A nomogram based on ultrasound radiomics for predicting the invasiveness of cN0 single papillary thyroid microcarcinoma
Highlight box
Key findings
• Nomogram based on thyroid ultrasound (US) radiomics has an excellent predictive value of the potential invasiveness of a single papillary thyroid microcarcinoma (PTMC) without clinical lymph node metastasis.
What is known and what is new?
• Conventional US focuses on the characteristics of tumor capsule and the periphery to determine whether the tumor has invasive growth.
• There is a strong correlation between US radiomics features and the invasiveness of PTMC. And the nomogram that combines radiomics features with clinical features can objectively and accurately predict the invasiveness of a single cN0 PTMC.
What is the implication, and what should change now?
• The prediction model provides the basis for the clinical treatment and prognosis of simple cN0 PTMC. The ability to capture invasive PTMC in the early stages would definitely improve the prognosis and justify an early definitive thyroid lobectomy.
Introduction
In recent years, the Surveillance, Epidemiology, and End Results (SEER) database (1,2) has shown that the prevalence of thyroid cancer has increased significantly, most commonly being papillary thyroid microcarcinoma (PTMC), which is defined as PTC with diameter less than 1 cm in size according to World Health Organization (WHO). More than 50% of the newly diagnosed thyroid cancer cases are PTMC, and its growth rate is the fastest. Despite its size, some tumors are more invasive and might spread well beyond the thyroid capsule causing extrathyroidal extension, regional or distant metastasis, and can even result in death (3,4). Local and international consensus is that active surveillance strategies can be adopted for non-invasive PTMC. However, highly invasive PTMC should be fully recognized and regulated in clinical work as it accounts for higher morbidity and mortality rate.
Ultrasound (US), as the first line of investigation for the examination of PTMC, is helpful in the detection of PTMC, but it cannot easily predict the invasiveness of PTMC. Previous research revealed that preoperative ultrasonography was not sensitive in detecting lymph node metastasis in the central neck; up to 15.3% of PTMC patients with negative clinical lymph node metastasis (cN0) were confirmed to have pathological lymph node metastasis in level VI (5,6). Conventional US focuses on the characteristics of tumor capsule and the periphery to determine whether the tumor has invasive growth. However, due to its small size, the typical features of invasiveness shown by conventional 2-dimensional (2D) US are not well visualized. Most PTMCs are single lesions and would rarely have regional or even lymph node metastasis at the time of presentation, the ability to capture invasive PTMC in the early stages would definitely improve the prognosis and justify an early definitive thyroid lobectomy.
Radiomics makes use of artificial intelligence and big data to build a model that can help improving diagnostic accuracy and providing prognostic implication of the disease (7,8). In recent years, US-based radiomics has developed rapidly and has been gradually applied to the differentiate between a benign and malignant thyroid nodule (9), and to evaluate the level of their invasiveness (10,11). However, so far there are only limited studies focusing on the invasiveness of a single cN0 PTMC. This study aimed to construct an US-based radiomics nomogram that combines radiomics features with clinical features to predict the invasiveness of a single cN0 PTMC. We hope to provide a basis for clinical treatment and prognosis of a single cN0 PTMC. We present this article in accordance with the TRIPOD reporting checklist (available at https://gs.amegroups.com/article/view/10.21037/gs-23-473/rc).
Methods
Patient selection
This study retrospectively analyzed 317 patients with newly diagnosed cN0 PTMC who underwent surgery at Ningbo No.2 Hospital, from May 2019 to December 2021. In addition to absence of clinical palpable cervical lymph node, cN0 is further confirmed by ultrasonography, i.e., those with the following findings were excluded: transverse/long diameter of cervical lymph node >0.5 cm, the unclear boundary of cortical medulla or disappearance of medulla structure, gravel calcification or cystic degeneration similar to the primary focus, hyperechoic mass in the cortex, or abundant or irregular blood flow around the cortex (12).
The inclusion criteria are as follows: (I) newly treated patients with single PTMC confirmed by postoperative pathology; (II) patients meeting the diagnostic criteria of cN0; (III) thyroid US examination 1 week before operation was available for review; (IV) the scope of surgical resection included at least the affected side gland and central lymph node. The exclusion criteria are as follows: (I) preoperative evaluation of cN1; (II) bilateral or multifocal cancer; (III) poor or missing US images or other incomplete clinical data; (IV) patients with concurrent malignancies; (V) patients with a prior history of chemotherapy and radiotherapy.
The study was conducted in accordance with the Declaration of Helsinki (as revised in 2013). This study was approved by the Research Ethics Committee of Ningbo No.2 Hospital (Ningbo, China) (No. PJ-NBEY-KY-2019-150-01). The informed consent requirement was waived due to the retrospective nature of this study.
Clinical data
Demographics including gender, age, body mass index (BMI, kg/m2), blood tests [thyroid stimulating hormone (TSH), thyroglobulin (TG) level], ultrasonography (USG) findings (size, location of the PTMC), past medical history, notably Hashimoto’s thyroiditis (HT) were obtained from the consultation notes. PTMC was further divided into an invasive group or a non-invasive group according to the pathological report, based on the presence of extrathyroidal extension and/or lymph node metastasis (13).
Standard ultrasonic image acquisition
MyLab90 color Doppler US diagnostic system (Esaote S.p.A., Genova, Italy) was used, equipped with a LA523 probe (4–13 MHz). Patients were asked to lie in a supine position with head tilted for full exposure of the anterior neck. Thyroid examination conditions of the superficial organs preset by the machine were selected. The gain, depth, dynamic range, and focus area were adjusted in order to obtain the optimal quality. Targeted lesions were studied in multiple sections, and the images were stored in the workstation in Digital Imaging and Communications in Medicine (DICOM) format. Of note, the US examinations were carried out by radiologists with more than 5 years of experience.
Region of interest segmentation and feature extraction
The ultrasonic DICOM image data was imported into ITK-SNAP (version 3.8; http://www.ITKSNAP.org). The region of interest (ROI) was manually drawn on the gray US image of the largest section PTMC. The intention was to include the whole thyroid cancer lesion in the ROI. All segmentation was performed by two radiologists, of which one of them repeat the procedure twice to confirm the validity. They were both blinded from the pathological results of the surgery and have more than 5 years of clinical experience in thyroid nodule ultrasonography. Gray standardization, discretization processing, and image resampling were then applied to the image before drawing the radiomics features automatically using the Pyradiomics software (https://www.radiomics.io/pyradiomics.html) package (v3.0). Targeted features include those reflecting phenotypic characteristics, e.g., texture, morphology, intensity, Laplacian of Gaussian, and wavelet. These radiomics features followed the Image Biomarker Standardization Initiative (IBSI; https://ibsi.readthedocs.io/en/latest/). The interclass correlation coefficient (ICC) was used to evaluate inter- and intra-observer consistency in the aforementioned data collection process. It is ensured that only the radiomics features with ICC >0.75, would be selected to construct radiomics signature.
Feature selection and radiomics signature construction
The patients were randomly assigned to the training and testing dataset according to the proportion of 8:2. The extracted radiomics feature data were normalized by the Z-score method, and the features were screened using minimum redundancy maximum relevance (mRMR) and least absolute shrinkage and selection operator (LASSO) regression. Features with non-zero coefficients were verified by 10 times cross-validation hyperparameters, and the selected optimal features were used to construct a radiomics signature. Finally, the radiomics score (Rad-score) of each patient was calculated by the Rad-score calculation formula in the official PyRadiomics document. The receiver operating characteristic (ROC) curve evaluated the radiomics signature’s performance, and the radiomics signature was verified in the testing data.
The establishment of the US radiomics nomogram
Clinical information and radiomics Rad-score were statistically analyzed by Student’s t-test (continuous variables) and Chi-squared test (categorical variables). The selected risk factors were further analyzed by multivariate logistic regression. With free P value less than 0.05 as the retention criterion, the potential predictors of PTMC invasiveness were identified by backward step-by-step selection method. Then the radiomics signature were combined with clinical risk factors to establish a radiomics nomogram.
Performance of the US radiomics nomogram
ROC curves of radiomics nomogram were plotted in training and testing data. Area under the curve (AUC) was used to evaluate the discriminatory performance of the radiomics nomogram. Calibration curves were used to evaluate the validity of the radiomics nomogram. Decision curve analysis (DCA) was used to evaluate the clinical value of the radiomics nomogram.
Statistical analysis
The SPSS 26.0 software (IBM Company, Armonk, NY, USA) was used for statistical analysis. The continuous data conforming to the normal distribution were expressed as mean (standard deviation) and analyzed by independent sample t-test. The categorical data were represented by n (%) and analyzed by Chi-square test or Fisher’s exact test. The Mann-Whitney U test was used to compare the serial data. In multivariate logistic analysis, invasiveness was taken as the dependent variable, and the factors with statistically significant differences in univariate analysis were taken as independent variables. Machine learning methods and radiomics were implemented using the Scikit-Learn package of Python 3.8.1 (https://www.python.org/downloads/release/python-381/). The nomogram and calibration curves of the diagnostic model were plotted using the matplotlib package for Python 3.8.1. DCA assesses the clinical validity of the model by quantifying the net benefit under different threshold probabilities. A P value <0.05 was considered statistically significant.
Results
Clinical characteristics
A total of 317 patients were included in this study. According to the operation and pathology results, there were 130 cases in the invasive group and 187 cases in the non-invasive group. The patients were randomly divided into a training dataset (n=254) and a testing dataset (n=63) in a 4:1 ratio. There was no significant difference in clinical features, invasiveness, and Rad-score between the two groups (all P>0.05), as shown in Table 1.
Table 1
Characteristics | Training dataset (n=254) | Testing dataset (n=63) | P |
---|---|---|---|
Age (years), mean (SD) | 44.6 (11.7) | 45 (12.5) | 0.854 |
BMI (kg/m2), mean (SD) | 23.3 (3.0) | 23.4 (3.1) | 0.696 |
TG (ng/mL), mean (SD) | 14.1 (31.1) | 10.1 (11.2) | 0.266 |
TSH (mU/L), mean (SD) | 2.1 (4.2) | 1.8 (1.0) | 0.539 |
Diameter (mm), mean (SD) | 5.5 (2.2) | 5.2 (2.1) | 0.405 |
Gender, n (%) | 0.669 | ||
Female | 201 (79.1) | 52 (82.5) | |
Male | 53 (20.9) | 11 (17.5) | |
HT, n (%) | >0.99 | ||
No | 200 (78.7) | 50 (79.4) | |
Yes | 54 (21.3) | 13 (20.6) | |
Location, n (%) | 0.225 | ||
Upper | 58 (22.8) | 15 (23.8) | |
Middle | 81 (31.9) | 12 (19.0) | |
Lower | 81 (31.9) | 25 (39.7) | |
Isthmus | 34 (13.4) | 11 (17.5) | |
Invasiveness, n (%) | 0.963 | ||
No | 150 (59.1) | 37 (58.7) | |
Yes | 104 (40.9) | 26 (41.3) | |
Rad-score, median [IQR] | −0.6 [−0.9, 0.0] | −0.4 [−0.8, 0.1] | 0.50904 |
SD, standard deviation; BMI, body mass index; TG, thyroglobulin; TSH, thyroid stimulating hormone; HT, Hashimoto’s thyroiditis; IQR, interquartile range.
Univariate analysis showed that gender, size/location of the lesion, and Rad-score were significantly correlated in the training data. According to the multivariate logical analysis, there was a significant correlation among gender [odds ratio (OR): 2.030, 95% confidence interval (CI): 1.101–3.742, P=0.023], lesion diameter (OR: 1.142, 95% CI: 1.014–1.286, P=0.029), lesion location (OR: 1.297, 95% CI: 1.009–1.666, P=0.042), and Rad-score (OR: 4.480, 95% CI: 2.720–7.390, P<0.001). It is regarded as an independent predictor of the PTMC invasiveness prediction model, as shown in Table 2.
Table 2
Characteristics | Univariate analysis | Multivariate logistic regression | |||||
---|---|---|---|---|---|---|---|
Negative (n=150) | Positive (n=104) | P | Beta | 95% CI | P | ||
Age (years), mean (SD) | 44.9 (11.3) | 44.2 (12.4) | 0.655 | ||||
BMI (kg/m2), mean (SD) | 23.2 (3.0) | 22.9 (3.1) | 0.386 | ||||
TG (ng/mL), mean (SD) | 13.5 (31.8) | 11.8 (19.5) | 0.671 | ||||
TSH (mU/L), mean (SD) | 2.3 (4.9) | 1.7 (0.9) | 0.225 | ||||
Diameter (mm), mean (SD) | 5 (2.0) | 5.6 (2.3) | 0.027* | 1.142 | 1.014–1.286 | 0.029* | |
Gender, n (%) | 0.033* | 2.030 | 1.101–3.742 | 0.023* | |||
Female | 126 (84.0) | 75 (72.1) | |||||
Male | 24 (16.0) | 29 (27.9) | |||||
HT, n (%) | >0.99 | ||||||
No | 118 (78.7) | 82 (78.8) | |||||
Yes | 32 (21.3) | 22 (21.2) | |||||
Location, n (%) | 0.011* | 1.297 | 1.009–1.666 | 0.042* | |||
Upper | 40 (26.7) | 14 (13.5) | |||||
Middle | 44 (29.3) | 34 (32.7) | |||||
Lower | 43 (28.7) | 46 (44.2) | |||||
Isthmus | 23 (15.3) | 10 (9.6) | |||||
Rad-score, median [IQR] | −0.6 [−1.1, −0.2] | 0 [−0.4, 0.2] | <0.001* | 4.480 | 2.720–7.390 | <0.001* |
*, P<0.05. CI, confidence interval; SD, standard deviation; BMI, body mass index; TG, thyroglobulin; TSH, thyroid stimulating hormone; HT, Hashimoto’s thyroiditis; IQR, interquartile range.
Feature selection and radiomics signature construction
A total of 816 features were drawn from original gray-scale ultrasonic images using the Pyradiomics software package. The ICCs of intra- and inter-observer reproducibility was 0.755–0.871 and 0.769–0.869, respectively. Seven features were selected after screened with mRMR and LASSO.
The radiomics score formula was generated based on these characteristics and their corresponding regression coefficients. The formula is as follows:
The Rad-score of each patient was calculated according to the above formula. The distribution and probability density of the Rad-score in the two data were more clearly shown by the box chart, as shown in Figure 1. The predictive results of the radiomics signature were analyzed by the ROC curve. The AUC of the radiomics signature in the training and testing group was 0.74 (95% CI: 0.68–0.80) and 0.73 (95% CI: 0.60–0.85).
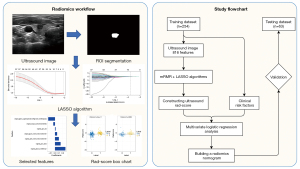
The establishment of the radiomics nomogram
Combined with clinical risk factors and radiomics signature, a nomogram based on US radiomics was constructed to predict the invasiveness of cN0 single PTMC, as shown in Figure 2. The prediction would be analyzed by the ROC curve and assessed using the AUC. The AUC in the training and testing group was 0.76 (95% CI: 0.71–0.82), 0.71 (95% CI: 0.58–0.84), as shown in Figure 3.
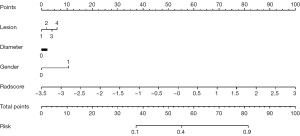
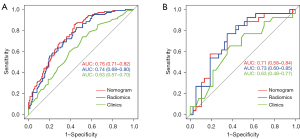
Clinical significance of US radiomics nomogram
In the training dataset, the nomogram’s accuracy, sensitivity, and specificity were 0.811, 0.914, and 0.727, respectively. The accuracy, sensitivity, and specificity of the testing dataset were 0.841, 0.533, and 0.868, respectively, as shown in Table 3. The calibration curve showed that the nomogram prediction curve aligned with the standard curve in the training and testing datasets, as shown in Figure 4. The DCA curve illustrated that the nomogram was more effective than the whole (all PTMC were considered invasive before surgery) or none (all PTMC were considered non-invasive before surgery) strategy when the threshold was 0.1–0.95, as shown in Figure 5.
Table 3
Predictive model | Training dataset | Testing dataset | |||||||
---|---|---|---|---|---|---|---|---|---|
Accuracy | Sensitivity | Specificity | AUC | Accuracy | Sensitivity | Specificity | AUC | ||
Clinical model | 0.737 | 0.914 | 0.722 | 0.63 | 0.646 | 0.733 | 0.638 | 0.63 | |
Radiomics signature | 0.789 | 0.714 | 0.796 | 0.74 | 0.646 | 0.800 | 0.632 | 0.73 | |
Radiomics nomogram | 0.811 | 0.914 | 0.727 | 0.76 | 0.841 | 0.533 | 0.868 | 0.71 |
AUC, area under the curve.
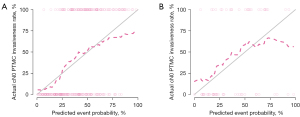
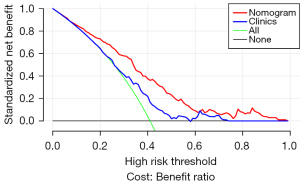
Discussion
Despite its small size, invasive PTMC progress rapidly, causing regional and even distant metastasis, therefore justifying an upfront surgical resection for curative purposes (14). On the other side of the spectrum, non-invasive PTMC is usually stable, it grows very slowly or even do not grow at all (15). Ability to differentiate between an invasive PTMC or not would definitely improve the prognosis and also survival rate (16,17). In addition, it would help avoid unnecessary surgery with diagnostic intent and avoid the potential surgical complication including vocal cord palsy, keloid scar changes. Therefore, the correct evaluation of the invasiveness of PTMC in the first place is critical to make an appropriate and personalized treatment plan.
Ultrasonography is the most commonly used method to evaluate the invasiveness of PTMC. Routine ultrasonographic examination of the neck can evaluate the size, boundary, shape, internal echo, calcification, multiple foci, extrathyroidal extension, and lymph node metastasis of thyroid nodules and indirectly predict the invasiveness of PTMC. The 2015 American Thyroid Association (ATA) guidelines for the diagnosis and treatment of thyroid nodule and differentiated thyroid carcinoma points out that multiple foci extrathyroidal extension, and lymph node metastasis are the characteristics of an invasive PTMC (7). For most single focus PTMC without clinical lymph nodes metastasis, their volumes are small and insufficient to assess the invasiveness of PTMC based on the unclear boundary and interrupted echo of the thyroid capsule (18). Conventional US, also is operator dependent and subject to variation, is easily affected by operator’s subjective factors in the scanning process. As such, methods to identify and assess the level of invasiveness of a single PTMC without clinical palpable neck lymph node has always been a challenging topic in the research (19).
US radiomics can quantitatively extract radiomics features from US images with high throughput and convert US images into minable data for analysis. Being a non-invasive investigation tool, radiomics in the same time can void the sampling error in tissue biopsy, and can accurately and quantitatively analyze the whole tumor, provide a new basis for personalized treatment (20-22). In this study, the invasiveness of PTMC was predicted based on the US radiomics signature of its primary foci. In the training and testing data, the AUC values of radiomics signature were 0.74 (95% CI: 0.68–0.80) and 0.73 (95% CI: 0.60–0.85), respectively. It is consistent with the results of many previous studies. Park et al. (23) retrospectively analyzed 730 radiomics features in the primary images of 768 patients with PTC and finally selected 14 feature sets to show that primary imaging could predict cervical lymph node metastasis. The AUC in the training and testing data were 0.710 and 0.621, respectively. Another study (24) retrospectively analyzed the radiomics characteristics of focus US images of 886 patients with PTC and randomly divided them into a training cohort (n=600) and a verification cohort (n=286). The results showed that the radiomics features were significantly correlated with the lateral neck lymph node metastasis in both cohorts (P<0.001).
Among the seven radiomics features screened out at the end of this study, two features come from gray-level cooccurrence matrix (GLCM), two from gray-level size zone matrix (GLSZM), one from gray-level difference matrix (GLDM), and one from gray-level run-length (GLRLM). All of them are texture feature subsets. Among the selected radiomics features, wavelet-based texture features account for the vast majority, indicating that texture features play a good role in classification. There is a strong correlation between ultrasonic quantitative features and biological features by imaging. Radiomics can comprehensively represent tumors and reveal the predictive correlation between images and medical results (25-28).
Previous studies have found that male sex (29), diameter >5 mm (30), and multifocal (31) are associated with the invasiveness of PTMC. This study showed that males, larger diameter, and inferior pole location were independent predictors of PMTC invasiveness in single PTMC with negative clinical lymph node metastasis. Some studies have shown that a radiomics nomogram was better than a radiomics signature in predicting lymph node metastasis (32,33). Therefore, a nomogram combined with the radiomics signature and clinical features was constructed in this study. In the training dataset and testing dataset, the AUC values of the nomogram were 0.76 (95% CI: 0.71–0.82) and 0.71 (95% CI: 0.58–0.84), respectively. In the training dataset, the AUC of the nomogram was higher than the AUC value of the radiomics signature. The diagnostic efficiency of the model was better in both the training and testing datasets. This showed that there was no significant difference between the predicted invasion and the final pathology in the two groups, and that it has a good calibration ability. Finally, the DCA showed that the nomogram has a high clinical application value.
The limitations of this study are as follows: (I) it was a retrospective study with small sample size and this is a single center study; (II) the largest US image section may not contain the most representative features of PTMC. It also needs to be verified by 3-dimensional image group analysis; (III) the recording of ROI was carried out manually, which has low efficiency, and there may have been a deviation between different descriptors, which affects the diagnostic efficiency of the model, so it is necessary to optimize the accuracy of automatic recording further.
Conclusions
The nomogram based on preoperative thyroid US radiomics shows promising results in detecting invasive cN0 single PTMC. It could be the potential missing piece as the imaging marker, to allow personalized medicine for these patients. While watchful waiting can be the treatment approach for non-invasive cN0 single PTMC, an upfront surgical resection or even lobectomy could be considered for the invasive cN0 single PTMC. The nomogram we made can potentially be the imaging marker in such cases. Hopefully with ongoing advancement of artificial intelligence and big data, the precision and accuracy of our clinical judgement can be stepped up.
Acknowledgments
Ling Ma, MD, is acknowledged for providing radiomics analysis of ultrasound images, and Jianjun Zheng, the chief radiologist in the authors’ hospital, is acknowledged for building a research platform on radiomics, and for his support and help for the research on ultrasound radiomics. We thank Dr. Giuseppe Cavallaro (Department of Medico-Surgical Sciences and Biotechnologies, Sapienza University, Rome, Italy) for the critical comments and valuable advice on this study.
Funding: The study was supported by
Footnote
Reporting Checklist: The authors have completed the TRIPOD reporting checklist. Available at https://gs.amegroups.com/article/view/10.21037/gs-23-473/rc
Data Sharing Statement: Available at https://gs.amegroups.com/article/view/10.21037/gs-23-473/dss
Peer Review File: Available at https://gs.amegroups.com/article/view/10.21037/gs-23-473/prf
Conflicts of Interest: All authors have completed the ICMJE uniform disclosure form (available at https://gs.amegroups.com/article/view/10.21037/gs-23-473/coif). The authors have no conflicts of interest to declare.
Ethical Statement: The authors are accountable for all aspects of the work in ensuring that questions related to the accuracy or integrity of any part of the work are appropriately investigated and resolved. The study was conducted in accordance with the Declaration of Helsinki (as revised in 2013). This study was approved by the Research Ethics Committee of Ningbo No.2 Hospital (Ningbo, China) (No. PJ-NBEY-KY-2019-150-01). The informed consent requirement was waived due to the retrospective nature of this study.
Open Access Statement: This is an Open Access article distributed in accordance with the Creative Commons Attribution-NonCommercial-NoDerivs 4.0 International License (CC BY-NC-ND 4.0), which permits the non-commercial replication and distribution of the article with the strict proviso that no changes or edits are made and the original work is properly cited (including links to both the formal publication through the relevant DOI and the license). See: https://creativecommons.org/licenses/by-nc-nd/4.0/.
References
- Siegel RL, Miller KD, Jemal A. Cancer statistics, 2020. CA Cancer J Clin 2020;70:7-30. [Crossref] [PubMed]
- Lee EK, Moon JH, Hwangbo Y, et al. Progression of Low-Risk Papillary Thyroid Microcarcinoma During Active Surveillance: Interim Analysis of a Multicenter Prospective Cohort Study of Active Surveillance on Papillary Thyroid Microcarcinoma in Korea. Thyroid 2022;32:1328-36. [Crossref] [PubMed]
- Samà MT, Grosso E, Mele C, et al. Molecular characterisation and clinical correlation of papillary thyroid microcarcinoma. Endocrine 2021;71:149-57. [Crossref] [PubMed]
- Al-Qurayshi Z, Nilubol N, Tufano RP, et al. Wolf in Sheep's Clothing: Papillary Thyroid Microcarcinoma in the US. J Am Coll Surg. 2020;230:484-91. [Crossref] [PubMed]
- Zhang Q, Wang Z, Meng X, et al. Predictors for central lymph node metastases in CN0 papillary thyroid microcarcinoma (mPTC): A retrospective analysis of 1304 cases. Asian J Surg 2019;42:571-6. [Crossref] [PubMed]
- Choi SM, Kim JK, Lee CR, et al. Completion Total Thyroidectomy Is Not Necessary for Papillary Thyroid Microcarcinoma with Occult Central Lymph Node Metastasis: A Long-Term Serial Follow-Up. Cancers (Basel) 2020;12:3032. [Crossref] [PubMed]
- Gillies RJ, Kinahan PE, Hricak H. Radiomics: Images Are More than Pictures, They Are Data. Radiology 2016;278:563-77. [Crossref] [PubMed]
- Lambin P, Rios-Velazquez E, Leijenaar R, et al. Radiomics: extracting more information from medical images using advanced feature analysis. Eur J Cancer 2012;48:441-6. [Crossref] [PubMed]
- Li X, Zhang S, Zhang Q, et al. Diagnosis of thyroid cancer using deep convolutional neural network models applied to sonographic images: a retrospective, multicohort, diagnostic study. Lancet Oncol 2019;20:193-201. [Crossref] [PubMed]
- Jiang M, Li C, Tang S, et al. Nomogram Based on Shear-Wave Elastography Radiomics Can Improve Preoperative Cervical Lymph Node Staging for Papillary Thyroid Carcinoma. Thyroid 2020;30:885-97. [Crossref] [PubMed]
- Wu Y, Rao K, Liu J, et al. Machine Learning Algorithms for the Prediction of Central Lymph Node Metastasis in Patients With Papillary Thyroid Cancer. Front Endocrinol (Lausanne) 2020;11:577537. [Crossref] [PubMed]
- Podnos YD, Smith D, Wagman LD, et al. The implication of lymph node metastasis on survival in patients with well-differentiated thyroid cancer. Am Surg 2005;71:731-4. [Crossref] [PubMed]
- Sugitani I, Ito Y, Miyauchi A, et al. Active Surveillance Versus Immediate Surgery: Questionnaire Survey on the Current Treatment Strategy for Adult Patients with Low-Risk Papillary Thyroid Microcarcinoma in Japan. Thyroid 2019;29:1563-71. [Crossref] [PubMed]
- Huang K, Gao N, Bian D, et al. Associations of BRAF V600E, clinical pathology and imaging factors with the recurrence rate of papillary thyroid microcarcinoma. Exp Ther Med 2020;20:243. [Crossref] [PubMed]
- Chen S, Mao Y, Chen G. Economic effect between surgery and thermal ablation for patients with papillary thyroid microcarcinoma: a systemic review and meta-analysis. Endocrine 2022;76:9-17. [Crossref] [PubMed]
- Dong Y, Wang D, Luo Y, et al. Comprehensive evaluation of risk factors for lymph node metastasis in patients with papillary thyroid carcinoma. Oncol Lett 2021;21:188. [Crossref] [PubMed]
- Bi J, Lyu PF, Wang Y, et al. Survival benefit of active surveillance for papillary thyroid carcinoma: a propensity score matching analysis based on SEER database. Front Oncol 2023;13:1185650. [Crossref] [PubMed]
- Haugen BR, Alexander EK, Bible KC, et al. 2015 American Thyroid Association Management Guidelines for Adult Patients with Thyroid Nodules and Differentiated Thyroid Cancer: The American Thyroid Association Guidelines Task Force on Thyroid Nodules and Differentiated Thyroid Cancer. Thyroid 2016;26:1-133. [Crossref] [PubMed]
- Song Y, Fu LJ, Li HT, et al. Evaluation of MEDAG gene expression in papillary thyroid microcarcinoma: associations with histological features, regional lymph node metastasis and prognosis. Sci Rep 2019;9:5800. [Crossref] [PubMed]
- Laghi A, Voena C. CT-based Radiomics for Biliary Tract Cancer: A Possible Solution for Predicting Lymph Node Metastases. Radiology 2019;290:99-100. [Crossref] [PubMed]
- Feng H, Shi G, Xu Q, et al. Radiomics-based analysis of CT imaging for the preoperative prediction of invasiveness in pure ground-glass nodule lung adenocarcinomas. Insights Imaging 2023;14:24. [Crossref] [PubMed]
- Xia H, Yuan L, Zhao W, et al. Predicting transient ischemic attack risk in patients with mild carotid stenosis using machine learning and CT radiomics. Front Neurol 2023;14:1105616. [Crossref] [PubMed]
- Park VY, Han K, Kim HJ, et al. Radiomics signature for prediction of lateral lymph node metastasis in conventional papillary thyroid carcinoma. PLoS One 2020;15:e0227315. [Crossref] [PubMed]
- Tong Y, Li J, Huang Y, et al. Ultrasound-Based Radiomic Nomogram for Predicting Lateral Cervical Lymph Node Metastasis in Papillary Thyroid Carcinoma. Acad Radiol 2021;28:1675-84. [Crossref] [PubMed]
- Jin X, Ai Y, Zhang J, et al. Noninvasive prediction of lymph node status for patients with early-stage cervical cancer based on radiomics features from ultrasound images. Eur Radiol 2020;30:4117-24. [Crossref] [PubMed]
- Zhang L, Jiang X, Xie X, et al. The Impact of Preoperative Radiomics Signature on the Survival of Breast Cancer Patients With Residual Tumors After NAC. Front Oncol 2020;10:523327. [Crossref] [PubMed]
- Xie J, Li B, Min X, et al. Prediction of Pathological Upgrading at Radical Prostatectomy in Prostate Cancer Eligible for Active Surveillance: A Texture Features and Machine Learning-Based Analysis of Apparent Diffusion Coefficient Maps. Front Oncol 2020;10:604266. [Crossref] [PubMed]
- Zhang Y, Zhu Y, Zhang K, et al. Invasive ductal breast cancer: preoperative predict Ki-67 index based on radiomics of ADC maps. Radiol Med 2020;125:109-16. [Crossref] [PubMed]
- Huang Y, Yin Y, Zhou W. Risk Factors for Central and Lateral Lymph Node Metastases in Patients With Papillary Thyroid Micro-Carcinoma: Retrospective Analysis on 484 Cases. Front Endocrinol (Lausanne) 2021;12:640565. [Crossref] [PubMed]
- Tallini G, De Leo A, Repaci A, et al. Does the Site of Origin of the Microcarcinoma with Respect to the Thyroid Surface Matter? A Multicenter Pathologic and Clinical Study for Risk Stratification. Cancers (Basel) 2020;12:246. [Crossref] [PubMed]
- Zhao L, Li H, He Y, et al. The role of multifocality in predicting central lymph node metastasis in initially treated 18-55 years old female patients with unilateral papillary thyroid microcarcinoma. Front Oncol 2023;13:1197232. [Crossref] [PubMed]
- Zhang J, Zhang Z, Mao N, et al. Radiomics nomogram for predicting axillary lymph node metastasis in breast cancer based on DCE-MRI: A multicenter study. J Xray Sci Technol 2023;31:247-63. [Crossref] [PubMed]
- Chen X, Huang Y, He L, et al. CT-Based Radiomics to Differentiate Pelvic Rhabdomyosarcoma From Yolk Sac Tumors in Children. Front Oncol 2020;10:584272. [Crossref] [PubMed]