Prediction of central lymph node metastasis in patients with papillary thyroid microcarcinoma by gradient-boosting decision tree model based on ultrasound radiomics and clinical features
Highlight box
Key findings
• The gradient boosting decision tree model (GBDT) model based on ultrasound (US) radiomics features and clinical factors is better for predicting compartment lymph node metastases (CLNMs) in papillary thyroid microcarcinoma (PTMC) patients than the US diagnosis alone.
What is known and what is new?
• Radiomics research is more carried out in the diagnosis of papillary thyroid carcinoma and lymph node metastasis, and previous radiomics research mostly uses logistic regression models or common nonlinear models (such as support vector machine models, random forest models, etc.).
• In this study, radiomics was used to predict the central lymph node metastasis of PTMC and achieved good results, and the GBDT model was used for the first time in thyroid radiomics studies.
What is the implication, and what should change now?
• The GBDT model established in this study is better than US for the diagnosis of CLNM. The GBDT model can guide clinical decisions about patient treatment.
Introduction
The Global Cancer Report states that the incidence of thyroid cancer has increased significantly worldwide in recent years (1). In China, thyroid cancer has the 7th highest incidence of tumors and is one of the most common malignant tumors. The predominant and fastest growing type of thyroid cancer is papillary thyroid microcarcinoma (PTMC), which represents more than 50% of new cases (2,3). Similar to the biological behavior of papillary thyroid carcinoma (PTC), PTMC is also prone to cervical lymph node metastasis, in which the central lymph nodes are most often involved (4). Several studies have been reported that the rate of central compartment lymph node metastases (CLNMs) of PTMC ranges from 30–82.0% (5-8). Lymph node metastasis is closely related to local recurrence and distant metastasis in PTMC (9,10).
According to the lymph node dissection strategy in the American Thyroid Association (ATA) guidelines (11), patients with PTMC with clinically diagnosed CLNM should undergo total thyroidectomy and therapeutic compartment lymph node dissection (CLND). The need for prophylactic CLND in patients without lymph node metastases (i.e., cN0) is inconclusive. Prophylactic CLND has been shown to improve disease-specific survival, reduce local recurrence rates, guide postoperative radioactive iodine (RAI) therapy (12-14), and improve the accuracy of recurrence risk assessment (15). Conversely, some studies have reported that prophylactic CLND increased the risk of recurrent laryngeal nerve and parathyroid injury (16,17). For low-risk PTMC, the presence or absence of lymph node metastasis is also an important reference indicator for dynamic follow-up observation (11). Therefore, accurate preoperative identification of CLNM in patients with PTMC is essential for the treatment strategy.
High-resolution ultrasound (US) is the preferred modality for assessing cervical lymph node status in patients with PTMC; however, US has low sensitivity (20–60%) for the detection of CLNM (18,19) and is susceptible to the quality of the US system, operator experience, and surrounding anatomy in the neck, such as the trachea, esophagus, and blood vessels (20). Furthermore, the evaluation of cervical lymph nodes by US-guided fine-needle aspiration cytology (FNAC) cannot rule out potential micrometastases or occult metastases. Computed tomography (CT) with a good tissue and spatial resolution has insufficient diagnostic efficacy for CLNM (21,22). Therefore, accurate preoperative assessment of CLNM based on imaging methods remains a challenge.
In recent years, the field of radiomics has developed rapidly for detection and diagnosis of tumor, management strategy, prognosis inference, and assistance of prediction of survival outcomes in PTC (23,24). However, previous studies have shown that models based solely on US radiomics characteristics are less effective in predicting CLNM in patients with PTC (25,26). Moreover, the value of area under the curve (AUC) based on clinical risk factors for CLNM in PTMC ranges from 0.709 to 0.780 (27,28). Studies based solely on US image radiomics features or clinical factors cannot achieve a high level of effectiveness. Therefore, this study aimed to establish a model for preoperative prediction of CLNM in patients with PTMC based on the combination US radiomics characteristics and clinical risk factors. We present this article in accordance with the TRIPOD reporting checklist (available at https://gs.amegroups.com/article/view/10.21037/gs-23-456/rc).
Methods
Patient source
This retrospective study was approved by the Ethics Committee of China-Japan Union Hospital of Jilin University (approval number: 20221020033), and the study conformed to the tenets of the Declaration of Helsinki (revised in 2013). The patients or their legal guardians signed a detailed informed consent form before the procedure and the personal information of the participants was protected. The data of this study were obtained from the database of patients who underwent surgery in the Division of Thyroid Surgery of China-Japan Union Hospital of Jilin University from January 2020 to July 2022. The inclusion and exclusion criteria were as follows. Inclusion criteria: (I) pathological diagnosis of PTC with maximum diameter ≤1 cm; (II) the patient was treated with thyroidectomy plus CLND (therapeutic or prophylactic); (III) complete clinical data and US image data. Exclusion criteria: (I) patients with inflammation of the thyroid gland, artifacts or noise affecting image quality in US images; (II) accompanied by non-PTC components (such as follicular tumors, medullary cancer, non-differentiated carcinoma, etc.); (III) previous radiofrequency or microwave therapy.
All patients in this study received therapeutic or prophylactic CLND, ATA’s statement on central group lymph node dissection (29), defined the area of CLND as all parathyroid and paratracheal soft tissue and lymph nodes with boundaries extending upward to the hyoid bone, the lower boundary to the innominate artery, and the lateral boundary to both common carotid arteries. Pathological examination of the removed thyroid tissue was performed to determine the type and size of the lesion. Removed lymph nodes were also examined pathologically and lymph node metastases were determined.
Clinical data
Figure 1 shows the patient recruitment process. A total of 274 patients met the criteria (mean age, 44.13±9.27 years; range, 21–67 years). Patients were divided into two groups in an 8:2 ratio using computer-generated random numbers: a training cohort (n=219; mean age 44.37±9.27 years; age 21–67 years) and a validation cohort (n=55, mean age 43.42±9.19 years; age 22–64 years). Clinical information was collected from each patient, including sex, age, body mass index (BMI), thyroid stimulating hormone (TSH), thyroglobulin (TG), anti-thyroglobulin antibodies (TGABs), and central lymph node status reported on preoperative US. According to the lymph node metastasis reported by the patient’s pathology, participants were divided into node-positive subgroups and node-metastasis-negative subgroups in the training cohort and the validation cohort, and the clinical information was compared between the two groups.
US image acquisition
All patients underwent a US of the thyroid and cervical lymph nodes before surgery. The US examination is performed using the SonoScape S50 Pro (SonoScape, Shenzhen, China) US device based on the Wi-Sono platform and a 12L-A multivariate single crystal transducer, with a frequency set to 8–13 MHz and a depth set to 4.5 cm. Thyroid US interpretation, diagnosis of disease, and evaluation of cervical lymph node status were performed by doctors with more than 5 years of experience in US. The retention of US images had the following requirements: (I) the maximum diameter of the lesion is used as the intercepted plane to retain as many ultrasonic features as possible; (II) the image contains data such as lesion size and Doppler measurement. All US images were stored in DICOM format.
Assessment of lymph node status in the central compartment
All patients underwent preoperative US of thyroid and cervical lymph nodes. The description “Lymph node structure is poorly defined, consider secondary” in the US report was defined as a positive CLNM reported on the US. “No abnormal enlargement of cervical lymph nodes”, “inflammatory lymph nodes”, and “structurally clear lymph nodes” were defined as CLNM negative on US. Abnormal US features suggestive of CLNM are defined as microcalcifications, partially cystic appearance, peripheral or diffusely increased vascularization, hyperechoic tissue looking like thyroid (30).
Region of interest (ROI) division and feature extraction
The compliant US images were imported into open-source software (ITK-SNAP 3.8.0; www.itksnap.org), and the ROI of the lesion was manually mapped in the US images. This step was performed by a doctor with more than 5 years of experience, and the doctor was blinded to the pathological outcome of the patient throughout the process of mapping the ROI. Image histology features were automatically extracted using open-source software (Pyradiomics; http://pyradiomics.readthedocs.io/en/latest/index.html), including shape features, first-order histogram features, gray level co-occurrence matrix features, gray level size zone matrix features, gray level run length matrix features, neighboring gray tone difference matrix features, gray level dependence matrix, and wavelet features.
Feature filtering
To ensure the reliability and accuracy of the results, the missing radiomics features were removed, the anomalous data in the remaining features were filled with medians, and then the original data were preprocessed by the maximum and minimum normalization method, and finally Waikato Environment for Knowledge Analysis (WEKA) software (WEKA 3.8.6; www.cs.waikato.ac.nz/ml/weka/) was used to screen for radiomics features.
Establishment and verification of the model
A machine learning model based on the gradient-boosting decision tree (GBDT) algorithm was constructed based on the radiomics characteristics and clinical risk factors obtained by feature screening. To evaluate the predictive performance of the model, the GBDT model was trained by 10-fold cross-validation. In order to avoid the overfitting phenomenon of the model, the parameters of the model are adjusted by the multi-objective optimization software modeFRONTIER (https://engineering.esteco.com/modefrontier/). We evaluated the diagnostic efficacy of CLNM status predicted by the GBDT model by the area under the receiver operating characteristic (ROC) curve and compared it with the diagnostic efficacy of lymph node status reported by US. The discriminant performance of the model in the training and validation queues was further evaluated by plotting the detection error tradeoff (DET) curve. To determine the clinical significance of the GBDT model, decision curve analysis (DCA) was used to quantify the net benefit at different threshold probabilities in the validation cohort.
Visual analysis of the model
Compared with linear models, nonlinear machine learning models face the “black box” problem, and it is difficult for people to understand the weights and decision-making processes of each feature in the model, which makes the interpretability of the model decrease. SHapley Additive ExPlanations (SHAP) is a game theory method used to explain the output of any machine learning model, the core idea of which is to calculate the contribution value of features to the output of the model, and then explain the model from both global and local levels. It provides an effective visualization method for machine learning models. The use of SHAP can be used to visualize the global and local analysis of the GBDT model, making the decision-making process of the model more transparent and easier to understand.
Statistical analysis
Statistical analysis was performed using the software SPSS 23.0 (IBM Corp., Armonk, NY, USA) and Python programming language (version 3.7.9; Python Software Foundation, Wilmington, DE, USA). Normally distributed measurement data was expressed as mean ± standard deviation. The Student’s t-test was used to compare differences between groups. Categorical data was represented as a number (percentage) and compared using a Chi-squared test. All statistical significance was bilateral, and a P value of <0.05 was considered statistically significant.
The area under curve (AUC) was used to evaluate the diagnostic performance of radiomics models and US-reported lymph node status. The scikit-learn Python library (version 1.0.2) was used to build GBDT models, calculates AUC values, and plots ROC curves, and the Delong test was used to compare differences between different AUCs. The GBDT model comes from the Gradient Boosting Classifier function in the scikit-learn library, and the SHAP Python framework (version 0.41.0) was used to execute the SHAP algorithm.
Results
Clinical features
The clinical features of patients in the training cohort and validation cohort are shown in Table 1. There were 219 people in the training cohort and 55 people in the validation cohort, and the proportion of CLNM-positive patients in the training cohort and the validation cohort was 50.7% and 49.1%, respectively (P=0.833). There were also no significant differences in other clinical features between the two cohorts indicating that the training cohort and the validation cohort were comparable in these clinical features. Patients with CLNM tend to be young, male, and higher positive rate of CLNM by US in the training and validation cohort (Table 2).
Table 1
Characteristics | Training cohort | Validation cohort | P |
---|---|---|---|
Age (years) | 44.37±9.27 | 43.42±9.19 | 0.667 |
Gender | 0.544 | ||
Male | 48 (21.9) | 10 (18.2) | |
Female | 171 (78.1) | 45 (81.8) | |
TSH | 0.897 | ||
Normal (<4.94 ng/mL) | 208 (95.0) | 52 (94.5) | |
Abnormal (≥4.94 ng/mL) | 11 (5.0) | 3 (5.5) | |
TG | 0.083 | ||
Normal (<77 ng/mL) | 13 (5.9) | 7 (12.7) | |
Abnormal (≥77 ng/mL) | 206 (94.1) | 48 (87.3) | |
TGABs | 0.541 | ||
Normal (<115.00 IU/mL) | 182 (83.1) | 48 (87.3) | |
Abnormal (≥115.00 IU/mL) | 37 (16.9) | 7 (12.7) | |
BMI (kg/m2) | 24.94±3.79 | 25.09±3.02 | 0.784 |
BMI grouped | 0.632 | ||
Thin (<18.5 kg/m2) | 3 (1.4) | 1 (1.8) | |
Normal (18.5 kg/m2≤ BMI <25 kg/m2) | 117 (53.4) | 28 (50.9) | |
Overweight (25 kg/m2≤ BMI <30 kg/m2) | 78 (35.6) | 23 (41.8) | |
Obese (≥30 kg/m2) | 21 (9.6) | 3 (5.5) | |
LN metastasis | 0.833 | ||
LN-positive | 111 (50.7) | 27 (49.1) | |
LN-negative | 108 (49.3) | 28 (50.9) |
Data are presented as mean ± SD or n (%). TSH, thyroid-stimulating hormone; TG, thyroglobulin; TGABs, anti-thyroglobulin antibodies; BMI, body mass index; LN, lymph node; SD, standard deviation.
Table 2
Characteristics | Training cohort | Validation cohort | |||||
---|---|---|---|---|---|---|---|
LN (+) | LN (−) | P | LN (+) | LN (−) | P | ||
Age (years) | 41.88±9.66 | 46.94±8.12 | <0.001 | 40.81±8.24 | 45.75±9.56 | 0.045 | |
Gender | <0.001 | 0.031 | |||||
Male | 35 (31.5) | 13 (12.0) | 8 (29.6) | 2 (7.1) | |||
Female | 76 (68.5) | 95 (88.0) | 19 (70.4) | 26 (92.9) | |||
TSH | 0.111 | 0.531 | |||||
Normal (<4.94 ng/mL) | 108 (97.3) | 100 (92.6) | 25 (92.6) | 27 (96.4) | |||
Abnormal (≥4.94 ng/mL) | 3 (2.7) | 8 (7.4) | 2 (7.4) | 1 (3.6) | |||
TG | 0.736 | 0.245 | |||||
Normal (<77 ng/mL) | 105 (94.6) | 101 (93.5) | 25 (92.6) | 23 (82.1) | |||
Abnormal (≥77 ng/mL) | 6 (5.4) | 7 (6.5) | 2 (7.4) | 5 (17.9) | |||
TGABs | 0.786 | 0.245 | |||||
Normal (<115.00 IU/mL) | 93 (83.8) | 89 (82.4) | 25 (92.6) | 23 (82.1) | |||
Abnormal (≥115.00 IU/mL) | 18 (16.2) | 19 (17.6) | 2 (7.4) | 5 (17.9) | |||
BMI (kg/m2) | 24.83±3.84 | 25.04±3.75 | 0.684 | 25.45±3.67 | 24.73±2.23 | 0.387 | |
BMI grouped | 0.845 | 0.053 | |||||
Thin (<18.5 kg/m2) | 2 (1.8) | 1 (0.9) | 1 (3.7) | 0 | |||
Normal (18.5 kg/m2≤ BMI <25 kg/m2) | 60 (54.1) | 57 (52.8) | 10 (37.0) | 18 (64.3) | |||
Overweight (25 kg/m2≤ BMI <30 kg/m2) | 40 (36.0) | 38 (35.2) | 13 (48.1) | 0 | |||
Obese (≥30 kg/m2) | 9 (8.1) | 12 (11.1) | 3 (11.1) | 10 (35.7) | |||
US reported LN status | 0.004 | 0.031 | |||||
LN-positive | 37 (33.3) | 18 (16.7) | 8 (29.6) | 2 (7.1) | |||
LN-negative | 74 (66.7) | 90 (83.3) | 19 (70.4) | 26 (92.9) |
Data are presented as mean ± SD or n (%). LN, lymph node; TSH, thyroid-stimulating hormone; TG, thyroglobulin; TGABs, anti-thyroglobulin antibodies; BMI, body mass index; US, ultrasound; SD, standard deviation.
Radiomics features
In the training cohort, 1,034 features were extracted from each raw US image. After screening, we removed 52 invalid features and retained 982 valid features. After the screening of WEKA software, seven radiomics features were finally obtained, including two first-order histogram features, one gray level co-occurrence matrix features, one gray level size zone matrix feature, one gray level run length matrix features, and one neighboring gray tone difference matrix feature.
GBDT model establishment and verification
The AUC values of the GBDT model in the training cohort and validation cohort were 0.946 and 0.845, respectively (Figure 2A), whereas the AUC values for lymph node status reported by the US alone were 0.583 and 0.582, respectively (Figure 2B). In the training cohort, the AUC value of GBDT was significantly higher compared to the AUC value of lymph node status reported by US, and the Delong test showed a significant difference between the two values (0.946 vs. 0.583, P<0.001), and the same result was obtained in the validation cohort (0.845 vs. 0.582, P<0.001). The modeFRONTIER software constrains the AUC values of the training and validation queue GBDT model to avoid overfitting of the model (Figure 3). To show the discriminant performance of the GBDT model, a DET curve (Figure 4) was plotted, showing that the curves of both cohorts were concentrated in the third quadrant, indicating that their false rejection rate and false acceptance rate were both at a low level. DCA (Figure 5) was used to evaluate the clinical significance of the radiomics GBDT model. The results showed that when the threshold probability is between 0.1 and 1.0, predicting CLNM with the GBDT model is more beneficial for all treated or untreated patients.
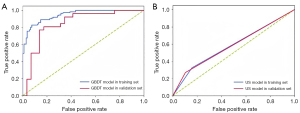
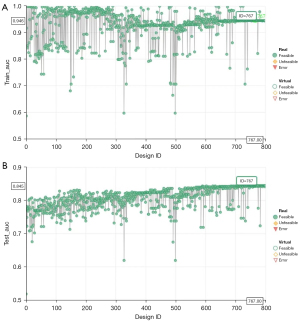
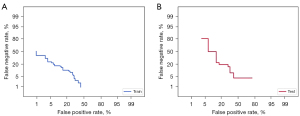

SHAP
The classification bar plot of the SHAP summary plot (Figure 6A) was obtained by extracting the average absolute values of each parameter, including seven radiomic features, gender, age after stratification, and BMI, to show global significance. Gender, imaging characteristics, 46–55 age group, and 26–35 age group are the key features of the model. A local bar chart (Figure 6B) showing the function transformation of data from one of the patients was created to illustrate the local meaning of each feature. The scatter plot of the SHAP summary plot (Figure 6C) reflected the relationship between each prediction parameter and the predicted probability through different colors, and the feature importance was arranged from high to low, including positive and negative effects. Overall, radiomics characteristics and age groups between 26 and 35 years played a positive role in assessing CLNM. Women and those aged 46–55 years had negative effects. The SHAP heat map (Figure 6D) hierarchically clustered patients according to SHAP values, showing the distribution of patients with CLNM and non-CLNM in a prominent form, where red represents cases with a high probability of CLNM and colorless or blue represents cases with no or low probability of CLNM.
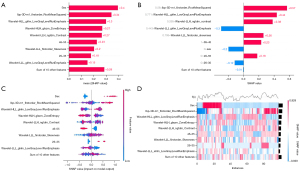
Discussion
Under the principle that the diagnosis and treatment of thyroid cancer are gradually becoming comprehensive, accurate, and individualized, whether cN0 PTMC patients should undergo prophylactic CLND is still controversial. ATA guidelines state that prophylactic CLND may be appropriate after thyroidectomy in PTMC patients without risk factors, whereas Chinese expert consensus prefers prophylactic CLND. Active surveillance can be considered in patients with PTMC because of slow progressive tumor characteristics, however, lymph node metastasis has been considered as an indication for surgical treatment of PTMC (31,32). Therefore, accurate preoperative determination of lymph node status in the central compartment is clinically important for the selection of treatment options.
Even though US is the first modality for evaluation of cervical lymph node, postoperative CLNM was revealed up to 50.6% of patients with cN0 PTMC (33). Detecting CLNM might be affected by several reasons like sternum occlusion and endotracheal gas and also by the tiny size of suspected lymph nodes, and para retro tracheal location. Furthermore, the US diagnosis of CLNM is closely related to operator experience and the quality of the US system. Contrast-enhanced CT is more effective than the US in evaluating CLNM in PTC, but a multicenter prospective study found that contrast-enhanced CT significantly improved the detection rate of neck-positive lymph nodes (21.3%), while the contrast-enhanced CT only improved the detection rate of CLNM by 8.9% (34) and predominantly in non-PTMC patients, suggesting that contrast-enhanced CT does not improve the diagnostic yield of CLNM in patients with PTMC. The traditional preoperative diagnosis method of CLNM cannot meet our needs for accurate diagnosis of CLNM before surgery.
Our study established a GBDT model of CLNM for preoperative prediction of PTMC based on US radiomics characteristics and clinical risk factors that may predict CLNM and evaluated the diagnostic efficacy and clinical utility of the model. Similar to previous clinical prediction studies (27,35), we included the sex, age, BMI, and serology of patients in the study of risk factors associated with CLNM. Among them, in the training and verification cohort, gender (<0.001 and 0.031) and age (<0.001 and 0.045) were associated with CLNM. Whether thyroid inflammation is related to CLNM of PTMC is controversial, and a study believes that there is no clear correlation between the two (36), considering that thyroid inflammation will lead to corresponding changes in thyroid US images, which will affect the division of ROIs and feature extraction, patients with thyroid inflammation are excluded, and inflammatory indicators such as TPOAB have not been included in the model. Although statistical analysis shows that BMI is not related to CLNM, a study has shown that obesity promotes the occurrence and development of thyroid malignant tumors (37), and we wanted to explore the relationship between BMI and CLNM of PTMC, so we added it to subsequent model construction. However, through subsequent visual analysis, it was found that BMI accounted for a low weight in the process of model prediction of CLNM in patients, and the SHAP scatterplot showed that it had no obvious predictive effect on CLNM, so BMI had a poor prediction effect on CLNM in patients in this study. Unlike previous studies, we stratified age and then incorporated it into the model construction to analyze the predictive contribution of different age stratification to the occurrence of CLNM. Through the scattered distribution of the SHAP summary plot, we found that the 46–55 age group had a negative effect on CLNM, whereas the 26–35 and 36–45 age groups had a positive effect on CLNM, and the distribution of SHAP scatter in the 26–35 age group was more concentrated than that in 36–45, which indicated that the younger the patient, the higher the chance of CLNM. This is consistent with previous studies (32,36). In this study, radiomics features can reflect high-throughput information from US images, so the features reported by US (echo, calcification, composition) were not added to the model alone.
In the process of image group feature selection, we used WEKA software (38), which can be widely used in the processing of high-throughput information in various studies. In our feature selection process, the correlation-based feature selection algorithm was applied to construct the correlation matrix of feature-class and feature-feature, and then the greedy algorithm of forward search was used to remove redundant features and filter out features that are highly correlated with the target and not related to each other. The advantage of correlation-based feature selection algorithms is that they improve the performance of machine learning algorithms while reducing the number of features used in machine learning. A total of seven radiomics features were screened and a GBDT model was established by combining clinical risk factors. During the modeling process, we used modeFRONTIER parameter adjustment software to adjust the important parameters of the GBDT model. modeFRONTIER uses genetic algorithms such as the multi-objective genetic algorithm to perform multi-parameter and multi-target automatic optimization operations, which can quickly find the optimal design solution in the shortest time. At the same time, the whole process is more efficient and automated, and a large number of algorithms can be explored quickly. Many drawbacks of manual parameter tuning are avoided. We take the algorithm parameters as the optimization variables, maximize the diagnostic performance of the model of the validation set as the optimization goal, and constrain the model process established based on the training set to achieve the optimal effect of the verification model while avoiding the occurrence of model overfitting.
In the training cohort and validation cohort, the AUC values of the GBDT model were high (0.946 and 0.845), indicating that the model had a good predictive effect on CLNM. The diagnostic performance of lymph node status was significantly better than that reported by US alone (0.583 and 0.582, Delong test, P<0.001), indicating that the GBDT model combined with radiomics and clinical features can predict CLNM more accurately than experienced sonographers. The prediction effect of the model in the verification queue is compared with that previously reported, and the results show that the model has more advantages. A study has explored the relationship between US texture analysis and lymph node metastasis of PTMC, but the results show that texture analysis cannot predict lymph node metastasis in patients with PTMC (39). There are also studies that combine US features and clinical features to establish Nomogram to predict the CLNM of PTMC, with an AUC value of only 0.709–0.780 (28,35). In our study, the AUC of GBDT model in verification queue can reach 0.845. This means that the omics features of US imaging may contain more information that can predict CLNM. All risk factors in this study model are available prior to surgery, so this model can be used for individualized assessment of CLNM risk in PTMC patients. The Detection error tradeoff (DET) curve showed that the curves of both cohorts are concentrated in the third quadrant, indicating that their false rejection rate and false acceptance rate were both at a low level. Although ROC curves and DET curves can be used to evaluate the predictive power of GBDT models, it is necessary to further evaluate the clinical benefits of patients after using this predictive model. Therefore, we plotted a clinical decision curve to assess the benefit of using the GBDT model to predict CLNM at different threshold probabilities. Our results show that patients can benefit more from the GBDT model predicting CLNM when the threshold probability is 0.1–1.0.
Previous studies have used machine learning methods to predict lymph node status for PTC (40,41), but the “black box” problem has made these models less clinically interpretable. In our study, visual analysis of the GBDT model from global and local levels via SHAP plots provides a visual interpretation of individual patients, including positive and negative effects. The GBDT model is not only able to predict CLNM, but can also make reasonable explanations for the decision-making process, which may improve the clinical utility of the model.
The study had several limitations. First, this study was conducted by a single center with the small sample size. Second, even though gene mutations are related to lymph node metastasis, not all patients in this study underwent BRAF mutation prior to surgery. Therefore, the effect of BRAF gene mutations on CLNM requires further investigation. Third, there were no cases of capsular invasion in the present study. Fourth, the differences in the radiomics features of tumors and surrounding tissues which may yield information about tumor aggressiveness and CLNM were not analyzed. Fifth, tumor location in the thyroid gland that could be impact on CLNM were not considered for analysis. Additional research involving a substantial sample size and pertinent data will be requisite.
Conclusions
The GBDT model based on US imaging radiomics and clinical risk factors is superior to conventional US in predicting CLNM in PTMC, which can predict CLNM in PTMC patients and guide clinical decision-making in patient treatment.
Acknowledgments
Funding: This work was supported by
Footnote
Reporting Checklist: The authors have completed the TRIPOD reporting checklist. Available at https://gs.amegroups.com/article/view/10.21037/gs-23-456/rc
Data Sharing Statement: Available at https://gs.amegroups.com/article/view/10.21037/gs-23-456/dss
Peer Review File: Available at https://gs.amegroups.com/article/view/10.21037/gs-23-456/prf
Conflicts of Interest: All authors have completed the ICMJE uniform disclosure form (available at https://gs.amegroups.com/article/view/10.21037/gs-23-456/coif). Y.L. is from Chengdu Zhitu Intelligent Technology Co., Ltd. The other authors have no conflicts of interest to declare.
Ethical Statement: The authors are accountable for all aspects of the work in ensuring that questions related to the accuracy or integrity of any part of the work are appropriately investigated and resolved. This retrospective study was approved by the Ethics Committee of China-Japan Union Hospital of Jilin University (approval number: 20221020033), and the study conformed to the tenets of the Declaration of Helsinki (revised in 2013). The patients or their legal guardians signed a detailed informed consent form before the procedure and the personal information of the participants was protected.
Open Access Statement: This is an Open Access article distributed in accordance with the Creative Commons Attribution-NonCommercial-NoDerivs 4.0 International License (CC BY-NC-ND 4.0), which permits the non-commercial replication and distribution of the article with the strict proviso that no changes or edits are made and the original work is properly cited (including links to both the formal publication through the relevant DOI and the license). See: https://creativecommons.org/licenses/by-nc-nd/4.0/.
References
- Sung H, Ferlay J, Siegel RL, et al. Global Cancer Statistics 2020: GLOBOCAN Estimates of Incidence and Mortality Worldwide for 36 Cancers in 185 Countries. CA Cancer J Clin 2021;71:209-49. [Crossref] [PubMed]
- Chen W, Zheng R, Baade PD, et al. Cancer statistics in China, 2015. CA Cancer J Clin 2016;66:115-32. [Crossref] [PubMed]
- Lamartina L, Leboulleux S, Borget I, et al. Global thyroid estimates in 2020. Lancet Diabetes Endocrinol 2022;10:235-6. [Crossref] [PubMed]
- Yang Z, Heng Y, Qiu W, et al. Cervical Lymph Node Metastasis Differences in Patients with Unilateral or Bilateral Papillary Thyroid Microcarcinoma: A Multi-Center Analysis. J Clin Med 2022;11:4929. [Crossref] [PubMed]
- Mehanna H, Al-Maqbili T, Carter B, et al. Differences in the recurrence and mortality outcomes rates of incidental and nonincidental papillary thyroid microcarcinoma: a systematic review and meta-analysis of 21 329 person-years of follow-up. J Clin Endocrinol Metab 2014;99:2834-43. [Crossref] [PubMed]
- Cho SY, Lee TH, Ku YH, et al. Central lymph node metastasis in papillary thyroid microcarcinoma can be stratified according to the number, the size of metastatic foci, and the presence of desmoplasia. Surgery 2015;157:111-8. [Crossref] [PubMed]
- Zhao Q, Ming J, Liu C, et al. Multifocality and total tumor diameter predict central neck lymph node metastases in papillary thyroid microcarcinoma. Ann Surg Oncol 2013;20:746-52. [Crossref] [PubMed]
- Zheng X, Peng C, Gao M, et al. Risk factors for cervical lymph node metastasis in papillary thyroid microcarcinoma: a study of 1,587 patients. Cancer Biol Med 2019;16:121-30. [Crossref] [PubMed]
- Liu XN, Duan YS, Yue K, et al. The optimal extent of lymph node dissection in N1b papillary thyroid microcarcinoma based on clinicopathological factors and preoperative ultrasonography. Gland Surg 2022;11:1047-56. [Crossref] [PubMed]
- Wen X, Jin Q, Cen X, et al. Clinicopathologic predictors of central lymph node metastases in clinical node-negative papillary thyroid microcarcinoma: a systematic review and meta-analysis. World J Surg Oncol 2022;20:106. [Crossref] [PubMed]
- Haugen BR, Alexander EK, Bible KC, et al. 2015 American Thyroid Association Management Guidelines for Adult Patients with Thyroid Nodules and Differentiated Thyroid Cancer: The American Thyroid Association Guidelines Task Force on Thyroid Nodules and Differentiated Thyroid Cancer. Thyroid 2016;26:1-133. [Crossref] [PubMed]
- Liu J, Fan XF, Yang M, et al. Analysis of the risk factors for central lymph-node metastasis of cN0 papillary thyroid microcarcinoma: A retrospective study. Asian J Surg 2022;45:1525-9. [Crossref] [PubMed]
- Barczyński M, Konturek A, Stopa M, et al. Prophylactic central neck dissection for papillary thyroid cancer. Br J Surg 2013;100:410-8. [Crossref] [PubMed]
- Hartl DM, Mamelle E, Borget I, et al. Influence of prophylactic neck dissection on rate of retreatment for papillary thyroid carcinoma. World J Surg 2013;37:1951-8. [Crossref] [PubMed]
- Ryu IS, Song CI, Choi SH, et al. Lymph node ratio of the central compartment is a significant predictor for locoregional recurrence after prophylactic central neck dissection in patients with thyroid papillary carcinoma. Ann Surg Oncol 2014;21:277-83. [Crossref] [PubMed]
- Yu Y, Yu Z, Li M, et al. Model development to predict central lymph node metastasis in cN0 papillary thyroid microcarcinoma by machine learning. Ann Transl Med 2022;10:892. [Crossref] [PubMed]
- Aydin OU, Soylu L, Ozbas S, et al. The risk of hypoparathyroidism after central compartment lymph node dissection in the surgical treatment of pT1, N0 thyroid papillary carcinoma. Eur Rev Med Pharmacol Sci 2016;20:1781-7.
- Lee DH, Kim YK, Yu HW, et al. Computed Tomography for Detecting Cervical Lymph Node Metastasis in Patients Who Have Papillary Thyroid Microcarcinoma with Tumor Characteristics Appropriate for Active Surveillance. Thyroid 2019;29:1653-9. [Crossref] [PubMed]
- Xing Z, Qiu Y, Yang Q, et al. Thyroid cancer neck lymph nodes metastasis: Meta-analysis of US and CT diagnosis. Eur J Radiol 2020;129:109103. [Crossref] [PubMed]
- Liu X, Ouyang D, Li H, et al. Papillary thyroid cancer: dual-energy spectral CT quantitative parameters for preoperative diagnosis of metastasis to the cervical lymph nodes. Radiology 2015;275:167-76. [Crossref] [PubMed]
- Kim SK, Woo JW, Park I, et al. Computed Tomography-Detected Central Lymph Node Metastasis in Ultrasonography Node-Negative Papillary Thyroid Carcinoma: Is It Really Significant? Ann Surg Oncol 2017;24:442-9. [Crossref] [PubMed]
- Yang SY, Shin JH, Hahn SY, et al. Comparison of ultrasonography and CT for preoperative nodal assessment of patients with papillary thyroid cancer: diagnostic performance according to primary tumor size. Acta Radiol 2020;61:21-7. [Crossref] [PubMed]
- Agyekum EA, Ren YZ, Wang X, et al. Evaluation of Cervical Lymph Node Metastasis in Papillary Thyroid Carcinoma Using Clinical-Ultrasound Radiomic Machine Learning-Based Model. Cancers (Basel) 2022;14:5266. [Crossref] [PubMed]
- Peng Y, Zhang ZT, Wang TT, et al. Prediction of Central Lymph Node Metastasis in cN0 Papillary Thyroid Carcinoma by CT Radiomics. Acad Radiol 2023;30:1400-7. [Crossref] [PubMed]
- Wen Q, Wang Z, Traverso A, et al. A radiomics nomogram for the ultrasound-based evaluation of central cervical lymph node metastasis in papillary thyroid carcinoma. Front Endocrinol (Lausanne) 2022;13:1064434. [Crossref] [PubMed]
- Zhou SC, Liu TT, Zhou J, et al. An Ultrasound Radiomics Nomogram for Preoperative Prediction of Central Neck Lymph Node Metastasis in Papillary Thyroid Carcinoma. Front Oncol 2020;10:1591. [Crossref] [PubMed]
- Luo QW, Gao S, Lv X, et al. A novel tool for predicting the risk of central lymph node metastasis in patients with papillary thyroid microcarcinoma: a retrospective cohort study. BMC Cancer 2022;22:606. [Crossref] [PubMed]
- Huang C, Cong S, Shang S, et al. Web-Based Ultrasonic Nomogram Predicts Preoperative Central Lymph Node Metastasis of cN0 Papillary Thyroid Microcarcinoma. Front Endocrinol (Lausanne) 2021;12:734900. [Crossref] [PubMed]
- American Thyroid Association Surgery Working Group. Consensus statement on the terminology and classification of central neck dissection for thyroid cancer. Thyroid 2009;19:1153-8. [Crossref] [PubMed]
- Leenhardt L, Erdogan MF, Hegedus L, et al. 2013 European thyroid association guidelines for cervical ultrasound scan and ultrasound-guided techniques in the postoperative management of patients with thyroid cancer. Eur Thyroid J 2013;2:147-59. [Crossref] [PubMed]
- Ullmann TM, Papaleontiou M, Sosa JA. Current Controversies in Low-Risk Differentiated Thyroid Cancer: Reducing Overtreatment in an Era of Overdiagnosis. J Clin Endocrinol Metab 2023;108:271-80. [Crossref] [PubMed]
- Chou R, Dana T, Haymart M, et al. Active Surveillance Versus Thyroid Surgery for Differentiated Thyroid Cancer: A Systematic Review. Thyroid 2022;32:351-67. [Crossref] [PubMed]
- Cai YF, Wang QX, Ni CJ, et al. A scoring system is an effective tool for predicting central lymph node metastasis in papillary thyroid microcarcinoma: a case-control study. World J Surg Oncol 2016;14:45. [Crossref] [PubMed]
- Ietto G, Amico F, Soldini G, et al. Real-time Intraoperative Fluorescent Lymphography: A New Technique for Lymphatic Sparing Surgery. Transplant Proc 2016;48:3073-8. [Crossref] [PubMed]
- Wang Z, Chang Q, Zhang H, et al. A Clinical Predictive Model of Central Lymph Node Metastases in Papillary Thyroid Carcinoma. Front Endocrinol (Lausanne) 2022;13:856278. [Crossref] [PubMed]
- Oh HS, Park S, Kim M, et al. Young Age and Male Sex Are Predictors of Large-Volume Central Neck Lymph Node Metastasis in Clinical N0 Papillary Thyroid Microcarcinomas. Thyroid 2017;27:1285-90. [Crossref] [PubMed]
- Kim KN, Hwang Y, Kim KH, et al. Adolescent overweight and obesity and the risk of papillary thyroid cancer in adulthood: a large-scale case-control study. Sci Rep 2020;10:5000. [Crossref] [PubMed]
- Hall M, Frank E, Holmes G, et al. The WEKA data mining software: an update. SIGKDD Explor Newsl 2009;11:10-8.
- Kim SY, Lee E, Nam SJ, et al. Ultrasound texture analysis: Association with lymph node metastasis of papillary thyroid microcarcinoma. PLoS One 2017;12:e0176103. [Crossref] [PubMed]
- Li J, Wu X, Mao N, et al. Computed Tomography-Based Radiomics Model to Predict Central Cervical Lymph Node Metastases in Papillary Thyroid Carcinoma: A Multicenter Study. Front Endocrinol (Lausanne) 2021;12:741698. [Crossref] [PubMed]
- Yu J, Deng Y, Liu T, et al. Lymph node metastasis prediction of papillary thyroid carcinoma based on transfer learning radiomics. Nat Commun 2020;11:4807. [Crossref] [PubMed]