CCNB1 may as a biomarker for the adipogenic differentiation of adipose-derived stem cells in the postoperative fat transplantation of breast cancer
Highlight box
Key findings
• The results of this study indicate that CCNB1 gene downregulation is pronounced in the early stage of adipogenic differentiation.
What is known and what is new?
• Adipose-derived stem cell differentiation includes distinct gene regulation.
• In our study, a combination of bioinformatics analysis and experimental validation was used to explore how gene behavior varies over time during adipogenic differentiation.
What is the implication, and what should change now?
• Our results suggest that the CCNB1 gene can be used as potential biomarker for reflecting adipogenic differentiation and may have clinical value and feasibility in evaluating the postoperative fat transplantation of breast cancer. Moreover, CCNB1 gene may be useful in future work involving the clinical treatment of patients of breast cancer. Moreover, bioinformatics analysis combined with experimental validation has greater accuracy in identifying important genes related to adipogenic differentiation.
Introduction
Breast disease is one of the most common illnesses in women and includes both benign lesions and malignant tumors (1,2). Although surgery is the primary treatment mode for breast cancer, millions of women experience breast defects after surgery, and thus one urgent issue is adequate breast reconstruction to improve the quality of life of women diagnosed with breast cancer (3). With the development of medical technology, autologous fat grafting has gradually become the main surgical option for treating breast defects after breast cancer surgery (4). The rate of breast reconstruction among women varies across different countries but has continued to rise (5,6). Although autologous fat grafting is a common means of treating of breast defects after breast cancer surgery; however, this method involves a few drawbacks, such as the unknown chance of tumor recurrence, the damage to the donor site, and an unsatisfactory survival rate of fat grafting, which greatly reduces the patient’s postoperative satisfaction and quality of life (7-10).
Breast tissue engineering after breast cancer surgery is applied to help alleviate the shortcomings of natural breast transplants by developing substitutes (11,12). In addition to the ability to form on demand, the tissue should be simple to use and prepare. For the past few years, considerable progress has been made in the study of human adipose-derived stem cells (ADSCs) (13), and many studies have shown that ADSCs have the ability to differentiate into adipocytes, contributing to fat tissue formation. And the expression of specific biomarkers like adiponectin and perilipin is used to confirm successful ADSCs adipogenic differentiation (14,15). Due to the properties of adipogenesis, ADSCs have significant value in clinical application in breast tissue engineering after breast cancer surgery, being practicable for the reconstruction of the breast with autologous cells. The transplantation of human ADSCs has thus attracted much attention as a promising therapeutic approach in recent years (16-20). Previous studies have shown that many transcription factors and cytokines regulate adipogenic differentiation of ADSCs, such as the CDK5/FOXC2, ERK1/2-PPARγ, and MAPK signaling pathways (21-26). Despite ADSCs becoming a research hotspot in breast tissue engineering after breast cancer surgery due to the abundance of their supply and high availability, there is still some uncertainty regarding the basic biological mechanism behind the adipogenic differentiation of ADSCs, whether there may be additional transcriptional regulators and epigenetic factors that influence ADSCs differentiation that are not yet fully understood (27-29). The impact of the microenvironment on ADSCs adipogenic differentiation, including the role of neighboring cells and extracellular matrix (ECM) components, requires further exploration. At last, ADSCs are a heterogeneous population, and understanding the factors that influence the variability in their adipogenic potential is an area that warrants more research (30).
With the rapid development of microarray chromatin immunoprecipitation (ChIP) and sequencing technology as well as the growing application of ADSCs in the field of clinical medicine, it is necessary to conduct bioinformatics studies related to ADSCs in order to further elucidate the specific mechanism related to the adipogenic differentiation of ADSCs. Bioinformatics analysis enables the integration of diverse datasets from various studies, allowing researchers to combine information on gene expression and signaling pathways. This integration can provide a more holistic view of the molecular mechanisms to understand ADSCs adipogenic differentiation. Furthermore, bioinformatics tools can be used to predict and analyze transcription factor binding sites, enhancers, and other regulatory elements in the genomic regions associated with adipogenic differentiation. Indeed, as the molecular biological mechanisms of adipogenic differentiation are still poorly understood, in this study, bioinformatics methods were used to screen the differentially expressed genes (DEGs) and their involved signaling pathways during the adipogenic differentiation of ADSCs in order to clarify the molecular biological mechanism of potential key genes during the adipogenic differentiation of ADSCs. Initially, we identified the DEGs from one microarray dataset selected from the Gene Expression Omnibus (GEO) database, and then a bioinformatics approach was used to analyze the signaling pathway and to construct a protein-protein interaction (PPI) network for targeting hub genes. Finally, in vitro experiments were conducted to verify the related genes. Ultimately, by mining the data related to the adipogenic differentiation of ADSCs in the GEO database, we identified the potential key genes and the pathways involved in the adipogenic differentiation of ADSCs. Our findings contribute to further exploring the molecular biological mechanism related to the occurrence and development of adipogenic differentiation of ADSCs and to providing a new direction for the future clinical application of breast reconstruction after breast cancer surgery. We present this article in accordance with the MDAR reporting checklist (available at https://gs.amegroups.com/article/view/10.21037/gs-23-493/rc).
Methods
Study design
GEO dataset GSE61302 was downloaded and analyzed to identify DEGs, then relevant signaling pathways were found by Gene Ontology (GO) and Kyoto Encyclopedia of Genes and Genomes (KEGG) analysis. Next, to establish PPIs network, so hub genes were obtained by Cytoscape software. In vitro, preadipocytes were cultured and identified, RNA isolation from differentiated and undifferentiated group. Finally, verification of the messenger RNA (mRNA) expression of hub genes at days 7 and 21.
Data collection
GEO (https://www.ncbi.nlm.nih.gov/geo) is an international, public database for functional genomics that includes high-throughput resources, gene expression data, ChIP sequencing (ChIP-seq), and microarrays. We obtained the transcript profile data on adipogenic differentiation from GSE61302 (31). The GSE61302 dataset contains five undifferentiated samples (GSM1501805, GSM1501806, GSM1501807, GSM1501808, and GSM1501809) and 10 adipogenic differentiated samples (GSM1501795, GSM1501796, GSM1501798, GSM1501799, GSM1501800, GSM1501801, GSM1501802, GSM1501803, GSM1501804, and GSM1501805). Based on the platform annotation information, probes were transformed into corresponding gene symbols.
Identification of DEGs
R software (The R Foundation for Statistical Computing, Vienna, Austria) was applied for data mining and statistical analyses. The “limma” package in R was subsequently used for removing batch effects and identifying DEGs, with those with a log2fold change (log2FC) >1 or <−1 and a P value <0.05 being considered significant.
GO and KEGG enrichment analysis
KEGG is a database resource for providing biological pathway information. GO is also a major bioinformatics database that for high-quality functional gene annotation and analyzing gene biological processes (BPs), molecular functions (MFs), and cellular components (CCs). GO and KEGG enrichment analyses of the identified DEGs were performed using the “clusterProfiler” 4.0 package in R (32-34). R software packages can be used to perform both enrichment analyses of genes and gene clusters and the visualization of gene profiles.
PPI network construction and module analysis
The PPI network was analyzed using the Search Tool for the Retrieval of Interacting Genes/Proteins (STRING; https://string-db.org). An interaction with a combined score >0.4 was chosen and used to construct a PPI network in Cytoscape software (version 3.9.1) (35). Cytoscape is an open-source platform for visualizing networks of molecular interaction (36). Dense connected regions were analyzed using the Cytoscape plug-in molecular complex detection (MCODE). Our selection criteria were as follows: degree cutoff =2, node score cutoff =0.2, max depth =100, and k-score =2 (37).
Selection and analysis of hub genes
The top 10 genes were obtained via the Matthews correlation coefficient (MCC) algorithm with the Cytoscape’s plug-in cytoHubba.
Cell culture and adipogenic differentiation
According to a previously described method, preadipocytes were isolated and cultured from aseptic human adipose tissue. Tissue samples were obtained from the patients with benign breast disease from the Department of Breast Surgery, the First Affiliated Hospital of Nanjing Medical University, between August 2023 and October 2023. This study was conducted in accordance with the Declaration of Helsinki (as revised in 2013) and approved by the Ethics Committee of the First Affiliated Hospital of Nanjing Medical University (protocol code: 2022-NT-79). Informed consent was obtained from all participants involved in the study. Aseptic human adipose tissue was obtained and digested with 1 mg/mL of type I collagenase for 60 minutes, terminated with preadipocyte medium (PAM; ScienCell, Carlsbad, CA, USA), and then centrifuged at 1,500 rpm for 10 minutes. The cells were seeded in a 10-cm dish and incubated in a 37 ℃ incubator with 5% CO2 for 24 hours, after which the medium was replaced. Cells cultured for 2–4 passages were used for seeding a 6-cm dish at a density of 20,000 cells/cm2. The adipogenic differentiation medium (Haixing Biosciences, Suzhou, China) was substituted for the original medium when cell confluence was 80–90%. The medium was changed every 3 days. The adipogenic effects were identified via oil red O (ORO) staining at days 7 and 14.
ORO staining
Lipid droplets were detected with ORO (Sigma-Aldrich, St. Louis, MO, USA) staining. ADSCs were washed with phosphate-buffered saline (PBS) and fixed with 4% paraformaldehyde for 60 minutes at room temperature; this was followed by another wash with PBS, after which the ADSCs were incubated with ORO staining solution for another 30 minutes at room temperature. Images were obtained using Olympus microscope.
RNA isolation and real-time quantitative polymerase chain reaction (RT-qPCR) analysis
Following the manufacturer’s protocol, total RNA was isolated from undifferentiated and differentiated cells with TRIzol LS (Life Technologies, Carlsbad, CA, USA; Thermo Fisher Scientific, Waltham, MA, USA). Complement DNA (cDNA) was prepared with a HiScript III First Strand cDNA Synthesis Kit [+genomic DNA (gDNA) wiper] (Vazyme Biotech, Nanjing, China). Next, RT-qPCR was performed using ChamQ SYBR qPCR Master Mix (Vazyme Biotech). The reactions were conducted in the Roche Light Cycler 480 Real-Time PCR System (Roche Diagnostics), with the 2−ΔΔCt method being used to calculate the expression level of genes in each sample. The primer sequences for RT-qPCR are presented in the supplementary information (Table 1).
Table 1
Gene symbol | Forward primers | Reverse primers |
---|---|---|
GAPDH | GGACCTGACCTGCCGTCTAG | GTAGCCCAGGATGCCCTTGA |
NUSAP1 | AGCCCATCAATAAGGGAGGG | ACCTGACACCCGTTTTAGCTG |
CCNB1 | AATAAGGCGAAGATCAACATGGC | TTTGTTACCAATGTCCCCAAGAG |
DLGAP5 | AAGTGGGTCGTTATAGACCTGA | TGCTCGAACATCACTCTCGTTAT |
TTK | TCATGCCCATTTGGAAGAGTC | CCACTTGGTTTAGATCCAGGC |
CCNB2 | CCGACGGTGTCCAGTGATTT | TGTTGTTTTGGTGGGTTGAACT |
BUB1B | AAATGACCCTCTGGATGTTTGG | GCATAAACGCCCTAATTTAAGCC |
KIF23 | AGTCAGCGAGAGCTAAGACAC | GGTTGAGTCTGTAGCCCTCAG |
CDCA8 | GCAGGAGAGCGGATTTACAAC | CTGGGCAATACTGTGCCTCTG |
CDC20 | GCACAGTTCGCGTTCGAGA | CTGGATTTGCCAGGAGTTCGG |
KIF11 | TCCCTTGGCTGGTATAATTCCA | GTTACGGGGATCATCAAACATCT |
RT-qPCR, real-time quantitative polymerase chain reaction; GAPDH, glyceraldehyde-3-phosphate dehydrogenase.
Statistical analysis
The bioinformatics analyses were conducted using R v.4.1.3, with R packages include “limma”, “dplyr”, “ggplot2”, and “clusterProfiler”, among others. Statistical analysis with the paired Student t-test was performed with GraphPad Prism 9.0 software (GraphPad Software, La Jolla, CA, USA). All data are presented as the mean ± stand error of the mean (SEM). Values of P<0.05 were considered statistically significant. All experiments were repeated three times to ensure the accuracy of the results.
Results
Identification of DEGs
After normalization of the data, the analysis of DEGs was performed in the dataset that included 10 adipogenic differentiated samples and five undifferentiated control samples. A total of 1,091 DEGs were screened according to the criteria of false discovery rate (FDR) <0.05 and log2FC >1 or < −1. Among these genes, 382 genes were upregulated and 709 downregulated, as shown in the volcano plot in Figure 1.
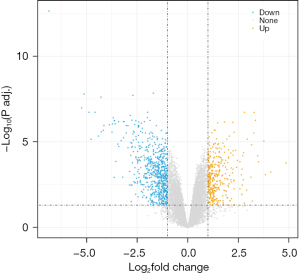
GO and KEGG enrichment analyses of DEGs
In order to analyze the DEGs at the functional level, we performed functional enrichment analysis of the corresponding genes to determine the potential functions. The GO analysis results were mainly associated with leukocyte migration, cell chemotaxis, collagen-containing ECM, and glycosaminoglycan binding (Figure 2A). KEGG pathway analysis indicated that DEG enrichment was mainly related to viral protein interaction with cytokine and cytokine receptor, tumor necrosis factor (TNF) signaling pathway, and cytokine-cytokine receptor interaction (Figure 2B). In addition, the related pathways of upregulated genes in KEGG mainly included viral protein interaction with cytokine and cytokine receptor, TNF signaling pathway, cytokine-cytokine receptor interaction, and peroxisome proliferator-activated receptor (PPAR) signaling pathway (Figure 2C); meanwhile, the pathways associated with downregulated genes mainly included complement and coagulation cascades and C-type lectin receptor signaling pathway (Figure 2D).
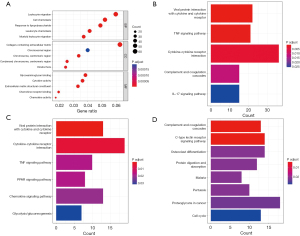
PPI network construction and module analysis
Based on the STRING database, the PPI network of the DEGs was constructed. We used 382 upregulated DEGs and 709 downregulated DEGs to create the PPI network (Figure 3A). We also selected and analyzed the central dense connected regions according to the corresponding criteria, as these central node genes may potentially be relevant to regulating adipogenic differentiation (Figure 3B).
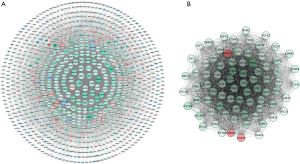
Selection and analysis of hub genes
The Cytoscape plug-in cytoHubba identified 10 hub genes. A centrality analysis of the nodes in the PPI network revealed that NUSAP1, CCNB1, DLGAP5, TTK, CCNB2, KIF23, BUB1B, CDC20, CDCA8, and KIF11 were crucial genes (Figure 4). The gene symbols, abbreviations, and functions are shown in the supplementary information (Table 2).
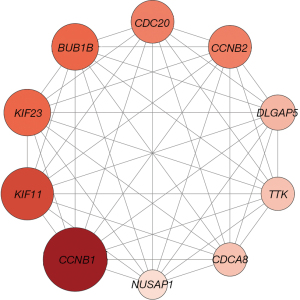
Table 2
Gene symbol | Description | Function |
---|---|---|
NUSAP1 | Nucleolar and spindle associated protein 1 | Microtubule-associated protein with the capacity to bundle and stabilize microtubules (by similarity) |
CCNB1 | Cyclin B1 | Essential for the control of the cell cycle at the G2/M (mitosis) transition |
DLGAP5 | Discs large homolog associated protein 5 | Potential cell cycle regulator that may play a role in carcinogenesis of cancer cells |
TTK | TTK protein kinase | Probably associated with cell proliferation |
CCNB2 | Cyclin B2 | Essential for the control of the cell cycle at the G2/M (mitosis) transition |
BUB1B | BUB1 mitotic checkpoint serine/threonine kinase B | Essential component of the mitotic checkpoint |
KIF23 | Kinesin family member 23 | Component of the centralspindlin complex that serves as a microtubule-dependent and Rho-mediated signaling required for the myosin contractile ring formation during the cell cycle cytokinesis |
CDCA8 | Cell division cycle associated 8 | Major effector of the TTK kinase in the control of attachment-error-correction and chromosome alignment |
CDC20 | Cell division cycle 20 | Required for full ubiquitin ligase activity of the APC/C and may confer substrate specificity upon the complex |
KIF11 | Kinesin family member 11 | Motor protein required for establishing a bipolar spindle during mitosis |
APC/C, anaphase promoting complex/cyclosome.
Preadipocyte culture and observation
In our study, preadipocytes were successfully isolated using the conventional method, and the growth of cells was observed. Morphologically, the preadipocytes appeared spindle-shaped under microscopy and the differentiated group showed obvious lipid droplets with ORO staining at days 7 and 14 (Figure 5).
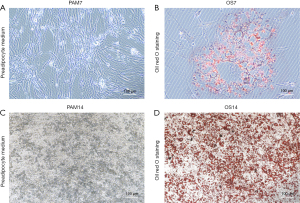
Verification of hub gene expression
To further validate the roles of hub genes in the differentiated and undifferentiated group as determined by the above-described data, the mRNA expression levels were examined with RT-qPCR at day 7 (early stage) and day 21 (late stage). The results indicated there to be no significant differences in NUSAP1, CCNB1, DLGAP5, TTK, CCNB2, KIF23, BUB1B, CDC20, CDCA8, or KIF11 between the differentiated group and the undifferentiated group (P>0.05) at day 7; however, the mRNA expression levels of the adipogenic marker gene (CCNB1) in adipogenic cells were significantly different from those in the cells of the control group (P=0.03, P<0.05) (Figure 6). As time elapsed, we further found that after 21 days of adipogenic differentiation, there was no significant difference in the detection of 10 hub genes between the differentiated and undifferentiated groups (P>0.05) (Figure 7).
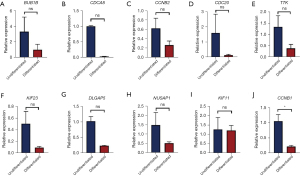
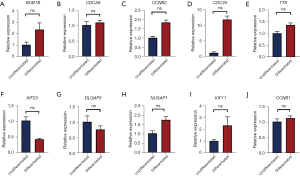
Discussion
In the field of breast tissue reconstruction for those who undergo breast cancer surgery, various biomaterials and synthetic materials have been employed extensively in recent years (38-40). The self-renewal ability, proliferation potential, and multidirectional differentiation characteristics of ADSCs are of great value in regenerative medicine applications after breast cancer surgery (41-43). The easily accessible and large number of ADSCs make them a promising seed cell for breast tissue engineering after breast cancer surgery. But some factors can influence the effectiveness of ADSCs, such as donor variability, isolation and culture conditions, cell proliferation and viability, immunomodulatory properties and so on, leading to the delayed wound healing in regenerative medicine and low retention rate of adipose tissue in fat transplantation (44). Several studies have explored the mechanisms underlying the survival of adipocytes. For example, LRG-1-mediated suppression of the ADSCs apoptosis induced by hypoxia was mediated by the upregulation of RAB31 expression in fat transplantation. Besides, browning of adipocytes in patients resulted in poorly survived fat grafts, because it had a higher gene expression level of uncoupling protein-1. Apart from the above-mentioned, A series of signaling pathways, epigenetic modifiers, and transcription factors play important roles in preadipocyte commitment and terminal differentiation into mature adipocytes. During the process, ADSCs can stimulate angiogenesis through differentiation into epithelial cells and release angiogenic factors by paracrine activity. Finally, dedifferentiation, which a mature adipocyte is reverted to an undifferentiated progenitor-like status under both physiological and pathological conditions, also has been reported as a mechanism explaining adipocyte plasticity (45-48). Therefore, the molecular mechanisms underlying the adipogenic differentiation of ADSCs still should be explored to facilitate the success of ADSCs-based therapies after breast cancer surgery.
In this study, we gathered and examined samples of ADSCs undergoing adipogenic differentiation from the GSE61302 dataset. Of the 1,091 DEGs that were evaluated in the dataset, 382 genes were upregulated and 709 genes were downregulated. Moreover, for a better understanding of the interactions between the identified DEGs, GO and KEGG enrichment analyses were performed. The GO analysis revealed that the 1,091 DEGs were mainly linked with leukocyte migration, cell chemotaxis, collagen-containing ECM, and glycosaminoglycan binding. A previous study has indicated that the ECMs from multiple sources include similar types of collagens but differ in the relative abundance of each; regardless, all of them are capable of inducing stronger osteogenesis and adipogenesis in mesenchymal stem cells (49). According to the KEGG pathway analysis in our study, the identified DEGs were related to viral protein interaction with cytokine and cytokine receptor, TNF signaling pathway, and cytokine-cytokine receptor interaction. Meanwhile, the pathways associated with upregulated and downregulated genes were TNF signaling pathway, cytokine-cytokine receptor interaction, PPARγ signaling pathway, complement and coagulation cascades, and C-type lectin receptor signaling pathway. Among these pathways, the TNF signaling pathway and the PPARγ signaling pathway are of particular importance, functioning as master transcriptional regulators for adipocyte differentiation. Indeed, PPARγ belongs to the nuclear hormone receptor superfamily of ligand-activated transcription factors, which is known to inhibit osteoblast differentiation and promote adipogenic differentiation (50,51).
Based on the centrality of the nodes in the PPI network, the NUSAP1, DLGAP5, TTK, CCNB2, KIF23, BUB1B, CDC20, CDCA8, KIF11, and CCNB1 genes were among the significant DEGs found. Of these 10 hub genes, 1 gene, CCNB1, was downregulated in adipogenic differentiation. CCNB1 is a protein-coding gene, and the protein encoded by this gene is a regulatory protein involved in mitosis and is necessary for proper control of the G2/M transition phase of the cell cycle. Among its related pathways are regulation of activated PAK-2p34 via proteasome-mediated degradation and loss of Nlp from mitotic centrosomes (52). Previous studies revealed that CCNB1 can regulate the proliferation of bone marrow stem cells, and other animal experiments have also demonstrated that the increase and decrease of CCNB1 gene expression level are related to the differentiation and proliferation of adipocytes (53,54).
However, previous study just showed that adipose ECM and microenvironment possess the capability to promote early adipogenesis, while the ECM alone cannot induce adipogenesis in vivo. ADSCs can adjust early adipogenesis with angiogenesis and tissue remodeling by directly differentiating into endothelial cells and regulating macrophage polarization, contributing to better long-term retention and greater tissue integrity (55). Few studies have explored the relationship between CCNB1 and adipogenic differentiation in human ADSCs, and this association is still poorly understood. Therefore, this study combined public databases and biological experimental techniques to investigate the genes related to adipogenic differentiation of human adipocytes. We discovered that the expression level of the CCNB1 gene in the differentiated group was significantly different from that in the undifferentiated group (P<0.05) in the early stage, but no significant difference was observed in the late stage (P>0.05). Therefore, it is worth continuing to investigate the underlying mechanisms of these changes.
This study offers a range of different perspectives on the adipogenic differentiation of human adipocytes. First, microarray and bioinformatics analyses were applied to assess whole transcriptome changes between differentiated and undifferentiated cells, and a preliminary investigation of the related mechanism was also carried out. Second, the combination of bioinformatics methods and molecular biology experiments in this study not only compensates for the limitations of bioinformatics methods but also improves the accuracy of the molecular biology experiments.
However, there are still some limitations to this study. First, more datasets or samples could have been included in the examined cohort. Second, the results and conclusions of this study should be verified by more experiments in the future.
In summary, these results provide the basis for further study of the adipogenic differentiation of ADSCs. One focus of future studies could involve the search for other genes closely related to the adipogenic differentiation of stem cells in order to better understand the related mechanisms and their value for breast reconstruction after breast cancer surgery.
Conclusions
Based on a bioinformatics method, this study conducted a comprehensive analysis of DEGs between differentiated and undifferentiated ADSCs. The key genes, CCNB1, NUSAP1, DLGAP5, TTK, CCNB2, KIF23, BUB1B, CDC20, CDCA8, and KIF11, may be significantly related to the adipogenic differentiation of ADSCs. After further experimental validation, we identified CCNB1 as a vital gene in the adipogenesis of ADSCs. The expression of the CCNB1 gene in adipocytes may contribute to an understanding of the biological characteristics of fat cells and the regulation of CCNB1 during the fat transplantation process could be manipulated to enhance the success rate of fat grafting. We believe our findings represent new insights useful for further investigating the mechanisms of ADSCs in adipogenesis and their clinical utilization as stem cells.
Acknowledgments
We would like to thank Dr. Enkang Lu, Dr. Beibei Xu, and Mrs. Yingchun Fan for their valuable advice in supporting this work.
Funding: This research was funded by
Footnote
Reporting Checklist: The authors have completed the MDAR reporting checklist. Available at https://gs.amegroups.com/article/view/10.21037/gs-23-493/rc
Data Sharing Statement: Available at https://gs.amegroups.com/article/view/10.21037/gs-23-493/dss
Peer Review File: Available at https://gs.amegroups.com/article/view/10.21037/gs-23-493/prf
Conflicts of Interest: All authors have completed the ICMJE uniform disclosure form (available at https://gs.amegroups.com/article/view/10.21037/gs-23-493/coif). Z.H.W. reports this research was funded by the Postgraduate Research & Practice Innovation Program of Jiangsu Province (No. JX10214035). The other authors have no conflicts of interest to declare.
Ethical Statement: The authors are accountable for all aspects of the work in ensuring that questions related to the accuracy or integrity of any part of the work are appropriately investigated and resolved. The study was conducted in accordance with the Declaration of Helsinki (as revised in 2013) and was approved by the Ethics Committee of the First Affiliated Hospital of Nanjing Medical University (protocol code: 2022-NT-79). Informed consent was obtained from all participants involved in the study.
Open Access Statement: This is an Open Access article distributed in accordance with the Creative Commons Attribution-NonCommercial-NoDerivs 4.0 International License (CC BY-NC-ND 4.0), which permits the non-commercial replication and distribution of the article with the strict proviso that no changes or edits are made and the original work is properly cited (including links to both the formal publication through the relevant DOI and the license). See: https://creativecommons.org/licenses/by-nc-nd/4.0/.
References
- Stachs A, Stubert J, Reimer T, et al. Benign Breast Disease in Women. Dtsch Arztebl Int 2019;116:565-74. [Crossref] [PubMed]
- Loibl S, Poortmans P, Morrow M, et al. Breast cancer. Lancet 2021;397:1750-69. [Crossref] [PubMed]
- Delay E, Guerid S, Meruta AC. Indications and Controversies in Lipofilling for Partial Breast Reconstruction. Clin Plast Surg 2018;45:101-10. [Crossref] [PubMed]
- Hanson SE, Kapur SK, Hwang RF, et al. Autologous fat grafting in breast reconstruction: implications for follow-up and surveillance. Gland Surg 2021;10:487-93. [Crossref] [PubMed]
- Soon PS, Ruban S, Mo HTJ, et al. Understanding patient choices regarding breast reconstruction after mastectomy for breast cancer. Support Care Cancer 2019;27:2135-42. [Crossref] [PubMed]
- Régis C, Le J, Chauvet MP, et al. Variations in the breast reconstruction rate in France: A nationwide study of 19,466 patients based on the French medico-administrative database. Breast 2018;42:74-80. [Crossref] [PubMed]
- Seitz AJ, Asaad M, Hanson SE, et al. Autologous Fat Grafting for Oncologic Patients: A Literature Review. Aesthet Surg J 2021;41:S61-8. [Crossref] [PubMed]
- Vyas KS, DeCoster RC, Burns JC, et al. Autologous Fat Grafting Does Not Increase Risk of Oncologic Recurrence in the Reconstructed Breast. Ann Plast Surg 2020;84:S405-10. [Crossref] [PubMed]
- Wederfoort JLM, Hebels SA, Heuts EM, et al. Donor site complications and satisfaction in autologous fat grafting for breast reconstruction: A systematic review. J Plast Reconstr Aesthet Surg 2022;75:1316-27. [Crossref] [PubMed]
- Zhang X, Mu D, Lin Y, et al. Prediction of the Postoperative Fat Volume Retention Rate After Augmentation Mammoplasty with Autologous Fat Grafting: From the Perspective of Preoperative Inflammatory Level. Aesthetic Plast Surg 2022;46:2488-99. [Crossref] [PubMed]
- Banani MA, Rahmatullah M, Farhan N, et al. Adipose tissue-derived mesenchymal stem cells for breast tissue regeneration. Regen Med 2021;16:47-70. [Crossref] [PubMed]
- Teixeira AM, Martins P. A review of bioengineering techniques applied to breast tissue: Mechanical properties, tissue engineering and finite element analysis. Front Bioeng Biotechnol 2023;11:1161815. [Crossref] [PubMed]
- Yilmaz TU, Karakayali L. The versatility of adipose derived stem cells in liver transplantation: a narrative review. Dig Med Res 2023;6:7.
- Shafaei H, Kalarestaghi H. Adipose-derived stem cells: An appropriate selection for osteogenic differentiation. J Cell Physiol 2020;235:8371-86. [Crossref] [PubMed]
- Ambele MA, Dhanraj P, Giles R, et al. Adipogenesis: A Complex Interplay of Multiple Molecular Determinants and Pathways. Int J Mol Sci 2020;21:4283. [Crossref] [PubMed]
- Fang J, Chen F, Liu D, et al. Adipose tissue-derived stem cells in breast reconstruction: a brief review on biology and translation. Stem Cell Res Ther 2021;12:8. [Crossref] [PubMed]
- Challapalli RS, Dwyer RM, McInerney N, et al. Effect of Breast Cancer and Adjuvant Therapy on Adipose-Derived Stromal Cells: Implications for the Role of ADSCs in Regenerative Strategies for Breast Reconstruction. Stem Cell Rev Rep 2021;17:523-38. [Crossref] [PubMed]
- Qin Y, Ge G, Yang P, et al. An Update on Adipose-Derived Stem Cells for Regenerative Medicine: Where Challenge Meets Opportunity. Adv Sci (Weinh) 2023;10:e2207334. [Crossref] [PubMed]
- Debuc B, Gendron N, Cras A, et al. Improving Autologous Fat Grafting in Regenerative Surgery through Stem Cell-Assisted Lipotransfer. Stem Cell Rev Rep 2023;19:1726-54. [Crossref] [PubMed]
- Huang H, Tang X, Li S, et al. Advanced platelet-rich fibrin promotes the paracrine function and proliferation of adipose-derived stem cells and contributes to micro-autologous fat transplantation by modulating HIF-1α and VEGF. Ann Transl Med 2022;10:60. [Crossref] [PubMed]
- Li X, Zhao Y, Li X, et al. MicroRNA-150 Modulates Adipogenic Differentiation of Adipose-Derived Stem Cells by Targeting Notch3. Stem Cells Int 2019;2019:2743047. [Crossref] [PubMed]
- Huang KT, Hsu LW, Chen KD, et al. Decreased PEDF Expression Promotes Adipogenic Differentiation through the Up-Regulation of CD36. Int J Mol Sci 2018;19:3992. [Crossref] [PubMed]
- Falavinha BC, Barisón MJ, Rebelatto CLK, et al. Interleukin 21 Receptor Affects Adipogenesis of Human Adipose-Derived Stem/Stromal Cells. Stem Cells Int 2022;2022:4930932. [Crossref] [PubMed]
- Zhang XX, Wang YM, Su YD, et al. MiR-26a regulated adipogenic differentiation of ADSCs induced by insulin through CDK5/FOXC2 pathway. Mol Cell Biochem 2021;476:1705-16. [Crossref] [PubMed]
- Liu Y, Guo L, Hu L, et al. D-Mannose Inhibits Adipogenic Differentiation of Adipose Tissue-Derived Stem Cells via the miR669b/MAPK Pathway. Stem Cells Int 2020;2020:8866048. [Crossref] [PubMed]
- Qian Y, Chen H, Pan T, et al. Autologous decellularized extracellular matrix promotes adipogenic differentiation of adipose derived stem cells in low serum culture system by regulating the ERK1/2-PPARγ pathway. Adipocyte 2021;10:174-88. [Crossref] [PubMed]
- da Silva C, Durandt C, Kallmeyer K, et al. The Role of Pref-1 during Adipogenic Differentiation: An Overview of Suggested Mechanisms. Int J Mol Sci 2020;21:4104. [Crossref] [PubMed]
- Pelegri NG, Milthorpe BK, Gorrie CA, et al. Neurogenic marker expression in differentiating human adipose derived adult mesenchymal stem cells. Stem Cell Investig 2023;10:7. [Crossref] [PubMed]
- Limoli PG, Nebbioso M. Immunophenotypic analysis of human adipose-derived stem cells through multi-color flow cytometry. Stem Cell Investig 2023;10:13. [Crossref] [PubMed]
- Corvera S. Cellular Heterogeneity in Adipose Tissues. Annu Rev Physiol 2021;83:257-78. [Crossref] [PubMed]
- Satish L, Krill-Burger JM, Gallo PH, et al. Expression analysis of human adipose-derived stem cells during in vitro differentiation to an adipocyte lineage. BMC Med Genomics 2015;8:41. [Crossref] [PubMed]
- Ashburner M, Ball CA, Blake JA, et al. Gene ontology: tool for the unification of biology. The Gene Ontology Consortium. Nat Genet 2000;25:25-9. [Crossref] [PubMed]
- Kanehisa M, Goto S, Sato Y, et al. KEGG for integration and interpretation of large-scale molecular data sets. Nucleic Acids Res 2012;40:D109-14. [Crossref] [PubMed]
- Yu G, Wang LG, Han Y, et al. clusterProfiler: an R package for comparing biological themes among gene clusters. OMICS 2012;16:284-7. [Crossref] [PubMed]
- Szklarczyk D, Franceschini A, Wyder S, et al. STRING v10: protein-protein interaction networks, integrated over the tree of life. Nucleic Acids Res 2015;43:D447-52. [Crossref] [PubMed]
- Shannon P, Markiel A, Ozier O, et al. Cytoscape: a software environment for integrated models of biomolecular interaction networks. Genome Res 2003;13:2498-504. [Crossref] [PubMed]
- Bader GD, Hogue CW. An automated method for finding molecular complexes in large protein interaction networks. BMC Bioinformatics 2003;4:2. [Crossref] [PubMed]
- Mu X, Zhang J, Jiang Y. 3D Printing in Breast Reconstruction: From Bench to Bed. Front Surg 2021;8:641370. [Crossref] [PubMed]
- Prasad K, Zhou R, Zhou R, et al. Cosmetic reconstruction in breast cancer patients: Opportunities for nanocomposite materials. Acta Biomater 2019;86:41-65. [Crossref] [PubMed]
- Zarei F, Daraee H. Recent Progresses in Breast Reconstruction: Stem Cells, Biomaterials, and Growth Factors. Drug Res (Stuttg) 2018;68:311-6. [Crossref] [PubMed]
- Mazini L, Rochette L, Amine M, et al. Regenerative Capacity of Adipose Derived Stem Cells (ADSCs), Comparison with Mesenchymal Stem Cells (MSCs). Int J Mol Sci 2019;20:2523. [Crossref] [PubMed]
- Wang K, Yu Z, Rong X, et al. Meta-Analysis of the Oncological Safety of Autologous Fat Grafting After Breast Cancer on Basic Science and Clinical Studies. Aesthetic Plast Surg 2023;47:1245-57. [Crossref] [PubMed]
- Wu Q, Chen S, Peng W, et al. Current perspectives on cell-assisted lipotransfer for breast cancer patients after radiotherapy. World J Surg Oncol 2023;21:133. [Crossref] [PubMed]
- Mazini L, Rochette L, Admou B, et al. Hopes and Limits of Adipose-Derived Stem Cells (ADSCs) and Mesenchymal Stem Cells (MSCs) in Wound Healing. Int J Mol Sci 2020;21:1306. [Crossref] [PubMed]
- Ho CK, Zheng D, Sun J, et al. LRG-1 promotes fat graft survival through the RAB31-mediated inhibition of hypoxia-induced apoptosis. J Cell Mol Med 2022;26:3153-68. [Crossref] [PubMed]
- Song T, Kuang S. Adipocyte dedifferentiation in health and diseases. Clin Sci (Lond) 2019;133:2107-19. [Crossref] [PubMed]
- Liu T, Fu S, Wang Q, et al. Evidence of Browning of White Adipocytes in Poorly Survived Fat Grafts in Patients. Aesthet Surg J 2021;41:NP1086-91. [Crossref] [PubMed]
- Hutchings G, Janowicz K, Moncrieff L, et al. The Proliferation and Differentiation of Adipose-Derived Stem Cells in Neovascularization and Angiogenesis. Int J Mol Sci 2020;21:3790. [Crossref] [PubMed]
- Marinkovic M, Tran ON, Block TJ, et al. Native extracellular matrix, synthesized ex vivo by bone marrow or adipose stromal cells, faithfully directs mesenchymal stem cell differentiation. Matrix Biol Plus 2020;8:100044. [Crossref] [PubMed]
- Yuan Z, Li Q, Luo S, et al. PPARγ and Wnt Signaling in Adipogenic and Osteogenic Differentiation of Mesenchymal Stem Cells. Curr Stem Cell Res Ther 2016;11:216-25. [Crossref] [PubMed]
- Zoller V, Funcke JB, Keuper M, et al. TRAIL (TNF-related apoptosis-inducing ligand) inhibits human adipocyte differentiation via caspase-mediated downregulation of adipogenic transcription factors. Cell Death Dis 2016;7:e2412. [Crossref] [PubMed]
- Xie B, Wang S, Jiang N, et al. Cyclin B1/CDK1-regulated mitochondrial bioenergetics in cell cycle progression and tumor resistance. Cancer Lett 2019;443:56-66. [Crossref] [PubMed]
- Dhoke NR, Kalabathula E, Kaushik K, et al. Histone deacetylases differentially regulate the proliferative phenotype of mouse bone marrow stromal and hematopoietic stem/progenitor cells. Stem Cell Res 2016;17:170-80. [Crossref] [PubMed]
- Yan M, Cai L, Duan X, et al. Novel mechanistic role of Kif26b in adipogenic differentiation of murine multipotent stromal cells. Biochem Biophys Res Commun 2022;592:125-33. [Crossref] [PubMed]
- Jiang W, Cai J, Guan J, et al. Characterized the Adipogenic Capacity of Adipose-Derived Stem Cell, Extracellular Matrix, and Microenvironment With Fat Components Grafting. Front Cell Dev Biol 2021;9:723057. [Crossref] [PubMed]