Ultrasound deep learning radiomics and clinical machine learning models to predict low nuclear grade, ER, PR, and HER2 receptor status in pure ductal carcinoma in situ
Highlight box
Key findings
• The ultrasound deep learning radiomics models developed by using RadImageNet had higher performance than deep learning models using ImageNet to identify low nuclear grade and underlying molecular markers of ductal carcinoma in situ. The new clinical machine learning models that may help predict the low nuclear grade, estrogen receptor positivity, progesterone receptor positivity, and human epidermal growth factor receptor 2 positivity ductal carcinoma in situ (DCIS) lesions were developed and validated.
What is known and what is new?
• The ultrasound features of ductal carcinoma in situ are diverse. The ultrasound characteristics of ductal carcinoma in situ are related to nuclear grade and molecular markers.
• This study provided the novel ultrasound deep learning radiomics and clinical machine learning models to identify nuclear grade and molecular markers of DCIS.
What is the implication, and what should change now?
• The study provided the novel ultrasound artificial intelligence models that may be used to preoperative assessment for ductal carcinoma in situ patients, so that patients can benefit from hierarchical and personalized treatment.
Introduction
In 2022, newly diagnosed ductal carcinoma in situ (DCIS) cases account for about 15% of diagnosed new breast cancer (1). Because DCIS is considered a noninvasive cancer with a low mortality rate, personalized treatment methods are increasingly being recommended (2-7). Several clinical trials are currently investigating individualized proactive surveillance based on genetic heterogeneity, tumor histologic grade, and biomarker status (2-4).
The preclinical detectable period of low-grade DCIS is longer than that of high-grade DCIS and should be managed with caution to reduce overtreatment (5). For example, proactive surveillance should be selected for patients with early detection of DCIS with low nuclear grade to avoid surgical overtreatment because the choice of treatment modality for low-grade DCIS does not affect overall survival (8).
Ultrasound is economical, convenient, and has advantages in detecting non-calcified DCIS lesions in dense breast tissue (9). The ultrasound detection rate of DCIS increased significantly over a 10-year period, with an increase in screening rate of low and moderate nuclear grade over the same period (10). Population-based mammography screening has a low cancer detection rate for low-grade DCIS (11). According to previous studies, cases of DCIS detected during ultrasound screening were not as invasive as DCIS detected on mammography, which may indicate that ultrasound has advantages for screening and regular imaging examination for this population of patients with low-grade DCIS (10,12). Moreover, human epidermal growth factor receptor 2 (HER2) positivity is associated with secondary breast cancer in patients with DCIS detected through ultrasound screening (13). Several previous clinical studies have confirmed that the ultrasonographic characteristics of DCIS are related to its pathology (14-17). Ultrasonographic findings of the mass and lack of calcifications are associated with low nuclear grade DCIS (14,15). Microcalcification is related to HER2+ DCIS (15). High grade DCIS often manifests with calcification and ductal changes (17).
Clinically, routine estrogen receptor (ER) or progesterone receptor (PR) tests are performed in patients with DCIS to determine the optimal adjuvant treatment after surgery (18). Patients with ER−, PR−, and HER2+ tumors are considered to be at high risk, therefore a more active treatment is needed (19). Patients with ER+ DCIS benefit from tamoxifen treatment (20), and HER2 overexpression is associated with increased recurrence risk and a predicted benefit of radiotherapy (21).
Preoperative imaging-guided core biopsy is an invasive testing method. The collected specimens are often inadequate and carry the risk of underdiagnosis, and the results of core biopsies are often not representative of the final surgical histopathology result (22). Lee et al. found that approximately 40% of cases with low nuclear grade diagnosed by biopsy were upgraded after surgery (23).
Recent studies have shown that the “white box” machine learning model based on image features has potential applications in studying the grading and molecular level of breast cancer (24-27). The advantage of these interpretive models is that they highlight the importance of image features to guide clinical practice, while the disadvantage is that image feature extraction is influenced by interobserver variability (25-27). Radiomics is a preeminent technique that converts medical images into high-throughput features (28). However, the traditional radiomics features are hand crafted which may not be the best design to target clinical issues, therefore limiting their predictive validity (29). Moreover, labeling the region of interest is time-consuming (30). Due to the heterogeneity and diverse growth distribution patterns of DCIS tumor cells (31), it is difficult to determine the boundaries of tumors. Accurately extracting the contours of non-mass DCIS is challenging. Recently, with the development of deep learning (DL) techniques, neural networks are more commonly used in radiomics studies and have achieved expert-level performance in medical image analysis (32,33). However, the degree of transparency in feature extraction is still unclear.
In view of the above, we present this article, wherein we evaluated DCIS using different methods; specifically, we aimed to develop and evaluate deep learning radiomics (DLR) and clinical machine learning (CML) models in identifying nuclear grade and molecular markers of DCIS in ultrasound images. Moreover, we compared and discussed the performances of CML and DLR models. We present this article in accordance with the TRIPOD reporting checklist (available at https://gs.amegroups.com/article/view/10.21037/gs-23-417/rc).
Methods
Patient data preparation
The study was conducted in accordance with the Declaration of Helsinki (as revised in 2013). The study was approved by the Ethics Committee of West China Hospital of Sichuan University (No. 2022-1612) and individual consent for this retrospective analysis was waived. Our study retrieved data from a hospital database and retrospectively analyzed 630 consecutive patients with a diagnosis of pure DCIS breast cancer between April 2003 and November 2019. All patients were confirmed by surgical pathology and underwent preoperative ultrasound examination. Among these, 238 patients with missing data were excluded, 24 patients were excluded due to the presence of mucinous carcinoma, and two male patients with DCIS were excluded. Of the remaining 366 patients with DCIS, 17 were excluded due to negative ultrasound images or ultrasound images that were inconsistent with the pathology results. The final 349 patients with DCIS were all female and had fully intact surgical excisional lesions with 2-mm negative margins with no or microinvasive tumor growth. Our study employed a previously used database; the aforementioned study identified 255 patients with pure DCIS from our hospital, which were used for a DL classification study with microinvasive ductal carcinoma (34). In the present study, we expanded the database to focus on identifying cases of low nuclear grade pure DCIS and their molecular markers. We excluded microinvasive cancers with higher risks, so the developed models are more suitable for accurate risk stratification.
Clinical feature selection
The ultrasound devices included equipment from Philips, Siemens, Hitachi, GE, Sonic (Italy), and Mindray (probe frequencies, 3–15 MHz). The ultrasonographic examination method of the current study was comparable to that of a previous study (34). All breast examinations were performed manually, and ultrasound images of the largest and shortest lesion diameters were routinely taken. Each patient had a stored ultrasound report for reference. Three experienced radiologists with an average of 10 years of experience in breast disease diagnosis, extracted the ultrasound features according to the 5th Edition of the American College of Radiology Breast Imaging Reporting and Data System (BI-RADS) standard (35). When their descriptions differed, the leader of the breast professional radiological group (with 30 years of experience) made the final judgment.
For the current study, the following variables were adopted: age, background texture, ultrasonographic manifestations, echogenic foci, duct changes, structural distortions, infiltration of the fat layer, and BI-RADS category. Based on the BI-RADS standard, the breast tissue background echotexture was divided into fat/fibroglandular echotexture and heterogeneous echotexture. Lesions were divided into mass and non-mass-like lesions A mass is defined as a mass that can be identified on multiple ultrasound sections (34).
Any of the following situations were considered as a duct change: (I) duct dilation occurring in the lesion; (II) ductal extension into the lesion; (III) single duct dilation; (IV) several irregular duct dilations; and (V) intraductal fragmentary solid component or debris (36). Any continuous interruption of the fat layer above the lesion was defined as fat layer infiltration. In our institution, fat layer infiltration is an indicator for routine evaluation of breast lesions. The definition of structural distortion was based on the destruction of the anatomical plane (36).
Pathological analysis
Pathological data were obtained from final postoperative pathology reports. Nuclear grades are classified as low, medium, and high according to World Health Organization (WHO) standards. Patients with DCIS were divided into low and medium-to-high nuclear grade group (23). ER positivity and PR positivity were defined as ≥1% of cells with positive nuclear staining (37). The expression of HER2 was analyzed according to immunohistochemical methods. According to HER2 guidelines, based on the staining rate of cancer cells, as well as the staining intensity and integrity of the cell membrane, the HER2 expression score was categorized as 0, 1+, 2+, and 3+ (38-40). In this study, 3+ was defined as HER2 positivity whereas scores of 0, 1+, and 2+ were defined as HER2 negativity. Ki-67 <14% and ≥14% indicated low and high expression levels, respectively (41).
Ultrasound data preprocessing
To maintain a high quality of ultrasound images, we conducted a thorough screening process, low-quality images that significant loss of resolution were removed. We divided each ultrasound image subtype into a training set (80%) and a validation set (20%). We employed data augmentation techniques such as rotation, flipping, and scaling to increase the size and diversity of the training dataset during neural network training. This move can enhance the model’s generalization ability. After data augmentation, all images were resized to 224×224 pixels. Image standardization ensured the stability and repeatability of artificial intelligence (AI) models.
DLR and CML modeling strategy
To identify low nuclear grade, ER+, PR+, and HER2+ DCIS lesions, we conducted four independent tasks in this study; each task was randomly distributed independently. To extract the features and classify each nuclear grade and molecular marker subtype, we developed two main ultrasound-based models, namely a DLR model and a CML model. The workflow of our study is shown in Figure 1.
Firstly, we used convolutional neural network (CNN) models pretrained on RadImageNet and ImageNet as the basis for transfer learning, including ResNet50 (42), InceptionV3 (43), and DenseNet121 (44). The DL models were trained and compared to identify the advantages of RadImageNet. Then, the DLR models were constructed based on RadImageNet pretrained models and as a comparison to the above DL models, to determine the best ultrasound-based modeling method. The CML models were built using the features chosen by experienced radiologists as the input. Logistic regression (LR), support vector machine (SVM), random forest (RF), and eXtreme Gradient Boosting (XGBoost) were implemented for the final classification modeling.
RadImageNet versus ImageNet
Due to the limited availability of annotated images and computing resources required for training new models from scratch, transfer learning has emerged as a popular approach in DL. By leveraging knowledge gained from pre-trained models, transfer learning can expedite the training process, improve model performance, and expand the scope of practical applications of DL in various fields (45). Transfer learning has been extensively explored in medical imaging AI applications due to the high performance of the models pretrained with ImageNet (46). Here, we mainly pretrained with RadImageNet, which is an open radiologic dataset (47) for effective transfer learning to compare it with ImageNet. The DL models were pretrained using RadImageNet and ImageNet respectively, then compared to select the best modeling method.
DL training
In this study, the DL network for differentiating nuclear grades and molecular marker levels was trained in two stages: pretraining and fine-tuning. In the pretraining, the network was trained on the RadImageNet dataset, and in the fine-tuning step, the pretrained network was further trained on local breast images. We used two fully connected layers, and a softmax function was applied to perform the final classification. Fine-tuning helps to adapt a pretrained CNN to a different dataset by updating the pretrained weights using backpropagation (48,49).
All the DL models were implemented using the Keras framework (50), and the Adam optimizer with an initial learning rate of 0.001 was used to train all networks. The training batch size was 16 for all models.
Handling of imbalanced datasets
We used two types of loss functions to handle different classifications in our study. For the balanced datasets ER and PR, we used cross entropy (CE) as the loss function.
where denotes the ground-truth class, and refers to the model’s estimated probability for the class with label . We calculated total loss as follows:
where N denotes the total number of training images, represents the ground truth label of the image, and stands for the probability that the image is positive as predicted by the model.
For the imbalanced datasets HER2, and nuclear grade, we used focal loss as the loss function.
We set and in this focal loss function.
DLR modeling
We used the RadImageNet pretrained network to construct the DLR models. As an end-to-end method, DLR can directly operate the whole image, avoid the tedious feature extraction process, and improve the prediction efficiency of the model. The pretrained deep neural network automatically learned and extracted hierarchical imaging features. Then, these DLR features were divided into training (80%) and validation (20%) datasets and used as inputs for the training and validation sets of machine learning models (LR, SVM, RF, and XGBoost) to finally classify the nuclear grade and molecular markers. Five-fold cross-validation was performed in the training sets and the models were evaluated in the validation sets.
CML modeling
We randomly divided the clinical features into training (80%) and validation (20%) datasets, consistent with the DLR grouping, and employed grid search to find the optimal parameters of machine learning algorithms (LR, SVM, RF, and XGBoost) for each task. Five-fold cross-validation and independent validation were implemented in the training and validation sets, respectively.
Evaluation metrics and statistical analysis
We trained the classification models for each nuclear grade and molecular marker type separately and compared the accuracy (ACC), sensitivity, specificity, and F1 score of the DLR and CML models. We also analyzed the receiver operating characteristic (ROC) curve and calculated the optimal area under the ROC curve (AUC) for different nuclear grade and molecular marker types. Quantitative baseline features between groups were compared using the t-test, and intergroup differences in rates were compared using the chi-squared test. A two-sided P value <0.05 was considered statistically significant. Differences among AUCs were compared using the DeLong test. The following formulas were used for sensitivity and specificity:
Obtain the AUC threshold by calculating the Youden index. All machine learning modeling and statistical analyses were implemented by using Python (version 3.8) and SPSS (version 22.0).
Results
Patient baseline characteristics in each classification task
All 349 patients entering the trial were female, ranging from 29 to 83 years old. Due to the lack of pathological information in a small number of patients, the available datasets were as follows: 281 patients (799 images) with information on nuclear grade, 271 patients (776 images) with information on ER status, 270 patients (767 images) with information on PR status, and 267 patients (763 images) with information on HER2 status. Table 1 compares the baseline data between the training and validation groups for each of the four tasks of identifying patients with low nuclear grade, ER+, PR+, and HER2+ DCIS.
Table 1
Characteristics | Training set | Validation set | P |
---|---|---|---|
Task 1: nuclear grade | n=224 | n=57 | |
Age (years) | 49.10±11.70 | 50.23±13.14 | 0.33 |
Size (mm) | 19.96±12.79 | 21.82±13.49 | 0.28 |
Necrosis | 0.55 | ||
Yes | 58 (25.89) | 10 (17.54) | |
No | 140 (62.50) | 39 (68.42) | |
Missing | 26 (11.61) | 8 (14.04) | |
Ki-67 | 0.66 | ||
Negative | 142 (63.39) | 30 (52.63) | |
Positive | 82 (36.61) | 27 (47.37) | |
Missing | 0 | 0 | |
Task 2: ER | n=215 | n=56 | |
Age (years) | 50.13±12.29 | 49.82±11.53 | 0.86 |
Size (mm) | 20.72±13.30 | 19.68±9.29 | 0.65 |
Necrosis | 0.94 | ||
Yes | 43 (20.00) | 11 (19.64) | |
No | 112 (52.09) | 29 (51.79) | |
Missing | 60 (27.91) | 16 (28.57) | |
Ki-67 | 0.25 | ||
Negative | 113 (52.56) | 23 (41.07) | |
Positive | 80 (37.21) | 29 (51.79) | |
Missing | 22 (10.23) | 4 (7.14) | |
Task 3: PR | n=214 | n=56 | |
Age (years) | 49.61±11.43 | 51.16±13.86 | 0.57 |
Size (mm) | 20.86±12.69 | 19.61±12.16 | 0.47 |
Necrosis | 0.32 | ||
Yes | 43 (20.09) | 11 (19.64) | |
No | 113 (52.80) | 26 (46.43) | |
Missing | 58 (27.10) | 19 (33.93) | |
Ki-67 | 0.95 | ||
Negative | 108 (50.47) | 27 (48.21) | |
Positive | 84 (39.25) | 25 (44.64) | |
Missing | 22 (10.28) | 4 (7.14) | |
Task 4: HER2 | n=212 | n=55 | |
Age (years) | 49.88±12.36 | 50.35±11.17 | 0.54 |
Size (mm) | 20.96±13.05 | 19.47±10.69 | 0.63 |
Necrosis | 0.25 | ||
Yes | 42 (19.81) | 12 (21.82) | |
No | 109 (51.42) | 32 (58.18) | |
Missing | 61 (28.77) | 11 (20.00) | |
Ki-67 | 0.16 | ||
Negative | 101 (47.64) | 32 (58.18) | |
Positive | 90 (42.45) | 19 (34.55) | |
Missing | 21 (9.91) | 4 (7.27) |
Data are presented as the mean ± standard deviation or number (percentage). ER, estrogen receptor; PR, progesterone receptor; HER2, human epidermal growth factor receptor 2.
The average age of patients, average size of lesions, presence of necrosis, and Ki-67 expression level in the training and validation sets were not significantly different in each of the four tasks. Table S1 compares the ultrasound features between the training and validation groups. Fat layer infiltrations, duct changes, structural distortions, echogenic foci, ultrasonographic manifestations, background textures, and BI-RADS categories in the training and validation groups were not significantly different.
Comparison between RadImageNet and ImageNet with pretrained models
As ImageNet has shown great transfer learning performance in medical classification tasks (39), we further compared the performance between RadImageNet and ImageNet to examine whether RadImageNet can achieve considerable results in medical imaging tasks. The results shown in Table 2 indicated that overall, RadImageNet pretrained models performed slightly better than ImageNet pretrained models (P=0.03).
Table 2
Tasks | Models | RadImageNet | ImageNet | |||||||||
---|---|---|---|---|---|---|---|---|---|---|---|---|
ACC | AUC (95% CI) | Sensitivity | Specificity | F1 | ACC | AUC (95% CI) | Sensitivity | Specificity | F1 | |||
Nuclear grade | ResNet50 | 0.667 | 0.560 (0.469–0.571) | 0.400 | 0.720 | 0.286 | 0.610 | 0.510 (0.486–0.619) | 0.474 | 0.458 | 0.452 | |
InceptionV3 | 0.828 | 0.510 (0.485–0.515) | 0.030 | 0.987 | 0.061 | 0.806 | 0.537 (0.465–0.563) | 0.531 | 0.500 | 0.513 | ||
DenseNet121 | 0.761 | 0.540 (0.474–0.547) | 0.200 | 0.873 | 0.218 | 0.650 | 0.563 (0.450–0.571) | 0.433 | 0.693 | 0.292 | ||
ER | ResNet50 | 0.558 | 0.574 (0.450–0.589) | 0.524 | 0.623 | 0.610 | 0.642 | 0.520 (0.417–0.548) | 0.903 | 0.151 | 0.772 | |
InceptionV3 | 0.532 | 0.480 (0.406–0.527) | 0.651 | 0.302 | 0.647 | 0.577 | 0.579 (0.448–0.586) | 0.573 | 0.585 | 0.641 | ||
DenseNet121 | 0.513 | 0.460 (0.447–0.513) | 0.621 | 0.302 | 0.628 | 0.526 | 0.540 (0.467–0.550) | 0.553 | 0.472 | 0.606 | ||
PR | ResNet50 | 0.610 | 0.570 (0.496–0.587) | 0.920 | 0.220 | 0.730 | 0.526 | 0.493 (0.472–0.537) | 0.744 | 0.242 | 0.640 | |
InceptionV3 | 0.474 | 0.460 (0.433–0.491) | 0.558 | 0.364 | 0.546 | 0.513 | 0.400 (0.386–0.533) | 0.872 | 0.045 | 0.669 | ||
DenseNet121 | 0.493 | 0.460 (0.453–0.521) | 0.698 | 0.227 | 0.609 | 0.552 | 0.530 (0.497–0.553) | 0.697 | 0.364 | 0.638 | ||
HER2 | ResNet50 | 0.649 | 0.583 (0.455–0.584) | 0.396 | 0.330 | 0.422 | 0.541 | 0.450 (0.416–0.566) | 0.563 | 0.530 | 0.442 | |
InceptionV3 | 0.541 | 0.573 (0.495–0.583) | 0.667 | 0.480 | 0.485 | 0.622 | 0.525 (0.489–0.568) | 0.250 | 0.800 | 0.300 | ||
DenseNet121 | 0.642 | 0.530 (0.455–0.535) | 0.208 | 0.850 | 0.274 | 0.669 | 0.560 (0.468–0.566) | 0.250 | 0.870 | 0.329 |
ACC, accuracy; AUC, area under the curve; CI, confidence interval; ER, estrogen receptor; PR, progesterone receptor; HER2, human epidermal growth factor receptor 2.
The diagnostic performance of DLR models
Based on the RadImageNet pretraining model, we performed DLR training. LR, SVM, RF, and XGBoost machine learning models were implemented for the classified models. The results are provided in Table 3, which showed that DLR models had improved on the pretrained DL models (versus Table 2). The best performance in the nuclear grade task was achieved by ResNet50 combined with XGBoost (ACC =0.818, AUC =0.633, sensitivity =0.919, specificity =0.367, and F1 =0.892). The best performance parameters for ER+ (InceptionV3 combined with RF; ACC =0.667, AUC =0.618, sensitivity =0.796, specificity =0.415, and F1 =0.759), PR+ (InceptionV3 combined with XGBoost; ACC =0.696, AUC =0.755, sensitivity =0.755, specificity =0.608, and F1 =0.748), and HER2+ (ResNet50 combined with LR; ACC =0.641, AUC =0.713, sensitivity =0.764, specificity =0.572, and F1 =0.604) were inferior compared to the nuclear grading task mainly based on ACC and F1.
Table 3
Tasks | Methods | DLR models | |||||
---|---|---|---|---|---|---|---|
Classifier | ACC | AUC (95% CI) | Sensitivity | Specificity | F1 | ||
Nuclear grade | ResNet50 | LR | 0.758 | 0.573 (0.521–0.625) | 0.867 | 0.267 | 0.854 |
SVM | 0.685 | 0.568 (0.522–0.670) | 0.763 | 0.333 | 0.798 | ||
RF | 0.673 | 0.596 (0.573–0.729) | 0.704 | 0.533 | 0.779 | ||
XGBoost | 0.818 | 0.633 (0.576–0.749) | 0.919 | 0.367 | 0.892 | ||
InceptionV3 | LR | 0.806 | 0.509 (0.472–0.647) | 0.947 | 0.100 | 0.890 | |
SVM | 0.818 | 0.524 (0.380–0.624) | 1 | 0.030 | 0.903 | ||
RF | 0.812 | 0.562 (0.499–0.689) | 0.948 | 0.267 | 0.898 | ||
XGBoost | 0.655 | 0.544 (0.511–0.658) | 0.696 | 0.433 | 0.764 | ||
DenseNet121 | LR | 0.711 | 0.535 (0.416–0.661) | 0.787 | 0.333 | 0.819 | |
SVM | 0.717 | 0.501 (0.438–0.616) | 0.860 | 0.267 | 0.857 | ||
RF | 0.778 | 0.562 (0.434–0.676) | 0.887 | 0.233 | 0.869 | ||
XGBoost | 0.721 | 0.553 (0.463–0.716) | 0.830 | 0.333 | 0.839 | ||
ER | ResNet50 | LR | 0.647 | 0.592 (0.536–0.689) | 0.806 | 0.340 | 0.751 |
SVM | 0.680 | 0.531 (0.514–0.606) | 0.990 | 0.075 | 0.803 | ||
RF | 0.692 | 0.588 (0.569–0.624) | 0.874 | 0.340 | 0.790 | ||
XGBoost | 0.660 | 0.601 (0.51–0.668) | 0.835 | 0.415 | 0.782 | ||
InceptionV3 | LR | 0.679 | 0.584 (0.552–0.626) | 0.913 | 0.226 | 0.790 | |
SVM | 0.660 | 0.515 (0.453–0.650) | 0.864 | 0.264 | 0.771 | ||
RF | 0.667 | 0.618 (0.592–0.647) | 0.796 | 0.415 | 0.759 | ||
XGBoost | 0.654 | 0.528 (0.495–0.581) | 0.806 | 0.359 | 0.755 | ||
DenseNet121 | LR | 0.667 | 0.514 (0.438–0.595) | 0.893 | 0.226 | 0.780 | |
SVM | 0.660 | 0.606 (0.546–0.692) | 0.669 | 0.642 | 0.723 | ||
RF | 0.660 | 0.541 (0.468–0.634) | 0.815 | 0.358 | 0.760 | ||
XGBoost | 0.641 | 0.570 (0.517–0.675) | 0.738 | 0.453 | 0.731 | ||
PR | ResNet50 | LR | 0.592 | 0.553 (0.437–0.628) | 0.848 | 0.257 | 0.702 |
SVM | 0.629 | 0.509 (0.446–0.664) | 0.908 | 0.143 | 0.757 | ||
RF | 0.636 | 0.576 (0.524–0.652) | 0.704 | 0.518 | 0.711 | ||
XGBoost | 0.662 | 0.675 (0.582–0.779) | 0.694 | 0.554 | 0.712 | ||
InceptionV3 | LR | 0.632 | 0.542 (0.497–0.640) | 0.837 | 0.364 | 0.720 | |
SVM | 0.711 | 0.727 (0.673–0.761) | 0.982 | 0.311 | 0.803 | ||
RF | 0.685 | 0.696 (0.641–0.763) | 0.800 | 0.516 | 0.752 | ||
XGBoost | 0.696 | 0.755 (0.707–0.806) | 0.755 | 0.608 | 0.748 | ||
DenseNet121 | LR | 0.566 | 0.504 (0.451–0.553) | 0.767 | 0.303 | 0.667 | |
SVM | 0.610 | 0.603 (0.581–0.636) | 0.735 | 0.393 | 0.706 | ||
RF | 0.623 | 0.601 (0.535–0.629) | 0.674 | 0.554 | 0.698 | ||
XGBoost | 0.649 | 0.592 (0.517–0.724) | 0.704 | 0.554 | 0.718 | ||
HER2 | ResNet50 | LR | 0.641 | 0.713 (0.656–0.774) | 0.764 | 0.572 | 0.604 |
SVM | 0.588 | 0.628 (0.581–0.722) | 0.646 | 0.560 | 0.504 | ||
RF | 0.634 | 0.626 (0.564–0.698) | 0.600 | 0.653 | 0.541 | ||
XGBoost | 0.635 | 0.597 (0.572–0.645) | 0.563 | 0.670 | 0.500 | ||
InceptionV3 | LR | 0.608 | 0.506 (0.408–0.552) | 0.309 | 0.776 | 0.362 | |
SVM | 0.614 | 0.5352 (0.415–0.543) | 0.182 | 0.857 | 0.253 | ||
RF | 0.680 | 0.582 (0.505–0.631) | 0.400 | 0.837 | 0.473 | ||
XGBoost | 0.640 | 0.547 (0.489–0.616) | 0.291 | 0.837 | 0.368 | ||
DenseNet121 | LR | 0.673 | 0.581 (0.568–0.606) | 0.182 | 0.948 | 0.285 | |
SVM | 0.607 | 0.568 (0.492–0.619) | 0.527 | 0.653 | 0.492 | ||
RF | 0.634 | 0.634 (0.593–0.701) | 0.673 | 0.612 | 0.569 | ||
XGBoost | 0.615 | 0.539 (0.493–0.639) | 0.542 | 0.650 | 0.477 |
DLR, deep learning radiomics; ER, estrogen receptor; PR, progesterone receptor; HER2, human epidermal growth factor receptor 2; ACC, accuracy; AUC, area under the curve; CI, confidence interval; LR, logistic regression; SVM, support vector machine; RF, random forest; XGBoost, eXtreme Gradient Boosting.
The diagnostic performance of CML models
Table 4 shows the performances of CML models in the four different identification tasks. In the classification of low nuclear grade DCIS and HER2+, the RF modeling was the best of the four CML models. The ACC, AUC, sensitivity, specificity, and F1 values for the low nuclear grade DCIS were 0.786, 0.719, 0.872, 0.333, and 0.872, respectively; the corresponding values for HER2+ task were 0.764, 0.723, 0.400, 0.900 and 0.480, respectively.
Table 4
Tasks | Models | ACC | AUC (95% CI) | Sensitivity | Specificity | F1 |
---|---|---|---|---|---|---|
Nuclear grade | LR | 0.714 | 0.679 (0.637–0.708) | 0.766 | 0.444 | 0.818 |
SVM | 0.643 | 0.674 (0.637–0.729) | 0.617 | 0.778 | 0.744 | |
RF | 0.786 | 0.719 (0.704–0.785) | 0.872 | 0.333 | 0.872 | |
XGBoost | 0.714 | 0.684 (0.575–0.764) | 0.745 | 0.556 | 0.814 | |
ER | LR | 0.673 | 0.683 (0.626–0.719) | 0.914 | 0.250 | 0.781 |
SVM | 0.727 | 0.751 (0.225–0.758) | 0.857 | 0.500 | 0.799 | |
RF | 0.746 | 0.701 (0.610–0.836) | 0.800 | 0.650 | 0.800 | |
XGBoost | 0.710 | 0.761 (0.684–0.803) | 0.743 | 0.650 | 0.765 | |
PR | LR | 0.691 | 0.668 (0.547–0.753) | 0.719 | 0.652 | 0.730 |
SVM | 0.691 | 0.718 (0.501–0.783) | 0.781 | 0.565 | 0.746 | |
RF | 0.727 | 0.658 (0.605–0.775) | 0.781 | 0.652 | 0.769 | |
XGBoost | 0.709 | 0.780 (0.733–0.859) | 0.813 | 0.565 | 0.765 | |
HER2 | LR | 0.527 | 0.565 (0.360–0.579) | 0.467 | 0.550 | 0.350 |
SVM | 0.800 | 0.615 (0.348–0.669) | 0.267 | 1 | 0.421 | |
RF | 0.764 | 0.723 (0.694–0.796) | 0.400 | 0.900 | 0.480 | |
XGBoost | 0.727 | 0.685 (0.610–0.758) | 0.533 | 0.800 | 0.516 |
CML, clinical machine learning; ER, estrogen receptor; PR, progesterone receptor; HER2, human epidermal growth factor receptor 2; ACC, accuracy; AUC, area under the curve; CI, confidence interval; LR, logistic regression; SVM, support vector machine; RF, random forest; XGBoost, eXtreme Gradient Boosting.
In the ER+ and PR+ classification tasks, the XGBoost modeling had the best performance. The best ACC, AUC, sensitivity, specificity, and F1 values for the ER+ task were 0.710, 0.761, 0.743, 0.650, and 0.765, respectively; the correspond values for the PR+ task were 0.709, 0.780, 0.813, 0.565, and 0.765, respectively.
Figure 2 shows the quantitative contribution of age and various ultrasonographic characteristics to the CML model with the highest AUC value. Age, echogenic foci, BI-RADS classification, and fat layer infiltration had diagnostic value with more advantages in all four tasks.
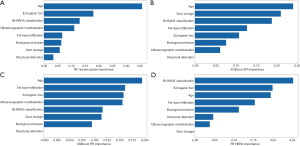
Comparison of the CML and DLR models
Figure 3 shows the AUC values of the best performing CML and DLR models in the validation sets of four classification tasks. The circle represents the cutoff values for well performing DLR and CML models. For recognizing low nuclear grade and ER+ DCIS, the CML models had significantly better performance than DLR models (P=0.01). However, for PR+ and HER2+ diagnosis, CML models had the same level of performance as the DLR model, with no significant difference (P=0.12).
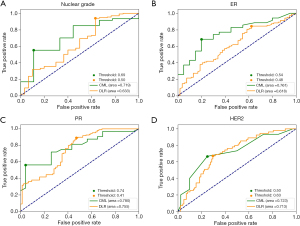
Discussion
In this study, we explored several advanced ultrasound AI methods to predict the presence of low nuclear grade, ER+, PR+, or HER2+ in pure DCIS. We developed, evaluated, and compared the diagnostic performance of the DLR and CML models. We found that CML models had better performances than DLR models in the four DCIS classification tasks. The optimal AI models predicted low nuclear grade, ER+, PR+, and HER2+ with AUC values of 0.719, 0.761, 0.780, and 0.723, respectively.
Currently, few studies have used RadImageNet as the basis for a pretrained DL network (47,51,52). Liu et al. (51) used pretrained models derived from the RadImageNet to measure leg length on radiographs, and Kihira et al. (52) developed a DL-based framework on RadImageNet for the automatic segmentation and characterization of gliomas. To the best of our knowledge, the current study is the first to investigate DLR based on ultrasound images to predict the nuclear grade and common clinical biomarkers in DCIS. We adopted three pretrained models to implement the classification tasks. Furthermore, we compared the performance of transfer learning between RadImageNet and ImageNet. In the best prediction of PR and HER2 tasks, the AUC values of ResNet50 model of RadImageNet were slightly higher than those of DenseNet121 model of ImageNet. Generally speaking, moving from ImageNet to RadImageNet can improve the transfer learning performance and generalizability. Due to the problem of sample data in this study, this difference was not very distinct, and should be further explored in subsequent large-scale data studies.
DCIS (clinical stage 0 cancer) is negatively correlated with the incidence rate of invasive interval cancer (53). Histologic grading of DCIS in the 8th edition of the American Joint Committee on Cancer (AJCC) guidelines refers to nuclear grade and also incorporates hormone receptor-related prognostic information, which provides more information on the treatment of patients with DCIS (54). These AI models have the potential to screen potential low-grade patients for imaging supervision, avoiding unnecessary surgical resection. Some of the better-known clinical trials with proactive monitoring include the COMET, LORD, and LORIS trials, all of which, despite having different study endpoints, include risk stratification of patients (55-57). The COMET trial required positive ER or PR biomarkers for inclusion and excluded triple-positive patients if usable HER2 results were available (55). The LORD trial included only patients with low-grade histology and had good concordance between vacuum-assisted core biopsy, pathology, and imaging results (56). The AI models developed above may help screen ER, PR, or HER2 positive patients for further risk stratification.
In the examined AI models, CML models performed best in all four tasks. This provides a reference for modeling some tasks. In this study, our models evaluated the importance of each feature in the four prediction tasks. Our model showed that age was the most important factor in identifying the nuclear grade and ER status of patients with DCIS, which has some similarities with a previous study (14). That study showed a statistically significant difference in the average age of high nuclear grade and non-high nuclear grade patients. In this study, the contribution of echogenic foci to nuclear grade prediction was second to age, which demonstrates the importance of this ultrasound feature in predicting nuclear grade as well. Our data also demonstrated the usefulness of the BI-RADS classification in identifying low nuclear grade and ER+ DCIS, which to our knowledge has not been studied yet. When the RF model identified HER2+ lesions, the most relevant factors were BI-RADS classification, echogenic foci, and age. The other two ultrasound features of BI-RADS classification and age have not been evaluated in previous study (15).
Building CML models based on the meaningful image characteristics, which stem from clinical practice experience, can reflect the weight of the importance of feature variables (26,27), thereby supplementing DL “black boxes”. CML models may be close to decision-making processes in clinical practice. Especially, the research of Bahl et al. (58) has proved that using machine learning models can reduce unnecessary operations in nearly one-third of patients with high-risk breast lesions.
A critical evaluation of our data suggests that the AI models did not achieve the desired effect. Possible reasons may be summarized as follows: first, our study data contained a wide variety of ultrasound manifestations of DCIS lesions because we believe that this can provide a broader range of effective AI models. Using radiomics methods, Wu et al. (59) identified molecular markers of DCIS, but they did not study non-mass DCIS lesions. However, as far as we know, most DCIS lesions present as non- mass structures. Second, in terms of the disease itself, non-mass DCIS lesions have various structural patterns on ultrasound, have no clear boundaries, and have been described using various methods (14-17,60,61). For example, some lesions only show echogenic foci or duct dilations (60,61). We used the DL method because it can reduce the deviation caused by manual feature extraction based on tumor heterogeneity, but it seems that clinical experience is more reliable. In addition to ultrasound, other medical imaging modes that have been dedicated to studying the risk levels of DCIS also showed usefulness for clinical application (23,62). Third, our experimental task was to recognize nuclear grade and molecular marker information based on a single imaging pattern, which is inherently challenging. The combination of DLR and pathology data will enable a deeper exploration of image information (63).
Our study also has several limitations. First, all ultrasound images used in this study were in JPEG format leading to a certain loss of image quality, which will decrease the accuracy of the model to some extent. Second, we have collected cases of DCIS confirmed after surgery in our hospital over the past 16 years. However, due to the single tumor type, the available ultrasound data is limited, and future multicenter population cohort studies are needed. Due to the lower proportion of postoperative low-grade DCIS patients, this may result in imbalanced datasets. Developing unified standards for data from different institutions and hospitals can form a more comprehensive and standardized training set. In the future, more precise layering is needed to study images from different ultrasound equipment. Although our study had a higher performance for the clinical model, imbalanced experimental data for each task will limit the applicability of our model, especially for low-grade patients. Third, some data were missing, so the sample may be subject to selection bias. Fourth, in our institution, for equivocal cases with a HER2 score of 2+, fluorescence in situ hybridization double-staining probes were used for clarification, but gene amplification results were not always available. Thus, our HER2 detection may have resulted in selection bias. Finally, the CML models did not include elastography and contrast-enhanced ultrasound.
Conclusions
In conclusion, the ultrasound DLR and CML models may be able to identify nuclear grade, ER+, PR+, and HER2+ lesions in patients with pure DCIS. This information can assist clinicians in the risk stratification of patients, thereby providing a basis for follow-up personalized treatment plans. In the future, the models can be further optimized through larger datasets or external validation.
Acknowledgments
Funding: None.
Footnote
Reporting Checklist: The authors have completed the TRIPOD reporting checklist. Available at https://gs.amegroups.com/article/view/10.21037/gs-23-417/rc
Data Sharing Statement: Available at https://gs.amegroups.com/article/view/10.21037/gs-23-417/dss
Peer Review File: Available at https://gs.amegroups.com/article/view/10.21037/gs-23-417/prf
Conflicts of Interest: All authors have completed the ICMJE uniform disclosure form (available at https://gs.amegroups.com/article/view/10.21037/gs-23-417/coif). The authors have no conflicts of interest to declare.
Ethical Statement: The authors are accountable for all aspects of the work in ensuring that questions related to the accuracy or integrity of any part of the work are appropriately investigated and resolved. The study was conducted in accordance with the Declaration of Helsinki (as revised in 2013). The study was approved by the Ethics Committee of West China Hospital of Sichuan University (No. 2022-1612) and individual consent for this retrospective analysis was waived.
Open Access Statement: This is an Open Access article distributed in accordance with the Creative Commons Attribution-NonCommercial-NoDerivs 4.0 International License (CC BY-NC-ND 4.0), which permits the non-commercial replication and distribution of the article with the strict proviso that no changes or edits are made and the original work is properly cited (including links to both the formal publication through the relevant DOI and the license). See: https://creativecommons.org/licenses/by-nc-nd/4.0/.
References
- Giaquinto AN, Sung H, Miller KD, et al. Breast Cancer Statistics, 2022. CA Cancer J Clin 2022;72:524-41. [Crossref] [PubMed]
- Grimm LJ, Ryser MD, Partridge AH, et al. Surgical Upstaging Rates for Vacuum Assisted Biopsy Proven DCIS: Implications for Active Surveillance Trials. Ann Surg Oncol 2017;24:3534-40. [Crossref] [PubMed]
- Grimm LJ, Ghate SV, Hwang ES, et al. Imaging Features of Patients Undergoing Active Surveillance for Ductal Carcinoma in Situ. Acad Radiol 2017;24:1364-71. [Crossref] [PubMed]
- Oseni TO, Smith BL, Lehman CD, et al. Do Eligibility Criteria for Ductal Carcinoma In Situ (DCIS) Active Surveillance Trials Identify Patients at Low Risk for Upgrade to Invasive Carcinoma? Ann Surg Oncol 2020;27:4459-65. [Crossref] [PubMed]
- Ponti A, Ronco G, Lynge E, et al. Low-grade screen-detected ductal carcinoma in situ progresses more slowly than high-grade lesions: evidence from an international multi-centre study. Breast Cancer Res Treat 2019;177:761-5. [Crossref] [PubMed]
- Fuentes-Sánchez C, González-San Segundo C. Can we avoid treatment in patients with low-risk ductal carcinoma in situ? Ann Breast Surg 2023;7:33. [Crossref]
- Bonev VV. Ductal carcinoma in situ: a comprehensive review on current and future management for the surgeon and non-surgeon. AME Surg J 2021;1:27. [Crossref]
- Sagara Y, Mallory MA, Wong S, et al. Survival Benefit of Breast Surgery for Low-Grade Ductal Carcinoma In Situ: A Population-Based Cohort Study. JAMA Surg 2015;150:739-45. [Crossref] [PubMed]
- Su X, Lin Q, Cui C, et al. Non-calcified ductal carcinoma in situ of the breast: comparison of diagnostic accuracy of digital breast tomosynthesis, digital mammography, and ultrasonography. Breast Cancer 2017;24:562-70. [Crossref] [PubMed]
- Lee SE, Kim HY, Yoon JH, et al. Chronological Trends of Breast Ductal Carcinoma In Situ: Clinical, Radiologic, and Pathologic Perspectives. Ann Surg Oncol 2021;28:8699-709. [Crossref] [PubMed]
- Weigel S, Khil L, Hense HW, et al. Detection Rates of Ductal Carcinoma in Situ with Biennial Digital Mammography Screening: Radiologic Findings Support Pathologic Model of Tumor Progression. Radiology 2018;286:424-32. [Crossref] [PubMed]
- Moon HJ, Kim EK, Kim MJ, et al. Comparison of Clinical and Pathologic Characteristics of Ductal Carcinoma in Situ Detected on Mammography versus Ultrasound Only in Asymptomatic Patients. Ultrasound Med Biol 2019;45:68-77. [Crossref] [PubMed]
- Choi SH, Choi JS, Han BK, et al. Long-term Surveillance of Ductal Carcinoma in Situ Detected with Screening Mammography versus US: Factors Associated with Second Breast Cancer. Radiology 2019;292:37-48. [Crossref] [PubMed]
- Scoggins ME, Fox PS, Kuerer HM, et al. Correlation between sonographic findings and clinicopathologic and biologic features of pure ductal carcinoma in situ in 691 patients. AJR Am J Roentgenol 2015;204:878-88. [Crossref] [PubMed]
- Cha H, Chang YW, Lee EJ, et al. Ultrasonographic features of pure ductal carcinoma in situ of the breast: correlations with pathologic features and biological markers. Ultrasonography 2018;37:307-14. [Crossref] [PubMed]
- Gunawardena DS, Burrows S, Taylor DB. Non-mass versus mass-like ultrasound patterns in ductal carcinoma in situ: is there an association with high-risk histology? Clin Radiol 2020;75:140-7. [Crossref] [PubMed]
- Park JS, Park YM, Kim EK, et al. Sonographic findings of high-grade and non-high-grade ductal carcinoma in situ of the breast. J Ultrasound Med 2010;29:1687-97. [Crossref] [PubMed]
- Allison KH, Hammond MEH, Dowsett M, et al. Estrogen and Progesterone Receptor Testing in Breast Cancer: ASCO/CAP Guideline Update. J Clin Oncol 2020;38:1346-66. [Crossref] [PubMed]
- Esserman L, Yau C. Rethinking the Standard for Ductal Carcinoma In Situ Treatment. JAMA Oncol 2015;1:881-3. [Crossref] [PubMed]
- Allred DC, Anderson SJ, Paik S, et al. Adjuvant tamoxifen reduces subsequent breast cancer in women with estrogen receptor-positive ductal carcinoma in situ: a study based on NSABP protocol B-24. J Clin Oncol 2012;30:1268-73. [Crossref] [PubMed]
- Thorat MA, Levey PM, Jones JL, et al. Prognostic and Predictive Value of HER2 Expression in Ductal Carcinoma In Situ: Results from the UK/ANZ DCIS Randomized Trial. Clin Cancer Res 2021;27:5317-24. [Crossref] [PubMed]
- Brennan ME, Turner RM, Ciatto S, et al. Ductal carcinoma in situ at core-needle biopsy: meta-analysis of underestimation and predictors of invasive breast cancer. Radiology 2011;260:119-28. [Crossref] [PubMed]
- Lee SE, Kim GR, Han K, et al. US, Mammography, and Histopathologic Evaluation to Identify Low Nuclear Grade Ductal Carcinoma in Situ. Radiology 2022;303:276-84. [Crossref] [PubMed]
- Bitencourt AGV, Gibbs P, Rossi Saccarelli C, et al. MRI-based machine learning radiomics can predict HER2 expression level and pathologic response after neoadjuvant therapy in HER2 overexpressing breast cancer. EBioMedicine 2020;61:103042. [Crossref] [PubMed]
- Song SE, Cho KR, Cho Y, et al. Machine learning with multiparametric breast MRI for prediction of Ki-67 and histologic grade in early-stage luminal breast cancer. Eur Radiol 2022;32:853-63. [Crossref] [PubMed]
- Wu M, Zhong X, Peng Q, et al. Prediction of molecular subtypes of breast cancer using BI-RADS features based on a "white box" machine learning approach in a multi-modal imaging setting. Eur J Radiol 2019;114:175-84. [Crossref] [PubMed]
- Ma M, Liu R, Wen C, et al. Predicting the molecular subtype of breast cancer and identifying interpretable imaging features using machine learning algorithms. Eur Radiol 2022;32:1652-62. [Crossref] [PubMed]
- Avanzo M, Wei L, Stancanello J, et al. Machine and deep learning methods for radiomics. Med Phys 2020;47:e185-202. [Crossref] [PubMed]
- Zheng X, Yao Z, Huang Y, et al. Deep learning radiomics can predict axillary lymph node status in early-stage breast cancer. Nat Commun 2020;11:1236. [Crossref] [PubMed]
- Arefan D, Chai R, Sun M, et al. Machine learning prediction of axillary lymph node metastasis in breast cancer: 2D versus 3D radiomic features. Med Phys 2020;47:6334-42. [Crossref] [PubMed]
- O'Keefe TJ, Harismendy O, Wallace AM. Histopathological growth distribution of ductal carcinoma in situ: tumor size is not "one size fits all". Gland Surg 2022;11:307-18. [Crossref] [PubMed]
- Wei J, Cheng J, Gu D, et al. Deep learning-based radiomics predicts response to chemotherapy in colorectal liver metastases. Med Phys 2021;48:513-22. [Crossref] [PubMed]
- Wang K, Lu X, Zhou H, et al. Deep learning Radiomics of shear wave elastography significantly improved diagnostic performance for assessing liver fibrosis in chronic hepatitis B: a prospective multicentre study. Gut 2019;68:729-41. [Crossref] [PubMed]
- Zhu M, Pi Y, Jiang Z, et al. Application of deep learning to identify ductal carcinoma in situ and microinvasion of the breast using ultrasound imaging. Quant Imaging Med Surg 2022;12:4633-46. [Crossref] [PubMed]
- Mendelson EB, Böhm-Vélez M, Berg WA, et al. ACR BI-RADS ultrasound. In: ACR BI-RADS Atlas, Breast Imaging Reporting and Data System, 5th Edition, American College of Radiology, Reston, VA; 2013:128-30.
- Selvi R. Breast Diseases: Imaging and Clinical Management. New Delhi: Springer; 2015:135-8.
- Hammond ME, Hayes DF, Dowsett M, et al. American Society of Clinical Oncology/College of American Pathologists guideline recommendations for immunohistochemical testing of estrogen and progesterone receptors in breast cancer. Arch Pathol Lab Med 2010;134:907-22. [Crossref] [PubMed]
- Wolff AC, Hammond ME, Schwartz JN, et al. American Society of Clinical Oncology/College of American Pathologists guideline recommendations for human epidermal growth factor receptor 2 testing in breast cancer. J Clin Oncol 2007;25:118-45. [Crossref] [PubMed]
- Wolff AC, Hammond ME, Hicks DG, et al. Recommendations for human epidermal growth factor receptor 2 testing in breast cancer: American Society of Clinical Oncology/College of American Pathologists clinical practice guideline update. J Clin Oncol 2013;31:3997-4013. [Crossref] [PubMed]
- Wolff AC, Hammond MEH, Allison KH, et al. Human Epidermal Growth Factor Receptor 2 Testing in Breast Cancer: American Society of Clinical Oncology/College of American Pathologists Clinical Practice Guideline Focused Update. J Clin Oncol 2018;36:2105-22. [Crossref] [PubMed]
- Williams KE, Barnes NLP, Cramer A, et al. Molecular phenotypes of DCIS predict overall and invasive recurrence. Ann Oncol 2015;26:1019-25. [Crossref] [PubMed]
- He K, Zhang X, Ren S, et al. Deep residual learning for image recognition. In: Conference on Computer Vision and Pattern Recognition (CVPR) 2016:770-8.
- Szegedy C, Liu W, Jia Y, et al. Going deeper with convolutions. In: IEEE Conference on Computer Vision and Pattern Recognition (CVPR) 2015;2015:1-9.
- Huang G, Liu Z, Pleiss G, et al. Convolutional Networks with Dense Connectivity. IEEE Trans Pattern Anal Mach Intell 2022;44:8704-16. [Crossref] [PubMed]
- Shin HC, Roth HR, Gao M, et al. Deep Convolutional Neural Networks for Computer-Aided Detection: CNN Architectures, Dataset Characteristics and Transfer Learning. IEEE Trans Med Imaging 2016;35:1285-98. [Crossref] [PubMed]
- Kermany DS, Goldbaum M, Cai W, et al. Identifying Medical Diagnoses and Treatable Diseases by Image-Based Deep Learning. Cell 2018;172:1122-1131.e9. [Crossref] [PubMed]
- Mei X, Liu Z, Robson PM, et al. RadImageNet: An Open Radiologic Deep Learning Research Dataset for Effective Transfer Learning. Radiol Artif Intell 2022;4:e210315. [Crossref] [PubMed]
- Anwar SM, Majid M, Qayyum A, et al. Medical Image Analysis using Convolutional Neural Networks: A Review. J Med Syst 2018;42:226. [Crossref] [PubMed]
- Tajbakhsh N, Shin JY, Gurudu SR, et al. Convolutional Neural Networks for Medical Image Analysis: Full Training or Fine Tuning? IEEE Trans Med Imaging 2016;35:1299-312. [Crossref] [PubMed]
- Gulli A, Pal S. Deep learning with Keras. Packt Publishing Ltd.; 2017.
- Liu Z, Deyer L, Yang A, et al. Automated machine learning-based radiomics analysis versus deep learning-based classification for thyroid nodule on ultrasound images: A multi-center study. 22nd International Conference on Bioinformatics and Bioengineering (BIBE) 2022;2022:23-8.
- Kihira S, Mei X, Mahmoudi K, et al. U-Net Based Segmentation and Characterization of Gliomas. Cancers (Basel) 2022;14:4457. [Crossref] [PubMed]
- Duffy SW, Dibden A, Michalopoulos D, et al. Screen detection of ductal carcinoma in situ and subsequent incidence of invasive interval breast cancers: a retrospective population-based study. Lancet Oncol 2016;17:109-14. [Crossref] [PubMed]
- Amin MB, Greene FL, Edge SB, et al. The Eighth Edition AJCC Cancer Staging Manual: Continuing to build a bridge from a population-based to a more "personalized" approach to cancer staging. CA Cancer J Clin 2017;67:93-9.
- Hwang ES, Hyslop T, Lynch T, et al. The COMET (Comparison of Operative versus Monitoring and Endocrine Therapy) trial: a phase III randomised controlled clinical trial for low-risk ductal carcinoma in situ (DCIS). BMJ Open 2019;9:e026797. [Crossref] [PubMed]
- Elshof LE, Tryfonidis K, Slaets L, et al. Feasibility of a prospective, randomised, open-label, international multicentre, phase III, non-inferiority trial to assess the safety of active surveillance for low risk ductal carcinoma in situ - The LORD study. Eur J Cancer 2015;51:1497-510. [Crossref] [PubMed]
- Francis A, Thomas J, Fallowfield L, et al. Addressing overtreatment of screen detected DCIS; the LORIS trial. Eur J Cancer 2015;51:2296-303. [Crossref] [PubMed]
- Bahl M, Barzilay R, Yedidia AB, et al. High-Risk Breast Lesions: A Machine Learning Model to Predict Pathologic Upgrade and Reduce Unnecessary Surgical Excision. Radiology 2018;286:810-8. [Crossref] [PubMed]
- Wu L, Zhao Y, Lin P, et al. Preoperative ultrasound radiomics analysis for expression of multiple molecular biomarkers in mass type of breast ductal carcinoma in situ. BMC Med Imaging 2021;21:84. [Crossref] [PubMed]
- Li JK, Wang HF, He Y, et al. Ultrasonographic features of ductal carcinoma in situ: analysis of 219 lesions. Gland Surg 2020;9:1945-54. [Crossref] [PubMed]
- Watanabe T, Yamaguchi T, Tsunoda H, et al. Ultrasound Image Classification of Ductal Carcinoma In Situ (DCIS) of the Breast: Analysis of 705 DCIS Lesions. Ultrasound Med Biol 2017;43:918-25. [Crossref] [PubMed]
- Iima M, Le Bihan D, Okumura R, et al. Apparent diffusion coefficient as an MR imaging biomarker of low-risk ductal carcinoma in situ: a pilot study. Radiology 2011;260:364-72. [Crossref] [PubMed]
- Zhu J, Liu M, Li X. Progress on deep learning in digital pathology of breast cancer: a narrative review. Gland Surg 2022;11:751-66. [Crossref] [PubMed]