Babiarz J, et al. Performance of a Genomic Sequencing Classifier for the Preoperative Diagnosis of Cytologically Indeterminate Thyroid Nodules. JAMA Surg 2018;153:817-24. [Crossref] [PubMed]
- Yu KH, Beam AL, Kohane IS. Artificial intelligence in healthcare. Nat Biomed Eng 2018;2:719-31. [Crossref] [PubMed]
- Syrykh C, Abreu A, Amara N, et al. Accurate diagnosis of lymphoma on whole-slide histopathology images using deep learning. NPJ Digit Med 2020;3:63. [Crossref] [PubMed]
- Deng X, Dragotti PL. Deep Convolutional Neural Network for Multi-Modal Image Restoration and Fusion. IEEE Trans Pattern Anal Mach Intell 2021;43:3333-48. [Crossref] [PubMed]
- Kim YJ, Choi Y, Hur SJ, et al. Deep convolutional neural network for classification of thyroid nodules on ultrasound: Comparison of the diagnostic performance with that of radiologists. Eur J Radiol 2022;152:110335. [Crossref] [PubMed]
- Chen PJ, Lin MC, Lai MJ, et al. Accurate Classification of Diminutive Colorectal Polyps Using Computer-Aided Analysis. Gastroenterology 2018;154:568-75. [Crossref] [PubMed]
- Durstewitz D, Koppe G, Meyer-Lindenberg A. Deep neural networks in psychiatry. Mol Psychiatry 2019;24:1583-98. [Crossref] [PubMed]
- Sun Y, Selvarajan S, Zang Z, et al. Artificial intelligence defines protein-based classification of thyroid nodules. Cell Discov 2022;8:85. [Crossref] [PubMed]
- Chen Y, Gao Z, He Y, et al. An Artificial Intelligence Model Based on ACR TI-RADS Characteristics for US Diagnosis of Thyroid Nodules. Radiology 2022;303:613-9. [Crossref] [PubMed]
- Tessler FN, Thomas J. Artificial Intelligence for Evaluation of Thyroid Nodules: A Primer. Thyroid 2023;33:150-8. [Crossref] [PubMed]
- Jassal K, Koohestani A, Kiu A, et al. Artificial Intelligence for Pre-operative Diagnosis of Malignant Thyroid Nodules Based on Sonographic Features and Cytology Category. World J Surg 2023;47:330-9. [Crossref] [PubMed]
- Huang P, Zheng B, Li M, et al. The Diagnostic Value of Artificial Intelligence Ultrasound S-Detect Technology for Thyroid Nodules. Comput Intell Neurosci 2022;2022:3656572. [Crossref] [PubMed]
- Gao X, Ran X, Ding W. The progress of radiomics in thyroid nodules. Front Oncol 2023;13:1109319. [Crossref] [PubMed]
- Sanyal P, Mukherjee T, Barui S, et al. Artificial Intelligence in Cytopathology: A Neural Network to Identify Papillary Carcinoma on Thyroid Fine-Needle Aspiration Cytology Smears. J Pathol Inform 2018;9:43. [Crossref] [PubMed]
- Guan Q, Wang Y, Ping B, et al. Deep convolutional neural network VGG-16 model for differential diagnosing of papillary thyroid carcinomas in cytological images: a pilot study. J Cancer 2019;10:4876-82. [Crossref] [PubMed]
- Wang Y, Guan Q, Lao I, et al. Using deep convolutional neural networks for multi-classification of thyroid tumor by histopathology: a large-scale pilot study. Ann Transl Med 2019;7:468. [Crossref] [PubMed]
- Zhang H, Wu C, Zhang Z, et al. Resnest: Split-Attention Networks. In: Proceedings of the IEEE/CVF Conference on Computer Vision and Pattern Recognition. 2022:2736-46.
- Chen K, Wang J, Pang J, et al. MMDetection: Open mmlab detection toolbox and benchmark. arXiv:1906.07155. [Preprint]. 2019. Available online: https://arxiv.org/abs/1906.07155
- Hagara M, Šatka A, Ondráček O, et al. Comparison of Otsu's and Rosin's Methods for Threshold Determination. In: 2023 12th Mediterranean Conference on Embedded Computing (MECO). IEEE; 2023:1-4.
- Momeni-Boroujeni A, Yousefi E, Somma J. Computer-assisted cytologic diagnosis in pancreatic FNA: An application of neural networks to image analysis. Cancer Cytopathol 2017;125:926-33. [Crossref] [PubMed]
- Livhits MJ, Zhu CY, Kuo EJ, et al. Effectiveness of Molecular Testing Techniques for Diagnosis of Indeterminate Thyroid Nodules: A Randomized Clinical Trial. JAMA Oncol 2021;7:70-7. [Crossref] [PubMed]
- Alexander EK, Kennedy GC, Baloch ZW, et al. Preoperative diagnosis of benign thyroid nodules with indeterminate cytology. N Engl J Med 2012;367:705-15. [Crossref] [PubMed]